We use cookies to give you the best experience possible. By continuing we’ll assume you’re on board with our cookie policy
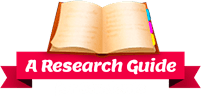
- A Research Guide
- Research Paper Topics

120 Music Research Paper Topics
How to choose a topic for music research paper:.
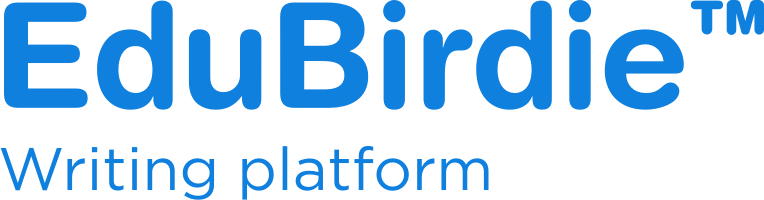
Music Theory Research Paper Topics:
- The influence of harmonic progression on emotional response in music
- Analyzing the use of chromaticism in the compositions of Johann Sebastian Bach
- The role of rhythm and meter in creating musical tension and release
- Examining the development of tonality in Western classical music
- Exploring the impact of cultural and historical context on musical form and structure
- Investigating the use of polyphony in Renaissance choral music
- Analyzing the compositional techniques of minimalist music
- The relationship between melody and harmony in popular music
- Examining the influence of jazz improvisation on contemporary music
- The role of counterpoint in the compositions of Ludwig van Beethoven
- Investigating the use of microtonality in experimental music
- Analyzing the impact of technology on music composition and production
- The influence of musical modes on the development of different musical genres
- Exploring the use of musical symbolism in film scoring
- Investigating the role of music theory in the analysis and interpretation of non-Western music
Music Industry Research Paper Topics:
- The impact of streaming services on music consumption patterns
- The role of social media in promoting and marketing music
- The effects of piracy on the music industry
- The influence of technology on music production and distribution
- The relationship between music and mental health
- The evolution of music genres and their impact on the industry
- The economics of live music events and festivals
- The role of record labels in shaping the music industry
- The impact of globalization on the music industry
- The representation and portrayal of gender in the music industry
- The effects of music streaming platforms on artist revenue
- The role of music education in fostering talent and creativity
- The influence of music videos on audience perception and engagement
- The impact of music streaming on physical album sales
- The role of music in advertising and brand marketing
Music Therapy Research Paper Topics:
- The effectiveness of music therapy in reducing anxiety in cancer patients
- The impact of music therapy on improving cognitive function in individuals with Alzheimer’s disease
- Exploring the use of music therapy in managing chronic pain
- The role of music therapy in promoting emotional well-being in children with autism spectrum disorder
- Music therapy as a complementary treatment for depression: A systematic review
- The effects of music therapy on stress reduction in pregnant women
- Examining the benefits of music therapy in improving communication skills in individuals with developmental disabilities
- The use of music therapy in enhancing motor skills rehabilitation after stroke
- Music therapy interventions for improving sleep quality in patients with insomnia
- Exploring the impact of music therapy on reducing symptoms of post-traumatic stress disorder (PTSD)
- The role of music therapy in improving social interaction and engagement in individuals with schizophrenia
- Music therapy as a non-pharmacological intervention for managing symptoms of dementia
- The effects of music therapy on pain perception and opioid use in hospitalized patients
- Exploring the use of music therapy in promoting relaxation and reducing anxiety during surgical procedures
- The impact of music therapy on improving quality of life in individuals with Parkinson’s disease
Music Psychology Research Paper Topics:
- The effects of music on mood and emotions
- The role of music in enhancing cognitive abilities
- The impact of music therapy on mental health disorders
- The relationship between music and memory recall
- The influence of music on stress reduction and relaxation
- The psychological effects of different genres of music
- The role of music in promoting social bonding and cohesion
- The effects of music on creativity and problem-solving abilities
- The psychological benefits of playing a musical instrument
- The impact of music on motivation and productivity
- The psychological effects of music on physical exercise performance
- The role of music in enhancing learning and academic performance
- The influence of music on sleep quality and patterns
- The psychological effects of music on individuals with autism spectrum disorder
- The relationship between music and personality traits
Music Education Research Paper Topics:
- The impact of music education on cognitive development in children
- The effectiveness of incorporating technology in music education
- The role of music education in promoting social and emotional development
- The benefits of music education for students with special needs
- The influence of music education on academic achievement
- The importance of music education in fostering creativity and innovation
- The relationship between music education and language development
- The impact of music education on self-esteem and self-confidence
- The role of music education in promoting cultural diversity and inclusivity
- The effects of music education on students’ overall well-being and mental health
- The significance of music education in developing critical thinking skills
- The role of music education in enhancing students’ teamwork and collaboration abilities
- The impact of music education on students’ motivation and engagement in school
- The effectiveness of different teaching methods in music education
- The relationship between music education and career opportunities in the music industry
Music History Research Paper Topics:
- The influence of African music on the development of jazz in the United States
- The role of women composers in classical music during the 18th century
- The impact of the Beatles on the evolution of popular music in the 1960s
- The cultural significance of hip-hop music in urban communities
- The development of opera in Italy during the Renaissance
- The influence of folk music on the protest movements of the 1960s
- The role of music in religious rituals and ceremonies throughout history
- The evolution of electronic music and its impact on contemporary music production
- The contribution of Latin American musicians to the development of salsa music
- The influence of classical music on film scores in the 20th century
- The role of music in the Civil Rights Movement in the United States
- The development of reggae music in Jamaica and its global impact
- The influence of Mozart’s compositions on the classical music era
- The role of music in the French Revolution and its impact on society
- The evolution of punk rock music and its influence on alternative music genres
Music Sociology Research Paper Topics:
- The impact of music streaming platforms on the music industry
- The role of music in shaping cultural identity
- Gender representation in popular music: A sociological analysis
- The influence of social media on music consumption patterns
- Music festivals as spaces for social interaction and community building
- The relationship between music and political activism
- The effects of globalization on local music scenes
- The role of music in constructing and challenging social norms
- The impact of technology on music production and distribution
- Music and social movements: A comparative study
- The role of music in promoting social change and social justice
- The influence of socioeconomic factors on music taste and preferences
- The role of music in constructing and reinforcing gender stereotypes
- The impact of music education on social and cognitive development
- The relationship between music and mental health: A sociological perspective
Classical Music Research Paper Topics:
- The influence of Ludwig van Beethoven on the development of classical music
- The role of women composers in classical music history
- The impact of Johann Sebastian Bach’s compositions on future generations
- The evolution of opera in the classical period
- The significance of Mozart’s symphonies in the classical era
- The influence of nationalism on classical music during the Romantic period
- The portrayal of emotions in classical music compositions
- The use of musical forms and structures in the works of Franz Joseph Haydn
- The impact of the Industrial Revolution on the production and dissemination of classical music
- The relationship between classical music and dance in the Baroque era
- The role of patronage in the development of classical music
- The influence of folk music on classical composers
- The representation of nature in classical music compositions
- The impact of technological advancements on classical music performance and recording
- The exploration of polyphony in the works of Johann Sebastian Bach
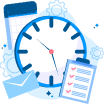
- Writing a Research Paper
- Research Paper Title
- Research Paper Sources
- Research Paper Problem Statement
- Research Paper Thesis Statement
- Hypothesis for a Research Paper
- Research Question
- Research Paper Outline
- Research Paper Summary
- Research Paper Prospectus
- Research Paper Proposal
- Research Paper Format
- Research Paper Styles
- AMA Style Research Paper
- MLA Style Research Paper
- Chicago Style Research Paper
- APA Style Research Paper
- Research Paper Structure
- Research Paper Cover Page
- Research Paper Abstract
- Research Paper Introduction
- Research Paper Body Paragraph
- Research Paper Literature Review
- Research Paper Background
- Research Paper Methods Section
- Research Paper Results Section
- Research Paper Discussion Section
- Research Paper Conclusion
- Research Paper Appendix
- Research Paper Bibliography
- APA Reference Page
- Annotated Bibliography
- Bibliography vs Works Cited vs References Page
- Research Paper Types
- What is Qualitative Research
Receive paper in 3 Hours!
- Choose the number of pages.
- Select your deadline.
- Complete your order.
Number of Pages
550 words (double spaced)
Deadline: 10 days left
By clicking "Log In", you agree to our terms of service and privacy policy . We'll occasionally send you account related and promo emails.
Sign Up for your FREE account
IEEE Account
- Change Username/Password
- Update Address
Purchase Details
- Payment Options
- Order History
- View Purchased Documents
Profile Information
- Communications Preferences
- Profession and Education
- Technical Interests
- US & Canada: +1 800 678 4333
- Worldwide: +1 732 981 0060
- Contact & Support
- About IEEE Xplore
- Accessibility
- Terms of Use
- Nondiscrimination Policy
- Privacy & Opting Out of Cookies
A not-for-profit organization, IEEE is the world's largest technical professional organization dedicated to advancing technology for the benefit of humanity. © Copyright 2024 IEEE - All rights reserved. Use of this web site signifies your agreement to the terms and conditions.
Information
- Author Services
Initiatives
You are accessing a machine-readable page. In order to be human-readable, please install an RSS reader.
All articles published by MDPI are made immediately available worldwide under an open access license. No special permission is required to reuse all or part of the article published by MDPI, including figures and tables. For articles published under an open access Creative Common CC BY license, any part of the article may be reused without permission provided that the original article is clearly cited. For more information, please refer to https://www.mdpi.com/openaccess .
Feature papers represent the most advanced research with significant potential for high impact in the field. A Feature Paper should be a substantial original Article that involves several techniques or approaches, provides an outlook for future research directions and describes possible research applications.
Feature papers are submitted upon individual invitation or recommendation by the scientific editors and must receive positive feedback from the reviewers.
Editor’s Choice articles are based on recommendations by the scientific editors of MDPI journals from around the world. Editors select a small number of articles recently published in the journal that they believe will be particularly interesting to readers, or important in the respective research area. The aim is to provide a snapshot of some of the most exciting work published in the various research areas of the journal.
Original Submission Date Received: .
- Active Journals
- Find a Journal
- Journal Proposal
- Proceedings Series
- For Authors
- For Reviewers
- For Editors
- For Librarians
- For Publishers
- For Societies
- For Conference Organizers
- Open Access Policy
- Institutional Open Access Program
- Special Issues Guidelines
- Editorial Process
- Research and Publication Ethics
- Article Processing Charges
- Testimonials
- Preprints.org
- SciProfiles
- Encyclopedia
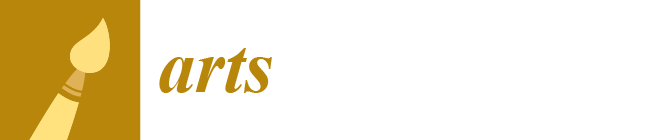
Article Menu
- Subscribe SciFeed
- Recommended Articles
- Google Scholar
- on Google Scholar
- Table of Contents
Find support for a specific problem in the support section of our website.
Please let us know what you think of our products and services.
Visit our dedicated information section to learn more about MDPI.
JSmol Viewer
Approaches in intelligent music production.

1. Introduction
2. levels of control, 2.1. insightive, 2.2. suggestive, 2.3. independent, 2.4. automatic, 2.5. control level summary, 3. knowledge representation, 3.1. grounded theory, 3.2. knowledge based systems, 3.3. data driven, 3.4. knowledge representation summary, 4. audio manipulation, 4.1. adaptive audio effects, 4.2. direct transformation, 4.3. audio manipulation summary, 5. discussion, 6. conclusions, author contributions, acknowledgments, conflicts of interest.
- Arel, Itamar, Derek C. Rose, and Thomas P. Karnowski. 2010. Deep machine learning—A new frontier in artificial intelligence research. IEEE Computational Intelligence Magazine 5: 13–18. [ Google Scholar ] [ CrossRef ]
- Barchiesi, Daniele, and Josh Reiss. 2009. Automatic target mixing using least-squares optimization of gains and equalization settings. Paper presented at the 12th Conference on Digital Audio Effects (DAFx-09), Como, Italy, September 1–4. [ Google Scholar ]
- Bartók, Béla, and Benjamin Suchoff. 1993. Béla Bartók Essays . Lincoln: University of Nebraska Press. [ Google Scholar ]
- Benito, Adán L., and Joshua D Reiss. 2017. Intelligent multitrack reverberation based on hinge-loss markov random fields. Paper presented at the Audio Engineering Society Conference: 2017 AES International Conference on Semantic Audio, Erlangen, Germany, June 22–24. [ Google Scholar ]
- Bittner, Rachel M., Justin Salamon, Mike Tierney, Matthias Mauch, Chris Cannam, and Juan Pablo Bello. 2014. Medleydb: A multitrack dataset for annotation-intensive MIR research. Paper presented at the International Society for Music Information Retrieval (ISMIR) 2014, Taipei, Taiwan, October 27–31; vol. 14, pp. 155–60. [ Google Scholar ]
- Bocko, Gregory, Mark F. Bocko, Dave Headlam, Justin Lundberg, and Gang Ren. 2010. Automatic music production system employing probabilistic expert systems. In Audio Engineering Society Convention 129 . New York: Audio Engineering Society. [ Google Scholar ]
- Bromham, Gary. 2016. How can academic practice inform mix-craft? In Mixing Music . Perspective on Music Production. Edited by Russ Hepworth-Sawyer and Jay Hodgson. Oxon: Taylor & Francis, chp. 16. pp. 245–56. [ Google Scholar ]
- Bromham, Gary, David Moffat, György Fazekas, Mathieu Barthet, and Mark B. Sandler. 2018. The impact of compressor ballistics on the perceived style of music. In Audio Engineering Society Convention 145 . New York: Audio Engineering Society. [ Google Scholar ]
- Bruford, Fred, Mathieu Barthet, SKoT McDonald, and Mark B. Sandler. 2019. Groove explorer: An intelligent visual interface for drum loop library navigation. Paper presented at the 24th ACM Intelligent User Interfaces Conference (IUI), Marina del Ray, CA, USA, March 17–20. [ Google Scholar ]
- Cascone, Kim. 2000. The aesthetics of failure: “Post-digital” tendencies in contemporary computer music. Computer Music Journal 24: 12–18. [ Google Scholar ] [ CrossRef ]
- Chourdakis, Emmanouil Theofanis, and Joshua D. Reiss. 2016. Automatic control of a digital reverberation effect using hybrid models. In Audio Engineering Society Conference: 60th International Conference: DREAMS (Dereverberation and Reverberation of Audio, Music, and Speech) . Leuven: Audio Engineering Society. [ Google Scholar ]
- Chourdakis, Emmanouil T., and Joshua D. Reiss. 2017. A machine-learning approach to application of intelligent artificial reverberation. Journal of the Audio Engineering Society 65: 56–65. [ Google Scholar ] [ CrossRef ]
- Cohen, Sara. 1993. Ethnography and popular music studies. Popular Music 12: 123–38. [ Google Scholar ] [ CrossRef ]
- De Man, Brecht, Nicholas Jillings, David Moffat, Joshua D. Reiss, and Ryan Stables. 2016. Subjective comparison of music production practices using the Web Audio Evaluation Tool. Paper presented at the 2nd AES Workshop on Intelligent Music Production, London, UK, September 13. [ Google Scholar ]
- De Man, Brecht, Kirk McNally, and Joshua D. Reiss. 2017. Perceptual evaluation and analysis of reverberation in multitrack music production. Journal of the Audio Engineering Society 65: 108–16. [ Google Scholar ] [ CrossRef ]
- De Man, Brecht, Mariano Mora-Mcginity, György Fazekas, and Joshua D. Reiss. 2014. The open multitrack testbed. Paper presented at the 137th Convention of the Audio Engineering Society, Los Angeles, CA, USA, October 9–12. [ Google Scholar ]
- De Man, Brecht, and Joshua D. Reiss. 2013a. A knowledge-engineered autonomous mixing system. In Audio Engineering Society Convention 135 . New York: Audio Engineering Society. [ Google Scholar ]
- De Man, Brecht, and Joshua D. Reiss. 2013b. A semantic approach to autonomous mixing. Paper presented at the 2013 Art of Record Production Conference (JARP) 8, Université Laval, Quebec, QC, Canada, December 1. [ Google Scholar ]
- De Man, Brecht, Joshua D. Reiss, and Ryan Stables. 2017. Ten years of automatic mixing. Paper presented at the 3rd AES Workshop on Intelligent Music Production, Salford, UK, September 15. [ Google Scholar ]
- Deruty, Emmanuel. 2016. Goal-oriented mixing. Paper presented at the 2nd AES Workshop on Intelligent Music Production, London, UK, September 13. [ Google Scholar ]
- Dugan, Dan. 1975. Automatic microphone mixing. Journal of the Audio Engineering Society 23: 442–49. [ Google Scholar ]
- Eno, Brian. 2004. The studio as compositional tool. In Audio Culture: Readings in Modern Music . Edited by Christoph Cox and Daniel Warner. Londres: Continum, chp. 22. pp. 127–30. [ Google Scholar ]
- Fenton, Steven. 2018. Automatic mixing of multitrack material using modified loudness models. In Audio Engineering Society Convention 145 . New York: Audio Engineering Society. [ Google Scholar ]
- Ford, Jon, Mark Cartwright, and Bryan Pardo. 2015. Mixviz: A tool to visualize masking in audio mixes. In Audio Engineering Society Convention 139 . New York: Audio Engineering Society. [ Google Scholar ]
- Glaser, Barney, and Anselm Strauss. 1967. Grounded theory: The discovery of grounded theory. Sociology the Journal of the British Sociological Association 12: 27–49. [ Google Scholar ]
- Gonzalez, Enrique Perez, and Joshua D. Reiss. 2007. Automatic mixing: Live downmixing stereo panner. Paper presented at the 10th International Conference on Digital Audio Effects (DAFx’07), Bordeaux, France, September 10–15; pp. 63–68. [ Google Scholar ]
- Gonzalez, Enrique Perez, and Joshua D. Reiss. 2008. Improved control for selective minimization of masking using interchannel dependancy effects. Paper presented at the 11th International Conference on Digital Audio Effects (DAFx’08), Espoo, Finland, September 1–4; p. 12. [ Google Scholar ]
- Hargreaves, Steven. 2014. Music Metadata Capture in the Studio from Audio and Symbolic Data. Ph.D. thesis, Queen Mary University of London, London, UK. [ Google Scholar ]
- Jillings, Nicholas, and Ryan Stables. 2017. Automatic masking reduction in balance mixes using evolutionary computing. In Audio Engineering Society Convention 143 . New York: Audio Engineering Society. [ Google Scholar ]
- Jun, Sanghoon, Daehoon Kim, Mina Jeon, Seungmin Rho, and Eenjun Hwang. 2015. Social mix: Automatic music recommendation and mixing scheme based on social network analysis. The Journal of Supercomputing 71: 1933–54. [ Google Scholar ] [ CrossRef ]
- King, Andrew. 2015. Technology as a vehicle (tool and practice) for developing diverse creativities. In Activating Diverse Musical Creativities: Teaching and Learning in Higher Music Education . Edited by Pamela Burnard and Elizabeth Haddon. London: Bloomsbury Publishing, chp. 11. pp. 203–22. [ Google Scholar ]
- Kolasinski, Bennett. 2008. A framework for automatic mixing using timbral similarity measures and genetic optimization. In Audio Engineering Society Convention 124 . Amsterdam: Audio Engineering Society. [ Google Scholar ]
- Martínez, Ramírez Marco A., Emmanouil Benetos, and Joshua D. Reiss. 2019. A general-purpose deep learning approach to model time-varying audio effects. Paper presented at the International Conference on Digital Audio Effects (DAFx19), Birmingham, UK, September 2–6. [ Google Scholar ]
- Martínez Ramírez, Marco A., and Joshua D. Reiss. 2017a. Deep learning and intelligent audio mixing. Paper presented at the 3rd Workshop on Intelligent Music Production, Salford, UK, September 15. [ Google Scholar ]
- Martínez Ramírez, Marco A., and Joshua D. Reiss. 2017b. Stem audio mixing as a content-based transformation of audio features. Paper presented at the 19th IEEE Workshop on Multimedia Signal Processing (MMSP), Luton, UK, October 16–18; pp. 1–6. [ Google Scholar ]
- Martínez Ramírez, Marco A., and Joshua D. Reiss. 2019. Modeling nonlinear audio effects with end-to-end deep neural networks. Paper presented at the ICASSP 2019—2019 IEEE International Conference on Acoustics, Speech and Signal Processing (ICASSP), Brighton, UK, May 12–17; pp. 171–75. [ Google Scholar ]
- Merchel, Sebastian, M. Ercan Altinsoy, and Maik Stamm. 2012. Touch the sound: Audio-driven tactile feedback for audio mixing applications. Journal of the Audio Engineering Society 60: 47–53. [ Google Scholar ]
- Mimilakis, Stylianos Ioannis, Estefanıa Cano, Jakob Abeßer, and Gerald Schuller. 2016b. New sonorities for jazz recordings: Separation and mixing using deep neural networks. Paper presented at the 2nd AES Workshop on Intelligent Music Production (WIMP), London, UK, September 13; Volume 2. [ Google Scholar ]
- Mimilakis, Stylianos Ioannis, Konstantinos Drossos, Andreas Floros, and Dionysios Katerelos. 2013. Automated tonal balance enhancement for audio mastering applications. In Audio Engineering Society Convention 134 . Rome: Audio Engineering Society. [ Google Scholar ]
- Moffat, David, David Ronan, and Joshusa D. Reiss. 2015. An evaluation of audio feature extraction toolboxes. Paper presented at the 18th International Conference on Digital Audio Effects (DAFx-15), Trondheim, Norway, November 30–December 3. [ Google Scholar ]
- Moffat, David, and Mark B. Sandler. 2018. Adaptive ballistics control of dynamic range compression for percussive tracks. In Audio Engineering Society Convention 145 . New York: Audio Engineering Society. [ Google Scholar ]
- Moffat, David, and Mark B. Sandler. 2019. Automatic mixing level balancing enhanced through source interference identification. In Audio Engineering Society Convention 146 . Dublin: Audio Engineering Society. [ Google Scholar ]
- Moffat, David, Florian Thalmann, and Mark B. Sandler. 2018. Towards a semantic web representation and application of audio mixing rules. Paper presented at the 4th Workshop on Intelligent Music Production (WIMP), Huddersfield, UK, September 14. [ Google Scholar ]
- Muir, Bonnie M. 1994. Trust in automation: Part i. theoretical issues in the study of trust and human intervention in automated systems. Ergonomics 37: 1905–22. [ Google Scholar ] [ CrossRef ]
- Ooi, Kazushige, Keiji Izumi, Mitsuyuki Nozaki, and Ikuya Takeda. 1990. An advanced autofocus system for video camera using quasi condition reasoning. IEEE Transactions on Consumer Electronics 36: 526–30. [ Google Scholar ] [ CrossRef ]
- Pachet, François, and Olivier Delerue. 2000. On-the-fly multi-track mixing. In Audio Engineering Society Convention 109 . Los Angeles: Audio Engineering Society. [ Google Scholar ]
- Palladini, Alessandro. 2018. Intelligent audio machines. Paper presented at the Keynote Talk at 4th Workshop on Intelligent Music Production (WIMP-18), Huddersfield, UK, September 14. [ Google Scholar ]
- Pardo, Bryan, Zafar Rafii, and Zhiyao Duan. 2018. Audio source separation in a musical context. In Springer Handbook of Systematic Musicology . Berlin: Springer, pp. 285–98. [ Google Scholar ]
- Paterson, Justin, Rob Toulson, Sebastian Lexer, Tim Webster, Steve Massey, and Jonas Ritter. 2016. Interactive digital music: Enhancing listener engagement with commercial music. KES Transaction on Innovation In Music 2: 193–209. [ Google Scholar ]
- Pestana, Pedro Duarte, Zheng Ma, Joshua D. Reiss, Alvaro Barbosa, and Dawn A.A. Black. 2013. Spectral characteristics of popular commercial recordings 1950–2010. In Audio Engineering Society Convention 135 . New York: Audio Engineering Society. [ Google Scholar ]
- Pestana, Pedro Duarte Leal Gomes. 2013. Automatic Mixing Systems Using Adaptive Digital Audio Effects. Ph.D. Thesis, Universidade Católica Portuguesa, Porto, Portugal. [ Google Scholar ]
- Prior, Nick. 2012. Digital formations of popular music. Réseaux 2: 66–90. [ Google Scholar ] [ CrossRef ]
- Reiss, Joshua, and yvind Brandtsegg. 2018. Applications of cross-adaptive audio effects: Automatic mixing, live performance and everything in between. Frontiers in Digital Humanities 5: 17. [ Google Scholar ] [ CrossRef ]
- Reiss, Joshua D. 2011. Intelligent systems for mixing multichannel audio. Paper presented at the17th International Conference on Digital Signal Processing (DSP), Corfu, Greece, July 6–8; pp. 1–6. [ Google Scholar ]
- Reiss, Joshua D. 2018. Do You Hear What I Hear? The Science of Everyday Sounds . Inaugural Lecture. London: Queen Mary University. [ Google Scholar ]
- Rödel, Christina, Susanne Stadler, Alexander Meschtscherjakov, and Manfred Tscheligi. 2014. Towards autonomous cars: The effect of autonomy levels on acceptance and user experience. Paper presented at the 6th International Conference on Automotive User Interfaces and Interactive Vehicular Applications, Seattle, WA, USA, September 17–19; New York: ACM, pp. 1–8. [ Google Scholar ]
- Ronan, David, Brecht De Man, Hatice Gunes, and Joshua D. Reiss. 2015. The impact of subgrouping practices on the perception of multitrack music mixes. In Audio Engineering Society Convention 139 . New York: Audio Engineering Society. [ Google Scholar ]
- Ronan, David, Hatice Gunes, and Joshua D. Reiss. 2017. Analysis of the subgrouping practices of professional mix engineers. In Audio Engineering Society Convention 142 . New York: Audio Engineering Society. [ Google Scholar ]
- Ronan, David, Zheng Ma, Paul Mc Namara, Hatice Gunes, and Joshua D. Reiss. 2018. Automatic minimisation of masking in multitrack audio using subgroups. arXiv . [ Google Scholar ]
- Ronan, David, David Moffat, Hatice Gunes, and Joshua D. Reiss. 2015. Automatic subgrouping of multitrack audio. Paper presented at the 18th International Conference on Digital Audio Effects (DAFx-15), Trondheim, Norway, November 30–December 3. [ Google Scholar ]
- Russell, Stuart J., and Peter Norvig. 2016. Artificial Intelligence: A Modern Approach , 3rd ed. Malaysia: Pearson Education Limited. [ Google Scholar ]
- Schmidt, Brian. 2003. Interactive mixing of game audio. In Audio Engineering Society Convention 115 . New York: Audio Engineering Society. [ Google Scholar ]
- Scott, Jeffrey J., and Youngmoo E. Kim. 2011. Analysis of acoustic features for automated multi-track mixing. Paper presented at the 12th International Society for Music Information Retrieval Conference (ISMIR), Miami, FL, USA, October 24–28; pp. 621–26. [ Google Scholar ]
- Selfridge, Rod, David Moffat, Eldad J. Avital, and Joshua D. Reiss. 2018. Creating real-time aeroacoustic sound effects using physically informed models. Journal of the Audio Engineering Society 66: 594–607. [ Google Scholar ] [ CrossRef ]
- Sheridan, Thomas B., and William L. Verplank. 1978. Human and Computer Control of Undersea Teleoperators . Technical Report. Cambridge: Massachusetts Inst of Tech Cambridge Man-Machine Systems Lab. [ Google Scholar ]
- Smolka, Bogdan, K. Czubin, Jon Yngve Hardeberg, Kostas N. Plataniotis, Marek Szczepanski, and Konrad Wojciechowski. 2003. Towards automatic redeye effect removal. Pattern Recognition Letters 24: 1767–85. [ Google Scholar ] [ CrossRef ]
- Stasis, Spyridon, Nicholas Jillings, Sean Enderby, and Ryan Stables. 2017. Audio processing chain recommendation. Paper presented at the 20th International Conference on Digital Audio Effects, Edinburgh, UK, September 5–9. [ Google Scholar ]
- Stevens, Richard, and Dave Raybould. 2013. The Game Audio Tutorial: A Practical Guide to Creating and Implementing Sound and Music for Interactive Games . London: Routledge. [ Google Scholar ]
- Terrell, Michael, and Mark Sandler. 2012. An offline, automatic mixing method for live music, incorporating multiple sources, loudspeakers, and room effects. Computer Music Journal 36: 37–54. [ Google Scholar ] [ CrossRef ]
- Terrell, Michael, Andrew Simpson, and Mark Sandler. 2014. The mathematics of mixing. Journal of the Audio Engineering Society 62: 4–13. [ Google Scholar ] [ CrossRef ]
- Van Waterschoot, Toon, and Marc Moonen. 2011. Fifty years of acoustic feedback control: State of the art and future challenges. Proceedings of the IEEE 99: 288–327. [ Google Scholar ] [ CrossRef ]
- Verfaille, Vincent, Daniel Arfib, Florian Keiler, Adrian von dem Knesebeck, and Udo Zölter. 2011. Adaptive digital audio effects. In DAFX—Digital Audio Effects . Edited by Udo Zölter. Hoboken: John Wiley & Sons, Inc., chp. 9. pp. 321–91. [ Google Scholar ]
- Verfaille, Vincent, Udo Zolzer, and Daniel Arfib. 2006. Adaptive digital audio effects (A-DAFx): A new class of sound transformations. IEEE Transactions on Audio, Speech, and Language Processing 14: 1817–31. [ Google Scholar ] [ CrossRef ]
- Verma, Prateek, and Julius O. Smith. 2018. Neural style transfer for audio spectograms. Paper presented at the 31st Conference on Neural Information Processing Systems (NIPS 2017), Workshop for Machine Learning for Creativity and Design, Long Beach, CA, USA, December 4–9. [ Google Scholar ]
- Vickers, Earl. 2010. The loudness war: Background, speculation, and recommendations. In Audio Engineering Society Convention 129 . San Francisco: Audio Engineering Society. [ Google Scholar ]
- Wichern, Gordon, Aaron Wishnick, Alexey Lukin, and Hannah Robertson. 2015. Comparison of loudness features for automatic level adjustment in mixing. In Audio Engineering Society Convention 139 . New York: Audio Engineering Society. [ Google Scholar ]
- Wilson, Alex, and Bruno Fazenda. 2015. 101 mixes: A statistical analysis of mix-variation in a dataset of multi-track music mixes. In Audio Engineering Society Convention 139 . New York: Audio Engineering Society. [ Google Scholar ]
- Witten, Ian H., Eibe Frank, Mark A. Hall, and Christopher J. Pal. 2016. Data Mining: Practical Machine Learning Tools and Techniques . Burlington: Morgan Kaufmann. [ Google Scholar ]
- Xambó, Anna, Frederic Font, György Fazekas, and Mathieu Barthet. 2019. Leveraging online audio commons content for media production. In Foundations in Sound Design for Linear Media . London: Routledge, pp. 248–82. [ Google Scholar ]
1 | . |
2 | . |
Click here to enlarge figure
Share and Cite
Moffat, D.; Sandler, M.B. Approaches in Intelligent Music Production. Arts 2019 , 8 , 125. https://doi.org/10.3390/arts8040125
Moffat D, Sandler MB. Approaches in Intelligent Music Production. Arts . 2019; 8(4):125. https://doi.org/10.3390/arts8040125
Moffat, David, and Mark B. Sandler. 2019. "Approaches in Intelligent Music Production" Arts 8, no. 4: 125. https://doi.org/10.3390/arts8040125
Article Metrics
Article access statistics, further information, mdpi initiatives, follow mdpi.
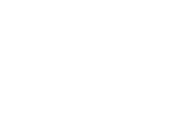
Subscribe to receive issue release notifications and newsletters from MDPI journals
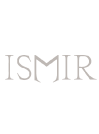
Transactions of the International Society for Music Information Retrieval
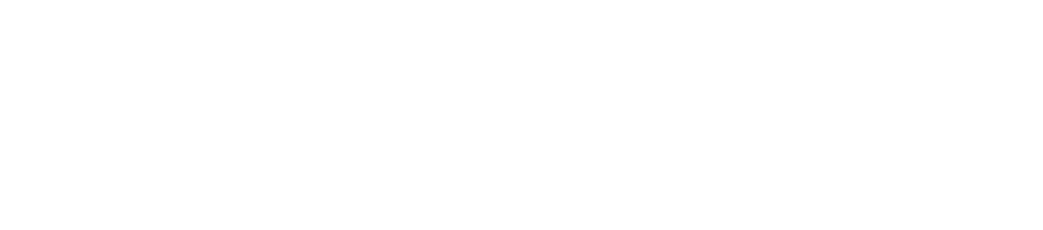
- Download PDF (English) XML (English)
- Alt. Display
- Collection: AI and Musical Creativity
Research articles
On the development and practice of ai technology for contemporary popular music production.
- Emmanuel Deruty
- Maarten Grachten
- Stefan Lattner
- Javier Nistal
- Cyran Aouameur
Although the use of AI technology for music production is still in its infancy, it has the potential to make a lasting impact on the way we produce music. In this paper we focus on the design and use of AI music tools for the production of contemporary Popular Music, in particular genres involving studio technology as part of the creative process. First we discuss how music production practices associated with those genres can differ significantly from traditional views of how a musical work is created, and how this affects AI music technology. We argue that—given the role of symbolic representations in this context, as well as the integration of composition activities with editing and mixing—audio-based AI tools are better suited to support the artist’s creative workflow than purely piano-roll/MIDI-based tools. Then we give a report of collaborations with professional artists, in which we look at how various AI tools are used in practice to produce music. We identify usage patterns as well as issues and challenges that arise in practical use of the tools. Based on this we formulate some recommendations and validation criteria for the development of AI technology for contemporary Popular Music.
- Artificial Intelligence
- Music Production
- Popular Music
1 Introduction
The practice of making music has since long involved instruments and—more generally—technology. As such, advances in technology can have a profoundly transformative effect on music making practice (which we will refer to as music production in this paper). This effect is witnessed for by the roles analog and later digital electronics have played in music, in the form of synthesizers, samplers, sound effect gear, and digital audio workstations.
More recently artificial intelligence (AI) has increasingly found application in music production. Although arguably the use of AI technology for music production is still largely experimental, it does not seem far-fetched that this technology will eventually have a lasting effect on music production.
The versatility of AI technology allows for a wide variety of applications in music, which has only just begun to be explored. Current applications include synthesis of individual musical sounds ( Engel et al., 2017 ; Aouameur et al., 2019 ), “musical inpainting” ( Hadjeres et al., 2017 ; Bazin et al., 2020 ), interpolation between musical material ( Roberts et al., 2018 ), or synthesis of complete multi-instrumental audio tracks ( Dhariwal et al., 2020 ).
A variety of AI music production services has emerged in recent years, with different focus areas. 1 These services are often offered on dedicated platforms rather than providing integration with an artist’s existing working environment, and as such are not yet a widely used commodity for artists. Understanding, installing, running, and using AI music tools independently is not trivial, as it tends to require technical expertise and familiarity with the basic notions of machine learning. This is also witnessed in the AI Song Contest ( Huang et al., 2020 ), a contest in which musicians and AI engineers often team-up in order to jointly produce music with AI tools.
As AI technology is starting to find its way into music production practice, there are several underlying questions that deserve attention. For example, which (sub)tasks of music production should AI tools address to be most useful and relevant for musicians? A further question concerns the possibilities to exert control over the output by the musician, and how the user interface and more generally the affordances of the model should be shaped ( Gibson, 1977 ). Lastly, an important consideration is how AI tools can become part of the music production process, in particular the musician’s creative workflow. Answers to these broad questions will necessarily be subjective, and vary depending on individual and style-specific musical practices.
As a music AI research lab specializing in music AI tools for commercial artists, 2 we aim to address the above questions in the context of music production practices of contemporary Popular Music (CPM). Popular Music is a very broad category typically used in contrast to Folk Music and Art Music ( Tagg, 1982 ; Middleton, 1990 ; Mazzanti, 2019 ). An important distinguishing feature (among others) of Popular Music in this characterization is the use of recorded sound as the main mode of transmission (storage/distribution) ( Tagg, 1982, page 42 ). This sets it apart from Folk Music (where oral transmission is predominant) and Art Music (where music notation is the main mode of transmission). Frith ( 2004 ) lists further features of Popular Music, like the prevalence of commercial/industrial interests, enjoyment/entertainment as the main purpose of the music, and strong ties with mass media like cinema, radio, and television.
The adjective “contemporary” does not seem to have a universal denotation in conjunction with Popular Music beyond “current”, or “present-time”. The Berklee College of Music 3 mentions a focus on technological innovation and cross-pollination across cultures and genres as characteristics of contemporary music—characteristics that have also been attributed to Popular Music in general ( Frith, 2004 ; Mazzanti, 2019 ). As such, CPM genres include post-rock, rap/hip-hop, electronica, as well as non-Western contemporary genres such as K-pop, J-pop, Bollywood, and reggaeton.
With this paper we intend to foster the development of AI technology geared towards music production in these genres. To this end we make two main contributions. Firstly, we present a discussion of how music production practices in these genres have diverged in some ways from a more established view of how music is produced (Section 3). We emphasize these differences because, as we will argue, they have implications for the design of AI music tools, but do not seem to be widely acknowledged in literature on AI music generation research.
Secondly, we give a qualitative report of collaborations of our music AI research lab with professional artists/acts producing music in several CPM genres such as urban, ambient, experimental, neo-classical, trance, and mainstream for commercials. In these collaborations the artists experiment with music AI tools developed at our lab in their creative process. We provide a detailed account of the creative process by one of the artists to illustrate the different roles the tools may play in the process, and we present and discuss feedback provided by the artists, linking aspects of their work to issues discussed in Section 3.
In Section 5 we summarize our findings in the form of recommendations for developing music AI tools in the context of contemporary music genres, as well as a list of validation criteria. Conclusions and future work are presented in Section 6. We start with a brief discussion of the possible music production use cases in which AI technology may play a role, and delimit the class of use cases on which we focus our attention (Section 2).
2 Music Production Use Cases
Music production in the general sense as the practice of creating musical works is a very diverse set of activities, and the ways in which AI tools can be used in music production are equally diverse. Without aiming to be exhaustive, we distinguish three broad types of music production contexts for AI tools. Firstly there is music composition in its traditional form, as the creation of a symbolic description of a musical work, be it as MIDI, Western staff notation, or some other symbolic format. Although any type of music may be created through this form of music production, the use of AI tools is somewhat limited to the genres for which substantial data is available in symbolic formats. Examples of AI tools designed for music composition are Magenta Studio ( Roberts et al., 2019 ), DeepBach ( Hadjeres et al., 2017 ), and the Piano Inpainting Application ( Hadjeres and Crestel, 2021 ).
Another type of music production is live performance and improvisation. This includes instrumental performances with or without a pre-determined score or schema to structure the performance. AI tools in this context tend to be focused on responsiveness and real-time interaction. A different type of live music production takes place in clubs and dance venues, where DJs mix electronic dance music, often mixing existing works with musical elements that are added live, and adapting playlists in response to the mood of the audience. See Knotts and Collins ( 2021 ) for an overview.
A third type of context is what can be broadly called in-studio composition. Activity in this context centers around the digital audio workstation (DAW), a piece of software in which the recording, editing, placement, and processing of recorded material, as well as the creation and synthesis of any symbolic representations is coordinated. AI tools for the first context can be useful in this context too, but a crucial difference from the first context is that the activity of writing music notation is combined with sound recording, editing, and mixing activities, and these activities do not necessarily take place in a fixed order. Knotts and Collins ( 2021 ) give a historical account of AI tools in this context.
It is this last context that we focus on when discussing AI for music production in this paper. In the next section we give a brief overview of the development of the in-studio composition practice, which has become a standard form of CPM music production in the past decades ( Bell, 2018 ). We focus on the role of symbolic representations and the different stages of music production. We then discuss some implications for AI technology to support this practice.
3 AI Technology for in-Studio Composition
3.1 music production as in-studio composition.
Western music production across the 18th and 19th centuries can be roughly schematized as follows: a composer produces a musical work in the form of music notation, after which one or more performers create the sounds the composer has notated in front of an audience. This music production practice was thus a linear process: composition → performance → consumption , where the roles of composer and performer/musician were typically separated. The modern sound recording studio elongated this linear chain with a number of steps: composition → performance → recording → editing → mixing → mastering → consumption . The recording, mixing, and mastering tasks were carried out by sound-engineers and overseen by record-producers.
The commodification of sound recording and processing technology since the 1980s has given amateur as well as professional artists permanent and low-barrier access to studio technology. Moreover, synthesizers, drum computers, sample libraries, and virtual instruments are nowadays widely used to produce sound, either in addition to or instead of recorded performances. These developments have blurred the distinction between the roles of composer, musician, sound engineer, and record producer ( Burgess, 2013 ), as well as the classification of these roles as either art-oriented or craft-oriented ( Lashua and Thompson, 2016 ). In many contemporary Popular Music genres a song or album is typically the work of a creative collective ( Hennion, 1983 )—or even a single individual—taking charge of all of these aspects of the music production process.
The notion of in-studio composition has obsoleted the strict linearity of the music production process: a musical work is no longer composed in a symbolic form as a whole before creating a sonic realization in the studio. Instead, compositional activities are often intertwined with sound editing and sound design activities.
Another consequence is that—compared to the aforementioned music practices where the roles of composer and performer are more strictly separated—the need for music notation as a means of transmitting information from composer to performer has diminished. Music notation as “the organization of instructions for the creation of sound” ( Jones, 1992 ) is dispensable when the sound is readily available in the form of recordings to be listened to, distributed, and re-used. As a result, many CPM artists—and in fact some of the professional artists we collaborate with—do not read music notation. They have little use for it in their music production workflow.
This is not to say that symbolic representation formats do not play a vital role in CPM music production—to the contrary, MIDI is widely used in CPM music production. Rather, we wish to highlight the distinction between the use of symbolic representations such as MIDI to convey the “essence” of the music, and the use of MIDI as a convenient means to organize and trigger sonic events.
In many musical genres (including perhaps some that can be regarded as CPM) the conventions of the genre warrant strong assumptions about the mapping from symbolic representations to sonic events and shapes (in other words, what the music sounds like). For example, the sonic realization of a MIDI file that represents a classical piano performance is relatively predictable, and as such justifies the use of MIDI data as conveying the essence of the music. Moreover, MIDI information is much sparser than audio, and explicitly represents the music in terms of meaningful terms like notes, beats, meter, tempo, and key. This provides a convenient and often very effective starting point for analysis and modelling of musical information.
In other genres however, notably those that substantially involve electronically designed sounds and samples, the mapping between MIDI data and its sonic realization is much looser. Synth pads for example are deliberately designed sounds to provide an ambient sonic background, and are ubiquitous in a wide range of music nowadays. Although these sounds are usually triggered in the form of MIDI notes, individual synth pad sounds may be sustained for tens of seconds, and typically have a salient temporal development in terms of timbre, dynamics, tuning, and/or localization that is not conveyed in a piano-roll representation. 4
It can of course be argued that such genres have their own sonic aesthetics and conventions that constrain the sonic manifestations of the music, and are thus amenable in principle to symbolic representation and analysis. While this is true, the vocabulary needed for this type of analysis is still largely undeveloped, and is the subject of works like those of Moore and Martin ( 2018 ) and Moylan ( 2020 ).
Although the traditional categories of music analysis are very effective means of understanding music in some musical styles, it is important to realize that they are not always pertinent, or they may not apply cleanly. For example, while melody and harmony are essential notions in Western classical music, jazz, and more traditional forms of rock and pop, they play a much less prominent role in rap and many electronic genres.
Furthermore the pitched quality of the 808 kick drum 5 (named after Roland’s early and emblematic TR-808 drum machine)—a widely used percussive element in many CPM genres—requires careful coordination with other pitched elements in the song, just like pitched percussion (e.g. timpani) in orchestral music. In contrast to performed music, where the tuning is done before the performance, pitched percussion in CPM is commonly tuned by way of post-production. Pitched kick drums, especially given their prominent use in some CPM genres, call into question the common view of rhythm and harmony as two largely orthogonal aspects of the music.
3.2 Implications for AI technology
The above demonstrates how traditional views of music production, specifically the notion of MIDI as conveying the essence of the music, and the separation of composition from other music production activities (recording, editing, and mixing), become problematic in the context of in-studio composition. In research concerning deep learning for music generation such views still seem predominant however. For example, Briot et al. ( 2020 ) justify the focus on symbolic representations in their review of deep learning techniques for music generation as follows:
[W]e believe that the essence of music (as opposed to sound) is in the compositional process, which is exposed via symbolic representations (like musical scores or lead sheets) and is subject to analysis (e.g., harmonic analysis) ( Briot et al., 2020, Sec. 4.2 )
Another recent survey by Ji et al. ( 2020 ) classifies deep learning music generation approaches along the linear view of music production, where information is transmitted from the composer via performer and instrument to the listener. In this perspective composition takes place at the start of the chain on a purely symbolic level, whereas the sonic manifestation of the symbolic score takes place at the end, and is regarded as a task that is largely separate from the composition. Moreover, sound editing, mixing and mastering—crucial constituents of in-studio composition—are not considered at all in the survey.
Nevertheless, awareness of the limitations of such implicit assumptions about the nature of music production has been raised in recent years, and explicit efforts have been made to overcome it, such as the CompMusic research project ( Serra, 2012 ), aimed at developing MIR methods for non-Western music traditions such as Hindustani, Carnatic, and Beijing Opera. Gioti ( 2021 ) also observes a discrepancy between the note/pitch-centric design of tools such as MusicVAE ( Roberts et al., 2018 ) and Nsynth ( Engel et al., 2017 ) and contemporary art aesthetics. This diminishes the relevance of the tools for contemporary art music genres like electro-acoustic music, she argues, and calls for increased collaboration between developers and contemporary art music composers to overcome this discrepancy.
It should be emphasized that none of the arguments raised above question the validity and utility of existing AI music tools in themselves. Indeed piano-roll-based modeling approaches like those of Chu et al. ( 2016 ); Yang et al. ( 2017 ); Payne ( 2019 ); Hadjeres et al. ( 2017 ); Hadjeres and Crestel ( 2021 ); Roberts et al. ( 2019 ) address relevant problems, such as generating music with coherent long-range structure, style transfer, style-specific continuation or in-painting, and often do so with impressive results. Instead, we intend to demonstrate that in-studio composition in recent CPM genres involves a wider variety of use cases that cannot be adequately addressed with piano-roll-based approaches.
How can AI technology be designed to support a broader range of in-studio composition activities and acknowledge the interplay between these activities? Although this question is complex and entails more specific questions like those raised in the introduction, a partial but straight-forward answer may be: design AI tools that are able to deal with all the types of information that CPM artists work with, most notably audio. This applies in particular to the broad category of AI tools that produce outputs conditional on some music inputs. It allows artists to use such AI tools to condition on arbitrary material they have in their DAW project, not just the subset that is encoded as MIDI. A further benefit of audio based AI tools is that they are exposed to the sonic qualities that are not represented by MIDI representations but are musically relevant, such as the pitched quality of the kick drum, or the temporal evolution of a synth pad sound, as discussed before. Moreover, it enables the integration of composition with other stages of music production, in particular mixing. For example, a drum track created by an AI model conditioned on some harmonic tracks can potentially be modulated by changing the mix of the different tracks that go into the model, such as varying the relative gain of the input tracks, the equalization, reverb, or other effects. In a way this allows the artist to use the familiar controls of their DAW to interact with the AI tool beyond the explicit controls of the tool itself.
Of course there are valid reasons for refraining from audio representations when developing AI tools, as mentioned above. In particular it can require significantly more computational resources to store and process audio. A further challenge is capturing long-range dependencies due to the high data rate, and—in generative scenarios—to produce outputs that are coherent both at short and at long time scales. Systems such as Jukebox ( Dhariwal et al., 2020 ) show that such challenges are not insurmountable however. Furthermore, conditional generation tasks can also become easier because of the density of audio as the conditioning signal compared to symbolic data.
In conclusion, we believe it is worthwhile to pursue audio-based AI tools, because they can provide CPM artists with a richer spectrum of creative opportunities than AI tools that work exclusively with symbolic notation.
4 Using AI Tools in Practice
In line with the principles of participatory design ( Muller and Kuhn, 1993 ), the development of AI tools for CPM is arguably most effective when it is a joint effort between engineers and musicians. Participatory design may involve end-users at any stage during the development of a product or tool. In our case the collaboration with artists does not usually take place at very early stages of design. One reason for this is that our music AI research lab team includes members with expertise in music production, who give early guidance and feedback on the conceptualization of the tool. Another reason is that the development of AI tools usually takes an engineering-heavy initial phase of experimentation to explore the feasibility of ideas, making smooth interaction with artists more challenging (which is not to say that artist involvement is undesirable at this stage).
In this section we report on experiences from collaborations of our lab with six professional music artists/acts, listed in Table 1 . These collaborations both allow artists to explore novel ways of producing music, and they allow us make the AI tools more useful and interesting as part of the creative process.
Collaborating artists/acts.
Artist/Act | Genre (s) | Time-span |
---|---|---|
Rap/Urban | 6 months | |
Beats/Urban | 6 months | |
Mainstream for commercial purposes | 18 months | |
Ambient, Experimental, Neo-classical | 6 months | |
Rock/Electronic | 6 months | |
Trance | 18 months | |
It is important to note that at the time the collaborations with artists started there was no detailed plan to carry out a systematic study of the overall results beyond gathering general feedback regarding the utility and value of the AI prototypes. Although the participating artists obviously have an intrinsic interest in the collaboration (they typically are keen to experiment with new technologies), the collaboration also implies a considerable time investment with uncertain outcomes, which may or may not be acceptable to artists from a professional point of view (the difficulty of engaging creative professionals in studies has also been reported by Csikszentmihalyi ( 1997 ); Bennett ( 2012 )). Rather than volunteering as research subjects, the artists agreed to produce works with the prototypes as contractors, and provide documentation of their workflows and experiences. They consent to the use of the material they produce for research and/or promotional purposes.
The results obtained from the collaborations (interviews, songs, workflow diagrams, see Section 4.2) were subjected to a thematic analysis ( Braun and Clarke, 2006 ), similar to the approach taken by Huang et al. ( 2020 ). The overall question guiding the analysis is: how do artists use the available tools?
The thematic analysis presented in Section 4.3 is not strictly inductive. It was primed in part by the themes identified by Huang et al. ( 2020 ) and Clark et al. ( 2018 ). Other themes, notably those in Section 4.3.3, emerged from our own use of the tools prior to the collaboration, and were reinforced by the results of the collaborations.
We begin by giving a brief overview of the tools used by the artists (Section 4.1), followed by a general description of the way the collaborations take place (Section 4.2). In Section 4.3 we present and discuss some of the artists’s experiences during the collaboration.
4.1 Tools used
The AI tools provided to the artists have been recently developed at our lab, and are generally prototypes in the form of either standalone applications, VST plug-ins for digital audio workstations (DAW), or servers accessible through a web-interface. They cover different aspects of the music production process, ranging from sound design to mixing, equalization, and the generation of melodic and rhythmic material. In line with our conclusions in Section 3.2, the tools work with audio representations as input data. Depending on the tool the output may be audio, MIDI, or both. The tools have been presented in more detail in prior publications, so here we provide only a brief introduction of the tools.
Notono: An interactive tool for generating instrumental one-shots ( Bazin et al., 2020 ). It uses a variational autoencoder (VAE) architecture ( Kingma and Welling, 2014 ) that operates on spectrograms, and is conditioned on instrument labels. You can start from a sound you like and interactively modify it through inpainting of the spectrogram.
Planet Drums, DrumGAN, Impact Drums: Three drum sound synthesizers. Planet Drums is based on a VAE architecture that allows the user to explore different drum sounds by traversing a low-dimensional embedding of the latent space ( Aouameur et al., 2019 ). DrumGAN and Impact Drums are based on generative adversarial networks ( Goodfellow et al., 2014 ). DrumGan is conditioned on perceptual features that can be used as controls ( Nistal et al., 2020 ).
DrumNet: A tool for creating drum tracks conditioned on existing audio tracks like guitar, bass, or keyboard tracks ( Lattner and Grachten, 2019 ). The output adapts to the tempo and rhythm of the existing tracks, and users can explore different rhythmic variations by traversing a latent space.
BassNet, LeadNet: Tools for creating bass tracks (BassNet) or lead tracks (LeadNet), conditioned on one or more existing audio tracks ( Grachten et al., 2020 ). The output adapts to the tonality of the existing tracks (if the input is tonal), and users can explore different rhythmic and melodic variations of the output by traversing a latent space. The model outputs both MIDI and audio, and conveys articulation, dynamics, timbre, and intonation. In terms of model architecture BassNet and LeadNet are identical. They differ in that BassNet was trained on bass guitar tracks, and LeadNet was trained on vocal and lead guitar tracks.
ResonanceEQ, ProfileEQ: Adaptive equalizers for audio mixing and mastering tasks ( Grachten et al., 2019 ). They consist of hand-designed processing pipelines to adjust the spectral characteristics of the sound in an adaptive way, and additional feed-forward convolutional neural networks to estimate optimal control parameters for the equalizer process conditional on the input audio.
4.2 Procedure
At the start of the collaboration we give the artists an overview of AI and machine learning in the context of music, and explain our vision of music AI as tools to enrich the creative workflow in music production. We give them a demonstration of the available tool prototypes in the lab where they can try out the software. When the artists are familiar with the ways the tools work, they use them in their own working environment, experimenting with the tools in their music production process over the course of 6 months or longer (see Table 1 ), next to their regular professional activities. Typically there are follow-up sessions after the first session where the artists talk about their experience, what they like and dislike about the tools, and what changes they would like to see. We modify the tools accordingly, whenever the changes can be realized within a reasonable effort, while proposals that imply more fundamental changes to the tools are used to guide future development. When the artists have finalized their work they send us the outcomes, and a description of their workflow, which typically includes the AI tools, along with several other music production tools they work with. Some of the artists describe their experiences with the tools in interviews. 6
4.3 Results and discussion
In this section we present results of the collaborations in terms of the reports and feedback from the artists. We group the results into themes (such as types of interaction with the tools) that have been identified in part in prior work, such as that by Huang et al. ( 2020 ). Before that we give an example of a workflow decomposition ( Figure 1 ) in which Luc Leroy and Yann Macé of Hyper Music use several AI-driven prototypes in conjunction with mainstream music production technology. 7 Although a detailed analysis is beyond the scope of this paper, the schema exemplifies the non-linearity of the music production process (as discussed in Section 3), involving iteration between compositional activities such as creating harmonic and melodic material, and mixing activities such as equalization. Different equalizations of an audio track may emphasize different acoustical elements of the sound, and can thus lead to different rhythmic or melodic variations when used as an input to DrumNet and BassNet/LeadNet.
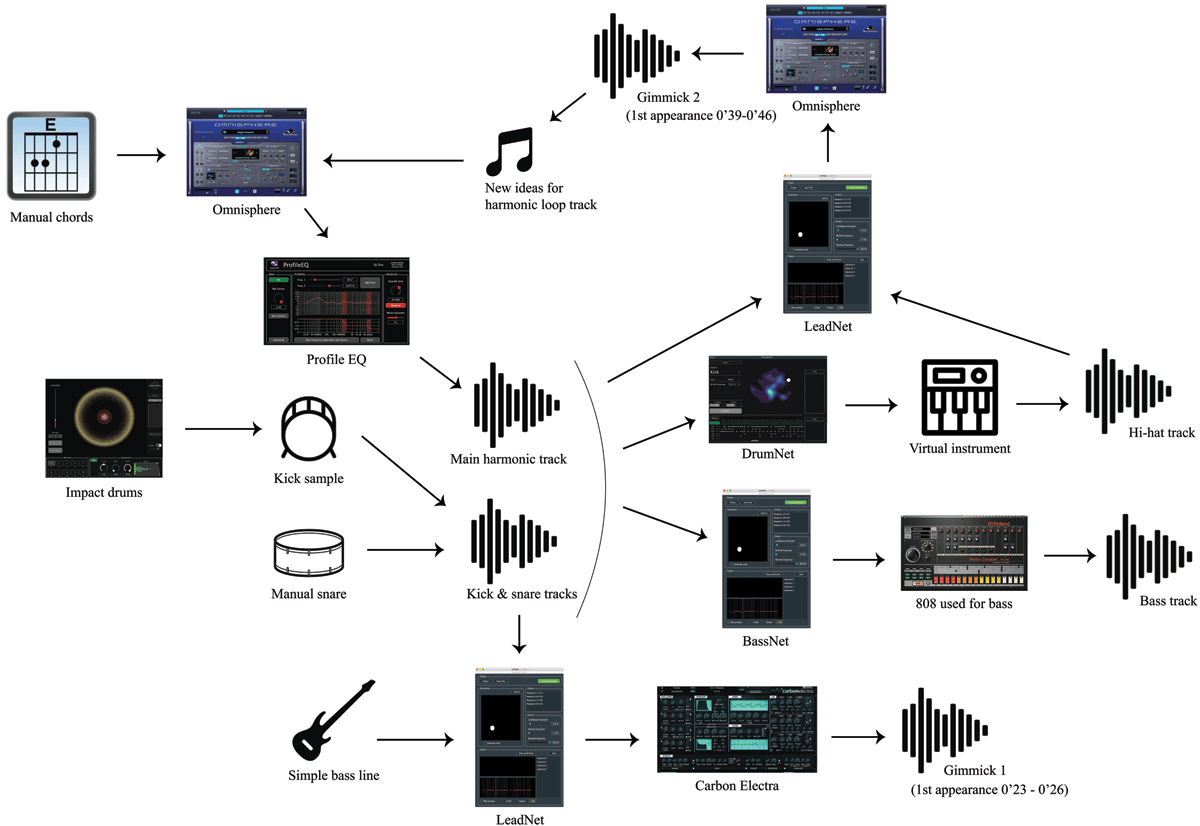
Example workflow diagram by artist duo Hyper Music . The diagram shows how various AI tool prototypes are used in the music production process.
4.3.1 Push and pull interactions
In the context of creative text writing, Clark et al. ( 2018 ) describe two approaches to start an interaction between the user and an AI tool: push interactions, where the tool makes spontaneous suggestions (e.g. in an auto-complete fashion), and pull interactions, where the user explicitly queries the tool for an output. The tools we focus on here (listed in Section 4.1) are primarily designed for pull interactions: rather than acting autonomously within a session, the tools are operated actively by the artists when they want an output.
One particular case of pull interaction is known as priming ( Huang et al., 2020 ): the artist designs an input to drive the generation process. The priming input can be used in diferent ways: it can serve as the start of a musical part to be continued by the tool, as the starting template from which variations can be explored, or as a part in a multi-part setting for which the tool generates accompanying parts. Priming amounts to what is referred to as dense conditioning ( Grachten et al., 2020 ), where the output of a model is controlled by providing a rich source of information (e.g. an audio or MIDI track) instead of sparser types of information that are provided by the typical UI elements of a control panel (sliders, buttons, presets, etc.). An example of the priming process used in production from Hyper Music :
“Made an 8-bar bounce with kick, snare plus a very simple legato bass part (not used thereafter). Fed this bounce to LeadNet. Tweaked around until I hear something inspiring: it plays a cool part with a 4-note hook that sounds good at the end of the chord cycle.”
As mentioned before, BassNet/LeadNet and DrumNet are designed to be primed on audio input. This makes it possible to react even to minor nuances in the input, like expressivity in terms of timing, dynamics, or timbre. Donn Healy’s comment underlines this:
“DrumNet handled this quirky input very well, it followed the expression to a T [very precisely]”.
4.3.2 Exploration and higher-level control
As witnessed by Huang et al. ( 2020 ) in their study, many musicians adopt the generate-then-curate strategy when working with specific AI-driven prototypes; they first generate many samples and then select those they deem valuable for further use. Artist Uèle Lamore adopted this strategy when working with the prototypes:
“The goal was to generate a selection of percussion/drum samples that I could see fit to use in any given setting. […] generating percussion sounds with DrumGAN and Planet Drums. I’m not interested in generating sounds that sound like a “real” or “classic” kit. I want sounds that are very abstract […] I now had this selection of sounds available.”
Although this strategy is not unique to AI-based approaches, the use of machine learning can potentially make it more efficient and rewarding. When creating a subset of interesting items in a large space of possibilities, there is generally a trade-off between covering a diverse range of interesting items ( recall in information retrieval terms) and avoiding uninteresting items ( precision ). As such this use case is related to recommendation in music information retrieval. By modeling the distribution of datasets, generative machine learning models are especially suited for this task, potentially reducing the need for cumbersome skipping through sample libraries, or fiddling with numerous controls of a complex synthesizer in order to realize an idea.
An alternative to generate-then-curate is exploration through higher-level control . Rather than producing a batch of possibilities at once, this strategy is interactive, allowing an artist to explore variations of an idea by varying the controls of the tool.
A prominent example of exploration in generative models is the navigation of latent spaces , which may or may not have a clear semantic interpretation, depending on how the model was trained ( Nistal et al., 2020 ; Aouameur et al., 2019 ; Engel et al., 2019 ).
BassNet/LeadNet and DrumNet also provide control over the output by way of latent space navigation, but here the output is conditioned on one or more input audio tracks. Importantly the latent space in these models does not provide absolute control over the output, which would defeat the purpose of the conditioning input audio, but rather they modify the way the models react to the input. The models are trained in such a way that the latent space is discouraged to encode (and thus provide control over) any qualities of the output that are (largely) constrained by the input. In DrumNet for instance, the tempo and metrical grid are inferred from the input, whereas the latent space encodes control over the remaining degrees of freedom for the drum track, such as its rhythmic patterns and the density of rhythmic events.
4.3.3 AI, the new analog?
Although the machine learning models that power AI tools are trained to perform specific tasks (e.g. to produce a specific type of output given a specific type of input), once an AI prototype is in the hands of artists, the scope and limitations of a model are regularly ignored, or even actively exploited. Here we cover three kinds of examples.
Glitches. Machine learning models often have some degree of systematic bias, which leads outputs to have some persistent characteristics that are not representative of the dataset they were trained on. Although this is considered a fault in terms of machine learning theory, musicians sometimes point out that they like particular artifacts for having a characteristic identity . Twenty9 speaks about the Impact Drums and Planet Drums prototype:
“[…] I love [the artifacts’] color, it changes from what I hear in the current available packs that do a lot of recycling. […] Artistically, this grain is interesting […], it is the fact of not being able to accentuate it, modify it or even play with it, which is slowing down and which limits the possibilities of sound palettes.”
Uèle Lamore speaks similarly about the Notono prototype:
“The biggest weakness of Notono at [this] moment [in development], was its extreme treatment of sound. This resulted in the creation of very “phasy”, filtered, samples with a very peculiar acoustic quality. However this was absolutely perfect to represent the Corruption of the Forest [song title], an unnatural, evil substance slowly spreading like a disease.”
Such a music process is reminiscent of the analog synth’s “grain”—a perceptual quality associated with raw, unpolished sound—that is so much sought after by pop musicians.
Of course this does not mean that every imperfection will pass for character. Donn Healy for example is critical of the sound quality of BassNet’s audio outputs, which forces him to apply heavy effect processing in order to obtain a usable sound. Nonetheless he prefers the audio outputs over the corresponding MIDI outputs, both because he is used to working with audio from analog synthesizers, and because he likes the intra-note modulation of intonation, timbre and dynamics that is not present in the MIDI output. 8
The notion of model output confidence can also play a role in the context of glitches. To give an example, Healy found that with an early version of BassNet it was difficult to produce interesting bass lines. A satisfactory resolution of this issue turned out to be simply to tweak the post-processing step of the model that filtered predicted notes based on the model confidence associated with the notes. Allowing more predicted notes to pass—even if the model is uncertain about these notes—produced much more interesting and useful results to Healy’s taste.
Out-of-domain input. Just like all machine learning models, the models that are conditioned on input audio tracks (BassNet/LeadNet, and DrumNet) have been trained on datasets covering a certain range of musical variation. Although the models will still produce outputs for inputs that lie outside that range, the relation between the output and the input is not covered by the training regime. We refer to this scenario as out-of-domain input .
Figure 2 shows a transcription of the input and output of BassNet used with out-of-domain input. 9 This version of BassNet was trained on complete multi-tracks of classic rock songs. However, the input to the example consists of the audio of death metal solo drums. BassNet (bottom-most track) adjusts its output’s spectral envelope to the kick’s attacks, and reacts to the tuning of the percussion, in particular the toms and the snare.
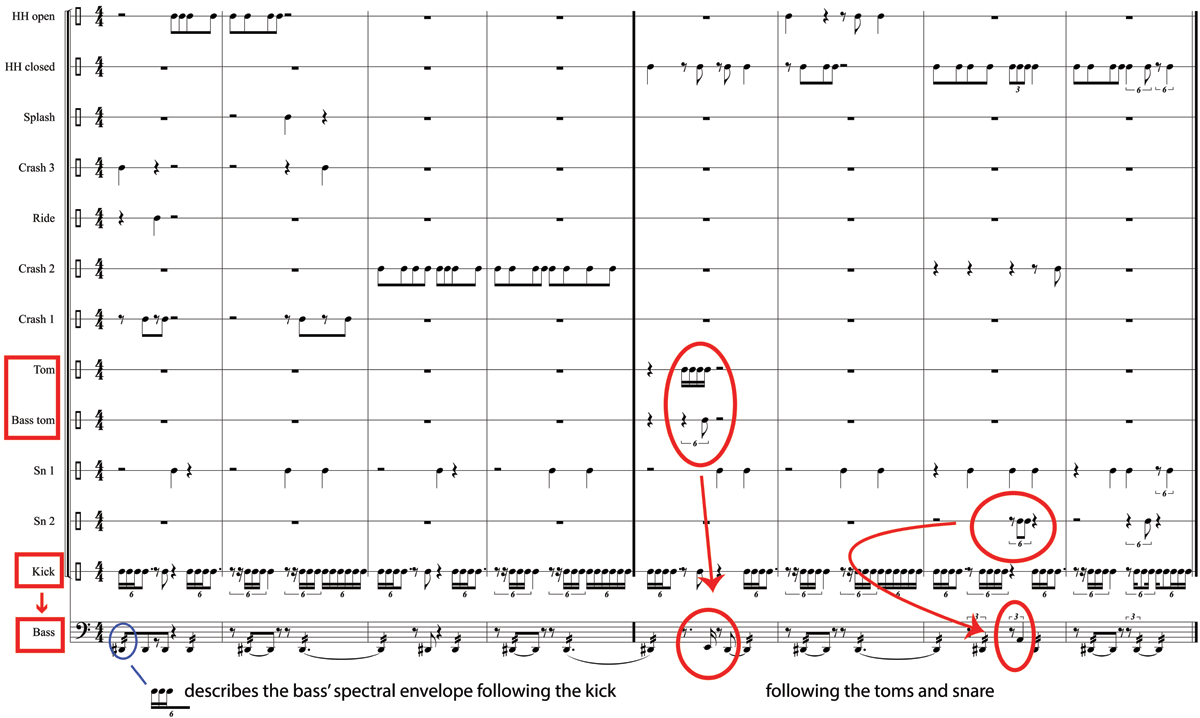
Example of BassNet’s behavior when confronted with out-of-domain input. BassNet (bottom-most track) adjusts its output’s spectral envelope to the kick’s attacks, and reacts to the tuning of the percussion.
Uèle Lamore encounters out-of-domain scenarios as well. She uses a version of BassNet that was trained on rock, pop and EDM multi-tracks with a prominent drum section as input, but her input track has an ambient character. She describes:
“[…] none of my music on this EP is in 4/4, it’s as far as you can get from pop or hip-hop and this track had zero percussion at this point. As a result of this, BassNet did not behave the way you would expect it to. However, I had the pleasant surprise to see the outputs—when transposed upwards by two octaves—were perfect melodies that worked really well in this ambient setting.”
Out-of-domain output. We denote “out-of-domain output” when an artist uses the output of a tool for a different purpose than intended. For example, Donn Healy states:
“I took a new snare pattern that DrumNet suggested and I brought it into a melodic Omnisphere sound, and I spread the notes in a way that they told a musically cohesive story [..] I really enjoyed that.”
Also, as illustrated by Uèle Lamore’s quote above, some artists took output of BassNet and tranposed it to obtain melodies instead of bass lines. Similarly, we discovered that ResonanceEQ, a tool designed to remove resonances, is usually inverted by artists to add resonances to audio.
4.3.4 On machine interference with the creative process
It has been noted before that AI-driven music tools can interfere with musical goals ( Huang et al., 2020 ). We have experienced that even at early stages, prototypes sometimes require compatibility of format (e.g. implementation as DAW plug-ins rather than web applications) and compatibility with the artist’s production methods in order to actually be used.
Even then, artists may be reluctant to depart from their creative goals to include inputs from AI tools. Yet overcoming this barrier can sometimes be rewarding in terms of results. Twenty9 testifies:
“[…]. Since I was a fan of this loop […] I went straight to drums. Honestly, in the euphoria, I wanted to jump on my usual sampler and set a rhythm in 5 min. I forced myself to confront DrumNet […]. To my surprise, […] I ended up with a pattern that worked well [even though] on my own I would not have placed my kicks like that.” “[Working with LeadNet], I am confronted with melodies that I would probably never have thought of.”
Donn Healy reports working with the AI tools changed his workflow into a more conversational process, and shifted his attitude to being more process-oriented rather than result-oriented 10
From artist feedback such as the above we witness creativity emerging from the machine’s interference with the artist’s goals, and even from its effect on their workflow. In this respect it seems that AI music technology offers more than just tools for musicians to produce and manipulate sound in order to realize their creative goals more efficiently. In the words of Gioti ( 2021 ), it enables co-exploration by “breaking creative habits”, and allows one (as an artist) to “reflect on one’s own creative practice and aesthetic values”.
This is in line with contemporary views of the nature of creativity, which have moved from regarding creativity primarily as an attribute of individuals that can be studied in isolation, to a systems perspective in which creativity stems from interactions between individuals, a group of experts that form a social environment (the field ), and a set of rules and practices that form a particular cultural context (the domain ) ( Csikszentmihalyi, 1999 ; Hennessey, 2017 ). Hennion ( 1983 ) and McIntyre ( 2008 ). provide a sociological account of the music production process that is similar in spirit. A more elaborate systems perspective on music production is given by Thompson ( 2019 ), who highlights how the common view of a creative work such as a song sprouting from the mind of the individual genius is a myth. Almost always, he argues, it is the result of interaction between a collective responsible for different aspects of the music production, like recording engineering, mixing, orchestration/arrangement, performance. Often several individuals collaborate even on a single aspect.
What does this type of creative collaboration look like in music production? A common technique is to start with a small idea (a title, lyric, or motif) that serves as a seed to be developed into a full song ( Bennett, 2012 ). Bennett argues that material produced in this way is subsequently evaluated by the individuals involved, and is either assimilated into the end product (through approval, negotiation, or adaptation), or it is rejected by means of a veto.
Although AI music tools are (at this stage) by no means the equivalent of a human creative collaborator to artists, arguably they can play an active role in the creative process. As we have also seen earlier in this Section, current AI tools typically participate in the process described by Bennett ( 2012 ) either by providing starting material (the push/pull generation, and generate-then-curate scenarios), or by exploring variations of existing material, as Notono and PlanetDrums are capable of.
4.4 Interaction and visualization
A recurring theme in feedback from the artists concerns the interaction with the tools. Sometimes artists simply suggest convenience functionality to improve usability, such as keeping a record of past interactions with the tool, or the ability to loop over a sound fragment to enable extensive exploration of control parameters. These suggestions are in line with some of the recommendations for design of creativity-support tools by Shneiderman ( 2007 ). On other occasions they miss control over some aspect of the output. In terms of AI tool development, this may translate to providing better access to latent dimensions, for example by conditioning on particular perceptual dimensions during training.
Another issue, especially in models with learned latent spaces that do not have a pre-defined interpretation, is understanding model behavior as a function of the latent space. Without any visualization, the only way to navigate variations in output is by trial-and-error, trying out different regions of the latent space. Although extended use of a tool may give the user an intuition of how it will react in different circumstances, a better approach is to signal to the user what behavior they can expect in response to the latent space controls. This can be done for example by a-posteriori mapping of the behavior to perceptual features of the output, and projecting these features on the latent space.
An interesting approach to the problem of appropriation of music software by users—the process of getting acquainted with the possibilities and limitations of the tool by exploration—is proposed by Scurto and Bevilacqua ( 2018 ). They observe that user interfaces of music software are often intimidating to novice users, and propose an AI framework to support the appropriation by the user through interactive feedback on the utility or value of some function or feature from the user, combined with reinforcement learning by the framework based on this feedback. A similar approach can also be envisioned to help artists explore latent space controls. More generally, this could lead to a two level design where AI at the first level learns (possibly complex, high-dimensional, and use-case agnostic) relationships or patterns from musical corpora based on generic criteria such as information-theoretic principles ( van den Oord et al., 2018 ; Piantanida and Vega, 2021 ), and AI at the second level assists the user in tailoring the first level into a personalized and use-case specific AI tool.
5 Lessons Learned from AI-Based Musical Research
The open-ended, exploratory nature of the creative process in music production (as in other creative fields) makes the development of AI tools to support and enhance this process an exciting, but certainly also a challenging topic. It is at the intersection of several fields of research, most notably machine learning, creativity studies, and user design.
As Gioti ( 2021 ) observes, there is a tension between this open-ended, under-determined process and the machine learning paradigm, which relies on well-defined problem descriptions and success criteria that are quantifiable in terms of data and model outputs. For the same reason, collaborating with artists in the development of AI tools for music production is not a straight-forward case of participatory design: user needs can be hard to formulate and may change as a result of interacting with the technology.
In this section we summarize some of the lessons we learned throughout our work in AI-based musical research. They relate closely to the notion of creativity-support in human-computer interaction ( Shneiderman, 2007 ; Cherry and Latulipe, 2014 ), and may provide orientation for formulating more rigorous success criteria specific to AI-based creativity support for music production.
5.1 Recommendations
Work alongside musicians. Research is only part of the story. A perfectly well-trained model may be irrelevant in music production. Conversely, in music production, it is not always a problem if the model does not work perfectly. Some systematic bias may even be a mark of style (see Section 4.3.3). Going beyond a proof-of-concept and creating usable tools is the only way to assess which qualities make an AI music tool interesting to artists.
Foster chance/serendipity. Create situations with a rich potential for unexpected results. This may mean using different prototypes together or along with third-party tools, modifying models in an unorthodox way to fit some specific purpose, or using models for applications they weren’t conceived for. In the most extreme case, AI models do not even need to be trained in order to emit musically valuable output ( Steinmetz and Reiss, 2020 ). As we have argued in Section 3.2, AI tools that work with audio offer better chances of a lucky find in the music production process than MIDI tools, simply because they can be driven by a wider range of inputs available in the DAW project. In a more practical vein, enabling artists to benefit from serendipity may be as simple as keeping a record of their interactions with the AI tool, and capturing the outputs (as discussed in the previous section).
AI does not need to entail autonomy. In a recent interview, 11 Uèle Lamore states “The computer wants to play everything perfectly, but the music I make isn’t perfect. The human will always add something of their own.” AI technology for music is sometimes designed in the form of fully autonomous systems creating full songs from only very sparse inputs (e.g. Dhariwal et al., 2020 ). Although they are important demonstrations of the potential of audio based AI systems, they are not well-adapted to typical in-studio composition use cases, where outputs of a music AI tool are often small parts to be integrated in and coordinated with a larger structure of musical elements.
Adapt to the music at hand. As obvious as this sounds, it is not always straight-forward to assess the limitations that assumptions of AI design and modeling approaches may impose on the success and potential of AI music tools. Specifically, as we have argued in Section 3, learning from scores is only partially relevant for contemporary Popular Music. Beyond the music itself, it is helpful to get acquainted with the workflows of artists. When distributing prototypes, using formats that artists are accustomed to, such as DAW plug-ins eliminates an unnecessary barrier to adoption. That said, there may be valid reasons to use less typical music tool formats. For example we found that web-interfaces and client-server architectures facilitate quick iteration and deployment of designs.
5.2 On the validation of AI-driven music technology
AI music tools rely on a machine learning model that is designed and trained to perform a specific task, and as such the most obvious way to validate an AI tool may seem to be to measure how well the machine learning performs its task. Although this is clearly an important criterion in initial stages of development of AI technology, as we have seen in Section 4.3.3, artists tend to use AI tools in ways that defy the basic tenets of machine learning, not seldomly to their satisfaction. The tool may be used on data that is unlike the data the model was trained on, the output may be used in other ways than its intended purpose, and whereas models that produce faithful data samples may be uninteresting to artists, they may have a weak spot for artifacts in the outputs, resulting for example from model bias.
This means that we need other ways of measuring the success of AI-driven music technology in addition to standard machine learning evaluation criteria such as prediction error or accuracy. Here we suggest some criteria that have emerged largely from our collaboration with artists.
Workflow integration. Validation may take into account if a tool finds its place in a production workflow. For that a tool needs to be useful, but also should not interrupt the workflow the artist is accustomed to. For example, artists are often reluctant to switch from their DAW to an external stand-alone application or web-interface. This criterion is not absolute however, and relies on specific and clearly defined use cases. For example, in Section 4.3.4 we discuss how a change in workflow may also be a valid outcome of the use of AI music tools.
Facilitation of production. Does the prototype simplify a difficult or time-consuming task? For instance, Yann Macé appreciates latent space navigation in DrumGAN, as it provides much quicker results than spending hours browsing a drum sample library.
Enhanced creativity. Does the prototype stimulate the artist’s creativity? Does it provide a good trade-off between quality and novelty (i.e., it avoids frustrating the artist with too many useless outputs or cumbersome usability)? For instance, Twenty9 and Uèle Lamore repeatedly mention that BassNet, LeadNet and DrumNet provide solutions they would have never considered, but turned out to be happy with.
Identifiable results. Did the technology bring recognizable elements to the music? For instance, Twenty9 enjoys the grain of the GAN-based drum generators, and Yann Macé appreciates the characteristic style of DrumNet’s hi-hat tracks.
Published content. The commercial compatibility of music content that includes the technology is an indirect form of validation. It should be kept in mind this measure presupposes access to commercial publishing in the first place, and is thus mostly limited to collaborations with professional artists. That said, the integration of AI music tool outputs into the commercial outputs of the artist signals a willingness to endorse the technology in a social context, which is a significant result from the systems perspective on creativity ( Csikszentmihalyi, 1999 ).
Three examples of published content by the collaborating artists that involve the AI tools they worked with:
- A drumkit designed by Twenty9, using Impact Drums, Planet Drums and DrumGAN 12
- An EP by Uèle Lamore and her label, featuring the AI technology described in Section 4.1 13
- A worldwide Azzaro advertisement campaign for which Yann Macé and Luc Leroy of HyperMusic wrote the music track, making use of Impact Drums and DrumGAN. 14
6. Conclusions and Future Work
In this paper we have provided a perspective on the development of AI tools for music production in contemporary Popular Music (CPM) genres, informed both by general considerations of the music production practice in those genres, and by a thematic analysis of reports on real-world AI music tool usage by professional artists.
We have taken a closer look at the in-studio composition process (Section 3) that has become a standard music production practice in many CPM genres, not least because of the increasingly prominent role of electronics and signal processing in music production. There is a discrepancy between what we believe is a widespread view of music production in AI music generation research, and two aspects of the in-studio composition process in particular, namely the role of symbolic representations, and the coupling of composition with sound editing and mixing stages of the process. From these characteristics we conclude that audio based AI tools are better suited to support the creative workflow of the artist than tools that work exclusively with piano-roll/MIDI representations.
In Section 4 we presented a thematic analysis of collaborations of our music AI research lab with professional artists, in which they produced music with various AI tools developed at the lab. Their feedback highlights a variety of aspects to be taken into account in AI tool development, ranging from relatively trivial issues such as the modality of the software (plug-in vs web-interface), to more fundamental issues such as the need for manual control over outputs, and the importance of providing insight in the effect controls have on the output, for example by means of visualizations. The results also show there can be a discrepancy between machine learning success criteria and intended purpose on the one hand, and the utility and value artists find in the AI tools on the other.
We have summarized our findings (Section 5) in the form of recommendations for the development of AI technology for CPM and proposed criteria for validation. We believe these can be a starting point for a more systematic methodology to assess the utility and value of AI music tools in CPM through artist collaborations. More concretely, we plan to use our findings to define a set of specific use-cases within the in-studio composition context, to enable a quantitative assessment of AI technology as creativity support tools using the creativity support index ( Cherry and Latulipe, 2014 ).
Additional Files
The additional files for this article can be found as follows:
Sound example 1. DOI: https://doi.org/10.5334/tismir.100.s1
Donn Healy on sample. DOI: https://doi.org/10.5334/tismir.100.s2
Sound example 2. DOI: https://doi.org/10.5334/tismir.100.s3
Some examples are Landr (mastering), Amper (media post-production), and Amadeuscode (song writing).
https://cslmusicteam.sony.fr/ .
https://www.berklee.edu/news/berklee-now/what-contemporary-music , accessed January 17 2022.
An example of this can be heard in Reach for the dead by Boards of Canada .
Pitched kick drum sounds are a cornerstone of hardcore/hardstyle dance genres, for example.
Parts of the interviews have been edited into published content: Uèle Lamore interview , Whim Therapy interview , Donn Healy interview , Donn Healy process description .
The corresponding audio track is available as Additional File 1 (DOI: https://doi.org/10.5334/tismir.100.s1 ).
Healy discusses his experiences in the video included as Additional File 2 (DOI: https://doi.org/10.5334/tismir.100.s2 ).
The corresponding audio track is available as Additional File 3 (DOI: https://doi.org/10.5334/tismir.100.s3 ).
https://youtu.be/uPvnpZ7mHCM?t=208 .
www.musicradar.com/news/uele-lamore .
The AI Drum-Kit .
Heqets Shadow EP .
Azzaro Advertisement .
Competing Interests
The authors have no competing interests to declare.
Aouameur, C., Esling, P., and Hadjeres, G. (2019). Neural drum machine: An interactive system for real-time synthesis of drum sounds. In Proceedings of the Tenth International Conference on Computational Creativity (ICCC), Charlotte, North Carolina, USA.
Bazin, T., Hadjeres, G., Esling, P., and Malt, M. (2020). Spectrogram inpainting for interactive generation of instrument sounds. In Joint Conference on AI Music Creativity.
Bell, A. P. (2018). Dawn of the DAW: The Studio as Musical Instrument . Oxford University Press. DOI: https://doi.org/10.1093/oso/9780190296605.001.0001
Bennett, J. (2012). Constraint, collaboration and creativity in popular songwriting teams. In Collins, D., editor, The Act of Musical Composition: Studies in the Creative Process , pages 139–69. Ashgate Farnham.
Braun, V., and Clarke, V. (2006). Using thematic analysis in psychology. Qualitative Research in Psychology , 3(2):77–101. DOI: https://doi.org/10.1191/1478088706qp063oa
Briot, J.-P., Hadjeres, G., and Pachet, F.-D. (2020). Deep Learning Techniques for Music Generation . Springer. DOI: https://doi.org/10.1007/978-3-319-70163-9
Burgess, R. J. (2013). The Art of Music Production: The Theory and Practice . Oxford University Press.
Cherry, E., and Latulipe, C. (2014). Quantifying the creativity support of digital tools through the creativity support index. ACM Transactions on Computer-Human Interaction (TOCHI) , 21(4):1–25. DOI: https://doi.org/10.1145/2617588
Chu, H., Urtasun, R., and Fidler, S. (2016). Song from PI: A musically plausible network for pop music generation. arXiv preprint arXiv:1611.03477 .
Clark, E., Ross, A. S., Tan, C., Ji, Y., and Smith, N. A. (2018). Creative writing with a machine in the loop: Case studies on slogans and stories. In 23rd International Conference on Intelligent User Interfaces (IUI), pages 329–340. ACM. DOI: https://doi.org/10.1145/3172944.3172983
Csikszentmihalyi, M. (1997). Creativity: Flow and the Psychology of Discovery and Invention . Harper-Perennial, New York.
Csikszentmihalyi, M. (1999). Implications of a systems perspective for the study of creativity. In Handbook of Creativity . Cambridge University Press, Cambridge, UK. DOI: https://doi.org/10.1017/CBO9780511807916.018
Dhariwal, P., Jun, H., Payne, C., Kim, J. W., Radford, A., and Sutskever, I. (2020). Jukebox: A generative model for music. CoRR , abs/2005.00341.
Engel, J. H., Agrawal, K. K., Chen, S., Gulrajani, I., Donahue, C., and Roberts, A. (2019). GANSynth: Adversarial neural audio synthesis. In 7th International Conference on Learning Representations (ICLR), New Orleans, USA.
Engel, J. H., Resnick, C., Roberts, A., Dieleman, S., Norouzi, M., Eck, D., and Simonyan, K. (2017). Neural audio synthesis of musical notes with WaveNet autoencoders. In Proceedings of the 34th International Conference on Machine Learning (ICML), Sydney, Australia.
Frith, S. (2004). Popular music: Critical concepts in media and cultural studies , volume 1. Psychology Press.
Gibson, J. J. (1977). The theory of affordances. In Shaw, R. and Bransford, J., editors, Perceiving, Acting and Knowing: Toward an Ecological Psychology , pages 67–82. Erlbaum, Hillsdale, New Jersey, USA.
Gioti, A.-M. (2021). Artificial intelligence for music composition. In Handbook of Artificial Intelligence for Music , pages 53–73. Springer. DOI: https://doi.org/10.1007/978-3-030-72116-9_3
Goodfellow, I. J., Pouget-Abadie, J., Mirza, M., Xu, B., Warde-Farley, D., Ozair, S., Courville, A., and Bengio, Y. (2014). Generative adversarial nets. In Advances in Neural Information Processing (NIPS) .
Grachten, M., Deruty, E., and Tanguy, A. (2019). Auto-adaptive resonance equalization using dilated residual networks. In Proceedings of the 20th International Society for Music Information Retrieval Conference (ISMIR), Delft, The Netherlands.
Grachten, M., Lattner, S., and Deruty, E. (2020). Bassnet: A variational gated autoencoder for conditional generation of bass guitar tracks with learned interactive control. Applied Sciences , 10(18). DOI: https://doi.org/10.3390/app10186627
Hadjeres, G., and Crestel, L. (2021). The piano inpainting application. CoRR , abs/2107.05944.
Hadjeres, G., Pachet, F., and Nielsen, F. (2017). Deep-Bach: A steerable model for Bach chorales generation. In Proceedings of the 34th International Conference on Machine Learning (ICML), volume 70, pages 1362–1371, Sydney, Australia.
Hennessey, B. A. (2017). Taking a systems view of creativity: On the right path toward understanding. The Journal of Creative Behavior , 51(4):341–344. DOI: https://doi.org/10.1002/jocb.196
Hennion, A. (1983). The production of success: An anti-musicology of the pop song. Popular Music , 3:159–193. DOI: https://doi.org/10.1017/S0261143000001616
Huang, C.-Z. A., Koops, H. V., Newton-Rex, E., Dinculescu, M., and Cai, C. (2020). AI Song Contest: Human-AI co-creation in songwriting. In Proceedings of the 21st International Society for Music Information Retrieval Conference (ISMIR), pages 708–716, Montreal, Canada.
Ji, S., Luo, J., and Yang, X. (2020). A comprehensive survey on deep music generation: Multi-level representations, algorithms, evaluations, and future directions. ArXiv , abs/2011.06801.
Jones, S. (1992). Rock Formation: Music, Technology, and Mass Communication, volume 3 of Foundations of Popular Culture . Sage Publications. DOI: https://doi.org/10.4135/9781483325491
Kingma, D. P., and Welling, M. (2014). Auto-encoding variational Bayes. In 2nd International Conference on Learning Representations (ICLR), Banff, AB, Canada.
Knotts, S., and Collins, N. (2021). AI-Lectronica: Music AI in clubs and studio production. In Handbook of Artificial Intelligence for Music , pages 849–871. Springer. DOI: https://doi.org/10.1007/978-3-030-72116-9_30
Lashua, B., and Thompson, P. (2016). Producing music, producing myth? Creativity in recording studios. International Association for the Study of Popular Music Journal , 6(2):70–90. DOI: https://doi.org/10.5429/2079-3871(2016)v6i2.5en
Lattner, S., and Grachten, M. (2019). High-level control of drum track generation using learned patterns of rhythmic interaction. In IEEE Workshop on Applications of Signal Processing to Audio and Acoustics (WASPAA), New Paltz, NY, USA. DOI: https://doi.org/10.1109/WASPAA.2019.8937261
Mazzanti, S. (2019). Defining popular music: Towards a “historical melodics”. In Vilotijević, M. D. and Medić, I., editors, Contemporary Popular Music Studies , pages 17–26. Springer VS, Wiesbaden. DOI: https://doi.org/10.1007/978-3-658-25253-3_2
McIntyre, P. (2008). Creativity and cultural production: A study of contemporary Western popular music songwriting. Creativity Research Journal , 20(1):40–52. DOI: https://doi.org/10.1080/10400410701841898
Middleton, R. (1990). Studying popular music . McGraw-Hill Education (UK).
Moore, A. F., and Martin, R. (2018). Rock: The Primary Text: Developing a Musicology of Rock . Routledge. DOI: https://doi.org/10.4324/9780429490170
Moylan, W. (2020). Recording Analysis: How the Record Shapes the Song . CRC Press. DOI: https://doi.org/10.4324/9781315617176
Muller, M. J., and Kuhn, S. (1993). Participatory design. Communications of the ACM , 36(6):24–28. DOI: https://doi.org/10.1145/153571.255960
Nistal, J., Lattner, S., and Richard, G. (2020). Drum-GAN: Synthesis of drum sounds with timbral feature conditioning using generative adversarial networks. In Proceedings of the 21st International Society for Music Information Retrieval Conference (ISMIR), Montreal, Canada.
Payne, C. (2019). Musenet. https://openai.com/blog/musenet/ . Retrieved Feb. 2021.
Piantanida, P., and Vega, L. R. (2021). Information bottleneck and representation learning. In Rodrigues, M. R. D. and Eldar, Y. C., editors, Information-Theoretic Methods in Data Science , chapter 11, pages 330–358. Cambridge University Press. DOI: https://doi.org/10.1017/9781108616799.012
Roberts, A., Engel, J., Mann, Y., Gillick, J., Kayacik, C., Nørly, S., Dinculescu, M., Radebaugh, C., Hawthorne, C., and Eck, D. (2019). Magenta Studio: Augmenting creativity with deep learning in Ableton Live. In Proceedings of the International Workshop on Musical Metacreation (MUME) .
Roberts, A., Engel, J., Raffel, C., Hawthorne, C., and Eck, D. (2018). A hierarchical latent vector model for learning long-term structure in music. In Dy, J. and Krause, A., editors, Proceedings of the 35th International Conference on Machine Learning (ICML), volume 80, pages 4364–4373, Stockholmsmässan, Stockholm Sweden. PMLR.
Scurto, H., and Bevilacqua, F. (2018). Appropriating music computing practices through human-AI collaboration. In Journées d’Informatique Musicale (JIM 2018) , Amiens, France.
Serra, X. (2012). Opportunities for a cultural specific approach in the computational description of music. In Serra, X., Rao, P., Murthy, H., and Bozkurt, B., editors, Proceedings of the 2nd Comp-Music Workshop. Universitat Pompeu Fabra.
Shneiderman, B. (2007). Creativity support tools: Accelerating discovery and innovation. Communications of the ACM , 50:20–32. DOI: https://doi.org/10.1145/1323688.1323689
Steinmetz, C. J., and Reiss, J. D. (2020). Randomized overdrive neural networks. CoRR , abs/2010.04237.
Tagg, P. (1982). Analysing popular music: theory, method and practice. Popular music , 2:37–67. DOI: https://doi.org/10.1017/S0261143000001227
Thompson, P. (2019). Creativity in the Recording Studio: Alternative Takes . Springer. DOI: https://doi.org/10.1007/978-3-030-01650-0
van den Oord, A., Li, Y., and Vinyals, O. (2018). Representation learning with contrastive predictive coding. arXiv preprint arXiv:1807.03748 .
Yang, L.-C., Chou, S.-Y., and Yang, Y.-H. (2017). MidiNet: A convolutional generative adversarial network for symbolic-domain music generation. arXiv preprint arXiv:1703.10847 .
The Rise of Streaming Music and Implications for Music Production
- December 2017
- Review of Network Economics 16(4):351-385
- 16(4):351-385

- Fairfield University

- University of Tulsa
Discover the world's research
- 25+ million members
- 160+ million publication pages
- 2.3+ billion citations
No full-text available

To read the full-text of this research, you can request a copy directly from the authors.
- Daniel Kaimann
- Ilka Tanneberg

- Lilian Marey

- Michael Kandziora

- Marin Milković

- Minghui Jiang
- Wentao Zhan

- Saeed Alaei
- Ali Makhdoumi

- Aimée M. Petitbon

- Rissa Amanda
- Dorothy Rouly Haratua Pandjaitan
- Artaditya Kosasi
- Dwi Asri Siti Ambarwati
- Bram Hadianto

- Dijana Vuković

- Ozana Ramljak

- INF ECON POLICY
- Luis Aguiar
- J Consum Behav

- INT J IND ORGAN

- Christian Essling

- George J. Stigler
- H.R. Varian

- Brett Danaher
- Michael D. Smith

- J MARKETING
- Anita Elberse
- Yannis Bakos

- Michael H. Riordan

- Phillip Leslie

- Marie Connolly
- Alan B. Krueger
- Jeremy Bulow

- Ronald H. Coase
- William James Adams

- R. Preston McAfee
- John McMillan
- Michael D. Whinston

- E. Christman
- Tobias Kretschmer
- Christian Peukert
- Recruit researchers
- Join for free
- Login Email Tip: Most researchers use their institutional email address as their ResearchGate login Password Forgot password? Keep me logged in Log in or Continue with Google Welcome back! Please log in. Email · Hint Tip: Most researchers use their institutional email address as their ResearchGate login Password Forgot password? Keep me logged in Log in or Continue with Google No account? Sign up
Issues Articles Editorials Provocations Conference Papers 2024: Artist Researcher Reflections Content Editorial Articles Conference Papers Interviews Reviews Search About Reviewers Contribute Contact


Examining the Impact of Multiple Technological, Legal, Social and Cultural Factors on the Creative Practice of Sampling Record Producers in Britain.
This paper presents evidence to suggest that, despite the obvious emphasis on the impact of the technologies of sampling and their influence on music producer’s cultural output, there is not one single causal mechanism which can be isolated as the major determining factor in sampling producers’ creative output. Instead, the interplay between a number of factors both constrains and enables their creative practice. These include not only important technological factors but also social, cultural, economic, legal, historical and geographical ones. Sampling producers bring to this complex world their own idiosyncratic social and cultural trajectories and act as agents predisposed to choose what they do within the constraints and possibilities afforded them.
The Discourse of Home Recording: Authority of “Pros” and the Sovereignty of the Big Studios
This article proposes a critical analysis of the discourse of home recording. Driven by enunciations regarding home recording’s accessibility and democratization, it examines the power/knowledge relations that have been produced and legitimized within the discourse. This work shows that the government in home recording seems to be exerted by recording professionals and home recording “pros”. It suggests that the enunciation of democratization legitimizes the discourse’s elitist and excluding aspects. This notion functions as a tool for the exercise of power within the discourse of home recording, one that is intrinsically connected to the norms of the professional studio.
Can We Fix It? – The consequences of ‘fixing it in the mix’ with common equalisation techniques are scientifically evaluated.
This article describes some common equalisation techniques which are regularly employed and discusses their impact on the overall reproduced sound. Sample waveform studies and published recommendations are used to highlight ways to avoid the incorrect and over-use of both analogue and digital equalisation. This article extends further to give scientific explanations as to what effects can be heard and seen at the waveform level when equalisation is incorrectly implemented.
‘You’re Not A Real Dj Unless You Play Vinyl’ – Technology And Formats – The Progression Of Dance Music And Dj Culture
Introduction Drawing on ethnographic research I conducted into the Sydney commercial house music scene between 2002 and 2007, this article explores some of the issues that have arisen in recent years in regard to the changes in technology that have brought about shifts not only in the way DJs play music while performing, but also […]
The Systems Model of Creativity: Analyzing the Distribution of Power in the Studio
It has been proposed that creativity comes about as result of a system in operation rather than, as a Romantic ethos would have it, being the result of the action of single individuals alone. Furthermore, Pierre Bourdieu has argued that the field in which cultural production occurs can be described as an arena of social contestation. Mihaly Csikszentmihalyi suggests, as well, that conflict within a field may also have an effect on that creative field’s output. If these statements are true then questions of power relationships become important in any analysis of creativity. In particular, analyzing Csikszentmihalyi’s systems approach to creativity and Bourdieu’s understanding of cultural production and what these conceptions have to say about the distribution of creative power in the studio may reveal important truths about creativity itself. It may also shed some light on the nature of the collaboration that occurs within creative groups; in this case those that consist of musicians, producers, record companies and technicians.
Waveform Pirates: Sampling, Piracy and Musical Creativity
“Thou shalt not steal” – the (uncited) admonishment from Exodus 20:15 opened Judge Kevin Duffy’s judgment in Grand Upright Music Ltd. v. Warner Bros. Records, Inc. 780 F. Supp. 182 (S.D.N.Y. 1991); a case concerned with two words that have become very familiar in the post-Napster world: music and piracy. But Grand Upright was neither […]
Producer Compensation: Challenges and Options in the New Music Business
The music business is in a transitional phase as the emphasis moves from physical to virtual distribution. There is increased competition for consumer entertainment dollars from many sources including video games and inexpensive DVDs and most music is still available online for free. The industry continues to experience a serious downturn in revenues with US […]
A Multi-Tiered Music Industry?: intellectual property rights, open access and the audience for music
In the recorded music sector, the era of the majors is unlikely to suddenly end; rather what we may see is an enhanced pluralisation of the market for recorded music, which while not being a radical reordering, nevertheless suggests some interesting shifts in the way we enjoy music. In the past the music industry has […]
Nile Rodgers: Navigating Production Space
Nile Rodgers is one of the few black producers to achieve creative and commercial success across multiple genres and decades, but the scope of his contributions rarely attracts detailed analysis despite an enduring influence, partly evidenced by significant sampling of his work in the digital era. This paper focuses primarily on the aesthetics of Rodgers’ production style, encompassing economic realities and ways in which focused creative experimentation can produce both artistically and commercially satisfying results.
Take The Last Train From Meeksville: Joe Meeks’s Holloway Road recording studio 1963-7
Writer and record producer Irwin Chusid called Joe Meek “The Ed Wood of Lo-Fi”[1] and Andy Partridge of XTC commented, “Meek spoke to the dead and heard music from other planets, making number one hit records in his kitchen.”[2] Joe Meek made some great records although I would argue they were not always his most […]
From the Scientific Revolution to Rock: Toward a Sociology of Feedback
For many people, rock’s primal scene is set in a recording studio, in Memphis, in 1954. There, three musicians (Scotty Moore, Bill Black and Elvis Presley), a producer/engineer (Sam Phillips) and a tape recorder (Ampex) create a song (‘All Right Mama’) that durably transforms the physiognomy of music. In this article, I examine the technological, political and intellectual circumstances that made this event possible. One word holds pride of place in my discussion: feedback, a mode of organisation that originated in British scientific laboratories of the eighteenth century.
Divide and Conquer: Power, Role Formation, and Conflict in Recording Studio Architecture
Throughout the history of recording studios, divisions of space have exerted a tremendous influence over the recording process, and have helped to shape the experiences of every recording participant, from the technicians behind the control room window, engineers and producers, to the musicians on the performance space floor. This article combines historical research with ethnographic inquiry in an attempt to analyze how power is enacted in the studio, and how studio design facilitates and maintains recording studio hierarchy.
back to top
The Journal on the Art of Record Production (JARP) is an international online peer-reviewed journal promoting the interdisciplinary study of record production. Copyright © 2024 JARP
Thank you for visiting nature.com. You are using a browser version with limited support for CSS. To obtain the best experience, we recommend you use a more up to date browser (or turn off compatibility mode in Internet Explorer). In the meantime, to ensure continued support, we are displaying the site without styles and JavaScript.
- View all journals
- Explore content
- About the journal
- Publish with us
- Sign up for alerts
- Open access
- Published: 30 August 2019
The effects of playing music on mental health outcomes
- Laura W. Wesseldijk 1 , 2 ,
- Fredrik Ullén 1 na1 &
- Miriam A. Mosing 1 , 3 na1
Scientific Reports volume 9 , Article number: 12606 ( 2019 ) Cite this article
95k Accesses
24 Citations
251 Altmetric
Metrics details
- Behavioural genetics
- Psychiatric disorders
- Risk factors
The association between active musical engagement (as leisure activity or professionally) and mental health is still unclear, with earlier studies reporting contrasting findings. Here we tested whether musical engagement predicts (1) a diagnosis of depression, anxiety, schizophrenia, bipolar or stress-related disorders based on nationwide patient registers or (2) self-reported depressive, burnout and schizotypal symptoms in 10,776 Swedish twins. Information was available on the years individuals played an instrument, including their start and stop date if applicable, and their level of achievement. Survival analyses were used to test the effect of musical engagement on the incidence of psychiatric disorders. Regression analyses were applied for self-reported psychiatric symptoms. Additionally, we conducted co-twin control analyses to further explore the association while controlling for genetic and shared environmental confounding. Results showed that overall individuals playing a musical instrument (independent of their musical achievement) may have a somewhat increased risk for mental health problems, though only significant for self-reported mental health measures. When controlling for familial liability associations diminished, suggesting that the association is likely not due to a causal negative effect of playing music, but rather to shared underlying environmental or genetic factors influencing both musicianship and mental health problems.
Similar content being viewed by others
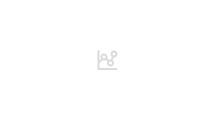
A comprehensive investigation into the genetic relationship between music engagement and mental health
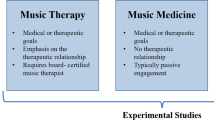
Mental health and music engagement: review, framework, and guidelines for future studies
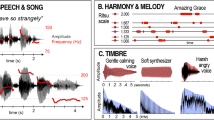
Biological principles for music and mental health
Introduction.
The high suicide rate among famous musicians over the last few years, e.g. Soundgarden’s Chris Cornell, Linkin Park’s Chester Bennington, Avicii and the Prodigy’s Keith Flint, has received a lot of media attention and raised the question about a possible relationship between mental health problems and musicianship. In line with that, a recent survey among 2,211 British self-identified professional musicians found musicians to be up to three times more likely to report depressive problems than individuals in the general population 1 . Furthermore, several famous people engaged in creative professions other than musicianship were also known for their psychiatric illnesses, like Vincent van Gogh, Ernest Hemingway or John Nash. It has been shown that unaffected relatives of individuals with bipolar disorder or schizophrenia have higher levels of creativity 2 , 3 , 4 , 5 . Overall, such findings suggest that creativity and musicianship are risk factors for mental health problems.
On the other hand, there are many studies that report positive relationships between musical engagement and indicators of mental health, thus suggesting the opposite, namely that engagement in music could be protective against psychiatric problems. Although epidemiological studies investigating the association between music and the risk of mental health problems are rare – for a review, see 6 – the few existing ones all tend to suggest a positive effect of music 7 . For example, singing or playing music has been reported to be have a positive influence on various subjective health outcomes, including anxiety and depression 8 . Singing in a choir is related to higher self-rated quality of life and satisfaction with health 9 , and playing an instrument, and singing or performing in theater, tend to be associated with increased self-rated health in women, but decreased all-cause mortality in men and not vice versa 10 . Hours of music practice has been shown to be associated with lower alexithymia (i.e., a dysfunction in emotional awareness, social attachment, and interpersonal relating) 11 . Finally, in 50,797 Norwegian males, but not in females, it was found that active participation in music, singing or theater predicted significantly lower depressive symptoms 12 . It is important to note that the measures of health outcomes in these studies are retrospective self-reports. Therefore, the outcomes could partly reflect characteristics of the rater and may be subject to a recall bias.
Furthermore, there are numerous reviews on the effect of music interventions, both active (e.g. performing) and passive (e.g. listening), on individuals in clinical settings, e.g. during medical procedures or in mental health clinics (for reviews in children, see 13 , 14 , 15 , 16 , 17 ; for reviews in adults, see 18 , 19 , 20 ). The majority of reviews conclude that music interventions have a positive effect on pain, mood, and anxious or depressive symptoms in both children and adults in clinical settings. This suggests not only a positive association in line with the epidemiological research, but also potentially a causal relationship. It is important to note that most of the music interventions described in these studies have been tailored to address individually assessed needs of a client by a music therapist, which differs significantly from self-initiated musical engagement in daily life. Furthermore, as pointed out in most of these reviews, it is difficult to draw firm conclusions about protective effect of music due to the mixed quality of many of the conducted studies, i.e., studies had small samples, suffered from bias due to methodological issues, and there was great variability among the results of the studies.
In sum, the direction of the association between musical engagement and mental health is still unclear with powerful population based research still failing to establish a relation unequivocally. Furthermore, it seems that differentiating between active amateur and professional musicians might explain the discrepancy between, on the one hand research reporting beneficial effects of music in everyday life on mental health, and on the other hand the high rate of depression and suicides among professional musicians. This view is in line with findings from the recent study of Bonde, et al . 21 in which active professional musicians reported more health problems than active amateur musicians, while active amateur musicians reported significantly better self-reported health than non-musicians. Possibly, the strain and pressure experienced by professional musicians may override a possible overall positive effect of musical engagement. Furthermore, an association between engagement in music and mental health problems on a population level does not necessarily reflect causal effects; it could also reflect reverse causation or underlying shared genetic or shared environmental factors that influence both the choice to engage in music and the development of psychiatric problems. It is well known that genetic factors play a role both in mental health problems 22 and in individual variation in music-related abilities 23 . In line with that, there is evidence that the association between creativity and psychiatric disorders is largely driven by underlying shared genetic factors 24 . Studying twins can reduce genetic and shared environmental confounding and strengthen causal inferences.
Here, using a large genetically informative sample of Swedish twins, we aim to investigate whether there is an association between active musical engagement defined by whether an individual plays an instrument, on an amateur and professional level, and mental health and if so, whether the relationship is consistent with a causal hypothesis, i.e., that musical engagement truly affects mental health. We use data from the Swedish nationwide in-patient and outpatient registers for psychiatric diagnoses (i.e., diagnosis of depression, anxiety disorder, schizophrenia, bipolar, stress disorder) as well as self-reports on mental health problems (depressive, burnout and schizotypal symptoms). As the association between playing sport and mental health is already well established, we conducted sensitivity analyses investigating a protective effect of sport against psychiatric problems in this sample.
Participants
Data for the present study was collected as part of “the Study of Twin Adults: Genes and Environment” (STAGE), a sub-study in a cohort of approximately 32,000 adult twins registered with the Swedish Twin Register (STR). The STAGE study sent out a web survey in 2012–2013 inquiring about, musical engagement and musical achievement and other potentially music related traits. The 11,543 responders were aged between 27 and 54 years and data were available for 10,776 individuals on musical engagement and for 6,833 on musical achievement.
The National Patient Register (NPR) records the use of the health care system in Sweden, which has nationwide coverage ensuring equal access to health care for all residents, using a 10-digit personal identification number assigned to all Swedish residents 25 . The NPR includes an in-patient register (IPR) and out-patient register (OPR). The IPR contains information about hospitalizations since 1964 (with full national coverage since 1977), while the OPR covers outpatient visits since 2001 26 . The Cause of Death Register (CDR) contains information from death records since 1961 27 . The Swedish twins from the STAGE study were linked to records from the IPR, OPR and CDR.
Informed consent was obtained from all participants. The study was approved by the Regional Ethics Review Board in Stockholm (Dnr 2011/570-31/5, 2012/1107-32, 2018/866-32). All research methods were performed in accordance with relevant guidelines and regulations.
Musical engagement
Participants were asked whether they ever played an instrument. Those who responded positively were asked at what age they started to play, whether they still played an instrument and, if not, at what age they stopped playing. From these questions, a music status variable was created (0: does not play, 1: used to play, 2: plays).
Sport engagement
Participants reported on whether they ever actively trained a sport (excluding exercise training or physical activity in general). Information on age they started training a sport, whether they still played and at what age they stopped playing resulted in a sport status variable with 0 ‘does not play’, 1 ‘used to play’ and 2 ‘plays sport’.
Musical achievement
Musical achievement was measured with a Swedish version of the Creative Achievement Questionnaire (CAQ) that assesses different domains of creativity, including music 28 , 29 . Individuals were asked to rate their musical achievement on a seven-point scale: 1 ‘I am not engaged in music at all’, 2’I have played or sang privately, but I have never played, sang or showed my music to others’, 3’I have taken music lessons, but I have never played, sang or showed my music to others’, 4 ‘I have played or sung, or my music has been played in public concerts in my home town, but I have not been paid for this’, 5 ‘I have played or sung, or my music has been played in public concerts in my home town, and I have been paid for this’, 6 ‘I am professionally active as a musician’ and 7 ‘I am professionally active as a musician and have been reviewed/featured in national or international media and/or have received an award for my musical activities’. To differentiate between amateur and professional musicians, we converted the scale to three groups: 1 ‘no engagement in music’, 2–4 ‘making music on an amateur level’, and 5–7 ‘professionally active in music’.
Registry-based mental health outcomes
For each individual we derived information (diagnosis and date of first diagnosis) on incidence of depression, anxiety disorder, schizophrenia, bipolar disorder, or stress disorder based on clinical diagnoses after any inpatient or outpatient visit, or underlying cause of death registered in the national registers according to the International Classification of Diseases (ICD) codes as reported in Table 1 . We created an ‘any psychiatric diagnosis’ variable indicating whether the participant has ever been diagnosed with any of the five categories of clinical diagnoses above. For this variable, we selected the earliest date of diagnosis in case of comorbidity.
Questionnaire-based self-reported mental health
In addition, self-reports on mental health outcomes (i.e., depressive, burnout and schizotypal symptoms) obtained in the web survey were analyzed. Depressive symptoms were measured with the depression scale of the Hopkins Symptom Checklist 30 . This scale contains of six items all ranging from 0 to 4 (0 ‘not at all’ to 4 ‘extremely’), measuring depressive symptoms in a work-related context, with higher scores indicating more depressive symptoms. Burn-out symptoms related to work were measured with the Emotional exhaustion subscale of the Maslach Burnout Inventory-General Survey 31 . This scale consists of five items that range from 1 (every day) to 6 (a few times per year or less/never). Therefore, as higher scores reflect less burnout symptoms, we reversed this scale so that higher scores indicate more burnout symptoms in line with the other mental health outcomes. Schizotypal symptoms were measured with the “Positive Dimension Frequency Scale” of the Community Assessment of Psychic Experiences (CAPE) questionnaire 32 . The score is based on 20 positive symptom items that can be answered with four different symptom frequency levels, from 1 ‘never’ to 4 ‘almost always’. Higher scores indicate more schizotypal symptoms. The Cronbach alpha reliability in present study was 0.89 for the depressive symptom scale, 0.87 for the burnout symptom scale and 0.79 for the schizotypal symptom scale.
Level of education
Educational achievement was dichotomized into ‘low and intermediate’ (1 to 7; unfinished primary school to bachelor education) and ‘high’ (8 to 10; master education to PhD).
Statistical analyses
All analyses were conducted in STATA 15.
Survival analyses , i.e., Cox proportional hazard regression, were conducted to explore the effect of musical engagement and musical achievement on the risk to receive a registry-based diagnosis of a psychiatric disorder 33 . Survival analysis is a method to analyze data where the outcome variable is the time until an event happens. The time (years) from the age of twelve to either the date of first receiving a psychiatric diagnosis or to the date of censoring (i.e., date of death or end of follow-up at January 1, 2015) were used as the time scale (i.e., the survival time). For the analyses on the effect of musical engagement , we had to take into account that some individuals had not yet started playing an instrument at the age of twelve (i.e., would start at a later age), or stopped playing at some stage. Therefore, years were split on whether the individual did not play, stopped or started playing, or currently played a musical instrument using the stsplit statement to differentiate between the three levels of musical engagement. We used Cox proportional hazard regressions, a method that assumes the effect upon survival to be constant over time, to calculate hazard ratios (HRs) with 95% confidence intervals. The HRs represent the effects of 1) playing an instrument versus never having played an instrument or 2) having played an instrument (but stopped before diagnosis) versus never having played an instrument on the baseline risk for a mental health diagnosis (independent of playing status) during the follow-up period. A HR value greater than one indicates an increased risk, while a value below one indicates a protective effect. Additionally, we conducted the survival analyses to estimate the effect of musical achievement in a lifetime on the risk of a mental health diagnosis, in which the HRs represent 1) the effect of having performed music as an amateur versus not being involved in music, or 2) the effect of having performed music professionally versus not being involved in music. As we analyzed the three level musical achievement in a lifetime, we did not split years on age (assuming that individuals have been on a lifelong ‘achievement’ trajectory). To correct for relatedness in the twin sample, the robust standard error estimator for clustered observations was used 34 . We fitted separate survival models for each of the five psychiatric disorder diagnoses as well as for the ‘any psychiatric diagnosis’ variable. Thus, first, we in total fitted six models for the effect of musical engagement and another six models for musical achievement. All models included sex as a covariate. Additionally, we fitted all models corrected for level of education, resulting in a small loss of data due to missing information for some individuals, therefore reducing the power. For each model, the proportional hazards assumption was tested using Schoenfeld residuals. No evidence for deviation from the proportional hazards assumption was found for any of the models (all p values > 0.01). As a sensitivity analysis, the above-described models for musical engagement (in which we used the stsplit statement) were repeated with sport engagement as the exposure variable instead, to estimate the effect of playing sport on registry-based psychiatric disorder diagnoses.
Self-reported mental health outcomes
Linear regression analyses were performed to explore the effect of musical engagement and musical achievement on the self-rated continuous measures of depressive symptoms, burnout symptoms and schizotypal symptoms. To correct for relatedness in the twin sample, we used the robust standard error estimator for clustered observations. We included sex as a covariate. Additionally, we ran the analyses corrected for level of education. As a sensitivity analysis, we estimated the effect of sport engagement on depressive, burnout and schizotypal symptoms using linear regression analyses.
Co-twin control analyses (within-pair analyses)
Within-pair analyses in identical twins were conducted to further explore the association between musical engagement and receiving a mental health diagnosis when controlling for genetic and shared environmental factors. As monozygotic (MZ) twins are genetically identical and share their family environment, studying identical twins excludes confounding in case a genetic predisposition or shared environmental influence affects both outcome (mental health problems), and exposure (music engagement). Therefore, if music engagement truly causes a lower/higher risk for receiving a mental health diagnosis, we would expect the MZ twin that plays music to have a lower/higher risk of psychiatric problems than his or her co-twin that does not play music. Conditional Cox regression models, with the strata statement to stratify by pair identifier, were fitted for the mental health diagnoses to estimate HRs with 95% confidence intervals. Notably, only complete identical twin pairs discordant for exposure (i.e., music engagement) and outcome (i.e., the psychiatric disorder diagnosis) contribute to the within-pair analyses. The conditional logistic regression estimates the effect of the difference between the two observations in the strata. Twins are regarded as discordant for the outcome when the time of the psychiatric diagnosis differs. Due to the low prevalence of schizophrenia and bipolar disorder in the complete twin pairs, these phenotypes were excluded from the within-pair analyses.
Additionally, to explore further the effect of music engagement on the self-rated continuous measures of depressive symptoms, burnout symptoms and schizotypal symptoms, we conducted within-pair linear regression analyses using the xtreg fe statement to stratify by twin pair. In within-pair analyses in identical twins correcting for sex is not required as each twin is matched to his or her co-twin. To increase power, we also included data from same-sex dizygotic (DZ) twins (who share on average 50% of their genetic makeup and 100% of their family environment).
Descriptives
Information on mental health outcomes and musical engagement was available for 9,816 individuals [2,212 complete twin pairs (1,055 MZ, 661 dizygotic same-sex (DZ), 496 dizygotic opposite-sex (DOS) twins) and 5,392 individual twins]. Among these individuals, data on musical achievement were available for 6,295 individuals [1,208 complete twin pairs (627 MZ, 342 DZ, 239 DOS) and 3,879 individual twins]. Characteristics of the participants are reported in Table 2 .
Women were more likely to initiate playing an instrument than men (37.7% of men versus 20.5% of women), while roughly the same amount of men and women remained actively involved in music in adulthood (23.3% of men and 21.9% of women). More men (8.5%) than women (5%) played music professionally.
Although overall, there was an overall trend towards a somewhat elevated risk for psychiatric disease in those engaged with music, neither playing music nor having played music in the past (Fig. 1 ), nor professional musicianship (Fig. 2 ) was significantly associated with the risk for any of the psychiatric disorders. The analyses adjusted for level of education showed similar results (see Table S1 for musical engagement and Table S2 for musical achievement, in the supplementary material), with the exception that individuals who played an instrument had a significantly higher risk (39%) of being diagnosed with an anxiety disorder (HR 1.39, CI 1.01–1.92) compared to those who never played an instrument. In terms of covariates, we found females to have a higher risk for depression (92%), anxiety disorder (92%), and stress-related disorders (58%) (Table S1 ). Additionally, individuals with higher levels of education had a significantly lower risk for psychiatric disorders, depression, anxiety disorder, schizophrenia or bipolar disorder (Table S1 ).
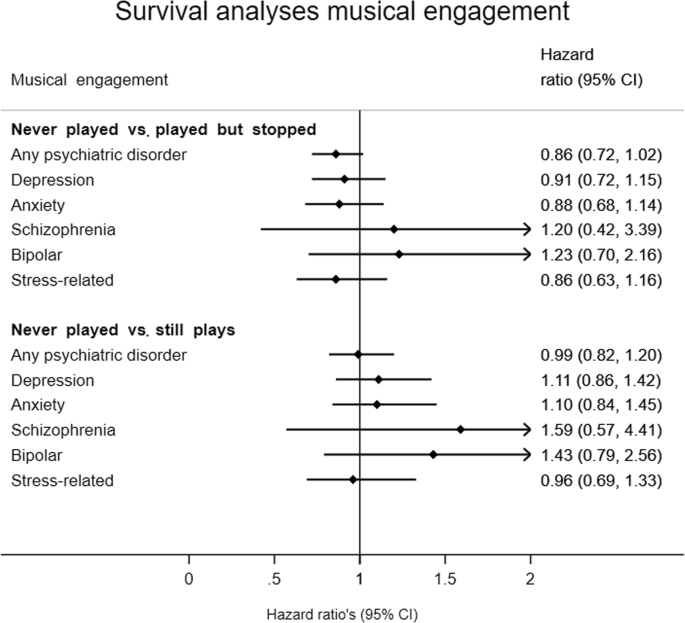
Music engagement and registry-based mental health outcomes. Sex is included as covariate.
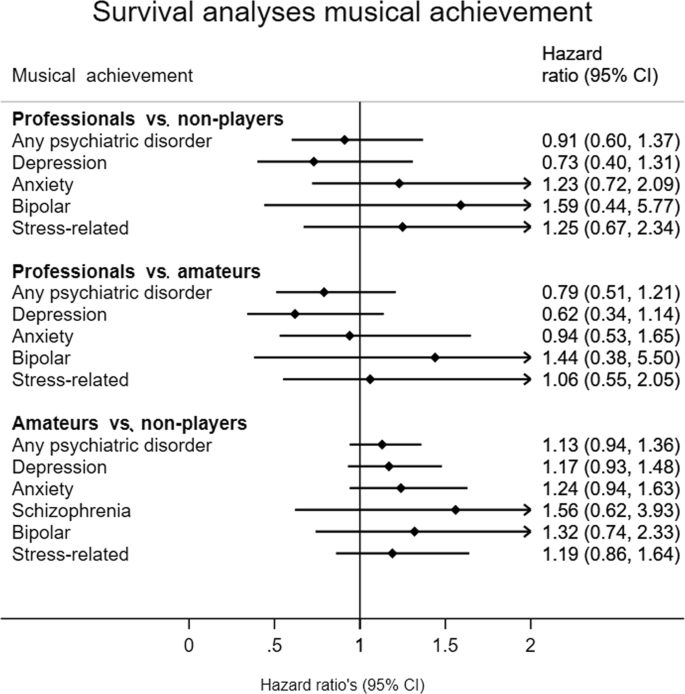
Music achievement and registry-based mental health outcomes. Sex is included as a covariate.
Self-reported mental health
Results of the regression analyses with self-reported mental health symptoms indicated that playing an instrument was significantly associated with more schizotypal symptoms and depressive and burnout symptoms in a work context (see left part of Table 3 ). Having played an instrument in the past did not significantly influence any of the self-rated mental health outcomes. Furthermore, even though professional and amateur musicians report more burnout and schizotypal symptoms than non-players, individuals who played music professionally did not experience significantly more depressive, burnout or schizotypal symptoms than individuals who play music on an amateur level (see right part of Table 3 ). When analyses were repeated adjusting for level of education (results not shown) all results remained the same.
Sensitivity sport analyses
Results of the sensitivity analyses on the registry-based mental health outcomes showed that individuals who actively played sports were less likely to develop any psychiatric disorder, as well as depression, anxiety, and bipolar disorder (see Fig. S1 ). There was no sustained beneficial effect of past sports engagement after stopping with exercise. The analyses adjusted for level of education showed the same results.
Regression analyses on the self-reported mental health outcomes showed that individuals who actively play sports were significantly less likely to report depressive symptoms (β = −0.23, p < 0.001) and burnout symptoms (β = −0.20, p < 0.001), but not schizotypal symptoms (β = 0.00, p = 0.96). Past sport activities were unrelated to the self-reported mental health outcomes (p values range between 0.08 and 0.20). Including level of education in the analyses did not affect the results.
Co-twin control analyses
Results of the co-twin control analyses for both the registry-based and self-reported mental health measures are shown in Table 4 . None of the within-pair estimates were significant. However, overall, the effect sizes (HR or beta) moved closer to zero with increased controlling of shared liability.
We aimed to investigate the association between musical engagement in everyday life and mental health in a large cohort of Swedish twins. Although the findings were somewhat mixed, overall results suggest that individuals who actively play a musical instrument (but not necessarily professionally) may have a somewhat increased risk for mental health problems. However, when controlling for familial liability these associations became weaker and non-significant suggesting that the association is likely explained by underlying shared factors influencing both musicianship and mental health problems.
While analyses using registry-based mental health diagnoses showed no significant association between music playing or professional musical engagement and psychiatric diagnoses, the direction of the effect was trending towards a somewhat increased risk for psychiatric diagnoses for those actively engaged with music. Results from the self-reported mental health outcomes further supported this; individuals playing an instrument report more depressive, burnout and schizotypal symptoms. This is in contrast with previous epidemiological and clinical studies reporting positive effects of musical engagement on anxious and depressive symptoms 6 , 7 , 8 , 9 , 10 , 11 , 12 , 13 , 14 , 15 , 16 , 17 , 18 , 19 , 20 . Further, a recent study by Fancourt and Steptoe 35 found cultural engagement to decrease the development of depression in older ages. However, it appears likely that it is important to distinguish between general cultural engagement, i.e., visits to the theatre, concerts or opera, the cinema or an art gallery, exhibition or museum) and active playing of a musical instrument, which is the focus of the present study. Playing a musical instrument is much more narrowly defined behavior and involves many (cognitive and physical) processes different from engaging in cultural musical activities or listening to music. On the other hand, our findings are in line with results from the survey among British professional musicians 1 and with previous findings of associations between creativity and mental health problems, i.e., that people engaging in creative activities tend to experience more psychiatric problems 4 . It is important to note that the previous epidemiological studies on mental health, the British musicians study, but also our continuous mental health outcomes, were based on self-report. An explanation could be that results of self-report reflect a different attitude towards mental health among more creative individuals, with higher acceptance and awareness of mental health problems, possibly resulting in over-reporting in the field.
Further, there is evidence that the association between creativity and psychiatric disorders can be largely attributed to underlying shared genetic factors 24 , 36 . This is in line with present results of our co-twin control analyses, which showed that the association between musicianship and mental health was attenuated when controlling for genetic and shared environmental confounding (although all analyses were non-significant). This suggests that the observed associations would partly be explained by a shared underlying etiology, (i.e., genetic or family environmental factors which affect both, individuals differences in music playing and mental health) and not by a causal effect of playing music. The within-pair results, however, should be interpreted with caution as only discordant twin pairs contribute to the co-twin control analyses, which reduced the power to find significant associations.
We found significant differences between professional or amateur musicians and non-players in self-rated health outcomes, which are in line with our findings on playing music in general. However, in neither self-rated nor registry-based data, we observed any significant differences in mental health problems between professional musicians compared to amateur musicians. This is in contrast to findings from the study of Bonde, et al . 21 in which active professional musicians reported higher numbers of overall health problems than active amateur musicians, while active amateur musicians reported significantly better self-reported health than non-musicians did. Whilst this was also a large population-based sample, this study analyzed general health instead of mental health, which likely contributes to the difference in findings.
The discrepancy in findings between registry-based mental health diagnoses and self-reported mental health could be due to an influence of rater and recall biases captured in the self-reported mental health outcomes, as discussed above. However, another explanation could be less power in the analyses with the registry-based mental health diagnoses to detect an existing effect. The power of a method to analyze survival time data depends partly on the number of psychiatric diagnoses rather than on the total sample size. In the present sample, observed post-hoc power for the survival analyses to detect a HR of 0.8 for music engagement is 88% for the incidence of a psychiatric disorder, 67% for depression, 61% for anxiety, 7% for schizophrenia, 18% for bipolar and 45% for stress disorder, reflecting the different incident rates of the disorders. As the self-reported mental health problems were measured on a continuous scale, these analyses have higher power (i.e., no cut-off score needs to be reached to obtain a full diagnosis). Nevertheless, our sensitivity analyses in the registry-based outcomes on the effect of sport did show a significant protective effect of sport against the risk of receiving a diagnosis of a psychiatric disorder, depression, anxiety and bipolar disorder in this sample, suggesting that an association can be found with the present distribution of the data if existent. Therefore, we conclude that a lack of power is not a likely explanation for our null findings in the registry-based health outcomes, and that if there truly were an effect, it would be very small.
There are some limitations of this study in addition to the ones we already touched upon. We analyzed data on psychiatric diagnoses obtained from the Swedish nationwide in-patient and outpatient registers. However, the outpatient register only reached full coverage in 2001 and it is therefore possible that some individuals were not classified with a psychiatric disorder, although they did experience mental health problems before 2001. The same holds for individuals with mental health problems who did not visit a doctor. In addition, the dichotomous rather than dimensional nature of psychiatric diagnoses excludes large parts of the continuous variation among individuals in psychiatric problems. The continuous symptom scales increase the power to detect an effect of engagement in music or sports, but may be somewhat biased. Furthermore, our study explored potential effects of active musical engagement (i.e., making music) in everyday life and therefore our findings do not allow for any conclusions about the potential effect of (personalized) musical interventions on mental health problems. Lastly, as mentioned earlier, the sample of discordant twin pairs contributing to the co-twin control analyses was small, resulting in low power to detect effects.
To our knowledge, the present population-based study is the only genetically informative large-scale study to investigate associations between active engagement in music (both as a leisure activity and professionally) and registry-based as well as self-reported mental health outcomes. Rather than a protective effect of music engagement in everyday life as often suggested, our findings suggest that individuals actively engaged in music playing, but not only professional musicians, may have a somewhat elevated risk for mental health problems. This association may at least partly be due to shared underlying etiology and it is unlikely that it reflects a causal effect of playing music.
Gross, S. A. & Musgrave, G. Can Music Make You Sick? Music and Depression. Part I: Pilot Survey Report , https://www.helpmusicians.org.uk/assets/publications/files/1st_nov_can_music_make_you_sick_part_1-_pilot_survey_report_.pdf (2016).
Simeonova, D. I., Chang, K. D., Strong, C. & Ketter, T. A. Creativity in familial bipolar disorder. J Psychiatr Res 39 , 623–631, https://doi.org/10.1016/j.jpsychires.2005.01.005 (2005).
Article PubMed Google Scholar
Lauronen, E. et al . Links between creativity and mental disorder. Psychiatry 67 , 81–98 (2004).
Article Google Scholar
Kyaga, S. et al . Creativity and mental disorder: family study of 300,000 people with severe mental disorder. Br J Psychiatry 199 , 373–379, https://doi.org/10.1192/bjp.bp.110.085316 (2011).
Kyaga, S. et al . Mental illness, suicide and creativity: 40-year prospective total population study. J Psychiatr Res 47 , 83–90, https://doi.org/10.1016/j.jpsychires.2012.09.010 (2013).
Theorell, T. & Kreutz, G. In Music , Health , and Wellbeing Ch. 28, (Oxford University Pres, 2012).
Bonde, L. O. & Theorell, T. Music and Public Health A Nordic Perspective . (Springer International Publishing, 2018).
Ekholm, O., Juel, K. & Bonde, L. O. Associations between daily musicking and health: Results from a nationwide survey in Denmark. Scand J Public Healt 44 , 726–732, https://doi.org/10.1177/1403494816664252 (2016).
Johnson, J. K., Louhivuori, J. & Siljander, E. Comparison of well-being of older adult choir singers and the general population in Finland: A case-control study. Music Sci 21 , 178–194, https://doi.org/10.1177/1029864916644486 (2017).
Lokken, B. Are playing instruments, singing or creating theatre good for health? Associations with self-related health and all-cause mortality in the HUNT3 Study (2006–08), Norway. Eur J Public Health 27 (2017).
Theorell, T., Lennartsson, A. K., Mosing, M. A. & Ullen, F. Musical activity and emotional competence - a twin study. Front Psychol 5 , 774, https://doi.org/10.3389/fpsyg.2014.00774 (2014).
Article PubMed PubMed Central Google Scholar
Cuypers, K. et al . Patterns of receptive and creative cultural activities and their association with perceived health, anxiety, depression and satisfaction with life among adults: the HUNT study, Norway. J Epidemiol Community Health 66 , 698–703, https://doi.org/10.1136/jech.2010.113571 (2012).
Kim, J. & Stegemann, T. Music listening for children and adolescents in health care contexts: A systematic review. Art Psychother 51 , 72–85, https://doi.org/10.1016/j.aip.2016.08.007 (2016).
Yinger, O. S. & Gooding, L. F. A Systematic Review of Music-Based Interventions for Procedural Support. J Music Ther 52 , 1–77, https://doi.org/10.1093/jmt/thv004 (2015).
Kemper, K. J. & Danhauer, S. C. Music as therapy. South Med J 98 , 282–288, https://doi.org/10.1097/01.Smj.0000154773.11986.39 (2005).
Archie, P., Bruera, E. & Cohen, L. Music-based interventions in palliative cancer care: a review of quantitative studies and neurobiological literature. Support Care Cancer 21 , 2609–2624, https://doi.org/10.1007/s00520-013-1841-4 (2013).
Bradt, J., Dileo, C., Magill, L. & Teague, A. Music interventions for improving psychological and physical outcomes in cancer patients. Cochrane Db Syst Rev , https://doi.org/10.1002/14651858.CD006911.pub3 (2016).
Carr, C., Odell-Miller, H. & Priebe, S. A Systematic Review of Music Therapy Practice and Outcomes with Acute Adult Psychiatric In-Patients. Plos One 8 , https://doi.org/10.1371/journal.pone.0070252 (2013).
van der Wal-Huisman, H., Dons, K. S. K., Smilde, R., Heineman, E. & van Leeuwen, B. L. The effect of music on postoperative recovery in older patients: A systematic review. J Geriatr Oncol , https://doi.org/10.1016/j.jgo.2018.03.010 (2018).
Kaptein, A. A., Hughes, B. M., Murray, M. & Smyth, J. M. Start making sense: Art informing health psychology. Health Psychol Open 5 , 2055102918760042, https://doi.org/10.1177/2055102918760042 (2018).
Bonde, L. O., Juel, K. & Ekholm, O. Associations between music and health-related outcomes in adult non-musicians, amateur musicians and professional musiciansResults from a nationwide Danish study. Nord J Music Ther 27 , 262–282, https://doi.org/10.1080/08098131.2018.1439086 (2018).
Polderman, T. J. et al . Meta-analysis of the heritability of human traits based on fifty years of twin studies. Nat genet , https://doi.org/10.1038/ng.3285 (2015).
Mosing, M. A., Madison, G., Pedersen, N. L., Kuja-Halkola, R. & Ullen, F. Practice does not make perfect: no causal effect of music practice on music ability. Psychol Sci 25 , 1795–1803, https://doi.org/10.1177/0956797614541990 (2014).
Power, R. A. et al . Polygenic risk scores for schizophrenia and bipolar disorder predict creativity. Nat Neurosci 18 , 953–955, https://doi.org/10.1038/nn.4040 (2015).
Article CAS PubMed Google Scholar
Ludvigsson, J. F., Otterblad-Olausson, P., Pettersson, B. U. & Ekbom, A. The Swedish personal identity number: possibilities and pitfalls in healthcare and medical research. Eur J Epidemiol 24 , 659–667, https://doi.org/10.1007/s10654-009-9350-y (2009).
Socialstyrelsen. The National Patient Register , https://www.socialstyrelsen.se/register/halsodataregister/patientregistret/inenglish .
Brooke, H. L. et al . The Swedish cause of death register. Eur J Epidemiol 32 , 765–773, https://doi.org/10.1007/s10654-017-0316-1 (2017).
Article CAS PubMed PubMed Central Google Scholar
Carson, S. H., Peterson, J. B. & Higgins, D. M. Reliability, Validity, and Factor Structure of the Creative Achievement Questionnaire. Creat Res 17 , 37–50, https://doi.org/10.1207/s15326934crj1701_4 (2005).
Mosing, M. A., Verweij, K. J., Abe, C., de Manzano, O. & Ullen, F. On the Relationship Between Domain-Specific Creative Achievement and Sexual Orientation in Swedish Twins. Arch Sex Beh 45 , 1799–1806, https://doi.org/10.1007/s10508-016-0708-4 (2016).
Derogatis, L. R., Lipman, R. S., Rickels, K., Uhlenhuth, E. H. & Covi, L. The Hopkins Symptom Checklist (HSCL): A self-report symptom inventory. Behav Sci 19 , 1–15, https://doi.org/10.1002/bs.3830190102 (1974).
Maslach, C., Jackson, S. & Leiter, M. The Maslach Burnout Inventory Manual Vol. 3 191–218 (1997).
Stefanis, N. C. et al . Evidence that three dimensions of psychosis have a distribution in the general population. Psychol Med 32 , 347–358, https://doi.org/10.1017/S0033291701005141 (2002).
Cleves, M. A., Gould, W. & Marchenko, Y. V. An introduction to survival analysis using Stata . Revised third edition. edn, (Stata Press, 2016).
Cameron, C. A. & Miller, D. L. In Handbook of Empirical Economics and Finance (eds Ullah, A. & Giles, D. E. A.) 1–28 (Taylor & Francis, 2011).
Fancourt, D. & Steptoe, A. Cultural engagement predicts changes in cognitive function in older adults over a 10 year period: findings from the English Longitudinal Study of Ageing. Sci Rep 8 , 10226, https://doi.org/10.1038/s41598-018-28591-8 (2018).
Article ADS CAS PubMed PubMed Central Google Scholar
Keller, M. C. & Visscher, P. M. Genetic variation links creativity to psychiatric disorders. Nat Neurosci 18 , 928–929, https://doi.org/10.1038/nn.4047 (2015).
Download references
Acknowledgements
The present work was supported by the Marcus and Amalia Wallenberg Foundation (MAW 2018.0017), and the Bank of Sweden Tercentenary Foundation (M11-0451:1). We acknowledge The Swedish Twin Registry for access to data. The Swedish Twin Registry is managed by the Karolinska Institutet and receives funding through the Swedish Research Council under the grant no 2017-00641. Open access funding provided by Karolinska Institute.
Author information
Fredrik Ullén and Miriam A. Mosing jointly supervised this work.
Authors and Affiliations
Department of Neuroscience, Karolinska Institutet, Solnavägen 9, SE-171 77, Stockholm, Sweden
Laura W. Wesseldijk, Fredrik Ullén & Miriam A. Mosing
Department of Psychiatry, Amsterdam UMC, University of Amsterdam, Meibergdreef 5, 1105 AZ, Amsterdam, The Netherlands
- Laura W. Wesseldijk
Department of Medical Epidemiology and Biostatistics, Karolinska Institutet, Nobels v 12A, 171 77, Stockholm, Sweden
- Miriam A. Mosing
You can also search for this author in PubMed Google Scholar
Contributions
F.U., M.M. and L.W. developed the study design. L.W. performed the data analysis and interpretation under the supervision of F.U., M.M. L.W. and M.M. drafted the manuscript, and F.U. provided critical revisions. All authors approved the final version of the manuscript for submission.
Corresponding author
Correspondence to Laura W. Wesseldijk .
Ethics declarations
Competing interests.
The authors declare no competing interests.
Additional information
Publisher’s note: Springer Nature remains neutral with regard to jurisdictional claims in published maps and institutional affiliations.
Supplementary information
Supplementary tables, rights and permissions.
Open Access This article is licensed under a Creative Commons Attribution 4.0 International License, which permits use, sharing, adaptation, distribution and reproduction in any medium or format, as long as you give appropriate credit to the original author(s) and the source, provide a link to the Creative Commons license, and indicate if changes were made. The images or other third party material in this article are included in the article’s Creative Commons license, unless indicated otherwise in a credit line to the material. If material is not included in the article’s Creative Commons license and your intended use is not permitted by statutory regulation or exceeds the permitted use, you will need to obtain permission directly from the copyright holder. To view a copy of this license, visit http://creativecommons.org/licenses/by/4.0/ .
Reprints and permissions
About this article
Cite this article.
Wesseldijk, L.W., Ullén, F. & Mosing, M.A. The effects of playing music on mental health outcomes. Sci Rep 9 , 12606 (2019). https://doi.org/10.1038/s41598-019-49099-9
Download citation
Received : 04 March 2019
Accepted : 19 August 2019
Published : 30 August 2019
DOI : https://doi.org/10.1038/s41598-019-49099-9
Share this article
Anyone you share the following link with will be able to read this content:
Sorry, a shareable link is not currently available for this article.
Provided by the Springer Nature SharedIt content-sharing initiative
This article is cited by
Can flow proneness be protective against mental and cardiovascular health problems a genetically informed prospective cohort study.
- Emma Gaston
- Fredrik Ullén
Translational Psychiatry (2024)
Translational Psychiatry (2023)
Heritability of Childhood Music Engagement and Associations with Language and Executive Function: Insights from the Adolescent Brain Cognitive Development (ABCD) Study
- Daniel E. Gustavson
- Srishti Nayak
- Hermine H. Maes
Behavior Genetics (2023)
Playing a musical instrument and the risk of dementia among older adults: a systematic review and meta-analysis of prospective cohort studies
- Ahmed Arafa
- Masayuki Teramoto
- Yoshihiro Kokubo
BMC Neurology (2022)
An RCT study showing few weeks of music lessons enhance audio-visual temporal processing
- Crescent Jicol
- Karin Petrini
Scientific Reports (2022)
By submitting a comment you agree to abide by our Terms and Community Guidelines . If you find something abusive or that does not comply with our terms or guidelines please flag it as inappropriate.
Quick links
- Explore articles by subject
- Guide to authors
- Editorial policies
Sign up for the Nature Briefing newsletter — what matters in science, free to your inbox daily.


An official website of the United States government
The .gov means it’s official. Federal government websites often end in .gov or .mil. Before sharing sensitive information, make sure you’re on a federal government site.
The site is secure. The https:// ensures that you are connecting to the official website and that any information you provide is encrypted and transmitted securely.
- Publications
- Account settings
The PMC website is updating on October 15, 2024. Learn More or Try it out now .
- Advanced Search
- Journal List
- Front Psychol
The psychological functions of music listening
Thomas schäfer.
1 Department of Psychology, Chemnitz University of Technology, Chemnitz, Germany
Peter Sedlmeier
Christine städtler, david huron.
2 School of Music, Cognitive and Systematic Musicology Laboratory, Ohio State University, Columbus, OH, USA
Why do people listen to music? Over the past several decades, scholars have proposed numerous functions that listening to music might fulfill. However, different theoretical approaches, different methods, and different samples have left a heterogeneous picture regarding the number and nature of musical functions. Moreover, there remains no agreement about the underlying dimensions of these functions. Part one of the paper reviews the research contributions that have explicitly referred to musical functions. It is concluded that a comprehensive investigation addressing the basic dimensions underlying the plethora of functions of music listening is warranted. Part two of the paper presents an empirical investigation of hundreds of functions that could be extracted from the reviewed contributions. These functions were distilled to 129 non-redundant functions that were then rated by 834 respondents. Principal component analysis suggested three distinct underlying dimensions: People listen to music to regulate arousal and mood , to achieve self-awareness , and as an expression of social relatedness . The first and second dimensions were judged to be much more important than the third—a result that contrasts with the idea that music has evolved primarily as a means for social cohesion and communication. The implications of these results are discussed in light of theories on the origin and the functionality of music listening and also for the application of musical stimuli in all areas of psychology and for research in music cognition.
Introduction
Music listening is one of the most enigmatic of human behaviors. Most common behaviors have a recognizable utility that can be plausibly traced to the practical motives of survival and procreation. Moreover, in the array of seemingly odd behaviors, few behaviors match music for commandeering so much time, energy, and money. Music listening is one of the most popular leisure activities. Music is a ubiquitous companion to people's everyday lives.
The enthusiasm for music is not a recent development. Recognizably musical activities appear to have been present in every known culture on earth, with ancient roots extending back 250,000 years or more (see Zatorre and Peretz, 2001 ). The ubiquity and antiquity of music has inspired considerable speculation regarding its origin and function.
Throughout history, scholars of various stripes have pondered the nature of music. Philosophers, psychologists, anthropologists, musicologists, and neuroscientists have proposed a number of theories concerning the origin and purpose of music and some have pursued scientific approaches to investigating them (e.g., Fitch, 2006 ; Peretz, 2006 ; Levitin, 2007 ; Schäfer and Sedlmeier, 2010 ).
The origin of music is shrouded in prehistory. There is little physical evidence—like stone carvings or fossilized footprints—that might provide clues to music's past. Necessarily, hypotheses concerning the original functions of music will remain speculative. Nevertheless, there are a number of plausible and interesting conjectures that offer useful starting-points for investigating the functions of music.
A promising approach to the question of music's origins focuses on how music is used—that is, it's various functions. In fact, many scholars have endeavored to enumerate various musical functions (see below). The assumption is that the function(s) that music is presumed to have served in the past would be echoed in at least one of the functions that music serves today. Of course, how music is used today need have no relationship with music's function(s) in the remote past. Nevertheless, evidence from modern listeners might provide useful clues pertinent to theorizing about origins.
In proposing various musical functions, not all scholars have related these functions to music's presumed evolutionary roots. For many scholars, the motivation has been simply to identify the multiple ways in which music is used in everyday lives (e.g., Chamorro-Premuzic and Furnham, 2007 ; Boer, 2009 ; Lonsdale and North, 2011 ; Packer and Ballantyne, 2011 ). Empirical studies of musical functions have been very heterogeneous. Some studies were motivated by questions related to development. Many related to social identity. Others were motivated by cognitive psychology, aesthetics, cultural psychology, or personality psychology. In addition, studies differed according to the target population. While some studies attempted to assemble representative samples of listeners, others explicitly focused on specific populations such as adolescents. Most studies rely on convenient samples of students. Consequently, the existing literature is something of a hodgepodge.
The aim of the present study is to use the extant literature as a point of departure for a fresh re-appraisal of possible musical functions. In Part 1 of our study, we summarize the results of an extensive literature survey concerning the possible functions of music. Specifically, we identified and skimmed hundreds of publications that explicitly suggest various functions, uses, or benefits for music. We provide separate overviews for the empirical literatures and the theoretical literatures. This survey resulted in just over 500 proposed musical functions. We do not refer to each of the identified publications but concentrate on the ones that have identified either more than one single function of music listening or a single unique function that is not captured in any other publication. In Part 2, we present the results of an empirical study whose purpose was to distill—using principal components analysis (PCA)—the many proposed functions of music listening. To anticipate our results, we will see that PCA suggests three main dimensions that can account for much of the shared variance in the proposed musical functions.
Review of the research on the functions of music
Discussions and speculations regarding the functions of music listening can be found in both theoretical literature concerning music as well as in empirical studies of music. Below, we offer a review of both literatures. The contents of the reviews are summarized in Tables TablesA1, A1 , ,A2. A2 . Table TableA1 A1 provides an overview of theoretical proposals regarding musical function, whereas Table TableA2 A2 provides an overview of empirical studies regarding musical function. Together, the two tables provide a broad inventory of potential functions for music.
Theoretical approaches
Many scholars have discussed potential functions of music exclusively from a theoretical point of view. The most prominent of these approaches or theories are the ones that make explicit evolutionary claims. However, there are also other, non-evolutionary approaches such as experimental aesthetics or the uses-and-gratifications approach. Functions of music were derived deductively from these approaches and theories. In addition, in the literature, one commonly finds lists or collections of functions that music can have. Most of these lists are the result of literature searches; in other cases authors provide no clear explanation for how they came up with the functions they list. Given the aim of assembling a comprehensive list, all works are included in our summary.
Functions of music as they derive from specific approaches or theories
Evolutionary approaches. Evolutionary discussions of music can already be found in the writings of Darwin. Darwin discussed some possibilities but felt there was no satisfactory solution to music's origins (Darwin, 1871 , 1872 ). His intellectual heirs have been less cautious. Miller ( 2000 ), for instance, has argued that music making is a reasonable index of biological fitness, and so a manifestation of sexual selection—analogous to the peacock's tail. Anyone who can afford the biological luxury of making music must be strong and healthy. Thus, music would offer an honest social signal of physiological fitness.
Another line of theorizing refers to music as a means of social and emotional communication. For example, Panksepp and Bernatzky ( 2002 , p. 139) argued that
in social creatures like ourselves, whose ancestors lived in arboreal environments where sound was one of the most effective ways to coordinate cohesive group activities, reinforce social bonds, resolve animosities, and to establish stable hierarchies of submission and dominance, there could have been a premium on being able to communicate shades of emotional meaning by the melodic character (prosody) of emitted sounds.
A similar idea is that music contributes to social cohesion and thereby increases the effectiveness of group action. Work and war songs, lullabies, and national anthems have bound together families, groups, or whole nations. Relatedly, music may provide a means to reduce social stress and temper aggression in others. The idea that music may function as a social cement has many proponents (see Huron, 2001 ; Mithen, 2006 ; Bicknell, 2007 ).
A novel evolutionary theory is offered by Falk ( 2004a , b ) who has proposed that music arose from humming or singing intended to maintain infant-mother attachment. Falk's “putting-down-the-baby hypothesis” suggests that mothers would have profited from putting down their infants in order to make their hands free for other activities. Humming or singing consequently arose as a consoling signal indicating caretaker proximity in the absence of physical touch.
Another interesting conjecture relates music to human anxiety related to death, and the consequent quest for meaning. Dissanayake ( 2009 ), for example, has argued that humans have used music to help cope with awareness of life's transitoriness. In a manner similar to religious beliefs about the hereafter or a higher transcendental purpose, music can help assuage human anxiety concerning mortality (see, e.g., Newberg et al., 2001 ). Neurophysiological studies regarding music-induced chills can be interpreted as congruent with this conjecture. For example, music-induced chills produce reduced activity in brain structures associated with anxiety (Blood and Zatorre, 2001 ).
Related ideas stress the role music plays in feelings of transcendence. For example, (Frith, 1996 , p. 275) has noted that: “We all hear the music we like as something special, as something that defies the mundane, takes us “out of ourselves,” puts us somewhere else.” Thus, music may provide a means of escape. The experience of flow states (Nakamura and Csikszentmihalyi, 2009 ), peaks (Maslow, 1968 ), and chills (Panksepp, 1995 ), which are often evoked by music listening, might similarly be interpreted as forms of transcendence or escapism (see also Fachner, 2008 ).
More generally, Schubert ( 2009 ) has argued that the fundamental function of music is its potential to produce pleasure in the listener (and in the performer, as well). All other functions may be considered subordinate to music's pleasure-producing capacity. Relatedly, music might have emerged as a safe form of time-passing—analogous to the sleeping behaviors found among many predators. As humans became more effective hunters, music might have emerged merely as an entertaining and innocuous way to pass time during waking hours (see Huron, 2001 ).
The above theories each stress a single account of music's origins. In addition, there are mixed theories that posit a constellation of several concurrent functions. Anthropological accounts of music often refer to multiple social and cultural benefits arising from music. Merriam ( 1964 ) provides a seminal example. In his book, The anthropology of music , Merriam proposed 10 social functions music can serve (e.g., emotional expression, communication, and symbolic representation). Merriam's work has had a lasting influence among music scholars, but also led many scholars to focus exclusively on the social functions of music. Following in the tradition of Merriam, Dissanayake ( 2006 ) proposed six social functions of ritual music (such as display of resources, control, and channeling of individual aggression, and the facilitation of courtship).
Non-evolutionary approaches. Many scholars have steered clear of evolutionary speculation about music, and have instead focused on the ways in which people use music in their everyday lives today. A prominent approach is the “uses-and-gratifications” approach (e.g., Arnett, 1995 ). This approach focuses on the needs and concerns of the listeners and tries to explain how people actively select and use media such as music to serve these needs and concerns. Arnett ( 1995 ) provides a list of potential uses of music such as entertainment, identity formation, sensation seeking, or culture identification.
Another line of research is “experimental aesthetics” whose proponents investigate the subjective experience of beauty (both artificial or natural), and the ensuing experience of pleasure. For example, in discussing the “recent work in experimental aesthetics,” Bullough ( 1921 ) distinguished several types of listeners and pointed to the fact that music can be used to activate associations, memories, experiences, moods, and emotions.
By way of summary, many musical functions have been proposed in the research literature. Evolutionary speculations have tended to focus on single-source causes such as music as an indicator of biological fitness, music as a means for social and emotional communication, music as social glue, music as a way of facilitating caretaker mobility, music as a means of tempering anxiety about mortality, music as escapism or transcendental meaning, music as a source of pleasure, and music as a means for passing time. Other accounts have posited multiple concurrent functions such as the plethora of social and cultural functions of music found in anthropological writings about music. Non-evolutionary approaches are evident in the uses-and-gratifications approach—which revealed a large number of functions that can be summarized as cognitive, emotional, social, and physiological functions—and the experimental aesthetics approach, whose proposed functions can similarly be summarized as cognitive and emotional functions.
Functions of music as they derive from literature research
As noted, many publications posit musical functions without providing a clear connection to any theory. Most of these works are just collections of functions of music from the literature. Not least, there are also accounts of such collections where it remained unclear how the author(s) came up with the functions contained. Some of these works refer to only one single function of music—most often because this functional aspect was investigated not with the focus on music but with a focus on other psychological phenomena. Yet other works list extensive collections of purported musical functions.
Works that refer to only one single functional aspect of music include possible therapeutic functions for music in clinical settings (Cook, 1986 ; Frohne-Hagemann and Pleß-Adamczyk, 2005 ), the use of music for symbolic exclusion in political terms (Bryson, 1996 ), the syntactic, semantic, and mediatizing use of film music (Maas, 1993 ), and the use of music to manage physiological arousal (Bartlett, 1996 ).
The vast majority of publications identify several possible musical functions, most of which—as stated above—are clearly focused on social aspects. Several comprehensive collections have been assembled, such as those by Baacke ( 1984 ), Gregory ( 1997 ), Ruud ( 1997 ), Roberts and Christenson ( 2001 ), Engh ( 2006 ), and Laiho ( 2004 ). Most of these studies identified a very large number of potential functions of music.
By way of summary, there exists a long tradition of theorizing about the potential functions of music. Although some of these theories have been deduced from a prior theoretical framework, none was the result of empirical testing or exploratory data-gathering. In the ensuing section, we turn to consider empirically-oriented research regarding the number and nature of potential musical functions.
Empirical investigations
A number of studies have approached the functions of music from an empirical perspective. Two main approaches might be distinguished. In the first approach, the research aim is to uncover or document actual musical functioning. That is, the research aims to observe or identify one or more ways in which music is used in daily life. In the second approach, the research goal is to infer the structure or pattern underlying the use of music. That is, the research aims to uncover potential basic or fundamental dimensions implied by the multiple functions of music. This is mostly done using PCA or factor analyses or cluster analyses that reduce a large number of functions to only a few basic dimensions. In some cases, the analyses are run exploratively whereas in other cases, they are run in a confirmatory way, that is—with a predefined number of dimensions. The empirical studies can be categorized according to several criteria (see Table TableA2). A2 ). However, when discussing some of the most important works here, we will separate studies where respondents were asked for the functions of music in open surveys from studies where the authors provided their own collections of functions, based on either literature research or face validity.
Surveys about the functions music can have
A number of studies have attempted to chronicle the broad range of musical functions. Most of these studies employed surveys in which people were asked to identify the ways in which they make use of music in their lives. In some studies, expert interviews were conducted in order to identify possible functions. Table TableA2 A2 provides a summary of all the pertinent studies including their collections of functions and—where applicable—their derived underlying dimensions. We will restrict our ensuing remarks to the largest and most comprehensive studies.
Chamorro-Premuzic and Furnham ( 2007 ) identified 15 functions of music among students and subsequently ran focus groups from which they distilled three distinct dimensions: emotional use, rational use, and background use. Some of the largest surveys have been carried out by Boer ( 2009 ). She interviewed more than a thousand young people in different countries and assembled a comprehensive collection of musical functions. Using factor analysis, she found 10 underlying dimensions: emotion, friends, family, venting, background, dancing, focus, values, politic, and culture. (Lonsdale and North, 2011 , Study 1) pursued a uses-and-gratifications approach. They identified 30 musical uses that could be reduced to six distinct dimensions. In a related study employing a larger sample, the same authors came up with eight distinct dimensions: identity, positive and negative mood management, reminiscing, diversion, arousal, surveillance, and social interaction (Lonsdale and North, 2011 , Study 4). When interviewing older participants, Hays and Minichiello ( 2005 ) qualitatively identified six dimensions: linking, life events, sharing and connecting, wellbeing, therapeutic benefits, escapism, and spirituality.
The various surveys and interview studies clearly diverge with regard to the number of different musical functions. Similarly, the various cluster and factor analyses often end up producing different numbers of distinct dimensions. Nevertheless, the results are often quite similar. On a very broad level, there are four categories that appear consistently: social functions, emotional functions, cognitive or self-related functions, and physiological or arousal-related functions (see also Hargreaves and North, 1999 ; Schäfer and Sedlmeier, 2009 , 2010 ).
Empirical studies using predefined collections of functions of music
Apart from the open-ended surveys and interview methods, a number of studies investigating musical functions begin with researcher-defined collections or even categories/dimensions. Some of these predefined collections or categories/dimensions were simply borrowed from the existing published research, whereas others were derived from specific theoretical perspectives.
Empirical studies on functions of music emerging from specific theoretical approaches. Some of the above mentioned theoretical approaches to the functionality of music have been investigated in empirical studies. Boehnke and Münch ( 2003 ) developed a model of the relationship of adolescents' development, music, and media use. They proposed seven functions of music that relate to the developmental issues of young people (such as peer group integration, physical maturation, or identity development). In two studies with a large number of participants, Lonsdale and North ( 2011 ) applied the model of media gratification (from McQuail et al., 1972 ) and used a collection of 30 functions of music they assembled from literature research and interviews. In both studies, they ran factor analyses—reducing the number of functions to six dimensions and eight dimensions, respectively. Lehmann ( 1994 ) developed a situations-functions-preference model and proposed that music preferences emerge from the successful use of music to serve specific functions for the listener, depending on the current situation. Lehmann identified 68 ways in which people use music, from which he was able to reduce them to 15 music reception strategies (Rezeptionsweisen) such as compensation/escapism, relaxation, and identification. Misenhelter and Kaiser ( 2008 ) adopted Merriam's ( 1964 ) anthropological approach and attempted to identify the functions of music in the context of music education. They surveyed teachers and students and found six basic functions that were quite similar to the ones proposed by Merriam ( 1964 ). Wells and Hakanen ( 1997 ) adopted Zillmann's ( 1988a , b ) mood management theory and identified four types of users regarding the emotional functions of music: mainstream, music lover, indifferent, and heavy rockers.
Empirical studies on functions of music emerging from literature research. A number of studies have made use of predefined musical functions borrowed from the existing research literature. The significance of these functions and/or their potential underlying structure has then been empirically investigated using different samples. As mentioned, not all of those studies tried to assemble an exhaustive collection of musical functions in order to produce a comprehensive picture of the functions of music; but many studies were focused on specific aspects such as the emotional, cognitive, or social functions of music.
Schäfer and Sedlmeier ( 2009 ) collected 17 functions of music from the literature and found functions related to the management of mood and arousal as well as self-related functions to be the ones that people highly ascribe to their favorite music. Tarrant et al. ( 2000 ) used a collection of 10 functions of music from the literature and factor analyzed them resulting in three distinct dimensions of music use: self-related, emotional, and social.
Sun and Lull ( 1986 ) collected 18 functions of music videos and were able to reduce them to four dimensions: social learning, passing time, escapism/mood, and social interaction. Melton and Galician ( 1987 ) identified 15 functions of radio music and music videos; and Greasley and Lamont ( 2011 ) collected 15 functions of music, as well. Ter Bogt et al. ( 2011 ) collected 19 functions of music from the literature and used confirmatory factor analysis to group them into five dimensions. In a clinical study with adolescents, Walker Kennedy ( 2010 ) found 47 functions of music that could be reduced to five dimensions.
By way of summary, extant empirical studies have used either an open approach—trying to capture the variety of musical functions in the course of surveys or questionnaire studies—or predefined collections of functions as they resulted from specific theoretical approaches or from literature research. These different approaches have led to quite heterogeneous collections of possible musical functions—from only few functions posited by a specific hypothesis, to long lists arising from open surveys. Moreover, although the many attempts to distill the functions of music to fewer dimensions have produced some points of agreement, the overall picture remains unclear.
The structure among the functions of music
With each successive study of musical functions, the aggregate list of potential uses has grown longer. Questionnaire studies, in particular, have led to the proliferation of possible ways in which music may be relevant in people's lives. Even if one sidesteps the question of possible evolutionary origins, the multitude of hundreds of proposed functions raises the question of whether these might not be distilled to a smaller set of basic dimensions.
As noted earlier, previous research appears to converge on four dimensions: social functions (such as the expression of one's identity or personality), emotional functions (such as the induction of positive feelings), cogni tive or self-related functions (such as escapism), and arousal-related functions (such as calming down or passing time). These four dimensions might well account for the basic ways in which people use music in their daily lives.
Notice that cluster analysis and PCA/factor analysis presume that the research begins with a range of variables that ultimately capture all of the factors or dimensions pertaining to the phenomenon under consideration. The omission of even a single variable can theoretically lead to incomplete results if that variable proves to share little variance in common with the other variables. For example, in studying the factors that contribute to a person's height, the failure to include a variable related to developmental nutrition will led to deceptive results; one might wrongly conclude that only genetic factors are important. The validity of these analyses depends, in part, on including a sufficient range of variables so that all of the pertinent factors or dimensions are likely to emerge.
Accordingly, we propose to address the question of musical functions anew, starting with the most comprehensive list yet of potential music-related functions. In addition, we will aim to recruit a sample of participants covering all age groups, a wide range of socio-economic backgrounds, and pursue our analysis without biasing the materials to any specific theory.
Fundamental functions of music—a comprehensive empirical study
The large number of functions of music that research has identified during the last decades has raised the question of a potential underlying structure: Are there functions that are more fundamental and are there others that can be subsumed under the fundamental ones? And if so, how many fundamental functions are there? As we have outlined above, many scientists have been in search of basic distinct dimensions among the functions of music. They have used statistical methods that help uncover such dimensions among a large number of variables: factor analyses or cluster analyses.
However, as we have also seen, the approaches and methods have been as different as the various functions suggested. For instance, some scholars have focused exclusively on the social functions of music while others have been interested in only the emotional ones; some used only adolescent participants while others consulted only older people. Thus, these researchers arrived at different categorizations according to their particular approach. To date, there is still no conclusive categorization of the functions of music into distinct dimensions, which makes psychological studies that rely on the use of music and its effects on cognition, emotion, and behavior still difficult (see also Stefanija, 2007 ). Although there exist some theoretically driven claims about what fundamental dimensions there might be (Tarrant et al., 2000 ; Laiho, 2004 ; Schubert, 2009 ; Lonsdale and North, 2011 ), there has been no large-scale empirical study that analyzed the number and nature of distinct dimensions using the broad range of all potential musical functions—known so far—all at once.
We sought to remedy this deficiency by assembling an exhaustive list of the functions of music that have been identified in past research and putting them together in one questionnaire study. Based on the research reviewed in the first part of this study, we identified more than 500 items concerned with musical use or function. Specifically, we assembled an aggregate list of all the questions and statements encountered in the reviewed research that were either theoretically derived or used in empirical studies. Of course, many of the items are similar, analogous, or true duplicates. After eliminating or combining redundant items, we settled on a list of 129 distinct items. All of the items were phrased as statements in the form “I listen to music because … ” The complete list of items is given in Table TableA3, A3 , together with their German versions as used in our study.
Participants were asked to rate how strongly they agreed with each item-statement on a scale from 0 ( not at all ) to 6 ( fully agree ). When responding to items, participants were instructed to think of any style of music and of any situation in which they would listen to music. In order to obtain a sample that was heterogeneous with regard to age and socioeconomic background, we distributed flyers promoting the Internet link to our study in a local electronics superstore. Recruitment of participants was further pursued via some mailing lists of German universities, students from comprehensive schools, and members of a local choir. As an incentive, respondents got the chance to win a tablet computer. A total of 834 people completed the survey. Respondents ranged from 8 to 85 years of age ( M = 26, SD = 10.4, 57% female).
Notice that in carrying out such a survey, we are assuming that participants have relatively accurate introspective access to their own motivations in pursuing particular musical behaviors, and that they are able to accurately recall the appropriate experiences. Of course, there exists considerable empirical research casting doubt on the accuracy of motivational introspection in self-report tasks (e.g., Wilson, 2002 ; Hirstein, 2005 ; Fine, 2006 ). These caveats notwithstanding, in light of the limited options for gathering pertinent empirical data, we nevertheless chose to pursue a survey-based approach.
Principal component analysis revealed three distinct dimensions behind the 129 items (accounting for about 40% of the variance), based on the scree plot. This solution was consistent over age groups and genders. The first dimension (eigenvalue: 15.2%) includes statements about self-related thoughts (e.g., music helps me think about myself), emotions and sentiments (e.g., music conveys feelings), absorption (e.g., music distracts my mind from the outside world), escapism (e.g., music makes me forget about reality), coping (e.g., music makes me believe I'm better able to cope with my worries), solace (e.g., music gives comfort to me when I'm sad), and meaning (e.g., music adds meaning to my life). It appears that this dimension expresses a very private relationship with music listening. Music helps people think about who they are, who they would like to be, and how to cut their own path. We suggest labeling this dimension self-awareness . The second dimension (eigenvalue: 13.7%) includes statements about social bonding and affiliation (e.g., music helps me show that I belong to a given social group; music makes me feel connected to my friends; music tells me how other people think). People can use music to feel close to their friends, to express their identity and values to others, and to gather information about their social environment. We suggest labeling this dimension social relatedness . The third dimension (eigenvalue: 10.2%) includes statements about the use of music as background entertainment and diversion (e.g., music is a great pastime; music can take my mind off things) and as a means to get into a positive mood and regulate one's physiological arousal (e.g., music can make me cheerful; music helps me relax; music makes me more alert). We suggest labeling this dimension arousal and mood regulation . All factor loadings are reported in Table TableA3 A3 .
In order to analyze the relative significance of the three derived dimensions for the listeners, we averaged the ratings for all items contained in each dimension (see Figure Figure1). 1 ). Arousal and mood regulation proved to be the most important dimension of music listening closely followed by self-awareness. These two dimensions appear to represent the two most potent reasons offered by people to explain why they listen to music, whereas social relatedness seems to be a relatively less important reason (ranging below the scale mean). This pattern was consistent across genders, socioeconomic backgrounds, and age groups. All differences between the three dimensions are significant (all p s < 0.001). The reliability indices (Cronbach's α) for the three dimensions are α = 0.97 for the first, α = 0.96 for the second, and α = 0.92 for the third dimension.

The three distinct dimensions emerging from 129 reasons for listening to music . Error bars are 95% confidence intervals. Self-awareness: M = 3.59 ( SE = 0.037); social relatedness: M = 2.01 ( SE = 0.035); arousal and mood regulation: M = 3.78 ( SE = 0.032).
General discussion
Since the earliest writing on the psychology of music, researchers have been concerned with the many ways in which people use music in their lives. In the first part of this paper, we reviewed literature spanning psychological, musicological, biological, and anthropological perspectives on musical function. The picture that emerged from our review was somewhat confusing. Surveying the literature from the past 50 years, we identified more than 500 purported functions for music. From this list, we assembled a somewhat catholic list of 129 non-redundant musical functions. We then tested the verisimilitude of these posited functions by collecting survey responses from a comparatively large sample. PCA revealed just three distinct dimensions: People listen to music to achieve self-awareness , social relatedness , and arousal and mood regulation . We propose calling these the Big Three of music listening.
In part one of our study we noted that several empirical studies suggest grouping musical functions according to four dimensions: cognitive, emotional, social/cultural, and physiological/arousal-related functions. This raises the question of how our three-dimensional result might be reconciled with the earlier work. We propose that there is a rather straightforward interpretation that allows the four-dimensional perspective to be understood within our three-dimensional result. Cognitive functions are captured by the first dimension (self-awareness); social/cultural functions are captured by the second dimensions (social relatedness); physiological/arousal-related functions are captured by the third dimension (arousal and mood regulation); and emotional functions are captured by the first and third dimensions (self-awareness + arousal and mood regulation). Notably—as can be seen with the items in Table TableA3—there A3 —there is a dissociation of emotion-related and mood-related functions. Emotions clearly appear in the first dimension (e.g., music conveys feelings; music can lighten my mood; music helps me better understand my thoughts and emotions), indicating that they might play an important role in achieving self-awareness, probably in terms of identity formation and self-perception, respectively. However, the regulation of moods clearly appears in the third dimension (e.g., music makes me cheerful; music can enhance my mood; I'm less bored when I listen to music), suggesting that moods are not central issues pertaining to identity. Along with the maintenance of a pleasant level of physiological arousal, the maintenance of pleasant moods is an effect of music that might rather be utilized as a “background” strategy, that is, not requiring a deep or aware involvement in the music. The regulation of emotions, on the other side, could be a much more conscious strategy requiring deliberate attention and devotion to the music. Music psychology so far has not made a clear distinction between music-related moods and emotions; and the several conceptions of music-related affect remain contentious (see Hunter and Schellenberg, 2010 ). Our results appear to call for a clearer distinction between moods and emotions in music psychology research.
As noted earlier, a presumed evolutionary origin for music need not be reflected in modern responses to music. Nevertheless, it is plausible that continuities exist between modern responses and possible archaic functions. Hence, the functions apparent in our study may echo possible evolutionary functions. The three functional dimensions found in our study are compatible with nearly all of the ideas about the potential evolutionary origin of music mentioned in the introduction. The idea that music had evolved as a means for establishing and regulating social cohesion and communication is consistent with the second dimension. The idea of music satisfying the basic human concerns of anxiety avoidance and quest for meaning is consistent with the first dimension. And the notion that the basic function of music could have been to produce dissociation and pleasure in the listener is consistent with the third dimension.
In light of claims that music evolved primarily as a means for promoting social cohesion and communication—a position favored by many scholars—the results appear noteworthy. Seemingly, people today hardly listen to music for social reasons, but instead use it principally to relieve boredom, maintain a pleasant mood, and create a comfortable private space. Such a private mode of music listening might simply reflect a Western emphasis on individuality: self-acknowledgement and well-being appear to be more highly valued than social relationships and relatedness (see also Roberts and Foehr, 2008 ; Heye and Lamont, 2010 ).
The results of the present study may be of interest to psychologists who make use of music as a tool or stimulus in their research. The way people usually listen to music outside the laboratory will surely influence how they respond to musical stimuli in psychological experiments. For those researchers who make use of music in psychological studies, some attention should be paid to how music is used in everyday life. The three dimensions uncovered in this study can provide a parsimonious means to identify the value a person sets on each of three different types of music use. It is also conceivable that individual patterns of music use are related to personality traits, a conjecture which may warrant future research.
With regard to music cognition, the present results are especially relevant to studies about aesthetic preferences, style or genre preferences, and musical choice. Recent research suggests that musical functions play an important role in the formation and development of music preferences (e.g., Schäfer and Sedlmeier, 2009 ; Rentfrow et al., 2011 ). It will be one of the future tasks of music cognition research to investigate the dependence of music preference and music choice on the functional use of music in people's lives.
By way of summary, in a self-report study, we found that people appear to listen to music for three major reasons, two of which are substantially more important than the third: music offers a valued companion, helps provide a comfortable level of activation and a positive mood, whereas its social importance may have been overvalued.
Conflict of interest statement
The authors declare that the research was conducted in the absence of any commercial or financial relationships that could be construed as a potential conflict of interest.
Overview of theoretical contributions that have derived, proposed, or addressed more than one function or functional aspect of music listening .

Overview about empirical studies that have identified and/or investigated more than one function or functional aspect of music listening .

In some places, we could only provide exemplary functions because either the total number of functions was too large to be displayed here or not all functions were given in the original publications .
The 129 statements referring to the functions of music exhaustively derived from past research, together with their means, standard deviations, and factor loadings (varimax rotated) .
Because it helps me think about myself. | |||||
[weil ich dann gut über mich nachdenken kann.] | 3.53 | 1.82 | 0.715 | ||
Because it can lead my thoughts to somewhere else. | |||||
[weil ich dann in Gedanken ganz weit weg sein kann.] | 4.21 | 1.70 | 0.671 | ||
Because it makes me believe I am better able to cope with my worries. | |||||
[weil ich dann das Gefühl habe, mit meinen Sorgen besser fertig zu werden.] | 3.37 | 1.87 | 0.668 | ||
Because it helps me better understand my thoughts and emotions. | |||||
[weil sie hilft, meine Gedanken und Gefühle besser zu verstehen.] | 3.02 | 1.85 | 0.667 | ||
Because it helps me think about my identity. | |||||
[weil sie mir hilft, über meine Identität nachzudenken.] | 2.87 | 1.94 | 0.665 | ||
Because it is therapy for my soul. | |||||
[weil sie eine Therapie für meine Seele ist.] | 3.82 | 1.84 | 0.656 | ||
Because it gives comfort to me when I'm sad. | |||||
[weil sie mir Trost spendet, wenn ich traurig bin.] | 4.10 | 1.73 | 0.645 | ||
Because it makes me feel secure. | |||||
[weil ich mich dann geborgen fühle.] | 3.00 | 1.79 | 0.637 | ||
Because it is a means to express myself. | |||||
[weil sie mir eine Möglichkeit bietet, mich selbst auszudrücken] | 3.39 | 1.89 | 0.632 | ||
Because it helps me find my own way. | |||||
[weil sie mir hilft, meinen Weg zu finden.] | 3.07 | 1.85 | 0.625 | ||
Because it mirrors my feelings and moods. | |||||
[weil ich darin meine Gefühle und Stimmungen wiederfinde.] | 4.92 | 1.31 | 0.610 | ||
Because it conveys feelings. | |||||
[weil sie Gefühle transportiert.] | 4.80 | 1.38 | 0.608 | ||
Because it expresses something that cannot be expressed in words. | |||||
[weil sie etwas vermittelt, was sich in Worten nicht ausdrücken lässt.] | 3.82 | 1.91 | 0.602 | ||
Because it helps me learn about myself. | |||||
[weil ich dadurch etwas über mich lernen kann.] | 2.30 | 1.82 | 0.589 | ||
Because it helps me be contemplative. | |||||
[weil sie mir beim Nachdenken hilft.] | 3.65 | 1.82 | 0.572 | ||
Because it helps me escape from my daily routines. | |||||
[weil ich dann aus dem grauen Alltag fliehen kann.] | 3.62 | 1.85 | 0.567 | ||
Because it often induces visual imagery. | |||||
[weil ich dabei oft bildhafte Vorstellungen habe.] | 3.88 | 1.72 | 0.564 | ||
Because it can make me dream. | |||||
[weil ich dabei träumen kann.] | 4.49 | 1.50 | 0.562 | ||
Because it distracts my mind from the outside world. | |||||
[weil sie mich von der “Außenwelt” ablenkt.] | 3.74 | 1.78 | 0.552 | ||
Because it lets me forget the world around me. | |||||
[weil ich dann die Welt um mich herum vergessen kann.] | 4.48 | 1.57 | 0.551 | ||
Because it makes me forget about reality. | |||||
[weil sie mich die Realität vergessen lässt.] | 3.30 | 1.91 | 0.544 | ||
Because it puts fantastic images or stories in my head. | |||||
[weil mir dann tolle Bilder oder Geschichten in den Kopf kommen.] | 3.88 | 1.76 | 0.543 | ||
Because it alleviates my inner tension. | |||||
[weil das die Anspannung in mir verringert.] | 3.85 | 1.55 | 0.542 | ||
Because it helps me reminisce. | |||||
[weil ich dabei in Erinnerungen schwelgen kann.] | 4.24 | 1.64 | 0.532 | ||
Because it gives me the energy I need for the day. | |||||
[weil sie mir Energie für den Tag gibt.] | 4.44 | 1.47 | 0.531 | ||
Because I can recognize myself in the lyrics. | |||||
[weil ich mich in den Texten wiederfinden kann.] | 3.72 | 1.70 | 0.524 | ||
Because it makes me feel somebody else feels the same as I do. | |||||
[weil sie mir das Gefühl gibt, dass jemand anderes dasselbe fühlt wie ich.] | 3.13 | 1.94 | 0.521 | ||
Because it supports my ideas. | |||||
[weil sie meine Ideen unterstützt.] | 2.86 | 1.82 | 0.512 | ||
Because it lets me be the way I am. | |||||
[weil ich dadurch so sein kann, wie ich bin.] | 3.66 | 1.89 | 0.508 | ||
Because it enables me to experiment with different facets of my personality. | |||||
[weil sie mir ermöglicht, mit verschiedenen Seiten meiner Persönlichkeit zu experimentieren.] | 2.52 | 1.94 | 0.508 | ||
Because it calms me. | |||||
[weil sie mich beruhigt.] | 4.32 | 1.37 | 0.501 | ||
Because it adds meaning to my life. | |||||
[weil sie mir Sinn im Leben gibt.] | 2.24 | 2.03 | 0.496 | ||
Because it can reduce my anxiety. | |||||
[weil sie meine Angst reduzieren kann.] | 2.51 | 1.93 | 0.493 | ||
Because it makes me feel that I want to change the world. | |||||
[weil ich dann das Gefühl bekomme, dass ich die Welt verändern möchte.] | 2.34 | 1.96 | 0.489 | ||
Because it is a means of venting my frustration. | |||||
[weil sie eine Möglichkeit bietet, meine Frustration abzuladen.] | 3.82 | 1.82 | 0.488 | ||
Because it can reduce my stress. | |||||
[weil sie meinen Stress reduziert.] | 4.42 | 1.39 | 0.487 | ||
Because it can make me feel less lonely. | |||||
[weil ich mich dann weniger einsam fühle.] | 2.93 | 1.92 | 0.486 | ||
Because I like the bodily changes it evokes (changes of heartbeat, prickling, etc.) | |||||
[weil ich die körperlichen Wirkungen mag (Veränderung des Herzschlags, Kribbeln auf der Haut usw.), die sie auslöst.] | 3.33 | 2.02 | 0.483 | ||
Because it gives me a way to let off steam. | |||||
[weil ich dadurch Dampf ablassen kann.] | 3.82 | 1.83 | 0.479 | ||
Because it can lighten my mood. | |||||
[weil sie meine Gefühle positiv beeinflussen kann.] | 4.86 | 1.22 | 0.473 | ||
Because it gives me pleasure. | |||||
[weil sie Wohlgefallen auslöst.] | 4.54 | 1.49 | 0.473 | ||
Because it reminds me of certain periods of my life or past experiences. | |||||
[weil sie mich an bestimmte Phasen meines Lebens bzw. an vergangene Ereignisse erinnert.] | 4.62 | 1.51 | 0.471 | ||
Because I just enjoy listening to music. | |||||
[weil ich es einfach genieße, Musik zu hören.] | 5.33 | 1.06 | 0.460 | ||
Because it gives me intellectual stimulation. | |||||
[weil es eine intellektuelle Stimulation für mich ist.] | 2.94 | 1.90 | 0.434 | ||
Because it gives me something that is mine alone. | |||||
[weil ich dann etwas für mich alleine habe.] | 2.58 | 2.00 | 0.418 | ||
Because it gives me goose bumps. | |||||
[weil ich dann Gänsehaut bekomme.] | 3.16 | 1.91 | 0.416 | ||
Because it addresses my sense of aesthetics. | |||||
[weil sie meinen Sinn für Ästhetik anspricht.] | 2.94 | 2.04 | 0.386 | ||
Because it reminds me of a particular person. | |||||
[weil sie mich an eine bestimmte Person erinnert.] | 3.39 | 1.88 | 0.379 | ||
Because it makes me feel my body. | |||||
[weil ich dabei meinen Körper spüre.] | 2.43 | 1.89 | 0.376 | ||
Because I can enjoy it as art. | |||||
[weil ich sie als Kunst genießen kann.] | 3.63 | 1.93 | 0.358 | ||
Because I want to play or sing it myself. | |||||
[weil ich sie nachspielen oder nachsingen möchte.] | 3.13 | 1.98 | 0.316 | ||
Because it helps me show that I belong to a given social group. | 1.30 | 1.62 | 0.726 | ||
[weil ich damit zeigen kann, dass ich einer bestimmten sozialen Gruppe angehöre.] | |||||
Because it makes me feel connected to all people who like the same kind of music. | |||||
[weil ich mich dann allen Leuten zugehörig fühle, die solche Musik hören.] | 1.68 | 1.71 | 0.686 | ||
Because it makes me feel connected to my friends. | |||||
[weil sie dazu führt, dass ich mich mit meinen Freunden verbunden fühle.] | 2.02 | 1.73 | 0.671 | ||
Because it provides me useful information for my everyday life. | |||||
[weil ich dadurch nützliche Informationen für das alltägliche Leben sammeln kann.] | 1.67 | 1.65 | 0.665 | ||
Because it is a reason to meet my friends. | |||||
[weil sie einen Grund dafür bietet, mit meinen Freunden zusammen zu sein.] | 1.86 | 1.73 | 0.662 | ||
Because it makes me feel connected to others. | |||||
[weil ich mich durch sie mit anderen verbunden fühle.] | 2.28 | 1.75 | 0.661 | ||
Because it can help me meet other people. | |||||
[weil ich dadurch neue Leute kennenlernen kann.] | 2.15 | 1.78 | 0.661 | ||
Because it helps me form friendships with people who have similar musical taste. | |||||
[weil sie mir hilft, Freundschaften mit Personen zu schließen, die einen ähnlichen Musikgeschmack haben wie ich.] | 2.17 | 1.82 | 0.660 | ||
Because it tells me how other people think. | |||||
[weil ich dann weiß, wie andere Leute denken.] | 1.89 | 1.72 | 0.636 | ||
Because I can learn something about other people. | |||||
[weil ich dadurch etwas über andere lernen kann.] | 2.49 | 1.76 | 0.629 | ||
Because music is a social experience. | |||||
[weil Musik eine Gruppenerfahrung ist.] | 2.00 | 1.77 | 0.628 | ||
Because it helps me develop social values. | |||||
[weil Musik hilft, soziale Werte zu entwickeln.] | 2.44 | 1.80 | 0.622 | ||
Because I would like to identify with a particular music scene. | |||||
[weil ich mich mit einer bestimmten Musikszene identifizieren möchte.] | 1.75 | 1.83 | 0.608 | ||
Because it helps me understand the world better. | |||||
[weil ich dadurch die Welt besser verstehen kann.] | 2.26 | 1.75 | 0.600 | ||
Because it mirrors the history and culture of my country. | |||||
[weil sie die Kultur und die Geschichte meines Landes widerspiegelt.] | 1.15 | 1.58 | 0.588 | ||
Because it can be a means to show political engagement. | |||||
[weil sie ein wichtiges Mittel für mich ist, um politisches Engagement zu zeigen.] | 1.00 | 1.50 | 0.582 | ||
Because it helps me develop my personal values. | |||||
[weil sie mir hilft, meine persönlichen Werte zu entwickeln.] | 2.34 | 1.79 | 0.581 | ||
Because it is a good way to express the uniqueness of our culture. | |||||
[weil das ein gutes Mittel ist, um die Einzigartigkeit unserer Kultur auszudrücken.] | 1.95 | 1.84 | 0.581 | ||
Because I would like to take the artists/musicians as role models. | |||||
[weil ich mir die Künstler/Musiker als Vorbild nehmen möchte.] | 1.83 | 1.83 | 0.575 | ||
Because it is something my friends like to do, as well. | |||||
[weil das etwas ist, was meine Freundinnen und Freunde auch gerne tun.] | 1.71 | 1.69 | 0.575 | ||
Because it makes me feel connected to the world. | |||||
[weil ich mich dann mit der Welt verbunden fühle.] | 2.03 | 1.76 | 0.571 | ||
Because it is something I can talk about with my friends. | |||||
[weil ich dann etwas habe, worüber ich mich mit meinen Freundinnen und Freunden unterhalten kann.] | 2.04 | 1.65 | 0.567 | ||
Because I can be together with my family. | |||||
[weil ich dabei mit meiner Familie zusammen sein kann.] | 1.38 | 1.53 | 0.565 | ||
Because it makes me belong. | |||||
[weil ich somit “dazu gehöre.”] | .88 | 1.32 | 0.560 | ||
Because my best friend and I can enthuse about it together. | |||||
[weil meine beste Freundin/mein bester Freund und ich dann gemeinsam für etwas schwärmen können.] | 1.62 | 1.69 | 0.543 | ||
Because it can express my political attitudes. | |||||
[weil sie meine politischen Überzeugungen ausdrücken kann.] | 1.48 | 1.77 | 0.531 | ||
Because my friends like the same music as I do. | |||||
[weil sie auch meinen Freundinnen und Freunden gefällt.] | 1.76 | 1.65 | 0.523 | ||
Because when listening, I can imagine how the music would sound in a concert. | |||||
[weil ich mir dabei vorstellen kann, wie die Musik wohl im Konzert wäre.] | 2.54 | 1.97 | 0.496 | ||
Because it is related to spirituality. | |||||
[weil sie für mich eng mit Spiritualität verbunden ist.] | 1.17 | 1.71 | 0.483 | ||
Because I learn a lot about the world. | |||||
[weil ich dadurch viel von der Welt erfahre.] | 2.30 | 1.64 | 0.472 | ||
Because I can identify with the musicians or bands. | |||||
[weil ich mich dadurch mit einigen MusikerInnen oder Gruppen so gut identifizieren kann.] | 2.58 | 1.79 | 0.465 | ||
Because it supports my religious faith. | |||||
[weil sie meinen Glauben unterstützt.] | 1.22 | 1.83 | 0.448 | ||
Because it has a supernatural meaning to me. | |||||
[weil sie für mich eine übersinnliche Bedeutung hat.] | 1.18 | 1.75 | 0.446 | ||
Because I want to know what's going on in the music scene. | |||||
[weil ich darüber Bescheid wissen will, was in der Musikszene gerade aktuell ist.] | 1.97 | 1.82 | 0.429 | ||
Because I want to find out something about the music. | |||||
[weil ich etwas über die Musik herausfinden möchte.] | 2.60 | 1.81 | 0.428 | ||
Because it makes me let go of myself when I'm in company. | |||||
[weil sie mir hilft, aus mir herauszugehen, wenn ich in Gesellschaft bin.] | 2.81 | 1.84 | 0.422 | ||
Because it contributes to my health. | |||||
[weil sie zu meiner Gesundheit beiträgt.] | 2.51 | 1.88 | 0.415 | ||
Because it can soothe my physical pain. | |||||
[weil sie meine körperlichen Beschwerden lindern kann.] | 1.95 | 1.82 | 0.412 | ||
Because you can learn something from the music. | |||||
[weil man etwas dabei lernen kann.] | 2.75 | 1.76 | 0.404 | ||
Because I want to be informed about hits and trends. | |||||
[weil ich mich über Hits und Trends informieren will.] | 1.86 | 1.71 | 0.402 | ||
Because it structures my everyday life. | |||||
[weil sie meinem Alltag Struktur gibt.] | 2.24 | 1.85 | 0.397 | ||
Because I can get away from my family. | |||||
[weil ich damit meiner Familie entkommen kann.] | 1.31 | 1.73 | 0.378 | ||
Because it is a means to share my memories with my friends. | |||||
[weil sie eine Möglichkeit bietet, Erinnerungen mit Freunden zu teilen.] | 3.40 | 1.81 | 0.375 | ||
Because it makes me feel sexy. | |||||
[weil ich mich dann sexy fühle.] | 1.68 | 1.87 | 0.372 | ||
Because I can learn about new pieces. | |||||
[weil ich dabei neue Stücke kennenlernen kann.] | 3.50 | 1.86 | 0.369 | ||
Because I'm interested in the musicians and bands. | |||||
[weil ich die MusikerInnen und Gruppen interessant finde.] | 3.86 | 1.66 | 0.312 | ||
Because it is a great pastime. | 3.97 | 1.68 | 0.640 | ||
[weil sie ein prima Zeitvertreib ist.] | |||||
Because it can take my mind off things. | |||||
[weil sie mich ablenken kann.] | 4.52 | 1.45 | 0.627 | ||
Because it prevents me from being bored while I do other things. | |||||
[weil ich dann weniger gelangweilt bin, während ich etwas anderes tue.] | 3.39 | 1.94 | 0.621 | ||
Because it makes time pass markedly faster. | |||||
[weil dann die Zeit deutlich schneller vergeht.] | 3.57 | 1.85 | 0.609 | ||
Because it enables me to kill time. | |||||
[weil ich damit die Zeit totschlagen kann.] | 2.64 | 1.99 | 0.598 | ||
Because I'm less bored then. | |||||
[weil es dann nicht so langweilig ist.] | 3.99 | 1.75 | 0.584 | ||
Because I need it in the background while I do other things. | |||||
[weil ich sie im Hintergrund brauche, während ich etwas anderes tue.] | 3.76 | 1.79 | 0.564 | ||
Because it makes me cheerful. | |||||
[weil ich dann gute Laune bekomme.] | 4.76 | 1.28 | 0.555 | ||
Because it can enhance my mood. | |||||
[weil sie meine Stimmung verbessern kann.] | 5.04 | 1.15 | 0.539 | ||
Because it fills the unpleasant silence when no one speaks. | |||||
[weil Musik die unangenehme Stille füllt, wenn gerade niemand spricht.] | 3.15 | 2.00 | 0.537 | ||
Because it helps me get up in the morning. | |||||
[weil sie mir morgens hilft, wach zu werden.] | 3.75 | 1.94 | 0.532 | ||
Because it helps me relax. | |||||
[weil ich mich dann besser entspannen kann.] | 4.84 | 1.18 | 0.520 | ||
Because it provides diversion. | |||||
[weil sie für mich eine gute Abwechslung bietet.] | 4.24 | 1.44 | 0.511 | ||
Because it puts me in the right mood for going out. | |||||
[weil ich mich damit einstimmen kann, bevor ich ausgehe.] | 3.69 | 2.05 | 0.508 | ||
Because it enhances my drive or my motivation for certain actions. | |||||
[weil sie meinen Antrieb bzw. meine Motivation für bestimmte Tätigkeiten steigert.] | 4.41 | 1.55 | 0.505 | ||
Because it is a good way to entertain myself. | |||||
[weil das eine gute Art ist, mich selbst zu unterhalten.] | 4.15 | 1.56 | 0.492 | ||
Because I take delight in doing so. | |||||
[weil ich dabei Spaß habe.] | 5.10 | 1.15 | 0.491 | ||
Because it makes me more alert. | |||||
[weil ich dann wacher bin.] | 3.32 | 1.72 | 0.477 | ||
Because it makes doing things seem effortless. | |||||
[weil mir dann vieles lockerer von der Hand geht.] | 4.40 | 1.40 | 0.464 | ||
Because it stimulates me. | |||||
[weil sie mich animiert.] | 3.96 | 1.63 | 0.441 | ||
Because I can dance to it. | |||||
[weil ich dazu tanzen kann.] | 3.53 | 2.04 | 0.436 | ||
Because it makes me feel fitter. | |||||
[weil ich mich dann fitter fühle.] | 3.37 | 1.81 | 0.432 | ||
Because it enables me to work off my aggression. | |||||
[weil ich dabei meine Aggressionen abreagieren kann.] | 3.48 | 2.02 | 0.427 | ||
Because it takes my mind off things. | |||||
[weil sie mich auf andere Gedanken bringt.] | 4.82 | 1.27 | 0.421 | ||
Because it provides a pleasant ambience for conversations. | |||||
[weil sie eine angenehme Atmosphäre beim Gespräch schafft.] | 3.17 | 1.72 | 0.416 | ||
Because music just fits into my life. | |||||
[weil Musik einfach gut in mein Leben passt.] | 4.90 | 1.37 | 0.403 | ||
Because it fits my sports. | |||||
[weil es zu meinem Sport passt.] | 2.62 | 2.16 | 0.400 | ||
Because working is easier with music. | |||||
[weil ich dann besser arbeiten kann.] | 3.43 | 1.83 | 0.389 | ||
Because it helps me fall asleep. | |||||
[weil sie mir beim Einschlafen hilft.] | 2.68 | 2.03 | 0.357 | ||
Because I can cuddle with my partner. | |||||
[weil ich mit meinem Partner bzw. meiner Partnerin dabei gut kuscheln kann.] | 2.50 | 1.85 | 0.354 | ||
Because I can sing or hum along. | |||||
[weil ich dabei mitsingen oder mitsummen kann.] | 3.91 | 1.80 | 0.346 | ||
Because I can try out new movements. | |||||
[weil ich dann neue Bewegungen ausprobieren kann.] | 1.83 | 1.83 | 0.337 |
Dimension 1, self-awareness; Dimension 2, social relatedness; Dimension 3, arousal and mood regulation .
- Arnett J. J. (1995). Adolescents' uses of media for self-socialisation . J. Youth Adolesc . 24 , 519–533 [ Google Scholar ]
- Baacke D. (1984). Kommunikations-kultur der Jugend , in Medienpädagogik and Kommunikationskultur. Referate und Texte Nach Dem Ersten “Forum Kommunikationskultur,” ed de Haen I. (Frankfurt am Main: GEP; ), 37–53 [ Google Scholar ]
- Bartlett D. L. (1996). Physiological responses to music and sound stimuli , in Handbook of Music Psychology, 2nd Edn , ed Hodges D. A. (St. Louis, MO: MMB Music; ), 343–385 [ Google Scholar ]
- Bicknell J. (2007). Explaining strong emotional responses to music: sociality and intimacy . J. Conscious. Stud . 14 , 5–23 [ Google Scholar ]
- Blood A. J., Zatorre R. J. (2001). Intensely pleasurable responses to music correlate with activity in brain regions implicated in reward and emotion . Proc. Natl. Acad. Sci. U.S.A 98 , 11818–11823 10.1073/pnas.191355898 [ PMC free article ] [ PubMed ] [ CrossRef ] [ Google Scholar ]
- Boehnke K., Münch T. (2003). Jugendsozialisation und Medien. Helfen Medien und Musik beim Erwachsenwerden? in Neue Medien im Alltag. Nutzung, Vernetzung, Interaktion , eds Keitel E., Boehnke K., Wenz K. (Lengerich: Pabst Science Publishers; ), 203–227 [ Google Scholar ]
- Boer D. (2009). Music Makes the People Come Together: Social Functions of Music Listening for Young People Across Cultures . Department of Psychology. Victoria University of Wellington, Wellington. Available online at: http://researcharchive.vuw.ac.nz/bitstream/handle/10063/1155/thesis.pdf?sequence=1
- Brown J. D., Campbell K., Fischer L. (1986). American adolescents and music videos: why do they watch . Int. Commun. Gaz . 37 , 19–32 10.1177/001654928603700104 [ CrossRef ] [ Google Scholar ]
- Brown S. (2006). How does music work? Toward a pragmatics of musical communication , in Music and Manipulation: On the Social Uses and Social Control of Music , eds Brown S., Volgsten U. (New York, NY: Berghahn Books; ), 1–30 [ Google Scholar ]
- Bryson B. (1996). “Anything but heavy metal”: symbolic exclusion and musical dislikes . Am. Soc. Rev . 61 , 884–899 10.2307/2096459 [ CrossRef ] [ Google Scholar ]
- Bullough E. (1921). Recent work in experimental aesthetics . Br. J. Psychol . 12 , 76–99 [ Google Scholar ]
- Campbell C., Connell S., Beegle A. P. (2007). Adolescents' expressed meanings of music in and out of school . J. Res. Music Educ . 55 , 220–236 [ Google Scholar ]
- Chamorro-Premuzic T., Furnham A. (2007). Personality and music: can traits explain how people use music in everyday life . Br. J. Psychol . 98 , 175–185 10.1348/000712606X111177 [ PubMed ] [ CrossRef ] [ Google Scholar ]
- Coleman J. S. (1961). Psychological effects of the social system , in The Adolescents Society: The Social Life of the Teenager and its Impact on Education , ed Coleman J. S. (Oxford: Free Press of Glencoe; ), 220–243 [ Google Scholar ]
- Cook J. D. (1986). Music as an intervention in the oncology setting . Cancer Nurs . 9 , 23–28 [ PubMed ] [ Google Scholar ]
- Darwin C. (1871). The Descent of Man, and Selection in Relation to Sex . London: John Murray [ Google Scholar ]
- Darwin C. (1872). The Expression of the Emotions in Man and Animals . London: John Murray [ Google Scholar ]
- DeNora T. (1999). Music as a technology of the self . Poetics 27 , 31–56 10.1016/S0304-422X(99)00017-0 [ CrossRef ] [ Google Scholar ]
- Dissanayake E. (2006). Ritual and ritualization: musical means of conveying and shaping emotion in humans and other animals , in Music and Manipulation: On the Social Uses and Social Control of Music , eds Brown S., Volgsten U. (New York, NY: Berghahn Books; ), 31–56 [ Google Scholar ]
- Dissanayake E. (2009). Root, leaf, blossom, or bole: concerning the origin and adaptive function of music , in Communicative Musicality: Exploring the Basis of Human Companionship , eds Malloch S., Trevarthen C. (New York, NY: Oxford University Press; ), 17–30 [ Google Scholar ]
- Engh M. (2006). Popstars als Marke: Identitätsorientiertes Marken-management für die Musikindustrielle Künstlerentwicklung und –Vermarktung . Wiesbaden: Deutscher Universitäts-Verlag [ Google Scholar ]
- Fachner J. (2008). Musik und veränderte Bewusstseinszustände [Music and altered states of consciousness] , in Musikpsychologie. Das neue Handbuch , eds Bruhn H., Kopiez R., Lehmann A. C. (Reinbek: Rowohlt; ), 594–612 [ Google Scholar ]
- Falk D. (2004a). Prelinguistic evolution in early hominins: whence motherese . Behav. Brain Sci . 27 , 491–503 10.1017/S0140525X04000111 [ PubMed ] [ CrossRef ] [ Google Scholar ]
- Falk D. (2004b). The “putting the baby down” hypothesis: bipedalism, babbling, and baby slings . Behav. Brain Sci . 27 , 526–534 10.1017/S0140525X0448011X [ CrossRef ] [ Google Scholar ]
- Fine C. (2006). A Mind of Its Own: How Your Brain Distorts and Deceives . New York, NY: W.W. Norton [ Google Scholar ]
- Fitch W. T. (2006). The biology and evolution of music: a comparative perspective . Cognition 100 , 173–215 10.1016/j.cognition.2005.11.009 [ PubMed ] [ CrossRef ] [ Google Scholar ]
- Frith S. (1996). Performing Rites. On the Value of Popular Music . Oxford: Oxford University Press [ Google Scholar ]
- Frohne-Hagemann I., Pleß-Adamczyk H. (2005). Indikation Musiktherapie bei psychischen Problemen im Kindes- und Jugendalter. Musiktherapeutische Diagnostik und Manual nach ICD-10 . Göttingen: Vandenhoeck and Ruprecht [ Google Scholar ]
- Gantz W., Gartenberg H. M., Pearson M. L., Schiller S. O. (1978). Gratifications and expectations associated with Pop music among adolescents . Pop. Music Soc . 6 , 81–89 10.1080/03007767808591113 [ CrossRef ] [ Google Scholar ]
- Greasley A. E., Lamont A. (2011). Exploring engagement with music in everyday life using experience sampling methodology . Music. Sci . 15 , 45–71 10.1177/1029864910393417 [ CrossRef ] [ Google Scholar ]
- Gregory A. H. (1997). The roles of music in society: the ethnomusicological perspective , in The Social Psychology of Music , eds Hargreaves D. J., North A. C. (New York, NY: Oxford University Press; ), 123–140 [ Google Scholar ]
- Hargreaves D. J., North A. C. (1999). The functions of music in everyday life: redefining the social in music psychology . Psychol. Music 27 , 71–83 10.1177/0305735699271007 [ CrossRef ] [ Google Scholar ]
- Hays T., Minichiello V. (2005). The meaning of music in the lives of older people: a qualitative study . Psychol. Music 33 , 437–451 10.1177/0305735605056160 [ CrossRef ] [ Google Scholar ]
- Heister H.-W. (1993). Stellenwert der Musik im gesellschaftlichen System , in Musikpsychologie. Ein Handbuch , eds Bruhn H, Oerter R., Rösing H. (Reinbek: Rowohlt; ), 103–112 [ Google Scholar ]
- Herbert R. (2011). Everyday Music Listening: Absorption, Dissociation and Trancing . Farnham, Burlington: Ashgate Publishing Limited [ Google Scholar ]
- Heye A., Lamont A. (2010). Mobile listening situations in everyday life: the use of MP3 players while travelling . Music. Sci . 14 , 95–120 [ Google Scholar ]
- Hirstein W. (2005). Brain Fiction: Self-Deception and the Riddle of Confabulation . Cambridge, MA: MIT Press [ Google Scholar ]
- Hunter P. G., Schellenberg E. G. (2010). Music and emotion , in Music Perception , Vol. 36 , eds Jones M. R., Fay R. R., Popper A. N. (New York, NY: Springer; ), 129–164 [ Google Scholar ]
- Huron D. (2001). Is music an evolutionary adaptation? , in The Biological Foundations of Music , eds Zatorre R. J., Peretz I. (New York, NY: New York Academy of Sciences; ), 43–61 [ PubMed ] [ Google Scholar ]
- Juslin P. N., Liljeström S., Västfjäll D., Barradas G., Silva A. (2008). An experience sampling study of emotional reactions to music: listener, music, and situation . Emotion 8 , 668–683 10.1037/a0013505 [ PubMed ] [ CrossRef ] [ Google Scholar ]
- Kapteina H. (2010). Was Geschieht, Wenn Wir Musik Hören. Fragmente Zur Psychologie Des Hörens . Available online at: http://www.musiktherapie.uni-siegen.de/kapteina/material/forschungsgebiete/neu_was_geschieht_wenn_wir_musik_hoeren.pdf
- Laiho S. (2004). The psychological functions of music in adolescence . Nord. J. Music Ther . 13 , 47–63 10.1080/08098130409478097 [ CrossRef ] [ Google Scholar ]
- Larson R. (1995). Secrets in the bedroom: adolescents' private use of media . J. Youth Adolesc . 24 , 535–550 10.1007/BF01537055 [ CrossRef ] [ Google Scholar ]
- Laukka P. (2007). Uses of music and psychological well-being among the elderly . J. Happiness Stud . 8 , 215–241 10.1007/s10902-006-9024-3 [ CrossRef ] [ Google Scholar ]
- Lehmann A. C. (1994). Habituelle Und Situative Rezeptionsweisen Beim Musikhören. Eine Einstellungstheoretische Untersuchung . Frankfurt: Peter Lang [ Google Scholar ]
- Levitin D. J. (2007). Life Soundtrack: The Uses of Music in Everyday Life . Montreal, QC: McGill University; Available online at: http://levitin.mcgill.ca/pdf/LifeSoundtracks.pdf [ Google Scholar ]
- Lonsdale A. J., North A. C. (2011). Why do we listen to music. a uses and gratifications analysis . Br. J. Psychol . 102 , 108–134 10.1348/000712610X506831 [ PubMed ] [ CrossRef ] [ Google Scholar ]
- Maas G. (1993). Filmmusik , in Musikpsychologie. Ein Handbuch , eds Bruhn H., Oerter R., Rösing H. (Reinbek: Rowohlt; ), 203–207 [ Google Scholar ]
- Maslow A. H. (1968). Toward a Psychology of Being . 2nd Edn New York, NY: Van Nostr and Company [ Google Scholar ]
- McQuail D., Blumler J. G., Brown J. (1972). The television audience: a revised perspective , in Sociology of Mass Communication , ed McQuail D. (Middlesex: Penguin; ), 135–165 [ Google Scholar ]
- Melton G. W., Galician M. Lou. (1987). A sociological approach to the pop music phenomenon: radio and music video utilization for expectation, motivation and satisfaction . Pop. Music Soc . 11 , 35–46 10.1080/03007768708591286 [ CrossRef ] [ Google Scholar ]
- Merriam A. P. (1964). The Anthropology of Music . Evanston, IL: Northwestern University Press [ Google Scholar ]
- Miller G. (2000). Evolution of human music through sexual selection , in The Origins of Music , eds Wallin N. L., Merker B., Brown S. (Cambridge: The MIT Press; ), 329–360 [ Google Scholar ]
- Misenhelter D., Kaiser K. (2008). Social functions of music in music education . J. Artistic Creat. Educ . 2 , 61–74 [ Google Scholar ]
- Mithen S. (2006). The Singing Neanderthals: The Origins of Music, Language, Mind, and Body . Cambridge: Harvard University Press [ Google Scholar ]
- Münch T., Bommersheim U., Müller-Bachmann E. (2005). Jugendliches Musikverhalten. Musikinvolvement, Nutzungsmotive und Musikpräferenzen , in Jugendsozialisation und Medien , eds Boehnke K., Münch T. (Lengerich: Pabst Science Publishers; ), 167–199 [ Google Scholar ]
- Nakamura J., Csikszentmihalyi M. (2009). Flow theory and research , in Oxford Handbook of Positive Psychology, 2nd Edn , eds Lopez S. J., Snyder C. R. (New York, NY: Oxford University Press; ), 195–206 [ Google Scholar ]
- Newberg A., D'Aquili E., Rause V. (2001). Why God Won't Go Away: Brain Science and the Biology of Belief . New York, NY: Ballantine Books [ Google Scholar ]
- North A. C., Hargreaves D. J., O'Neill S. A. (2000). The importance of music to adolescents . Br. J. Educ. Psychol . 70 , 255–272 [ PubMed ] [ Google Scholar ]
- Packer J., Ballantyne J. (2011). The impact of music festival attendance on young people's psychological and social well-being . Psychol. Music 39 , 164–181 10.1177/0305735610372611 [ CrossRef ] [ Google Scholar ]
- Panksepp J. (1995). The emotional sources of “chills” induced by music . Music Percept . 13 , 171–207 [ Google Scholar ]
- Panksepp J., Bernatzky G. (2002). Emotional sounds and the brain: the neuro-affective foundations of musical appreciation . Behav. Process . 60 , 133–155 10.1016/S0376-635700080-3 [ PubMed ] [ CrossRef ] [ Google Scholar ]
- Peretz I. (2006). The nature of music from a biological perspective . Cognition 100 , 1–32 10.1016/j.cognition.2005.11.004 [ PubMed ] [ CrossRef ] [ Google Scholar ]
- Rentfrow P. J., Goldberg L. R., Levitin D. J. (2011). The structure of musical preferences: a five-factor model . J. Pers. Soc. Psychol . 100 , 1139–1157 [ PMC free article ] [ PubMed ] [ Google Scholar ]
- Roberts D. F., Christenson P. G. (2001). Popular music in childhood and adolescence , in Handbook of Children and the Media , eds Singer D. G., Singer J. L. (Thousand Oaks, CA: Sage Publications, Inc; ), 395–414 [ Google Scholar ]
- Roberts D. F., Foehr U. G. (2008). Trends in media use . Future Child . 18 , 11–37 [ PubMed ] [ Google Scholar ]
- Roe K. (1985). Swedish youth and music: listening patterns and motivations . Commun. Res . 12 , 353–362 10.1177/009365085012003007 [ CrossRef ] [ Google Scholar ]
- Rösing H. (1993). Musik im Alltag , in Musikpsychologie. Ein Handbuch , eds Bruhn H., Oerter R., Rösing H. (Reinbek: Rowohlt; ), 113–130 [ Google Scholar ]
- Ruud E. (1997). Music and the quality of life . Nord. J. Music Ther . 6 , 86–97 10.1080/08098139709477902 [ CrossRef ] [ Google Scholar ]
- Schäfer T., Sedlmeier P. (2009). From the functions of music to music preference . Psychol. Music 37 , 279–300 10.1177/0305735608097247 [ CrossRef ] [ Google Scholar ]
- Schäfer T., Sedlmeier P. (2010). What makes us like music. Determinants of music preference . Psychol. Aesthe. Creativity Arts 4 , 223–234 10.1037/a0018374 [ CrossRef ] [ Google Scholar ]
- Schubert E. (2009). The fundamental function of music . Music. Sci . 13 , 63–81 10.1177/1029864909013002051 [ CrossRef ] [ Google Scholar ]
- Steele J. R., Brown J. D. (1995). Adolescent room culture: studying the media in the context of everyday life . J. Youth Adolesc . 24 , 551–576 10.1007/BF01537056 [ CrossRef ] [ Google Scholar ]
- Stefanija L. (2007). Functions of music: a survey of research vocabularies . Muzikos funkcijos: tyrimø terminologijos apžvalga. (Lithuanian) 7 , 6–17 [ Google Scholar ]
- Sun S., Lull J. (1986). The adolescent audience for music videos and why they watch . J. Commun . 36 , 115–125 10.1111/j.1460-2466.1986.tb03043.x [ CrossRef ] [ Google Scholar ]
- Tarrant M., North A. C., Hargreaves D. J. (2000). English and American adolescents' reasons for listening to music . Psychol. Music 28 , 166–173 10.1177/0305735600282005 [ CrossRef ] [ Google Scholar ]
- Ter Bogt T. F. M., Mulder J., Raaijmakers Q. A. W., Gabhainn S. N. (2011). Moved by music: a typology of music listeners . Psychol.Music 39 , 147–163 10.1177/0305735610370223 [ CrossRef ] [ Google Scholar ]
- Troldahl V. C., Skolnik R. (1967). The meanings people have for radio today . J. Broadcast . 12 , 57–67 10.1080/08838156709386226 [ CrossRef ] [ Google Scholar ]
- Walker Kennedy S. (2010). An Exploration of Differences in Response to Music Related to Levels of Psychological Health in Adolescents . Toronto, ON: University of Toronto [ Google Scholar ]
- Wells A., Hakanen E. A. (1997). The emotional use of popular music by adolescents , in Mass Media and Society , eds Wells A., Hakanen E. A. (Greenwich: Ablex Publishing Corporation; ), 217–228 [ Google Scholar ]
- Wilson T. D. (2002). Strangers to Ourselves: Discovering the Adaptive Unconscious . Cambridge, MA: Harvard University Press [ Google Scholar ]
- Zatorre R. J., Peretz I. (2001). The Biological Foundations of Music . New York, NY: New York Academy of Sciences [ Google Scholar ]
- Zillmann D. (1988a). Mood management through communication choices . Am. Behav. Sci . 31 , 327–341 [ Google Scholar ]
- Zillmann D. (1988b). Mood management: using entertainment to full advantage , in Communication, Social Cognition, and Affect , eds Donohew L., Sypher H. E., Higgins E. T. (Hillsdale, NJ: Lawrence Erlbaum Associates; ), 147–171 [ Google Scholar ]
Mechanistic Study on the Fine-Scale Arch Effect of Moisture Content on the Dense Moulding of Mushroom Pomace Particles
- Original Paper
- Published: 23 September 2024
Cite this article
- Zhen Li 1 ,
- Hengyang Sun ORCID: orcid.org/0009-0003-3648-9338 1 ,
- Zhao Lei 1 ,
- Sheng Xu 2 ,
- Zhizhong Qiao 1 &
In the dense molding process of shiitake mushroom dregs waste, the existence of the arch effect between particles not only restricts the quality of molded particles, but also increases the energy consumption of production, so it is necessary to explore the reasonable arch effect in the molding process. In this paper, the water content of mushroom pomace as an important factor in the molding process was used as a variable to study the effect of water content of mushroom pomace particles on the internal arching effect of the particle body in the dense molding process at a fine scale. Four water content conditions of 8%, 10%, 12% and 14% were selected in the optimal molding water content interval. The research methodology included closed-end compression experiments and EDEM software simulation experiments. The internal force of bulk molded particles was analyzed by uniaxial compression replica paper indentation method and simulation cross-section method to reflect the strength of the arch effect. The results show that the experimental and simulation results are highly consistent with each other, resulting in the law of the influence of dense molding on the arch effect of mushroom slag particles at different moisture contents (10%>12%>14%>8%), and the law derived from this study is of certain guiding significance for the production of mushroom slag biomass combustion particles.
This is a preview of subscription content, log in via an institution to check access.
Access this article
Subscribe and save.
- Get 10 units per month
- Download Article/Chapter or eBook
- 1 Unit = 1 Article or 1 Chapter
- Cancel anytime
Price includes VAT (Russian Federation)
Instant access to the full article PDF.
Rent this article via DeepDyve
Institutional subscriptions
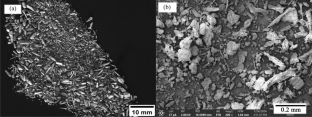
Staples, M.D., Malina, R., Barrett, S.R.: The limits of bioenergy for mitigating global life-cycle greenhouse gas emissions from fossil fuels. Nat. Energy. 2 (2), 1–8 (2017). https://doi.org/10.1038/nenergy.2016.202
Article Google Scholar
Chen, J.M.: Carbon neutrality: Toward a sustainable future. Innov. 2 (2021). https://doi.org/10.1016/j.xinn.2021.100127
Picchio, R., Latterini, F., Venanzi, R., Stefanoni, W., Suardi, A., Tocci, D., Pari, L.: Pellet production from woody and non-woody feedstocks: A review on biomass quality evaluation. Energies. 13 (11) (2020). https://doi.org/10.3390/en13112937
Yang, G., Hou, H., Lin, Z.: Effects of waste liquid on the formation and properties of wheat slag pellet fuel. Trans. Chin. Soc. Agric. Eng. 38 , 197–203 (2022). https://doi.org/10.11975/j.issn.1002-6819.2022.03.023
Fufa, B.K., Tadesse, B.A., Tulu, M.M.: Cultivation of pleurotus ostreatus on agricultural wastes and their combination. Int. J. Agron. 20211 , 1465597 (2021). https://doi.org/10.1155/2021/1465597
Li, F.N., Zhang, J.B., Shen, X.: Analysis of the changing dynamics of the edible mushroom industry layout in China. Edible Med. Mushrooms. 25 (01), 1–5 (2017)
Google Scholar
Gao, Q., Zhu, Q.F., Zhou, J.Y., Li, Y.: Current status of research on utilisation of waste mushroom residue. Anhui Agricultural Sci. Bull. (2015). https://doi.org/10.16377/j.cnki.issn1007-7731.2015.19.032 ,21(19):81 + 89.
Tian, D., Zhang, M., Zhao, A., Wang, B., Shi, J., Feng, J.: Agent-based modeling and simulation of edible fungi growers’ adoption behavior towards fungal chaff recycling technology. Agric. Syst. 190 , 103138 (2021). https://doi.org/10.1016/j.agsy.2021.103138
Xia, X., Zhang, K., Xiao, H., Xiao, S., Song, Z., Yang, Z.: Effects of additives and hydrothermal pretreatment on the pelleting process of rice straw: Energy consumption and pellets quality. Ind. Crops Prod. 133 , 178–184 (2019). https://doi.org/10.1016/j.indcrop.2019.03.007
Yu, Y., Sokhansanj, S., Lau, A., El-Kassaby, Y.A., Wang, G., Guo, Y.: Hydrothermal carbonization of mixture waste Gingko leaf and wheat straw for solid biofuel production. Ind. Crops Prod. 206 , 117633 (2023). https://doi.org/10.1016/j.indcrop.2023.117633
Wang, G., Jiang, Y., Li, W., Yin, X.: Process optimization of corn stover compression molding experiments based on response surface method. Trans. Chin. Soc. Agricultural Eng. 32 (13), 223–227 (2016). https://doi.org/10.11975/j.issn.1002-6819.2016.13.032
Wittmer, J.P., Claudin, P., Cates, M.E., Bouchaud, J.P.: An explanation for the central stress minimum in sand piles. Nature. 382 (6589), 336–338 (1996). https://doi.org/10.1038/382336a0
Zhang, D., Cui, H., Lei, Z., Zhang, X., Wang, Z., Bai, Y., Zhao, H.: Soil arching effect of composite piles supporting foundation pits based on mechanical model and photoelastic experiment. Opt. Lasers Eng. 168 , 107644 (2023). https://doi.org/10.1016/j.optlaseng.2023.107644
Sha, Q.Y.: Influence of arch effect on the salix biomass forming process (Master’s thesis).Inner Mongolia University of Science and Technology. (2022). https://doi.org/10.27724/d.cnki.gnmgk.2022.000590
Zhao, Z.G., Wang, B.L., Han, G.X., Tang, B., Liang, J.: Soil arching of trapdoor tests based on non-circular particles. J. East. China Jiaotong Univ. 32 (04), 78–84 (2015). https://doi.org/10.16749/j.cnki.jecjtu.2015.04.011
Cui, P.B., Zhu, Y.Q., Liu, Y., Zhu, Z.G., Pan, Y.D.: Model test and particle flow numerical simulation of soil arching effect for unsaturated sandy soil tunnel. Rock. Soil. Mech. 42 (12), 7 (2022). https://doi.org/10.16285/j.rsm.2021.0557
Wang, P., Zhu, X., Liu, M., Li, Y.: Buckling behaviors and simplified design method for steel silos under locally distributed axial load. J. Constr. Steel Res. 134 , 114–134 (2017). https://doi.org/10.1016/j.jcsr.2017.03.019
Zhang, Z.L., Wang, T.: Numerical analysis of loess and weak intercalated layer failure behavior under direct shearing and cyclic loading. J. Mt. Sci. 17 (11), 2796–2815 (2020). https://doi.org/10.1007/s11629-020-6178-0
Zhao, Y.H., Yu, J., Zhou, C.H., Zhao, K., Xiao, H.G.: Characterization of pressure arching effect of arch shell surrounding rock considering deviation of principal stress axis. Chin. J. Geotech. Eng. 43 (10), 1842–1850 (2021). https://doi.org/10.11779/CJGE202110010
Yi, C.H., Mu, Q.S., Miao, T.D.: Discrete element method simulation on the force chains in the two-dimensional granular system under gravity. Acta Phys. Sinica. 58 (11), 7750–7755 (2009)
De, X.H.: Research on mechanism of wear about plunger biomass ring die forming mould based on fractal theory (Doctoral dissertation). Beijing Forestry University. (2014)
Mueth, D.M., Jaeger, H.M., Nagel, S.R.: Force distribution in a granular medium. Phys. Rev. E. 57 (3) (1998). https://doi.org/10.1103/PhysRevE.57.3164
Hou, M.X.: The test on the stress grid of granular material and characteristic research on arching effect (Doctoral dissertation). South China University of Technology. (2016)
Cil, M.B., Alshibli, K.A.: 3D assessment of fracture of sand particles using discrete element method. Géotechnique Lett. 2 (3), 161–166 (2012). https://doi.org/10.1680/geolett.12.00024
Yu, J.: Research on the influence of moisture content on the densification of sand shrubs particles (Master’s thesis). Inner Mongolia University of Science and Technology. (2021). https://doi.org/10.27724/d.cnki.gnmgk.2021.000012
Li, Z., Yu, J., Yue, Q., Yu, Y., Guo, X.: Study on meso-mechanical mechanism and energy of moisture content on densification of salix psammophila particles. Renew. Energy. 205 , 1071–1081 (2023). https://doi.org/10.1016/j.renene.2023.01.042
Yan, L.: Reserch on the macro-mechanical models in dense forming of salix psammophila granules (Master’s thesis).Inner Mongolia University of Science and Technology. (2020). https://doi.org/10.27724/d.cnki.gnmgk.2020.000136
Wang, Y., Liang, Z., Zhang, D., Cui, T., Shi, S., Li, K., Yang, L.: Calibration method of contact characteristic parameters for corn seeds based on EDEM. Trans. Chin. Soc. Agricultural Eng. 32 (22), 36–42 (2016). https://doi.org/10.11975/j.issn.1002-6819.2016.22.005
Yan, W.G., Fu, J.R., Li, Z., Yan, L.: Research on macro and meso simulation in compression process of salix psammophila granules. Acta Energiae Solar Sinica. 09 , 449–454 (2023). https://doi.org/10.19912/j.0254-0096.tynxb.2022-0790
Cao, D.L.: Multi-objective structure design and optimization of Y-type screen filter based on CFD-DEM coupling (Master’s thesis). Kunming University of Science and Technology. (2022). https://doi.org/10.27200/d.cnki.gkmlu.2022.000376
Li, H.C.: Theoretical and experimental study on air-and-screen cleaning unit (Doctoral dissertation).Jiangsu University. (2011)
Han, D.A., Zhang, D.X., Yang, L., Cui, T., Ding, Y.Q., Bian, X.H.: Nongye Jixie Xuebao/Transactions Chin. Soc. Agricultural Mach. 48 (11) (2017). https://doi.org/10.6041/j.issn.1000-1298.2017.11.006 Optimization and experiment of inside-filling air-blowing seed metering device based on EDEM-CFD
Yue, Q.: Research on energy consumption per unit mass of mixed molding of mushroom residue and salix psammophila (Master’s thesis).Inner Mongolia University of Science and Technology. (2023). https://doi.org/10.27724/d.cnki.gnmgk.2023.000949
Download references
Author information
Authors and affiliations.
School of Mechanical Engineering, Inner Mongolia University of Science and Technology, Baotou, 014010, China
Zhen Li, Hengyang Sun, Zhao Lei, Zhizhong Qiao & Bin Li
School of Civil Engineering, Inner Mongolia University of Science and Technology, Baotou, 014010, China
You can also search for this author in PubMed Google Scholar
Corresponding author
Correspondence to Hengyang Sun .
Additional information
Publisher’s note.
Springer Nature remains neutral with regard to jurisdictional claims in published maps and institutional affiliations.
Rights and permissions
Springer Nature or its licensor (e.g. a society or other partner) holds exclusive rights to this article under a publishing agreement with the author(s) or other rightsholder(s); author self-archiving of the accepted manuscript version of this article is solely governed by the terms of such publishing agreement and applicable law.
Reprints and permissions
About this article
Li, Z., Sun, H., Lei, Z. et al. Mechanistic Study on the Fine-Scale Arch Effect of Moisture Content on the Dense Moulding of Mushroom Pomace Particles. Waste Biomass Valor (2024). https://doi.org/10.1007/s12649-024-02730-x
Download citation
Received : 01 June 2024
Accepted : 03 September 2024
Published : 23 September 2024
DOI : https://doi.org/10.1007/s12649-024-02730-x
Share this article
Anyone you share the following link with will be able to read this content:
Sorry, a shareable link is not currently available for this article.
Provided by the Springer Nature SharedIt content-sharing initiative
- Mesosocopic scale
- Mushroom residue
- Dense molding
- Moisture content
- Arch effect
- Find a journal
- Publish with us
- Track your research

IMAGES
VIDEO
COMMENTS
The most cited paper comes from Sony CSL Paris, while two other heavily cited papers come from research groups at Google. Two more are from Academia Sinica in Taiwan. Table 2. ... music production, composing for media or as aids to musical composition. The evaluation took into account the current state of each system, the music it produced, its ...
The Journal of New Music Research (JNMR) publishes material which increases our understanding of music and musical processes by systematic, scientific and technological means. Research published in the journal is innovative, empirically grounded and often, but not exclusively, uses quantitative methods. Articles are both musically relevant and scientifically rigorous, giving full technical ...
This research focuses on describing technology use in music education at a university in North Cyprus, according to 18 student music teachers, to highlight the extent to which technology has been ...
Explore the latest full-text research PDFs, articles, conference papers, preprints and more on MUSIC PRODUCTION. Find methods information, sources, references or conduct a literature review on ...
3.2. Popularity and significance. The popularity of a given album is strongly linked to its commercial success, connected, in its turn, to the number of people who have listened to it since its release date. Playcount values on Last.fm represent a good proxy to assess popularity.
Music Industry Research Paper Topics: The impact of streaming services on music consumption patterns. The role of social media in promoting and marketing music. The effects of piracy on the music industry. The influence of technology on music production and distribution. The relationship between music and mental health.
The integration of artificial intelligence (AI) and music generation has revolutionized the field of musical composition, ushering in a new era of creative possibilities. This comprehensive review explores two groundbreaking studies that illustrate the profound impact of AI in music: AI-driven music remixing and AI-generated music composition. The first approach employs state-of-the-art deep ...
Music production technology has made few advancements over the past few decades. State-of-the-art approaches are based on traditional studio paradigms with new developments primarily focusing on digital modelling of analog equipment. ... Feature papers represent the most advanced research with significant potential for high impact in the field ...
Two essays in the recording section (Chapters 2 and 3) offer empirical evidence of the challenges facing music production in a digital world. At the micro-level, Watson examines freelance music producers and their working conditions within a framework created by the significant mobility and plasticity of contemporary digital recording technologies.
The Transactions of the International Society for Music Information Retrieval publishes novel scientific research in the field of music information retrieval (MIR), an interdisciplinary research area concerned with processing, analysing, organising and accessing music information. We welcome submissions from a wide range of disciplines, including computer science, musicology, cognitive science ...
The digitization of music has changed how we consume, produce, and distribute music. In this paper, we explore the effects of digitization and streaming on the globalization of popular music.
Submit Paper. Musicae Scientiae. Impact Factor: 2.2 / 5-Year Impact Factor: 2.2 . Journal Homepage. Submit Paper. ... Music production: A manual for producers, composers, arrangers, and students. Lanham, MD: Scarecrow Press. ... School of Information Studies, McGill University and Centre for Interdisciplinary Research in Music Media and ...
In conceptualizing possible research topics in music, this paper includes two (2) categories: curriculum-aligned research topics and academic and practical research topics.
Music production involves coordinating efforts among diverse experts, namely recording engineers and musicians. Each contributes specialized work to the production by utilizing shared resources such as music, recorded sound and technologies. ... The research presented in this paper evaluates the album app format and resolves some of the ...
Music Production as a Tool of Research, and Impact Lucy Duran For many years, my professional life has straddled two worlds: that of academia, as Lecturer in African music at the School of Oriental and African Studies; and that of the music industry, as radio presenter and music producer. From this experience, it
1. Introduction. Since the beginning of the oldest societies, music has played a fundamental role in the life of human beings, being undeniably a form of universal expression that unites old and future generations culturally and emotionally (Larsen et al., 2009, Larsen et al., 2010; Naveed et al., 2017).The importance of music in our society has led to creating an industry that includes all ...
With the growth in the accessibility, exposure, and consumption of music in everyday life, people engage with music listening in a wide variety of situations and contexts (Bull, 2006; North et al., 2004).Interestingly, amongst these music listening behaviors, research shows that on most occasions people listen to music when they are engaged with other tasks like studying or working, exercising ...
Women were more likely to initiate playing an instrument than men (37.7% of men versus 20.5% of women), while roughly the same amount of men and women remained actively involved in music in ...
This new system for the production, circulation and consumption of recorded music centred on MSPs has generated a huge amount of commentary, debate and controversy, across multiple online sites: mainstream journalism outlets focused on general news and business; in social media, blogs and the proliferating world of business analysis and commentary; academic research; and across numerous other ...
Modern research investigations give a new avenue for music perception and the understanding of the underlying neurological mechanisms, using neuroimaging, especially magnetic resonance imaging. Multiple brain areas were depicted in the last decades as being of high value for music processing, and further analyses in the neuropsychology field ...
In this paper, we focus on the duality between what is successful and what is significant in the musical context. To this end, we consider a user-generated set of tags collected through an online music platform, whose evolving co-occurrence network mirrors the growing conceptual space underlying music production.
In his book, The anthropology of music, Merriam proposed 10 social functions music can serve (e.g., emotional expression, communication, and symbolic representation). Merriam's work has had a lasting influence among music scholars, but also led many scholars to focus exclusively on the social functions of music.
This study explores the influence of music on audiences' cognition of animation by using both quantitative and qualitative methods. Scholars specializing in aesthetics and music have conducted much research on music aesthetics and music itself. In recent years, further studies on music and film have also been carried out.
In the dense molding process of shiitake mushroom dregs waste, the existence of the arch effect between particles not only restricts the quality of molded particles, but also increases the energy consumption of production, so it is necessary to explore the reasonable arch effect in the molding process. In this paper, the water content of mushroom pomace as an important factor in the molding ...