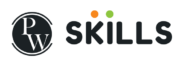

Data Analysis Techniques in Research – Methods, Tools & Examples
Varun Saharawat is a seasoned professional in the fields of SEO and content writing. With a profound knowledge of the intricate aspects of these disciplines, Varun has established himself as a valuable asset in the world of digital marketing and online content creation.
Data analysis techniques in research are essential because they allow researchers to derive meaningful insights from data sets to support their hypotheses or research objectives.
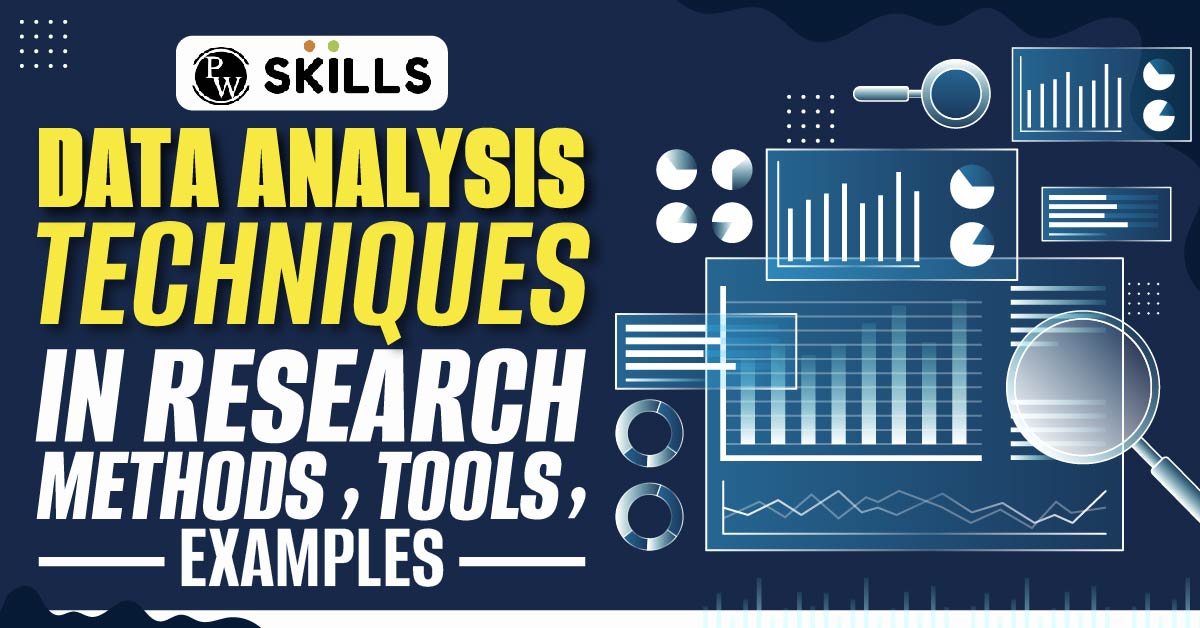
Data Analysis Techniques in Research : While various groups, institutions, and professionals may have diverse approaches to data analysis, a universal definition captures its essence. Data analysis involves refining, transforming, and interpreting raw data to derive actionable insights that guide informed decision-making for businesses.
A straightforward illustration of data analysis emerges when we make everyday decisions, basing our choices on past experiences or predictions of potential outcomes.
If you want to learn more about this topic and acquire valuable skills that will set you apart in today’s data-driven world, we highly recommend enrolling in the Data Analytics Course by Physics Wallah . And as a special offer for our readers, use the coupon code “READER” to get a discount on this course.
Table of Contents
What is Data Analysis?
Data analysis is the systematic process of inspecting, cleaning, transforming, and interpreting data with the objective of discovering valuable insights and drawing meaningful conclusions. This process involves several steps:
- Inspecting : Initial examination of data to understand its structure, quality, and completeness.
- Cleaning : Removing errors, inconsistencies, or irrelevant information to ensure accurate analysis.
- Transforming : Converting data into a format suitable for analysis, such as normalization or aggregation.
- Interpreting : Analyzing the transformed data to identify patterns, trends, and relationships.
Types of Data Analysis Techniques in Research
Data analysis techniques in research are categorized into qualitative and quantitative methods, each with its specific approaches and tools. These techniques are instrumental in extracting meaningful insights, patterns, and relationships from data to support informed decision-making, validate hypotheses, and derive actionable recommendations. Below is an in-depth exploration of the various types of data analysis techniques commonly employed in research:
1) Qualitative Analysis:
Definition: Qualitative analysis focuses on understanding non-numerical data, such as opinions, concepts, or experiences, to derive insights into human behavior, attitudes, and perceptions.
- Content Analysis: Examines textual data, such as interview transcripts, articles, or open-ended survey responses, to identify themes, patterns, or trends.
- Narrative Analysis: Analyzes personal stories or narratives to understand individuals’ experiences, emotions, or perspectives.
- Ethnographic Studies: Involves observing and analyzing cultural practices, behaviors, and norms within specific communities or settings.
2) Quantitative Analysis:
Quantitative analysis emphasizes numerical data and employs statistical methods to explore relationships, patterns, and trends. It encompasses several approaches:
Descriptive Analysis:
- Frequency Distribution: Represents the number of occurrences of distinct values within a dataset.
- Central Tendency: Measures such as mean, median, and mode provide insights into the central values of a dataset.
- Dispersion: Techniques like variance and standard deviation indicate the spread or variability of data.
Diagnostic Analysis:
- Regression Analysis: Assesses the relationship between dependent and independent variables, enabling prediction or understanding causality.
- ANOVA (Analysis of Variance): Examines differences between groups to identify significant variations or effects.
Predictive Analysis:
- Time Series Forecasting: Uses historical data points to predict future trends or outcomes.
- Machine Learning Algorithms: Techniques like decision trees, random forests, and neural networks predict outcomes based on patterns in data.
Prescriptive Analysis:
- Optimization Models: Utilizes linear programming, integer programming, or other optimization techniques to identify the best solutions or strategies.
- Simulation: Mimics real-world scenarios to evaluate various strategies or decisions and determine optimal outcomes.
Specific Techniques:
- Monte Carlo Simulation: Models probabilistic outcomes to assess risk and uncertainty.
- Factor Analysis: Reduces the dimensionality of data by identifying underlying factors or components.
- Cohort Analysis: Studies specific groups or cohorts over time to understand trends, behaviors, or patterns within these groups.
- Cluster Analysis: Classifies objects or individuals into homogeneous groups or clusters based on similarities or attributes.
- Sentiment Analysis: Uses natural language processing and machine learning techniques to determine sentiment, emotions, or opinions from textual data.
Also Read: AI and Predictive Analytics: Examples, Tools, Uses, Ai Vs Predictive Analytics
Data Analysis Techniques in Research Examples
To provide a clearer understanding of how data analysis techniques are applied in research, let’s consider a hypothetical research study focused on evaluating the impact of online learning platforms on students’ academic performance.
Research Objective:
Determine if students using online learning platforms achieve higher academic performance compared to those relying solely on traditional classroom instruction.
Data Collection:
- Quantitative Data: Academic scores (grades) of students using online platforms and those using traditional classroom methods.
- Qualitative Data: Feedback from students regarding their learning experiences, challenges faced, and preferences.
Data Analysis Techniques Applied:
1) Descriptive Analysis:
- Calculate the mean, median, and mode of academic scores for both groups.
- Create frequency distributions to represent the distribution of grades in each group.
2) Diagnostic Analysis:
- Conduct an Analysis of Variance (ANOVA) to determine if there’s a statistically significant difference in academic scores between the two groups.
- Perform Regression Analysis to assess the relationship between the time spent on online platforms and academic performance.
3) Predictive Analysis:
- Utilize Time Series Forecasting to predict future academic performance trends based on historical data.
- Implement Machine Learning algorithms to develop a predictive model that identifies factors contributing to academic success on online platforms.
4) Prescriptive Analysis:
- Apply Optimization Models to identify the optimal combination of online learning resources (e.g., video lectures, interactive quizzes) that maximize academic performance.
- Use Simulation Techniques to evaluate different scenarios, such as varying student engagement levels with online resources, to determine the most effective strategies for improving learning outcomes.
5) Specific Techniques:
- Conduct Factor Analysis on qualitative feedback to identify common themes or factors influencing students’ perceptions and experiences with online learning.
- Perform Cluster Analysis to segment students based on their engagement levels, preferences, or academic outcomes, enabling targeted interventions or personalized learning strategies.
- Apply Sentiment Analysis on textual feedback to categorize students’ sentiments as positive, negative, or neutral regarding online learning experiences.
By applying a combination of qualitative and quantitative data analysis techniques, this research example aims to provide comprehensive insights into the effectiveness of online learning platforms.
Also Read: Learning Path to Become a Data Analyst in 2024
Data Analysis Techniques in Quantitative Research
Quantitative research involves collecting numerical data to examine relationships, test hypotheses, and make predictions. Various data analysis techniques are employed to interpret and draw conclusions from quantitative data. Here are some key data analysis techniques commonly used in quantitative research:
1) Descriptive Statistics:
- Description: Descriptive statistics are used to summarize and describe the main aspects of a dataset, such as central tendency (mean, median, mode), variability (range, variance, standard deviation), and distribution (skewness, kurtosis).
- Applications: Summarizing data, identifying patterns, and providing initial insights into the dataset.
2) Inferential Statistics:
- Description: Inferential statistics involve making predictions or inferences about a population based on a sample of data. This technique includes hypothesis testing, confidence intervals, t-tests, chi-square tests, analysis of variance (ANOVA), regression analysis, and correlation analysis.
- Applications: Testing hypotheses, making predictions, and generalizing findings from a sample to a larger population.
3) Regression Analysis:
- Description: Regression analysis is a statistical technique used to model and examine the relationship between a dependent variable and one or more independent variables. Linear regression, multiple regression, logistic regression, and nonlinear regression are common types of regression analysis .
- Applications: Predicting outcomes, identifying relationships between variables, and understanding the impact of independent variables on the dependent variable.
4) Correlation Analysis:
- Description: Correlation analysis is used to measure and assess the strength and direction of the relationship between two or more variables. The Pearson correlation coefficient, Spearman rank correlation coefficient, and Kendall’s tau are commonly used measures of correlation.
- Applications: Identifying associations between variables and assessing the degree and nature of the relationship.
5) Factor Analysis:
- Description: Factor analysis is a multivariate statistical technique used to identify and analyze underlying relationships or factors among a set of observed variables. It helps in reducing the dimensionality of data and identifying latent variables or constructs.
- Applications: Identifying underlying factors or constructs, simplifying data structures, and understanding the underlying relationships among variables.
6) Time Series Analysis:
- Description: Time series analysis involves analyzing data collected or recorded over a specific period at regular intervals to identify patterns, trends, and seasonality. Techniques such as moving averages, exponential smoothing, autoregressive integrated moving average (ARIMA), and Fourier analysis are used.
- Applications: Forecasting future trends, analyzing seasonal patterns, and understanding time-dependent relationships in data.
7) ANOVA (Analysis of Variance):
- Description: Analysis of variance (ANOVA) is a statistical technique used to analyze and compare the means of two or more groups or treatments to determine if they are statistically different from each other. One-way ANOVA, two-way ANOVA, and MANOVA (Multivariate Analysis of Variance) are common types of ANOVA.
- Applications: Comparing group means, testing hypotheses, and determining the effects of categorical independent variables on a continuous dependent variable.
8) Chi-Square Tests:
- Description: Chi-square tests are non-parametric statistical tests used to assess the association between categorical variables in a contingency table. The Chi-square test of independence, goodness-of-fit test, and test of homogeneity are common chi-square tests.
- Applications: Testing relationships between categorical variables, assessing goodness-of-fit, and evaluating independence.
These quantitative data analysis techniques provide researchers with valuable tools and methods to analyze, interpret, and derive meaningful insights from numerical data. The selection of a specific technique often depends on the research objectives, the nature of the data, and the underlying assumptions of the statistical methods being used.
Also Read: Analysis vs. Analytics: How Are They Different?
Data Analysis Methods
Data analysis methods refer to the techniques and procedures used to analyze, interpret, and draw conclusions from data. These methods are essential for transforming raw data into meaningful insights, facilitating decision-making processes, and driving strategies across various fields. Here are some common data analysis methods:
- Description: Descriptive statistics summarize and organize data to provide a clear and concise overview of the dataset. Measures such as mean, median, mode, range, variance, and standard deviation are commonly used.
- Description: Inferential statistics involve making predictions or inferences about a population based on a sample of data. Techniques such as hypothesis testing, confidence intervals, and regression analysis are used.
3) Exploratory Data Analysis (EDA):
- Description: EDA techniques involve visually exploring and analyzing data to discover patterns, relationships, anomalies, and insights. Methods such as scatter plots, histograms, box plots, and correlation matrices are utilized.
- Applications: Identifying trends, patterns, outliers, and relationships within the dataset.
4) Predictive Analytics:
- Description: Predictive analytics use statistical algorithms and machine learning techniques to analyze historical data and make predictions about future events or outcomes. Techniques such as regression analysis, time series forecasting, and machine learning algorithms (e.g., decision trees, random forests, neural networks) are employed.
- Applications: Forecasting future trends, predicting outcomes, and identifying potential risks or opportunities.
5) Prescriptive Analytics:
- Description: Prescriptive analytics involve analyzing data to recommend actions or strategies that optimize specific objectives or outcomes. Optimization techniques, simulation models, and decision-making algorithms are utilized.
- Applications: Recommending optimal strategies, decision-making support, and resource allocation.
6) Qualitative Data Analysis:
- Description: Qualitative data analysis involves analyzing non-numerical data, such as text, images, videos, or audio, to identify themes, patterns, and insights. Methods such as content analysis, thematic analysis, and narrative analysis are used.
- Applications: Understanding human behavior, attitudes, perceptions, and experiences.
7) Big Data Analytics:
- Description: Big data analytics methods are designed to analyze large volumes of structured and unstructured data to extract valuable insights. Technologies such as Hadoop, Spark, and NoSQL databases are used to process and analyze big data.
- Applications: Analyzing large datasets, identifying trends, patterns, and insights from big data sources.
8) Text Analytics:
- Description: Text analytics methods involve analyzing textual data, such as customer reviews, social media posts, emails, and documents, to extract meaningful information and insights. Techniques such as sentiment analysis, text mining, and natural language processing (NLP) are used.
- Applications: Analyzing customer feedback, monitoring brand reputation, and extracting insights from textual data sources.
These data analysis methods are instrumental in transforming data into actionable insights, informing decision-making processes, and driving organizational success across various sectors, including business, healthcare, finance, marketing, and research. The selection of a specific method often depends on the nature of the data, the research objectives, and the analytical requirements of the project or organization.
Also Read: Quantitative Data Analysis: Types, Analysis & Examples
Data Analysis Tools
Data analysis tools are essential instruments that facilitate the process of examining, cleaning, transforming, and modeling data to uncover useful information, make informed decisions, and drive strategies. Here are some prominent data analysis tools widely used across various industries:
1) Microsoft Excel:
- Description: A spreadsheet software that offers basic to advanced data analysis features, including pivot tables, data visualization tools, and statistical functions.
- Applications: Data cleaning, basic statistical analysis, visualization, and reporting.
2) R Programming Language :
- Description: An open-source programming language specifically designed for statistical computing and data visualization.
- Applications: Advanced statistical analysis, data manipulation, visualization, and machine learning.
3) Python (with Libraries like Pandas, NumPy, Matplotlib, and Seaborn):
- Description: A versatile programming language with libraries that support data manipulation, analysis, and visualization.
- Applications: Data cleaning, statistical analysis, machine learning, and data visualization.
4) SPSS (Statistical Package for the Social Sciences):
- Description: A comprehensive statistical software suite used for data analysis, data mining, and predictive analytics.
- Applications: Descriptive statistics, hypothesis testing, regression analysis, and advanced analytics.
5) SAS (Statistical Analysis System):
- Description: A software suite used for advanced analytics, multivariate analysis, and predictive modeling.
- Applications: Data management, statistical analysis, predictive modeling, and business intelligence.
6) Tableau:
- Description: A data visualization tool that allows users to create interactive and shareable dashboards and reports.
- Applications: Data visualization , business intelligence , and interactive dashboard creation.
7) Power BI:
- Description: A business analytics tool developed by Microsoft that provides interactive visualizations and business intelligence capabilities.
- Applications: Data visualization, business intelligence, reporting, and dashboard creation.
8) SQL (Structured Query Language) Databases (e.g., MySQL, PostgreSQL, Microsoft SQL Server):
- Description: Database management systems that support data storage, retrieval, and manipulation using SQL queries.
- Applications: Data retrieval, data cleaning, data transformation, and database management.
9) Apache Spark:
- Description: A fast and general-purpose distributed computing system designed for big data processing and analytics.
- Applications: Big data processing, machine learning, data streaming, and real-time analytics.
10) IBM SPSS Modeler:
- Description: A data mining software application used for building predictive models and conducting advanced analytics.
- Applications: Predictive modeling, data mining, statistical analysis, and decision optimization.
These tools serve various purposes and cater to different data analysis needs, from basic statistical analysis and data visualization to advanced analytics, machine learning, and big data processing. The choice of a specific tool often depends on the nature of the data, the complexity of the analysis, and the specific requirements of the project or organization.
Also Read: How to Analyze Survey Data: Methods & Examples
Importance of Data Analysis in Research
The importance of data analysis in research cannot be overstated; it serves as the backbone of any scientific investigation or study. Here are several key reasons why data analysis is crucial in the research process:
- Data analysis helps ensure that the results obtained are valid and reliable. By systematically examining the data, researchers can identify any inconsistencies or anomalies that may affect the credibility of the findings.
- Effective data analysis provides researchers with the necessary information to make informed decisions. By interpreting the collected data, researchers can draw conclusions, make predictions, or formulate recommendations based on evidence rather than intuition or guesswork.
- Data analysis allows researchers to identify patterns, trends, and relationships within the data. This can lead to a deeper understanding of the research topic, enabling researchers to uncover insights that may not be immediately apparent.
- In empirical research, data analysis plays a critical role in testing hypotheses. Researchers collect data to either support or refute their hypotheses, and data analysis provides the tools and techniques to evaluate these hypotheses rigorously.
- Transparent and well-executed data analysis enhances the credibility of research findings. By clearly documenting the data analysis methods and procedures, researchers allow others to replicate the study, thereby contributing to the reproducibility of research findings.
- In fields such as business or healthcare, data analysis helps organizations allocate resources more efficiently. By analyzing data on consumer behavior, market trends, or patient outcomes, organizations can make strategic decisions about resource allocation, budgeting, and planning.
- In public policy and social sciences, data analysis is instrumental in developing and evaluating policies and interventions. By analyzing data on social, economic, or environmental factors, policymakers can assess the effectiveness of existing policies and inform the development of new ones.
- Data analysis allows for continuous improvement in research methods and practices. By analyzing past research projects, identifying areas for improvement, and implementing changes based on data-driven insights, researchers can refine their approaches and enhance the quality of future research endeavors.
However, it is important to remember that mastering these techniques requires practice and continuous learning. That’s why we highly recommend the Data Analytics Course by Physics Wallah . Not only does it cover all the fundamentals of data analysis, but it also provides hands-on experience with various tools such as Excel, Python, and Tableau. Plus, if you use the “ READER ” coupon code at checkout, you can get a special discount on the course.
For Latest Tech Related Information, Join Our Official Free Telegram Group : PW Skills Telegram Group
Data Analysis Techniques in Research FAQs
What are the 5 techniques for data analysis.
The five techniques for data analysis include: Descriptive Analysis Diagnostic Analysis Predictive Analysis Prescriptive Analysis Qualitative Analysis
What are techniques of data analysis in research?
Techniques of data analysis in research encompass both qualitative and quantitative methods. These techniques involve processes like summarizing raw data, investigating causes of events, forecasting future outcomes, offering recommendations based on predictions, and examining non-numerical data to understand concepts or experiences.
What are the 3 methods of data analysis?
The three primary methods of data analysis are: Qualitative Analysis Quantitative Analysis Mixed-Methods Analysis
What are the four types of data analysis techniques?
The four types of data analysis techniques are: Descriptive Analysis Diagnostic Analysis Predictive Analysis Prescriptive Analysis
- 10 Best Companies For Data Analysis Internships 2024
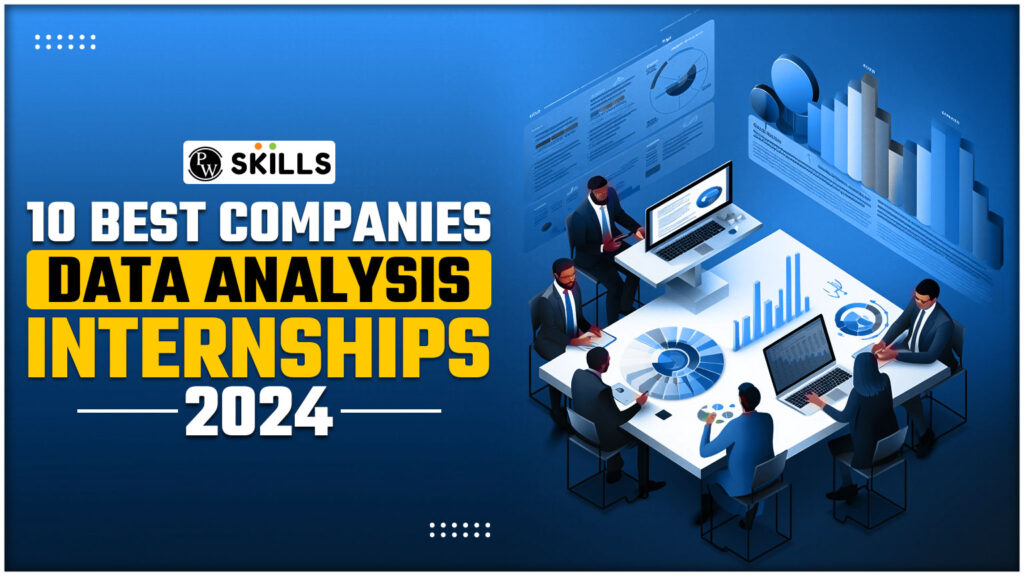
This article will help you provide the top 10 best companies for a Data Analysis Internship which will not only…
- Top Best Big Data Analytics Classes 2024
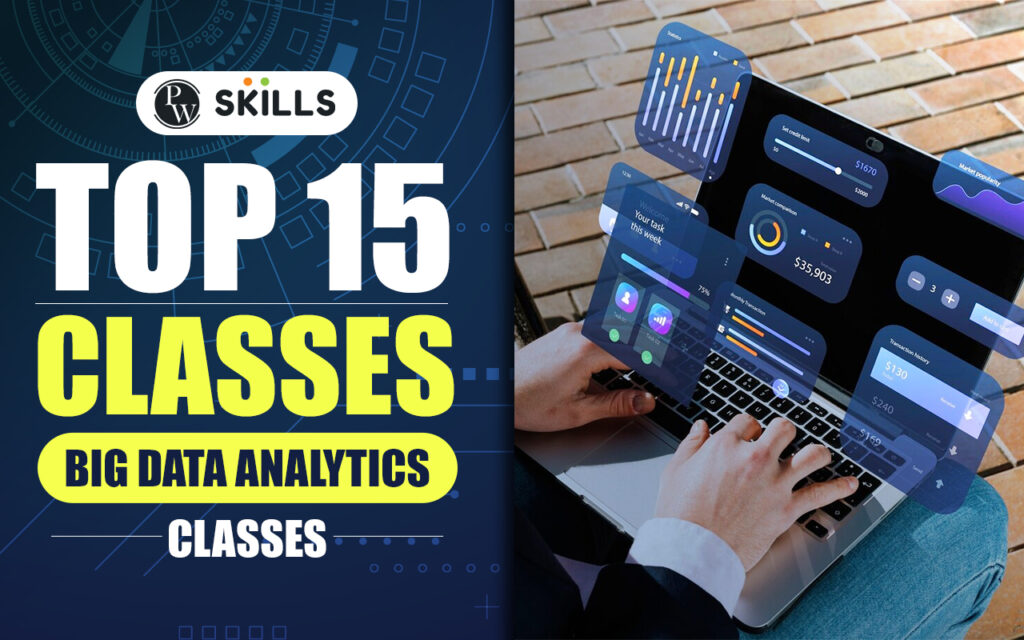
Many websites and institutions provide online remote big data analytics classes to help you learn and also earn certifications for…
- Data Analyst Roadmap 2024: Responsibilities, Skills Required, Career Path
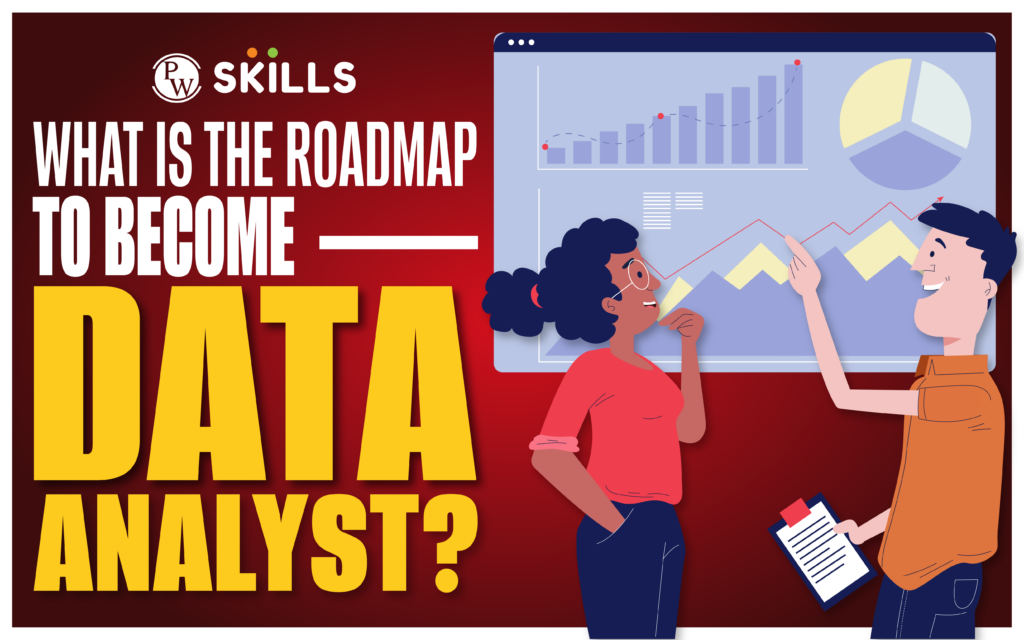
Data Analyst Roadmap: The field of data analysis is booming and is very rewarding for those with the right skills.…
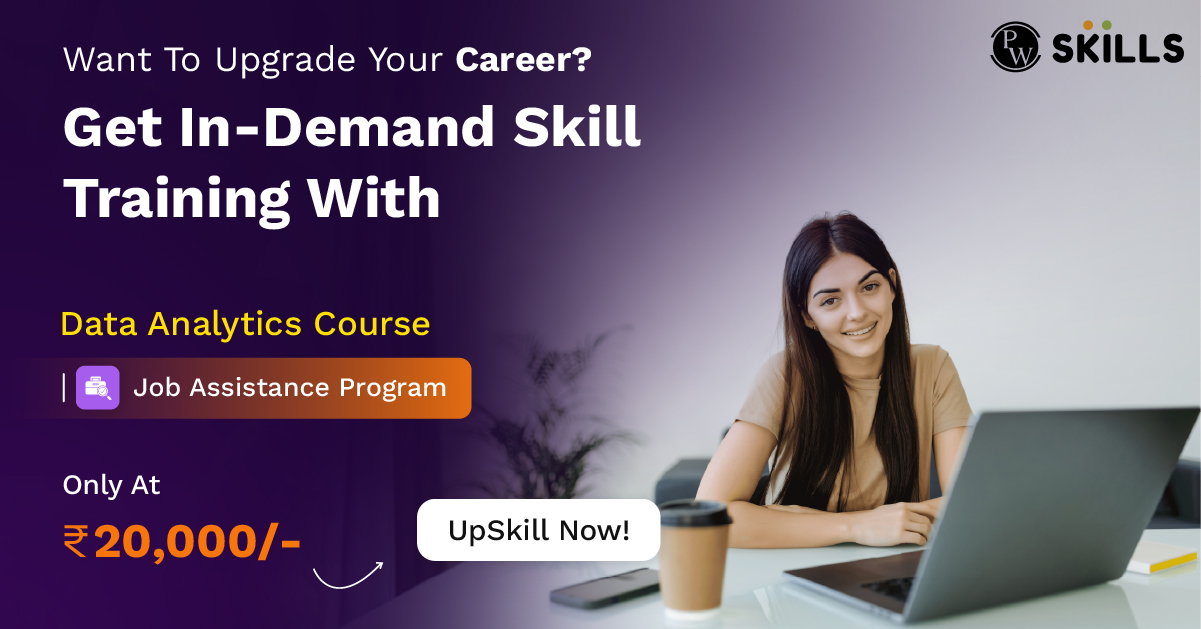
Related Articles
- The Best Data And Analytics Courses For Beginners
- Best Courses For Data Analytics: Top 10 Courses For Your Career in Trend
- BI & Analytics: What’s The Difference?
- Predictive Analysis: Predicting the Future with Data
- Graph Analytics – What Is it and Why Does It Matter?
- How to Analysis of Survey Data: Methods & Examples
- Google Data Analytics Professional Certificate Review, Cost, Eligibility
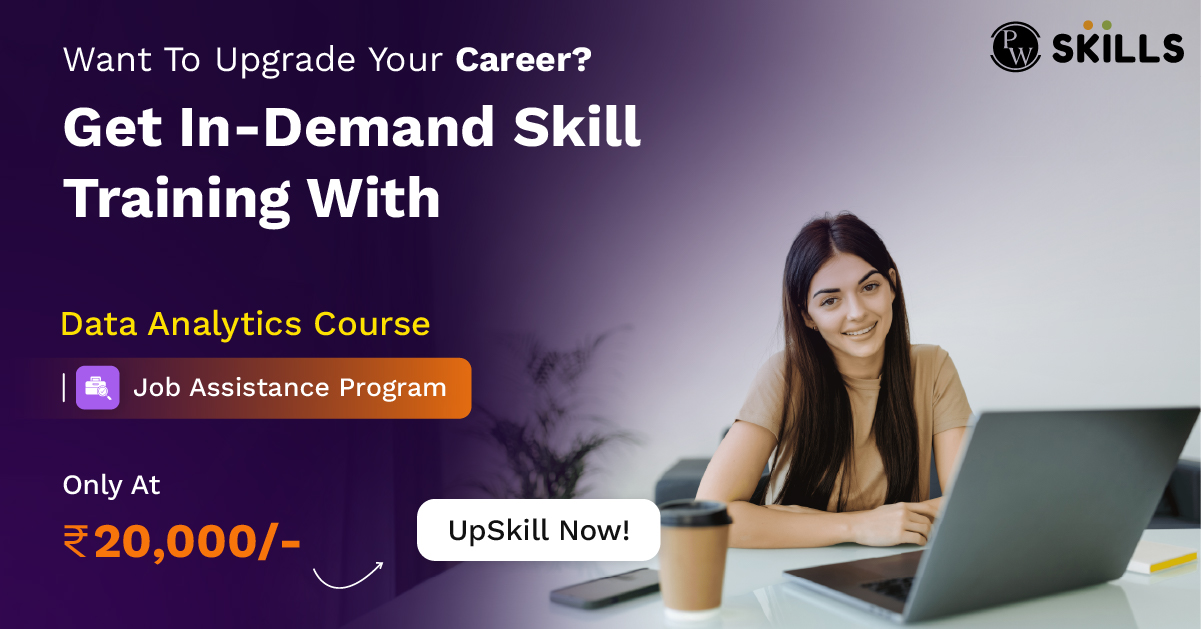
Have a language expert improve your writing
Run a free plagiarism check in 10 minutes, generate accurate citations for free.
- Knowledge Base
The Beginner's Guide to Statistical Analysis | 5 Steps & Examples
Statistical analysis means investigating trends, patterns, and relationships using quantitative data . It is an important research tool used by scientists, governments, businesses, and other organizations.
To draw valid conclusions, statistical analysis requires careful planning from the very start of the research process . You need to specify your hypotheses and make decisions about your research design, sample size, and sampling procedure.
After collecting data from your sample, you can organize and summarize the data using descriptive statistics . Then, you can use inferential statistics to formally test hypotheses and make estimates about the population. Finally, you can interpret and generalize your findings.
This article is a practical introduction to statistical analysis for students and researchers. We’ll walk you through the steps using two research examples. The first investigates a potential cause-and-effect relationship, while the second investigates a potential correlation between variables.
Table of contents
Step 1: write your hypotheses and plan your research design, step 2: collect data from a sample, step 3: summarize your data with descriptive statistics, step 4: test hypotheses or make estimates with inferential statistics, step 5: interpret your results, other interesting articles.
To collect valid data for statistical analysis, you first need to specify your hypotheses and plan out your research design.
Writing statistical hypotheses
The goal of research is often to investigate a relationship between variables within a population . You start with a prediction, and use statistical analysis to test that prediction.
A statistical hypothesis is a formal way of writing a prediction about a population. Every research prediction is rephrased into null and alternative hypotheses that can be tested using sample data.
While the null hypothesis always predicts no effect or no relationship between variables, the alternative hypothesis states your research prediction of an effect or relationship.
- Null hypothesis: A 5-minute meditation exercise will have no effect on math test scores in teenagers.
- Alternative hypothesis: A 5-minute meditation exercise will improve math test scores in teenagers.
- Null hypothesis: Parental income and GPA have no relationship with each other in college students.
- Alternative hypothesis: Parental income and GPA are positively correlated in college students.
Planning your research design
A research design is your overall strategy for data collection and analysis. It determines the statistical tests you can use to test your hypothesis later on.
First, decide whether your research will use a descriptive, correlational, or experimental design. Experiments directly influence variables, whereas descriptive and correlational studies only measure variables.
- In an experimental design , you can assess a cause-and-effect relationship (e.g., the effect of meditation on test scores) using statistical tests of comparison or regression.
- In a correlational design , you can explore relationships between variables (e.g., parental income and GPA) without any assumption of causality using correlation coefficients and significance tests.
- In a descriptive design , you can study the characteristics of a population or phenomenon (e.g., the prevalence of anxiety in U.S. college students) using statistical tests to draw inferences from sample data.
Your research design also concerns whether you’ll compare participants at the group level or individual level, or both.
- In a between-subjects design , you compare the group-level outcomes of participants who have been exposed to different treatments (e.g., those who performed a meditation exercise vs those who didn’t).
- In a within-subjects design , you compare repeated measures from participants who have participated in all treatments of a study (e.g., scores from before and after performing a meditation exercise).
- In a mixed (factorial) design , one variable is altered between subjects and another is altered within subjects (e.g., pretest and posttest scores from participants who either did or didn’t do a meditation exercise).
- Experimental
- Correlational
First, you’ll take baseline test scores from participants. Then, your participants will undergo a 5-minute meditation exercise. Finally, you’ll record participants’ scores from a second math test.
In this experiment, the independent variable is the 5-minute meditation exercise, and the dependent variable is the math test score from before and after the intervention. Example: Correlational research design In a correlational study, you test whether there is a relationship between parental income and GPA in graduating college students. To collect your data, you will ask participants to fill in a survey and self-report their parents’ incomes and their own GPA.
Measuring variables
When planning a research design, you should operationalize your variables and decide exactly how you will measure them.
For statistical analysis, it’s important to consider the level of measurement of your variables, which tells you what kind of data they contain:
- Categorical data represents groupings. These may be nominal (e.g., gender) or ordinal (e.g. level of language ability).
- Quantitative data represents amounts. These may be on an interval scale (e.g. test score) or a ratio scale (e.g. age).
Many variables can be measured at different levels of precision. For example, age data can be quantitative (8 years old) or categorical (young). If a variable is coded numerically (e.g., level of agreement from 1–5), it doesn’t automatically mean that it’s quantitative instead of categorical.
Identifying the measurement level is important for choosing appropriate statistics and hypothesis tests. For example, you can calculate a mean score with quantitative data, but not with categorical data.
In a research study, along with measures of your variables of interest, you’ll often collect data on relevant participant characteristics.
Variable | Type of data |
---|---|
Age | Quantitative (ratio) |
Gender | Categorical (nominal) |
Race or ethnicity | Categorical (nominal) |
Baseline test scores | Quantitative (interval) |
Final test scores | Quantitative (interval) |
Parental income | Quantitative (ratio) |
---|---|
GPA | Quantitative (interval) |
Receive feedback on language, structure, and formatting
Professional editors proofread and edit your paper by focusing on:
- Academic style
- Vague sentences
- Style consistency
See an example
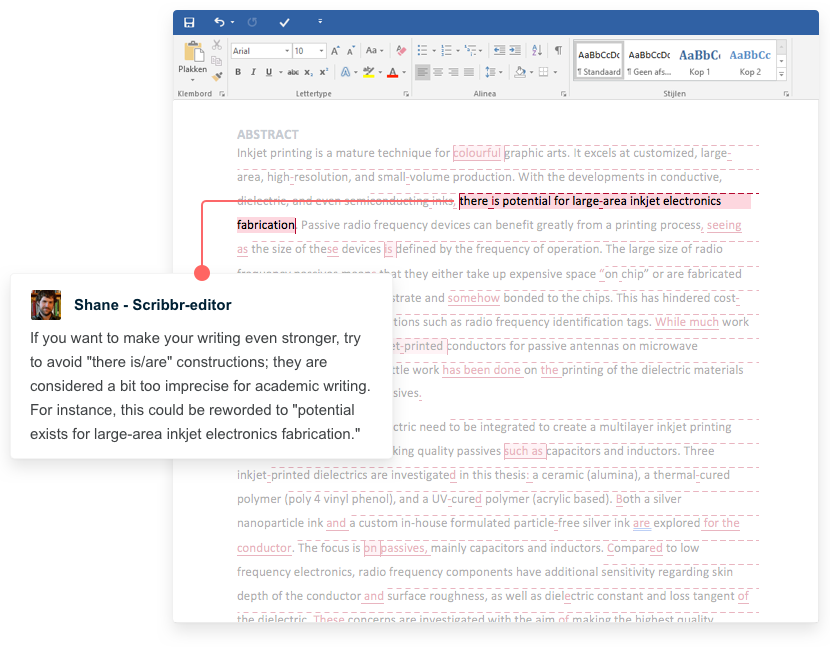
In most cases, it’s too difficult or expensive to collect data from every member of the population you’re interested in studying. Instead, you’ll collect data from a sample.
Statistical analysis allows you to apply your findings beyond your own sample as long as you use appropriate sampling procedures . You should aim for a sample that is representative of the population.
Sampling for statistical analysis
There are two main approaches to selecting a sample.
- Probability sampling: every member of the population has a chance of being selected for the study through random selection.
- Non-probability sampling: some members of the population are more likely than others to be selected for the study because of criteria such as convenience or voluntary self-selection.
In theory, for highly generalizable findings, you should use a probability sampling method. Random selection reduces several types of research bias , like sampling bias , and ensures that data from your sample is actually typical of the population. Parametric tests can be used to make strong statistical inferences when data are collected using probability sampling.
But in practice, it’s rarely possible to gather the ideal sample. While non-probability samples are more likely to at risk for biases like self-selection bias , they are much easier to recruit and collect data from. Non-parametric tests are more appropriate for non-probability samples, but they result in weaker inferences about the population.
If you want to use parametric tests for non-probability samples, you have to make the case that:
- your sample is representative of the population you’re generalizing your findings to.
- your sample lacks systematic bias.
Keep in mind that external validity means that you can only generalize your conclusions to others who share the characteristics of your sample. For instance, results from Western, Educated, Industrialized, Rich and Democratic samples (e.g., college students in the US) aren’t automatically applicable to all non-WEIRD populations.
If you apply parametric tests to data from non-probability samples, be sure to elaborate on the limitations of how far your results can be generalized in your discussion section .
Create an appropriate sampling procedure
Based on the resources available for your research, decide on how you’ll recruit participants.
- Will you have resources to advertise your study widely, including outside of your university setting?
- Will you have the means to recruit a diverse sample that represents a broad population?
- Do you have time to contact and follow up with members of hard-to-reach groups?
Your participants are self-selected by their schools. Although you’re using a non-probability sample, you aim for a diverse and representative sample. Example: Sampling (correlational study) Your main population of interest is male college students in the US. Using social media advertising, you recruit senior-year male college students from a smaller subpopulation: seven universities in the Boston area.
Calculate sufficient sample size
Before recruiting participants, decide on your sample size either by looking at other studies in your field or using statistics. A sample that’s too small may be unrepresentative of the sample, while a sample that’s too large will be more costly than necessary.
There are many sample size calculators online. Different formulas are used depending on whether you have subgroups or how rigorous your study should be (e.g., in clinical research). As a rule of thumb, a minimum of 30 units or more per subgroup is necessary.
To use these calculators, you have to understand and input these key components:
- Significance level (alpha): the risk of rejecting a true null hypothesis that you are willing to take, usually set at 5%.
- Statistical power : the probability of your study detecting an effect of a certain size if there is one, usually 80% or higher.
- Expected effect size : a standardized indication of how large the expected result of your study will be, usually based on other similar studies.
- Population standard deviation: an estimate of the population parameter based on a previous study or a pilot study of your own.
Once you’ve collected all of your data, you can inspect them and calculate descriptive statistics that summarize them.
Inspect your data
There are various ways to inspect your data, including the following:
- Organizing data from each variable in frequency distribution tables .
- Displaying data from a key variable in a bar chart to view the distribution of responses.
- Visualizing the relationship between two variables using a scatter plot .
By visualizing your data in tables and graphs, you can assess whether your data follow a skewed or normal distribution and whether there are any outliers or missing data.
A normal distribution means that your data are symmetrically distributed around a center where most values lie, with the values tapering off at the tail ends.
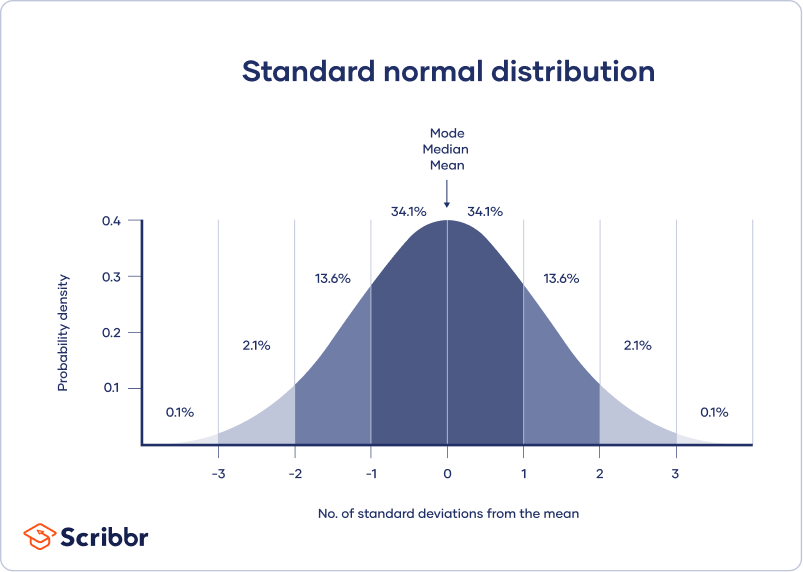
In contrast, a skewed distribution is asymmetric and has more values on one end than the other. The shape of the distribution is important to keep in mind because only some descriptive statistics should be used with skewed distributions.
Extreme outliers can also produce misleading statistics, so you may need a systematic approach to dealing with these values.
Calculate measures of central tendency
Measures of central tendency describe where most of the values in a data set lie. Three main measures of central tendency are often reported:
- Mode : the most popular response or value in the data set.
- Median : the value in the exact middle of the data set when ordered from low to high.
- Mean : the sum of all values divided by the number of values.
However, depending on the shape of the distribution and level of measurement, only one or two of these measures may be appropriate. For example, many demographic characteristics can only be described using the mode or proportions, while a variable like reaction time may not have a mode at all.
Calculate measures of variability
Measures of variability tell you how spread out the values in a data set are. Four main measures of variability are often reported:
- Range : the highest value minus the lowest value of the data set.
- Interquartile range : the range of the middle half of the data set.
- Standard deviation : the average distance between each value in your data set and the mean.
- Variance : the square of the standard deviation.
Once again, the shape of the distribution and level of measurement should guide your choice of variability statistics. The interquartile range is the best measure for skewed distributions, while standard deviation and variance provide the best information for normal distributions.
Using your table, you should check whether the units of the descriptive statistics are comparable for pretest and posttest scores. For example, are the variance levels similar across the groups? Are there any extreme values? If there are, you may need to identify and remove extreme outliers in your data set or transform your data before performing a statistical test.
Pretest scores | Posttest scores | |
---|---|---|
Mean | 68.44 | 75.25 |
Standard deviation | 9.43 | 9.88 |
Variance | 88.96 | 97.96 |
Range | 36.25 | 45.12 |
30 |
From this table, we can see that the mean score increased after the meditation exercise, and the variances of the two scores are comparable. Next, we can perform a statistical test to find out if this improvement in test scores is statistically significant in the population. Example: Descriptive statistics (correlational study) After collecting data from 653 students, you tabulate descriptive statistics for annual parental income and GPA.
It’s important to check whether you have a broad range of data points. If you don’t, your data may be skewed towards some groups more than others (e.g., high academic achievers), and only limited inferences can be made about a relationship.
Parental income (USD) | GPA | |
---|---|---|
Mean | 62,100 | 3.12 |
Standard deviation | 15,000 | 0.45 |
Variance | 225,000,000 | 0.16 |
Range | 8,000–378,000 | 2.64–4.00 |
653 |
A number that describes a sample is called a statistic , while a number describing a population is called a parameter . Using inferential statistics , you can make conclusions about population parameters based on sample statistics.
Researchers often use two main methods (simultaneously) to make inferences in statistics.
- Estimation: calculating population parameters based on sample statistics.
- Hypothesis testing: a formal process for testing research predictions about the population using samples.
You can make two types of estimates of population parameters from sample statistics:
- A point estimate : a value that represents your best guess of the exact parameter.
- An interval estimate : a range of values that represent your best guess of where the parameter lies.
If your aim is to infer and report population characteristics from sample data, it’s best to use both point and interval estimates in your paper.
You can consider a sample statistic a point estimate for the population parameter when you have a representative sample (e.g., in a wide public opinion poll, the proportion of a sample that supports the current government is taken as the population proportion of government supporters).
There’s always error involved in estimation, so you should also provide a confidence interval as an interval estimate to show the variability around a point estimate.
A confidence interval uses the standard error and the z score from the standard normal distribution to convey where you’d generally expect to find the population parameter most of the time.
Hypothesis testing
Using data from a sample, you can test hypotheses about relationships between variables in the population. Hypothesis testing starts with the assumption that the null hypothesis is true in the population, and you use statistical tests to assess whether the null hypothesis can be rejected or not.
Statistical tests determine where your sample data would lie on an expected distribution of sample data if the null hypothesis were true. These tests give two main outputs:
- A test statistic tells you how much your data differs from the null hypothesis of the test.
- A p value tells you the likelihood of obtaining your results if the null hypothesis is actually true in the population.
Statistical tests come in three main varieties:
- Comparison tests assess group differences in outcomes.
- Regression tests assess cause-and-effect relationships between variables.
- Correlation tests assess relationships between variables without assuming causation.
Your choice of statistical test depends on your research questions, research design, sampling method, and data characteristics.
Parametric tests
Parametric tests make powerful inferences about the population based on sample data. But to use them, some assumptions must be met, and only some types of variables can be used. If your data violate these assumptions, you can perform appropriate data transformations or use alternative non-parametric tests instead.
A regression models the extent to which changes in a predictor variable results in changes in outcome variable(s).
- A simple linear regression includes one predictor variable and one outcome variable.
- A multiple linear regression includes two or more predictor variables and one outcome variable.
Comparison tests usually compare the means of groups. These may be the means of different groups within a sample (e.g., a treatment and control group), the means of one sample group taken at different times (e.g., pretest and posttest scores), or a sample mean and a population mean.
- A t test is for exactly 1 or 2 groups when the sample is small (30 or less).
- A z test is for exactly 1 or 2 groups when the sample is large.
- An ANOVA is for 3 or more groups.
The z and t tests have subtypes based on the number and types of samples and the hypotheses:
- If you have only one sample that you want to compare to a population mean, use a one-sample test .
- If you have paired measurements (within-subjects design), use a dependent (paired) samples test .
- If you have completely separate measurements from two unmatched groups (between-subjects design), use an independent (unpaired) samples test .
- If you expect a difference between groups in a specific direction, use a one-tailed test .
- If you don’t have any expectations for the direction of a difference between groups, use a two-tailed test .
The only parametric correlation test is Pearson’s r . The correlation coefficient ( r ) tells you the strength of a linear relationship between two quantitative variables.
However, to test whether the correlation in the sample is strong enough to be important in the population, you also need to perform a significance test of the correlation coefficient, usually a t test, to obtain a p value. This test uses your sample size to calculate how much the correlation coefficient differs from zero in the population.
You use a dependent-samples, one-tailed t test to assess whether the meditation exercise significantly improved math test scores. The test gives you:
- a t value (test statistic) of 3.00
- a p value of 0.0028
Although Pearson’s r is a test statistic, it doesn’t tell you anything about how significant the correlation is in the population. You also need to test whether this sample correlation coefficient is large enough to demonstrate a correlation in the population.
A t test can also determine how significantly a correlation coefficient differs from zero based on sample size. Since you expect a positive correlation between parental income and GPA, you use a one-sample, one-tailed t test. The t test gives you:
- a t value of 3.08
- a p value of 0.001
Prevent plagiarism. Run a free check.
The final step of statistical analysis is interpreting your results.
Statistical significance
In hypothesis testing, statistical significance is the main criterion for forming conclusions. You compare your p value to a set significance level (usually 0.05) to decide whether your results are statistically significant or non-significant.
Statistically significant results are considered unlikely to have arisen solely due to chance. There is only a very low chance of such a result occurring if the null hypothesis is true in the population.
This means that you believe the meditation intervention, rather than random factors, directly caused the increase in test scores. Example: Interpret your results (correlational study) You compare your p value of 0.001 to your significance threshold of 0.05. With a p value under this threshold, you can reject the null hypothesis. This indicates a statistically significant correlation between parental income and GPA in male college students.
Note that correlation doesn’t always mean causation, because there are often many underlying factors contributing to a complex variable like GPA. Even if one variable is related to another, this may be because of a third variable influencing both of them, or indirect links between the two variables.
Effect size
A statistically significant result doesn’t necessarily mean that there are important real life applications or clinical outcomes for a finding.
In contrast, the effect size indicates the practical significance of your results. It’s important to report effect sizes along with your inferential statistics for a complete picture of your results. You should also report interval estimates of effect sizes if you’re writing an APA style paper .
With a Cohen’s d of 0.72, there’s medium to high practical significance to your finding that the meditation exercise improved test scores. Example: Effect size (correlational study) To determine the effect size of the correlation coefficient, you compare your Pearson’s r value to Cohen’s effect size criteria.
Decision errors
Type I and Type II errors are mistakes made in research conclusions. A Type I error means rejecting the null hypothesis when it’s actually true, while a Type II error means failing to reject the null hypothesis when it’s false.
You can aim to minimize the risk of these errors by selecting an optimal significance level and ensuring high power . However, there’s a trade-off between the two errors, so a fine balance is necessary.
Frequentist versus Bayesian statistics
Traditionally, frequentist statistics emphasizes null hypothesis significance testing and always starts with the assumption of a true null hypothesis.
However, Bayesian statistics has grown in popularity as an alternative approach in the last few decades. In this approach, you use previous research to continually update your hypotheses based on your expectations and observations.
Bayes factor compares the relative strength of evidence for the null versus the alternative hypothesis rather than making a conclusion about rejecting the null hypothesis or not.
If you want to know more about statistics , methodology , or research bias , make sure to check out some of our other articles with explanations and examples.
- Student’s t -distribution
- Normal distribution
- Null and Alternative Hypotheses
- Chi square tests
- Confidence interval
Methodology
- Cluster sampling
- Stratified sampling
- Data cleansing
- Reproducibility vs Replicability
- Peer review
- Likert scale
Research bias
- Implicit bias
- Framing effect
- Cognitive bias
- Placebo effect
- Hawthorne effect
- Hostile attribution bias
- Affect heuristic
Is this article helpful?
Other students also liked.
- Descriptive Statistics | Definitions, Types, Examples
- Inferential Statistics | An Easy Introduction & Examples
- Choosing the Right Statistical Test | Types & Examples
More interesting articles
- Akaike Information Criterion | When & How to Use It (Example)
- An Easy Introduction to Statistical Significance (With Examples)
- An Introduction to t Tests | Definitions, Formula and Examples
- ANOVA in R | A Complete Step-by-Step Guide with Examples
- Central Limit Theorem | Formula, Definition & Examples
- Central Tendency | Understanding the Mean, Median & Mode
- Chi-Square (Χ²) Distributions | Definition & Examples
- Chi-Square (Χ²) Table | Examples & Downloadable Table
- Chi-Square (Χ²) Tests | Types, Formula & Examples
- Chi-Square Goodness of Fit Test | Formula, Guide & Examples
- Chi-Square Test of Independence | Formula, Guide & Examples
- Coefficient of Determination (R²) | Calculation & Interpretation
- Correlation Coefficient | Types, Formulas & Examples
- Frequency Distribution | Tables, Types & Examples
- How to Calculate Standard Deviation (Guide) | Calculator & Examples
- How to Calculate Variance | Calculator, Analysis & Examples
- How to Find Degrees of Freedom | Definition & Formula
- How to Find Interquartile Range (IQR) | Calculator & Examples
- How to Find Outliers | 4 Ways with Examples & Explanation
- How to Find the Geometric Mean | Calculator & Formula
- How to Find the Mean | Definition, Examples & Calculator
- How to Find the Median | Definition, Examples & Calculator
- How to Find the Mode | Definition, Examples & Calculator
- How to Find the Range of a Data Set | Calculator & Formula
- Hypothesis Testing | A Step-by-Step Guide with Easy Examples
- Interval Data and How to Analyze It | Definitions & Examples
- Levels of Measurement | Nominal, Ordinal, Interval and Ratio
- Linear Regression in R | A Step-by-Step Guide & Examples
- Missing Data | Types, Explanation, & Imputation
- Multiple Linear Regression | A Quick Guide (Examples)
- Nominal Data | Definition, Examples, Data Collection & Analysis
- Normal Distribution | Examples, Formulas, & Uses
- Null and Alternative Hypotheses | Definitions & Examples
- One-way ANOVA | When and How to Use It (With Examples)
- Ordinal Data | Definition, Examples, Data Collection & Analysis
- Parameter vs Statistic | Definitions, Differences & Examples
- Pearson Correlation Coefficient (r) | Guide & Examples
- Poisson Distributions | Definition, Formula & Examples
- Probability Distribution | Formula, Types, & Examples
- Quartiles & Quantiles | Calculation, Definition & Interpretation
- Ratio Scales | Definition, Examples, & Data Analysis
- Simple Linear Regression | An Easy Introduction & Examples
- Skewness | Definition, Examples & Formula
- Statistical Power and Why It Matters | A Simple Introduction
- Student's t Table (Free Download) | Guide & Examples
- T-distribution: What it is and how to use it
- Test statistics | Definition, Interpretation, and Examples
- The Standard Normal Distribution | Calculator, Examples & Uses
- Two-Way ANOVA | Examples & When To Use It
- Type I & Type II Errors | Differences, Examples, Visualizations
- Understanding Confidence Intervals | Easy Examples & Formulas
- Understanding P values | Definition and Examples
- Variability | Calculating Range, IQR, Variance, Standard Deviation
- What is Effect Size and Why Does It Matter? (Examples)
- What Is Kurtosis? | Definition, Examples & Formula
- What Is Standard Error? | How to Calculate (Guide with Examples)
What is your plagiarism score?

Quantitative Data Analysis 101
The lingo, methods and techniques, explained simply.
By: Derek Jansen (MBA) and Kerryn Warren (PhD) | December 2020
Quantitative data analysis is one of those things that often strikes fear in students. It’s totally understandable – quantitative analysis is a complex topic, full of daunting lingo , like medians, modes, correlation and regression. Suddenly we’re all wishing we’d paid a little more attention in math class…
The good news is that while quantitative data analysis is a mammoth topic, gaining a working understanding of the basics isn’t that hard , even for those of us who avoid numbers and math . In this post, we’ll break quantitative analysis down into simple , bite-sized chunks so you can approach your research with confidence.
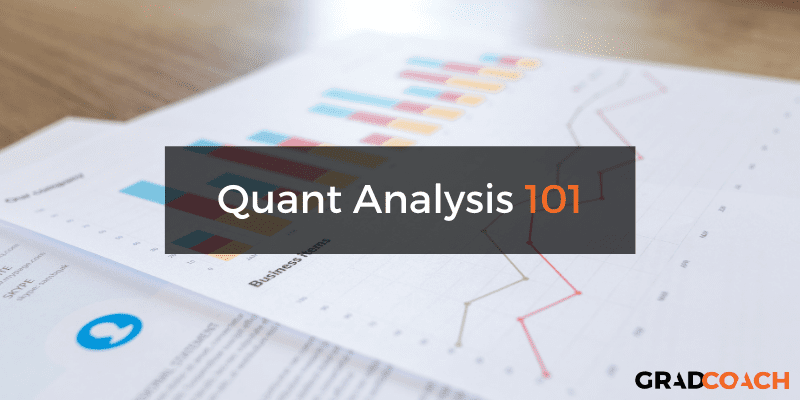
Overview: Quantitative Data Analysis 101
- What (exactly) is quantitative data analysis?
- When to use quantitative analysis
- How quantitative analysis works
The two “branches” of quantitative analysis
- Descriptive statistics 101
- Inferential statistics 101
- How to choose the right quantitative methods
- Recap & summary
What is quantitative data analysis?
Despite being a mouthful, quantitative data analysis simply means analysing data that is numbers-based – or data that can be easily “converted” into numbers without losing any meaning.
For example, category-based variables like gender, ethnicity, or native language could all be “converted” into numbers without losing meaning – for example, English could equal 1, French 2, etc.
This contrasts against qualitative data analysis, where the focus is on words, phrases and expressions that can’t be reduced to numbers. If you’re interested in learning about qualitative analysis, check out our post and video here .
What is quantitative analysis used for?
Quantitative analysis is generally used for three purposes.
- Firstly, it’s used to measure differences between groups . For example, the popularity of different clothing colours or brands.
- Secondly, it’s used to assess relationships between variables . For example, the relationship between weather temperature and voter turnout.
- And third, it’s used to test hypotheses in a scientifically rigorous way. For example, a hypothesis about the impact of a certain vaccine.
Again, this contrasts with qualitative analysis , which can be used to analyse people’s perceptions and feelings about an event or situation. In other words, things that can’t be reduced to numbers.
How does quantitative analysis work?
Well, since quantitative data analysis is all about analysing numbers , it’s no surprise that it involves statistics . Statistical analysis methods form the engine that powers quantitative analysis, and these methods can vary from pretty basic calculations (for example, averages and medians) to more sophisticated analyses (for example, correlations and regressions).
Sounds like gibberish? Don’t worry. We’ll explain all of that in this post. Importantly, you don’t need to be a statistician or math wiz to pull off a good quantitative analysis. We’ll break down all the technical mumbo jumbo in this post.
Need a helping hand?
As I mentioned, quantitative analysis is powered by statistical analysis methods . There are two main “branches” of statistical methods that are used – descriptive statistics and inferential statistics . In your research, you might only use descriptive statistics, or you might use a mix of both , depending on what you’re trying to figure out. In other words, depending on your research questions, aims and objectives . I’ll explain how to choose your methods later.
So, what are descriptive and inferential statistics?
Well, before I can explain that, we need to take a quick detour to explain some lingo. To understand the difference between these two branches of statistics, you need to understand two important words. These words are population and sample .
First up, population . In statistics, the population is the entire group of people (or animals or organisations or whatever) that you’re interested in researching. For example, if you were interested in researching Tesla owners in the US, then the population would be all Tesla owners in the US.
However, it’s extremely unlikely that you’re going to be able to interview or survey every single Tesla owner in the US. Realistically, you’ll likely only get access to a few hundred, or maybe a few thousand owners using an online survey. This smaller group of accessible people whose data you actually collect is called your sample .
So, to recap – the population is the entire group of people you’re interested in, and the sample is the subset of the population that you can actually get access to. In other words, the population is the full chocolate cake , whereas the sample is a slice of that cake.
So, why is this sample-population thing important?
Well, descriptive statistics focus on describing the sample , while inferential statistics aim to make predictions about the population, based on the findings within the sample. In other words, we use one group of statistical methods – descriptive statistics – to investigate the slice of cake, and another group of methods – inferential statistics – to draw conclusions about the entire cake. There I go with the cake analogy again…
With that out the way, let’s take a closer look at each of these branches in more detail.
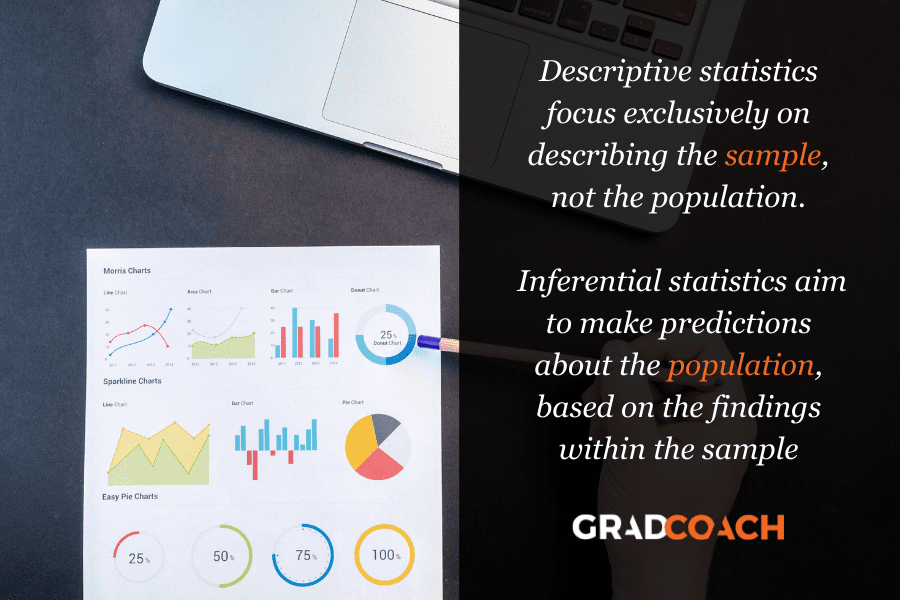
Branch 1: Descriptive Statistics
Descriptive statistics serve a simple but critically important role in your research – to describe your data set – hence the name. In other words, they help you understand the details of your sample . Unlike inferential statistics (which we’ll get to soon), descriptive statistics don’t aim to make inferences or predictions about the entire population – they’re purely interested in the details of your specific sample .
When you’re writing up your analysis, descriptive statistics are the first set of stats you’ll cover, before moving on to inferential statistics. But, that said, depending on your research objectives and research questions , they may be the only type of statistics you use. We’ll explore that a little later.
So, what kind of statistics are usually covered in this section?
Some common statistical tests used in this branch include the following:
- Mean – this is simply the mathematical average of a range of numbers.
- Median – this is the midpoint in a range of numbers when the numbers are arranged in numerical order. If the data set makes up an odd number, then the median is the number right in the middle of the set. If the data set makes up an even number, then the median is the midpoint between the two middle numbers.
- Mode – this is simply the most commonly occurring number in the data set.
- In cases where most of the numbers are quite close to the average, the standard deviation will be relatively low.
- Conversely, in cases where the numbers are scattered all over the place, the standard deviation will be relatively high.
- Skewness . As the name suggests, skewness indicates how symmetrical a range of numbers is. In other words, do they tend to cluster into a smooth bell curve shape in the middle of the graph, or do they skew to the left or right?
Feeling a bit confused? Let’s look at a practical example using a small data set.
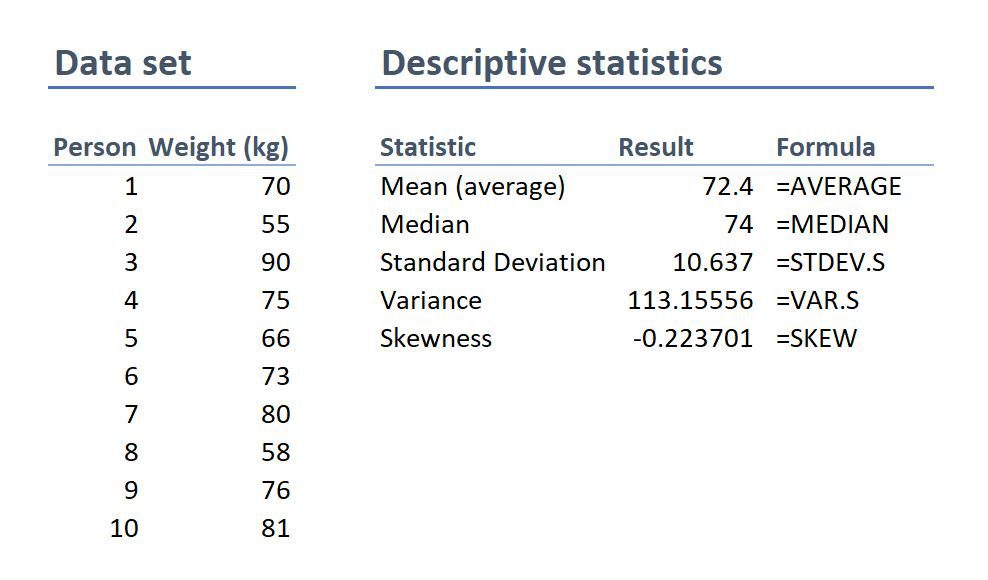
On the left-hand side is the data set. This details the bodyweight of a sample of 10 people. On the right-hand side, we have the descriptive statistics. Let’s take a look at each of them.
First, we can see that the mean weight is 72.4 kilograms. In other words, the average weight across the sample is 72.4 kilograms. Straightforward.
Next, we can see that the median is very similar to the mean (the average). This suggests that this data set has a reasonably symmetrical distribution (in other words, a relatively smooth, centred distribution of weights, clustered towards the centre).
In terms of the mode , there is no mode in this data set. This is because each number is present only once and so there cannot be a “most common number”. If there were two people who were both 65 kilograms, for example, then the mode would be 65.
Next up is the standard deviation . 10.6 indicates that there’s quite a wide spread of numbers. We can see this quite easily by looking at the numbers themselves, which range from 55 to 90, which is quite a stretch from the mean of 72.4.
And lastly, the skewness of -0.2 tells us that the data is very slightly negatively skewed. This makes sense since the mean and the median are slightly different.
As you can see, these descriptive statistics give us some useful insight into the data set. Of course, this is a very small data set (only 10 records), so we can’t read into these statistics too much. Also, keep in mind that this is not a list of all possible descriptive statistics – just the most common ones.
But why do all of these numbers matter?
While these descriptive statistics are all fairly basic, they’re important for a few reasons:
- Firstly, they help you get both a macro and micro-level view of your data. In other words, they help you understand both the big picture and the finer details.
- Secondly, they help you spot potential errors in the data – for example, if an average is way higher than you’d expect, or responses to a question are highly varied, this can act as a warning sign that you need to double-check the data.
- And lastly, these descriptive statistics help inform which inferential statistical techniques you can use, as those techniques depend on the skewness (in other words, the symmetry and normality) of the data.
Simply put, descriptive statistics are really important , even though the statistical techniques used are fairly basic. All too often at Grad Coach, we see students skimming over the descriptives in their eagerness to get to the more exciting inferential methods, and then landing up with some very flawed results.
Don’t be a sucker – give your descriptive statistics the love and attention they deserve!
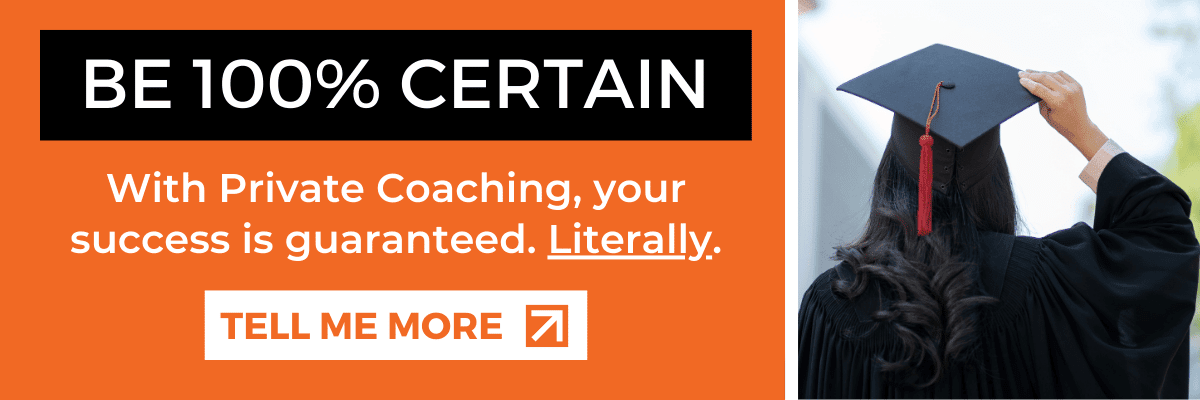
Branch 2: Inferential Statistics
As I mentioned, while descriptive statistics are all about the details of your specific data set – your sample – inferential statistics aim to make inferences about the population . In other words, you’ll use inferential statistics to make predictions about what you’d expect to find in the full population.
What kind of predictions, you ask? Well, there are two common types of predictions that researchers try to make using inferential stats:
- Firstly, predictions about differences between groups – for example, height differences between children grouped by their favourite meal or gender.
- And secondly, relationships between variables – for example, the relationship between body weight and the number of hours a week a person does yoga.
In other words, inferential statistics (when done correctly), allow you to connect the dots and make predictions about what you expect to see in the real world population, based on what you observe in your sample data. For this reason, inferential statistics are used for hypothesis testing – in other words, to test hypotheses that predict changes or differences.
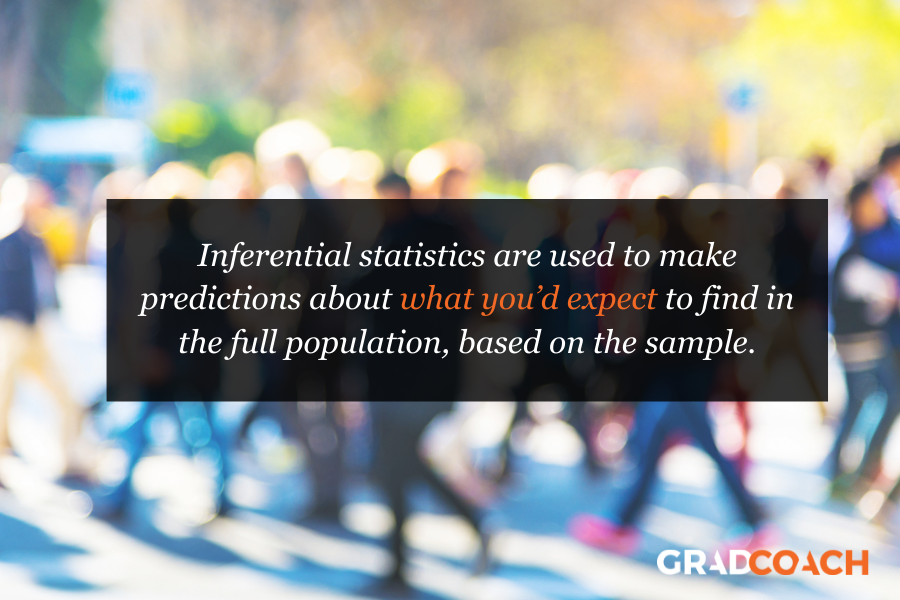
Of course, when you’re working with inferential statistics, the composition of your sample is really important. In other words, if your sample doesn’t accurately represent the population you’re researching, then your findings won’t necessarily be very useful.
For example, if your population of interest is a mix of 50% male and 50% female , but your sample is 80% male , you can’t make inferences about the population based on your sample, since it’s not representative. This area of statistics is called sampling, but we won’t go down that rabbit hole here (it’s a deep one!) – we’ll save that for another post .
What statistics are usually used in this branch?
There are many, many different statistical analysis methods within the inferential branch and it’d be impossible for us to discuss them all here. So we’ll just take a look at some of the most common inferential statistical methods so that you have a solid starting point.
First up are T-Tests . T-tests compare the means (the averages) of two groups of data to assess whether they’re statistically significantly different. In other words, do they have significantly different means, standard deviations and skewness.
This type of testing is very useful for understanding just how similar or different two groups of data are. For example, you might want to compare the mean blood pressure between two groups of people – one that has taken a new medication and one that hasn’t – to assess whether they are significantly different.
Kicking things up a level, we have ANOVA, which stands for “analysis of variance”. This test is similar to a T-test in that it compares the means of various groups, but ANOVA allows you to analyse multiple groups , not just two groups So it’s basically a t-test on steroids…
Next, we have correlation analysis . This type of analysis assesses the relationship between two variables. In other words, if one variable increases, does the other variable also increase, decrease or stay the same. For example, if the average temperature goes up, do average ice creams sales increase too? We’d expect some sort of relationship between these two variables intuitively , but correlation analysis allows us to measure that relationship scientifically .
Lastly, we have regression analysis – this is quite similar to correlation in that it assesses the relationship between variables, but it goes a step further to understand cause and effect between variables, not just whether they move together. In other words, does the one variable actually cause the other one to move, or do they just happen to move together naturally thanks to another force? Just because two variables correlate doesn’t necessarily mean that one causes the other.
Stats overload…
I hear you. To make this all a little more tangible, let’s take a look at an example of a correlation in action.
Here’s a scatter plot demonstrating the correlation (relationship) between weight and height. Intuitively, we’d expect there to be some relationship between these two variables, which is what we see in this scatter plot. In other words, the results tend to cluster together in a diagonal line from bottom left to top right.
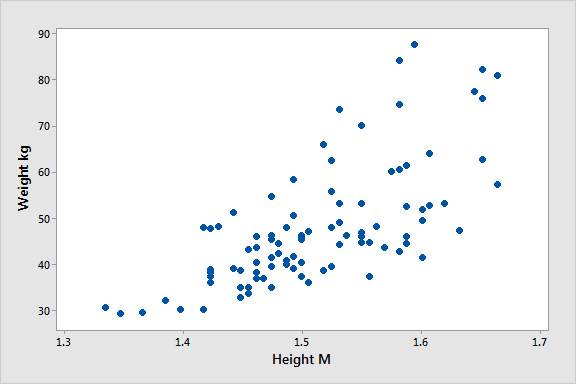
As I mentioned, these are are just a handful of inferential techniques – there are many, many more. Importantly, each statistical method has its own assumptions and limitations .
For example, some methods only work with normally distributed (parametric) data, while other methods are designed specifically for non-parametric data. And that’s exactly why descriptive statistics are so important – they’re the first step to knowing which inferential techniques you can and can’t use.
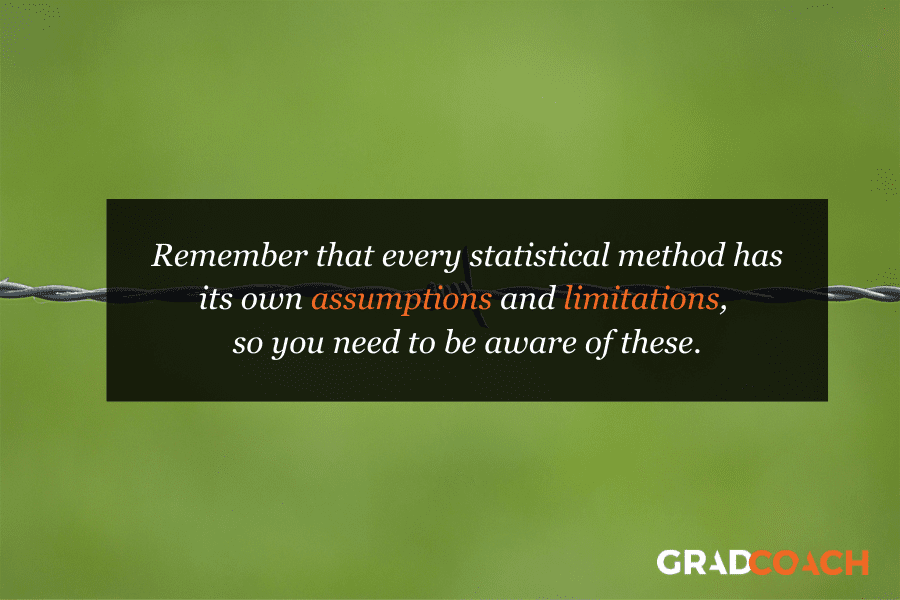
How to choose the right analysis method
To choose the right statistical methods, you need to think about two important factors :
- The type of quantitative data you have (specifically, level of measurement and the shape of the data). And,
- Your research questions and hypotheses
Let’s take a closer look at each of these.
Factor 1 – Data type
The first thing you need to consider is the type of data you’ve collected (or the type of data you will collect). By data types, I’m referring to the four levels of measurement – namely, nominal, ordinal, interval and ratio. If you’re not familiar with this lingo, check out the video below.
Why does this matter?
Well, because different statistical methods and techniques require different types of data. This is one of the “assumptions” I mentioned earlier – every method has its assumptions regarding the type of data.
For example, some techniques work with categorical data (for example, yes/no type questions, or gender or ethnicity), while others work with continuous numerical data (for example, age, weight or income) – and, of course, some work with multiple data types.
If you try to use a statistical method that doesn’t support the data type you have, your results will be largely meaningless . So, make sure that you have a clear understanding of what types of data you’ve collected (or will collect). Once you have this, you can then check which statistical methods would support your data types here .
If you haven’t collected your data yet, you can work in reverse and look at which statistical method would give you the most useful insights, and then design your data collection strategy to collect the correct data types.
Another important factor to consider is the shape of your data . Specifically, does it have a normal distribution (in other words, is it a bell-shaped curve, centred in the middle) or is it very skewed to the left or the right? Again, different statistical techniques work for different shapes of data – some are designed for symmetrical data while others are designed for skewed data.
This is another reminder of why descriptive statistics are so important – they tell you all about the shape of your data.
Factor 2: Your research questions
The next thing you need to consider is your specific research questions, as well as your hypotheses (if you have some). The nature of your research questions and research hypotheses will heavily influence which statistical methods and techniques you should use.
If you’re just interested in understanding the attributes of your sample (as opposed to the entire population), then descriptive statistics are probably all you need. For example, if you just want to assess the means (averages) and medians (centre points) of variables in a group of people.
On the other hand, if you aim to understand differences between groups or relationships between variables and to infer or predict outcomes in the population, then you’ll likely need both descriptive statistics and inferential statistics.
So, it’s really important to get very clear about your research aims and research questions, as well your hypotheses – before you start looking at which statistical techniques to use.
Never shoehorn a specific statistical technique into your research just because you like it or have some experience with it. Your choice of methods must align with all the factors we’ve covered here.
Time to recap…
You’re still with me? That’s impressive. We’ve covered a lot of ground here, so let’s recap on the key points:
- Quantitative data analysis is all about analysing number-based data (which includes categorical and numerical data) using various statistical techniques.
- The two main branches of statistics are descriptive statistics and inferential statistics . Descriptives describe your sample, whereas inferentials make predictions about what you’ll find in the population.
- Common descriptive statistical methods include mean (average), median , standard deviation and skewness .
- Common inferential statistical methods include t-tests , ANOVA , correlation and regression analysis.
- To choose the right statistical methods and techniques, you need to consider the type of data you’re working with , as well as your research questions and hypotheses.
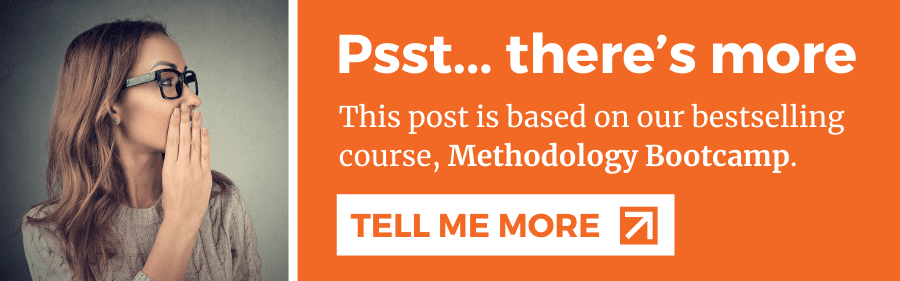
77 Comments
Hi, I have read your article. Such a brilliant post you have created.
Thank you for the feedback. Good luck with your quantitative analysis.
Thank you so much.
Thank you so much. I learnt much well. I love your summaries of the concepts. I had love you to explain how to input data using SPSS
Very useful, I have got the concept
Amazing and simple way of breaking down quantitative methods.
This is beautiful….especially for non-statisticians. I have skimmed through but I wish to read again. and please include me in other articles of the same nature when you do post. I am interested. I am sure, I could easily learn from you and get off the fear that I have had in the past. Thank you sincerely.
Send me every new information you might have.
i need every new information
Thank you for the blog. It is quite informative. Dr Peter Nemaenzhe PhD
It is wonderful. l’ve understood some of the concepts in a more compréhensive manner
Your article is so good! However, I am still a bit lost. I am doing a secondary research on Gun control in the US and increase in crime rates and I am not sure which analysis method I should use?
Based on the given learning points, this is inferential analysis, thus, use ‘t-tests, ANOVA, correlation and regression analysis’
Well explained notes. Am an MPH student and currently working on my thesis proposal, this has really helped me understand some of the things I didn’t know.
I like your page..helpful
wonderful i got my concept crystal clear. thankyou!!
This is really helpful , thank you
Thank you so much this helped
Wonderfully explained
thank u so much, it was so informative
THANKYOU, this was very informative and very helpful
This is great GRADACOACH I am not a statistician but I require more of this in my thesis
Include me in your posts.
This is so great and fully useful. I would like to thank you again and again.
Glad to read this article. I’ve read lot of articles but this article is clear on all concepts. Thanks for sharing.
Thank you so much. This is a very good foundation and intro into quantitative data analysis. Appreciate!
You have a very impressive, simple but concise explanation of data analysis for Quantitative Research here. This is a God-send link for me to appreciate research more. Thank you so much!
Avery good presentation followed by the write up. yes you simplified statistics to make sense even to a layman like me. Thank so much keep it up. The presenter did ell too. i would like more of this for Qualitative and exhaust more of the test example like the Anova.
This is a very helpful article, couldn’t have been clearer. Thank you.
Awesome and phenomenal information.Well done
The video with the accompanying article is super helpful to demystify this topic. Very well done. Thank you so much.
thank you so much, your presentation helped me a lot
I don’t know how should I express that ur article is saviour for me 🥺😍
It is well defined information and thanks for sharing. It helps me a lot in understanding the statistical data.
I gain a lot and thanks for sharing brilliant ideas, so wish to be linked on your email update.
Very helpful and clear .Thank you Gradcoach.
Thank for sharing this article, well organized and information presented are very clear.
VERY INTERESTING AND SUPPORTIVE TO NEW RESEARCHERS LIKE ME. AT LEAST SOME BASICS ABOUT QUANTITATIVE.
An outstanding, well explained and helpful article. This will help me so much with my data analysis for my research project. Thank you!
wow this has just simplified everything i was scared of how i am gonna analyse my data but thanks to you i will be able to do so
simple and constant direction to research. thanks
This is helpful
Great writing!! Comprehensive and very helpful.
Do you provide any assistance for other steps of research methodology like making research problem testing hypothesis report and thesis writing?
Thank you so much for such useful article!
Amazing article. So nicely explained. Wow
Very insightfull. Thanks
I am doing a quality improvement project to determine if the implementation of a protocol will change prescribing habits. Would this be a t-test?
The is a very helpful blog, however, I’m still not sure how to analyze my data collected. I’m doing a research on “Free Education at the University of Guyana”
tnx. fruitful blog!
So I am writing exams and would like to know how do establish which method of data analysis to use from the below research questions: I am a bit lost as to how I determine the data analysis method from the research questions.
Do female employees report higher job satisfaction than male employees with similar job descriptions across the South African telecommunications sector? – I though that maybe Chi Square could be used here. – Is there a gender difference in talented employees’ actual turnover decisions across the South African telecommunications sector? T-tests or Correlation in this one. – Is there a gender difference in the cost of actual turnover decisions across the South African telecommunications sector? T-tests or Correlation in this one. – What practical recommendations can be made to the management of South African telecommunications companies on leveraging gender to mitigate employee turnover decisions?
Your assistance will be appreciated if I could get a response as early as possible tomorrow
This was quite helpful. Thank you so much.
wow I got a lot from this article, thank you very much, keep it up
Thanks for yhe guidance. Can you send me this guidance on my email? To enable offline reading?
Thank you very much, this service is very helpful.
Every novice researcher needs to read this article as it puts things so clear and easy to follow. Its been very helpful.
Wonderful!!!! you explained everything in a way that anyone can learn. Thank you!!
I really enjoyed reading though this. Very easy to follow. Thank you
Many thanks for your useful lecture, I would be really appreciated if you could possibly share with me the PPT of presentation related to Data type?
Thank you very much for sharing, I got much from this article
This is a very informative write-up. Kindly include me in your latest posts.
Very interesting mostly for social scientists
Thank you so much, very helpfull
You’re welcome 🙂
woow, its great, its very informative and well understood because of your way of writing like teaching in front of me in simple languages.
I have been struggling to understand a lot of these concepts. Thank you for the informative piece which is written with outstanding clarity.
very informative article. Easy to understand
Beautiful read, much needed.
Always greet intro and summary. I learn so much from GradCoach
Quite informative. Simple and clear summary.
I thoroughly enjoyed reading your informative and inspiring piece. Your profound insights into this topic truly provide a better understanding of its complexity. I agree with the points you raised, especially when you delved into the specifics of the article. In my opinion, that aspect is often overlooked and deserves further attention.
Absolutely!!! Thank you
Thank you very much for this post. It made me to understand how to do my data analysis.
its nice work and excellent job ,you have made my work easier
Wow! So explicit. Well done.
Submit a Comment Cancel reply
Your email address will not be published. Required fields are marked *
Save my name, email, and website in this browser for the next time I comment.
- Print Friendly
- Privacy Policy

Home » Data Analysis – Process, Methods and Types
Data Analysis – Process, Methods and Types
Table of Contents

Data Analysis
Definition:
Data analysis refers to the process of inspecting, cleaning, transforming, and modeling data with the goal of discovering useful information, drawing conclusions, and supporting decision-making. It involves applying various statistical and computational techniques to interpret and derive insights from large datasets. The ultimate aim of data analysis is to convert raw data into actionable insights that can inform business decisions, scientific research, and other endeavors.
Data Analysis Process
The following are step-by-step guides to the data analysis process:
Define the Problem
The first step in data analysis is to clearly define the problem or question that needs to be answered. This involves identifying the purpose of the analysis, the data required, and the intended outcome.
Collect the Data
The next step is to collect the relevant data from various sources. This may involve collecting data from surveys, databases, or other sources. It is important to ensure that the data collected is accurate, complete, and relevant to the problem being analyzed.
Clean and Organize the Data
Once the data has been collected, it needs to be cleaned and organized. This involves removing any errors or inconsistencies in the data, filling in missing values, and ensuring that the data is in a format that can be easily analyzed.
Analyze the Data
The next step is to analyze the data using various statistical and analytical techniques. This may involve identifying patterns in the data, conducting statistical tests, or using machine learning algorithms to identify trends and insights.
Interpret the Results
After analyzing the data, the next step is to interpret the results. This involves drawing conclusions based on the analysis and identifying any significant findings or trends.
Communicate the Findings
Once the results have been interpreted, they need to be communicated to stakeholders. This may involve creating reports, visualizations, or presentations to effectively communicate the findings and recommendations.
Take Action
The final step in the data analysis process is to take action based on the findings. This may involve implementing new policies or procedures, making strategic decisions, or taking other actions based on the insights gained from the analysis.
Types of Data Analysis
Types of Data Analysis are as follows:
Descriptive Analysis
This type of analysis involves summarizing and describing the main characteristics of a dataset, such as the mean, median, mode, standard deviation, and range.
Inferential Analysis
This type of analysis involves making inferences about a population based on a sample. Inferential analysis can help determine whether a certain relationship or pattern observed in a sample is likely to be present in the entire population.
Diagnostic Analysis
This type of analysis involves identifying and diagnosing problems or issues within a dataset. Diagnostic analysis can help identify outliers, errors, missing data, or other anomalies in the dataset.
Predictive Analysis
This type of analysis involves using statistical models and algorithms to predict future outcomes or trends based on historical data. Predictive analysis can help businesses and organizations make informed decisions about the future.
Prescriptive Analysis
This type of analysis involves recommending a course of action based on the results of previous analyses. Prescriptive analysis can help organizations make data-driven decisions about how to optimize their operations, products, or services.
Exploratory Analysis
This type of analysis involves exploring the relationships and patterns within a dataset to identify new insights and trends. Exploratory analysis is often used in the early stages of research or data analysis to generate hypotheses and identify areas for further investigation.
Data Analysis Methods
Data Analysis Methods are as follows:
Statistical Analysis
This method involves the use of mathematical models and statistical tools to analyze and interpret data. It includes measures of central tendency, correlation analysis, regression analysis, hypothesis testing, and more.
Machine Learning
This method involves the use of algorithms to identify patterns and relationships in data. It includes supervised and unsupervised learning, classification, clustering, and predictive modeling.
Data Mining
This method involves using statistical and machine learning techniques to extract information and insights from large and complex datasets.
Text Analysis
This method involves using natural language processing (NLP) techniques to analyze and interpret text data. It includes sentiment analysis, topic modeling, and entity recognition.
Network Analysis
This method involves analyzing the relationships and connections between entities in a network, such as social networks or computer networks. It includes social network analysis and graph theory.
Time Series Analysis
This method involves analyzing data collected over time to identify patterns and trends. It includes forecasting, decomposition, and smoothing techniques.
Spatial Analysis
This method involves analyzing geographic data to identify spatial patterns and relationships. It includes spatial statistics, spatial regression, and geospatial data visualization.
Data Visualization
This method involves using graphs, charts, and other visual representations to help communicate the findings of the analysis. It includes scatter plots, bar charts, heat maps, and interactive dashboards.
Qualitative Analysis
This method involves analyzing non-numeric data such as interviews, observations, and open-ended survey responses. It includes thematic analysis, content analysis, and grounded theory.
Multi-criteria Decision Analysis
This method involves analyzing multiple criteria and objectives to support decision-making. It includes techniques such as the analytical hierarchy process, TOPSIS, and ELECTRE.
Data Analysis Tools
There are various data analysis tools available that can help with different aspects of data analysis. Below is a list of some commonly used data analysis tools:
- Microsoft Excel: A widely used spreadsheet program that allows for data organization, analysis, and visualization.
- SQL : A programming language used to manage and manipulate relational databases.
- R : An open-source programming language and software environment for statistical computing and graphics.
- Python : A general-purpose programming language that is widely used in data analysis and machine learning.
- Tableau : A data visualization software that allows for interactive and dynamic visualizations of data.
- SAS : A statistical analysis software used for data management, analysis, and reporting.
- SPSS : A statistical analysis software used for data analysis, reporting, and modeling.
- Matlab : A numerical computing software that is widely used in scientific research and engineering.
- RapidMiner : A data science platform that offers a wide range of data analysis and machine learning tools.
Applications of Data Analysis
Data analysis has numerous applications across various fields. Below are some examples of how data analysis is used in different fields:
- Business : Data analysis is used to gain insights into customer behavior, market trends, and financial performance. This includes customer segmentation, sales forecasting, and market research.
- Healthcare : Data analysis is used to identify patterns and trends in patient data, improve patient outcomes, and optimize healthcare operations. This includes clinical decision support, disease surveillance, and healthcare cost analysis.
- Education : Data analysis is used to measure student performance, evaluate teaching effectiveness, and improve educational programs. This includes assessment analytics, learning analytics, and program evaluation.
- Finance : Data analysis is used to monitor and evaluate financial performance, identify risks, and make investment decisions. This includes risk management, portfolio optimization, and fraud detection.
- Government : Data analysis is used to inform policy-making, improve public services, and enhance public safety. This includes crime analysis, disaster response planning, and social welfare program evaluation.
- Sports : Data analysis is used to gain insights into athlete performance, improve team strategy, and enhance fan engagement. This includes player evaluation, scouting analysis, and game strategy optimization.
- Marketing : Data analysis is used to measure the effectiveness of marketing campaigns, understand customer behavior, and develop targeted marketing strategies. This includes customer segmentation, marketing attribution analysis, and social media analytics.
- Environmental science : Data analysis is used to monitor and evaluate environmental conditions, assess the impact of human activities on the environment, and develop environmental policies. This includes climate modeling, ecological forecasting, and pollution monitoring.
When to Use Data Analysis
Data analysis is useful when you need to extract meaningful insights and information from large and complex datasets. It is a crucial step in the decision-making process, as it helps you understand the underlying patterns and relationships within the data, and identify potential areas for improvement or opportunities for growth.
Here are some specific scenarios where data analysis can be particularly helpful:
- Problem-solving : When you encounter a problem or challenge, data analysis can help you identify the root cause and develop effective solutions.
- Optimization : Data analysis can help you optimize processes, products, or services to increase efficiency, reduce costs, and improve overall performance.
- Prediction: Data analysis can help you make predictions about future trends or outcomes, which can inform strategic planning and decision-making.
- Performance evaluation : Data analysis can help you evaluate the performance of a process, product, or service to identify areas for improvement and potential opportunities for growth.
- Risk assessment : Data analysis can help you assess and mitigate risks, whether it is financial, operational, or related to safety.
- Market research : Data analysis can help you understand customer behavior and preferences, identify market trends, and develop effective marketing strategies.
- Quality control: Data analysis can help you ensure product quality and customer satisfaction by identifying and addressing quality issues.
Purpose of Data Analysis
The primary purposes of data analysis can be summarized as follows:
- To gain insights: Data analysis allows you to identify patterns and trends in data, which can provide valuable insights into the underlying factors that influence a particular phenomenon or process.
- To inform decision-making: Data analysis can help you make informed decisions based on the information that is available. By analyzing data, you can identify potential risks, opportunities, and solutions to problems.
- To improve performance: Data analysis can help you optimize processes, products, or services by identifying areas for improvement and potential opportunities for growth.
- To measure progress: Data analysis can help you measure progress towards a specific goal or objective, allowing you to track performance over time and adjust your strategies accordingly.
- To identify new opportunities: Data analysis can help you identify new opportunities for growth and innovation by identifying patterns and trends that may not have been visible before.
Examples of Data Analysis
Some Examples of Data Analysis are as follows:
- Social Media Monitoring: Companies use data analysis to monitor social media activity in real-time to understand their brand reputation, identify potential customer issues, and track competitors. By analyzing social media data, businesses can make informed decisions on product development, marketing strategies, and customer service.
- Financial Trading: Financial traders use data analysis to make real-time decisions about buying and selling stocks, bonds, and other financial instruments. By analyzing real-time market data, traders can identify trends and patterns that help them make informed investment decisions.
- Traffic Monitoring : Cities use data analysis to monitor traffic patterns and make real-time decisions about traffic management. By analyzing data from traffic cameras, sensors, and other sources, cities can identify congestion hotspots and make changes to improve traffic flow.
- Healthcare Monitoring: Healthcare providers use data analysis to monitor patient health in real-time. By analyzing data from wearable devices, electronic health records, and other sources, healthcare providers can identify potential health issues and provide timely interventions.
- Online Advertising: Online advertisers use data analysis to make real-time decisions about advertising campaigns. By analyzing data on user behavior and ad performance, advertisers can make adjustments to their campaigns to improve their effectiveness.
- Sports Analysis : Sports teams use data analysis to make real-time decisions about strategy and player performance. By analyzing data on player movement, ball position, and other variables, coaches can make informed decisions about substitutions, game strategy, and training regimens.
- Energy Management : Energy companies use data analysis to monitor energy consumption in real-time. By analyzing data on energy usage patterns, companies can identify opportunities to reduce energy consumption and improve efficiency.
Characteristics of Data Analysis
Characteristics of Data Analysis are as follows:
- Objective : Data analysis should be objective and based on empirical evidence, rather than subjective assumptions or opinions.
- Systematic : Data analysis should follow a systematic approach, using established methods and procedures for collecting, cleaning, and analyzing data.
- Accurate : Data analysis should produce accurate results, free from errors and bias. Data should be validated and verified to ensure its quality.
- Relevant : Data analysis should be relevant to the research question or problem being addressed. It should focus on the data that is most useful for answering the research question or solving the problem.
- Comprehensive : Data analysis should be comprehensive and consider all relevant factors that may affect the research question or problem.
- Timely : Data analysis should be conducted in a timely manner, so that the results are available when they are needed.
- Reproducible : Data analysis should be reproducible, meaning that other researchers should be able to replicate the analysis using the same data and methods.
- Communicable : Data analysis should be communicated clearly and effectively to stakeholders and other interested parties. The results should be presented in a way that is understandable and useful for decision-making.
Advantages of Data Analysis
Advantages of Data Analysis are as follows:
- Better decision-making: Data analysis helps in making informed decisions based on facts and evidence, rather than intuition or guesswork.
- Improved efficiency: Data analysis can identify inefficiencies and bottlenecks in business processes, allowing organizations to optimize their operations and reduce costs.
- Increased accuracy: Data analysis helps to reduce errors and bias, providing more accurate and reliable information.
- Better customer service: Data analysis can help organizations understand their customers better, allowing them to provide better customer service and improve customer satisfaction.
- Competitive advantage: Data analysis can provide organizations with insights into their competitors, allowing them to identify areas where they can gain a competitive advantage.
- Identification of trends and patterns : Data analysis can identify trends and patterns in data that may not be immediately apparent, helping organizations to make predictions and plan for the future.
- Improved risk management : Data analysis can help organizations identify potential risks and take proactive steps to mitigate them.
- Innovation: Data analysis can inspire innovation and new ideas by revealing new opportunities or previously unknown correlations in data.
Limitations of Data Analysis
- Data quality: The quality of data can impact the accuracy and reliability of analysis results. If data is incomplete, inconsistent, or outdated, the analysis may not provide meaningful insights.
- Limited scope: Data analysis is limited by the scope of the data available. If data is incomplete or does not capture all relevant factors, the analysis may not provide a complete picture.
- Human error : Data analysis is often conducted by humans, and errors can occur in data collection, cleaning, and analysis.
- Cost : Data analysis can be expensive, requiring specialized tools, software, and expertise.
- Time-consuming : Data analysis can be time-consuming, especially when working with large datasets or conducting complex analyses.
- Overreliance on data: Data analysis should be complemented with human intuition and expertise. Overreliance on data can lead to a lack of creativity and innovation.
- Privacy concerns: Data analysis can raise privacy concerns if personal or sensitive information is used without proper consent or security measures.
About the author
Muhammad Hassan
Researcher, Academic Writer, Web developer
You may also like

Discourse Analysis – Methods, Types and Examples

Research Techniques – Methods, Types and Examples

Research Results Section – Writing Guide and...

References in Research – Types, Examples and...

Chapter Summary & Overview – Writing Guide...

Documentary Analysis – Methods, Applications and...
8 Types of Data Analysis
The different types of data analysis include descriptive, diagnostic, exploratory, inferential, predictive, causal, mechanistic and prescriptive. Here’s what you need to know about each one.

Data analysis is an aspect of data science and data analytics that is all about analyzing data for different kinds of purposes. The data analysis process involves inspecting, cleaning, transforming and modeling data to draw useful insights from it.
Types of Data Analysis
- Descriptive analysis
- Diagnostic analysis
- Exploratory analysis
- Inferential analysis
- Predictive analysis
- Causal analysis
- Mechanistic analysis
- Prescriptive analysis
With its multiple facets, methodologies and techniques, data analysis is used in a variety of fields, including energy, healthcare and marketing, among others. As businesses thrive under the influence of technological advancements in data analytics, data analysis plays a huge role in decision-making , providing a better, faster and more effective system that minimizes risks and reduces human biases .
That said, there are different kinds of data analysis with different goals. We’ll examine each one below.
Two Camps of Data Analysis
Data analysis can be divided into two camps, according to the book R for Data Science :
- Hypothesis Generation: This involves looking deeply at the data and combining your domain knowledge to generate hypotheses about why the data behaves the way it does.
- Hypothesis Confirmation: This involves using a precise mathematical model to generate falsifiable predictions with statistical sophistication to confirm your prior hypotheses.
More on Data Analysis: Data Analyst vs. Data Scientist: Similarities and Differences Explained
Data analysis can be separated and organized into types, arranged in an increasing order of complexity.
1. Descriptive Analysis
The goal of descriptive analysis is to describe or summarize a set of data . Here’s what you need to know:
- Descriptive analysis is the very first analysis performed in the data analysis process.
- It generates simple summaries of samples and measurements.
- It involves common, descriptive statistics like measures of central tendency, variability, frequency and position.
Descriptive Analysis Example
Take the Covid-19 statistics page on Google, for example. The line graph is a pure summary of the cases/deaths, a presentation and description of the population of a particular country infected by the virus.
Descriptive analysis is the first step in analysis where you summarize and describe the data you have using descriptive statistics, and the result is a simple presentation of your data.
2. Diagnostic Analysis
Diagnostic analysis seeks to answer the question “Why did this happen?” by taking a more in-depth look at data to uncover subtle patterns. Here’s what you need to know:
- Diagnostic analysis typically comes after descriptive analysis, taking initial findings and investigating why certain patterns in data happen.
- Diagnostic analysis may involve analyzing other related data sources, including past data, to reveal more insights into current data trends.
- Diagnostic analysis is ideal for further exploring patterns in data to explain anomalies .
Diagnostic Analysis Example
A footwear store wants to review its website traffic levels over the previous 12 months. Upon compiling and assessing the data, the company’s marketing team finds that June experienced above-average levels of traffic while July and August witnessed slightly lower levels of traffic.
To find out why this difference occurred, the marketing team takes a deeper look. Team members break down the data to focus on specific categories of footwear. In the month of June, they discovered that pages featuring sandals and other beach-related footwear received a high number of views while these numbers dropped in July and August.
Marketers may also review other factors like seasonal changes and company sales events to see if other variables could have contributed to this trend.
3. Exploratory Analysis (EDA)
Exploratory analysis involves examining or exploring data and finding relationships between variables that were previously unknown. Here’s what you need to know:
- EDA helps you discover relationships between measures in your data, which are not evidence for the existence of the correlation, as denoted by the phrase, “ Correlation doesn’t imply causation .”
- It’s useful for discovering new connections and forming hypotheses. It drives design planning and data collection .
Exploratory Analysis Example
Climate change is an increasingly important topic as the global temperature has gradually risen over the years. One example of an exploratory data analysis on climate change involves taking the rise in temperature over the years from 1950 to 2020 and the increase of human activities and industrialization to find relationships from the data. For example, you may increase the number of factories, cars on the road and airplane flights to see how that correlates with the rise in temperature.
Exploratory analysis explores data to find relationships between measures without identifying the cause. It’s most useful when formulating hypotheses.
4. Inferential Analysis
Inferential analysis involves using a small sample of data to infer information about a larger population of data.
The goal of statistical modeling itself is all about using a small amount of information to extrapolate and generalize information to a larger group. Here’s what you need to know:
- Inferential analysis involves using estimated data that is representative of a population and gives a measure of uncertainty or standard deviation to your estimation.
- The accuracy of inference depends heavily on your sampling scheme. If the sample isn’t representative of the population, the generalization will be inaccurate. This is known as the central limit theorem .

Inferential Analysis Example
A psychological study on the benefits of sleep might have a total of 500 people involved. When they followed up with the candidates, the candidates reported to have better overall attention spans and well-being with seven to nine hours of sleep, while those with less sleep and more sleep than the given range suffered from reduced attention spans and energy. This study drawn from 500 people was just a tiny portion of the 7 billion people in the world, and is thus an inference of the larger population.
Inferential analysis extrapolates and generalizes the information of the larger group with a smaller sample to generate analysis and predictions.
5. Predictive Analysis
Predictive analysis involves using historical or current data to find patterns and make predictions about the future. Here’s what you need to know:
- The accuracy of the predictions depends on the input variables.
- Accuracy also depends on the types of models. A linear model might work well in some cases, and in other cases it might not.
- Using a variable to predict another one doesn’t denote a causal relationship.
Predictive Analysis Example
The 2020 United States election is a popular topic and many prediction models are built to predict the winning candidate. FiveThirtyEight did this to forecast the 2016 and 2020 elections. Prediction analysis for an election would require input variables such as historical polling data, trends and current polling data in order to return a good prediction. Something as large as an election wouldn’t just be using a linear model, but a complex model with certain tunings to best serve its purpose.
6. Causal Analysis
Causal analysis looks at the cause and effect of relationships between variables and is focused on finding the cause of a correlation. This way, researchers can examine how a change in one variable affects another. Here’s what you need to know:
- To find the cause, you have to question whether the observed correlations driving your conclusion are valid. Just looking at the surface data won’t help you discover the hidden mechanisms underlying the correlations.
- Causal analysis is applied in randomized studies focused on identifying causation.
- Causal analysis is the gold standard in data analysis and scientific studies where the cause of a phenomenon is to be extracted and singled out, like separating wheat from chaff.
- Good data is hard to find and requires expensive research and studies. These studies are analyzed in aggregate (multiple groups), and the observed relationships are just average effects (mean) of the whole population. This means the results might not apply to everyone.
Causal Analysis Example
Say you want to test out whether a new drug improves human strength and focus. To do that, you perform randomized control trials for the drug to test its effect. You compare the sample of candidates for your new drug against the candidates receiving a mock control drug through a few tests focused on strength and overall focus and attention. This will allow you to observe how the drug affects the outcome.
7. Mechanistic Analysis
Mechanistic analysis is used to understand exact changes in variables that lead to other changes in other variables . In some ways, it is a predictive analysis, but it’s modified to tackle studies that require high precision and meticulous methodologies for physical or engineering science. Here’s what you need to know:
- It’s applied in physical or engineering sciences, situations that require high precision and little room for error, only noise in data is measurement error.
- It’s designed to understand a biological or behavioral process, the pathophysiology of a disease or the mechanism of action of an intervention.
Mechanistic Analysis Example
Say an experiment is done to simulate safe and effective nuclear fusion to power the world. A mechanistic analysis of the study would entail a precise balance of controlling and manipulating variables with highly accurate measures of both variables and the desired outcomes. It’s this intricate and meticulous modus operandi toward these big topics that allows for scientific breakthroughs and advancement of society.
8. Prescriptive Analysis
Prescriptive analysis compiles insights from other previous data analyses and determines actions that teams or companies can take to prepare for predicted trends. Here’s what you need to know:
- Prescriptive analysis may come right after predictive analysis, but it may involve combining many different data analyses.
- Companies need advanced technology and plenty of resources to conduct prescriptive analysis. Artificial intelligence systems that process data and adjust automated tasks are an example of the technology required to perform prescriptive analysis.
Prescriptive Analysis Example
Prescriptive analysis is pervasive in everyday life, driving the curated content users consume on social media. On platforms like TikTok and Instagram, algorithms can apply prescriptive analysis to review past content a user has engaged with and the kinds of behaviors they exhibited with specific posts. Based on these factors, an algorithm seeks out similar content that is likely to elicit the same response and recommends it on a user’s personal feed.
More on Data Explaining the Empirical Rule for Normal Distribution
When to Use the Different Types of Data Analysis
- Descriptive analysis summarizes the data at hand and presents your data in a comprehensible way.
- Diagnostic analysis takes a more detailed look at data to reveal why certain patterns occur, making it a good method for explaining anomalies.
- Exploratory data analysis helps you discover correlations and relationships between variables in your data.
- Inferential analysis is for generalizing the larger population with a smaller sample size of data.
- Predictive analysis helps you make predictions about the future with data.
- Causal analysis emphasizes finding the cause of a correlation between variables.
- Mechanistic analysis is for measuring the exact changes in variables that lead to other changes in other variables.
- Prescriptive analysis combines insights from different data analyses to develop a course of action teams and companies can take to capitalize on predicted outcomes.
A few important tips to remember about data analysis include:
- Correlation doesn’t imply causation.
- EDA helps discover new connections and form hypotheses.
- Accuracy of inference depends on the sampling scheme.
- A good prediction depends on the right input variables.
- A simple linear model with enough data usually does the trick.
- Using a variable to predict another doesn’t denote causal relationships.
- Good data is hard to find, and to produce it requires expensive research.
- Results from studies are done in aggregate and are average effects and might not apply to everyone.
Frequently Asked Questions
What is an example of data analysis.
A marketing team reviews a company’s web traffic over the past 12 months. To understand why sales rise and fall during certain months, the team breaks down the data to look at shoe type, seasonal patterns and sales events. Based on this in-depth analysis, the team can determine variables that influenced web traffic and make adjustments as needed.
How do you know which data analysis method to use?
Selecting a data analysis method depends on the goals of the analysis and the complexity of the task, among other factors. It’s best to assess the circumstances and consider the pros and cons of each type of data analysis before moving forward with a particular method.
Recent Data Science Articles
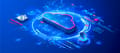
- Skip to main content
- Skip to primary sidebar
- Skip to footer
- QuestionPro

- Solutions Industries Gaming Automotive Sports and events Education Government Travel & Hospitality Financial Services Healthcare Cannabis Technology Use Case AskWhy Communities Audience Contactless surveys Mobile LivePolls Member Experience GDPR Positive People Science 360 Feedback Surveys
- Resources Blog eBooks Survey Templates Case Studies Training Help center

Home Market Research
Qualitative Data Analysis: What is it, Methods + Examples
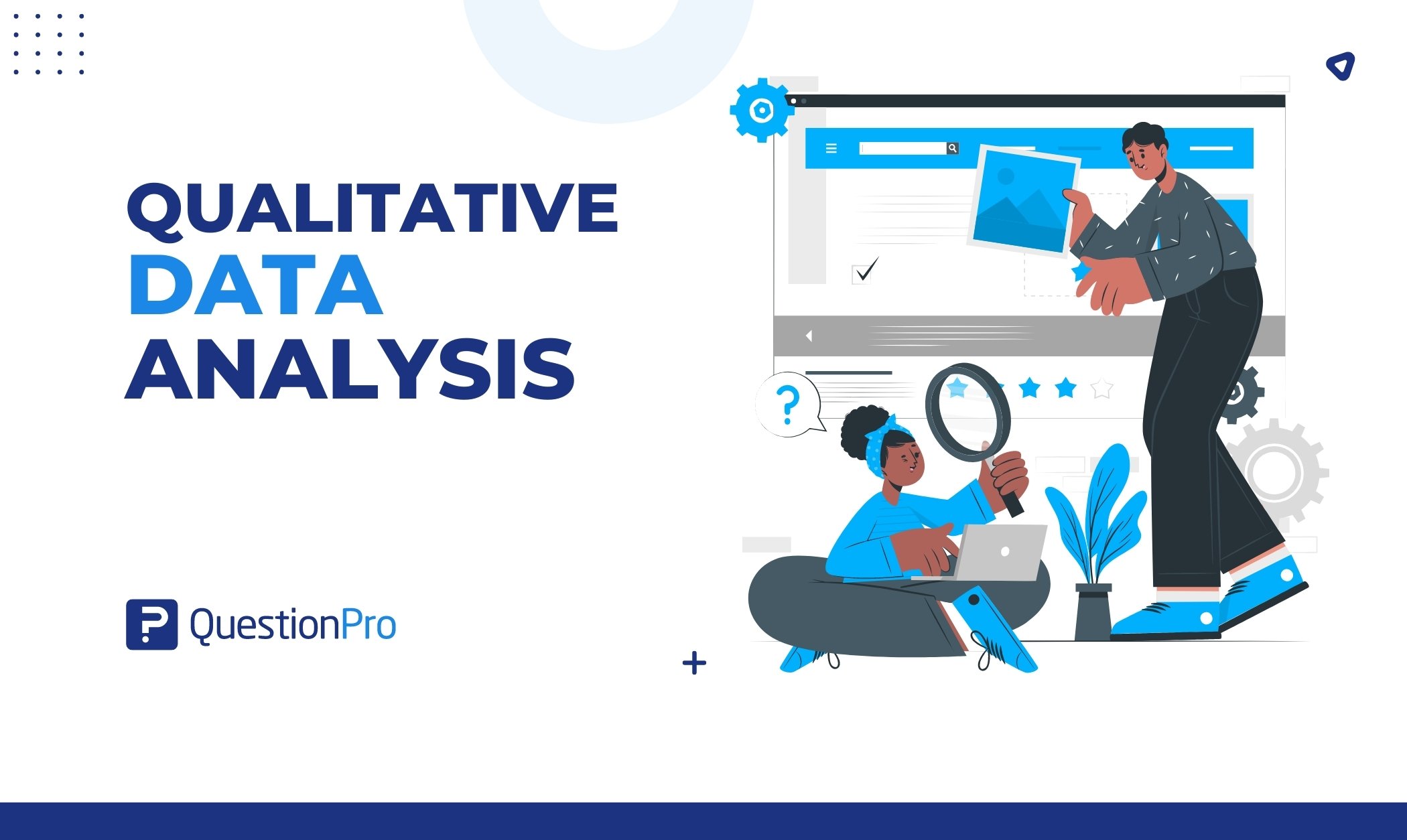
In a world rich with information and narrative, understanding the deeper layers of human experiences requires a unique vision that goes beyond numbers and figures. This is where the power of qualitative data analysis comes to light.
In this blog, we’ll learn about qualitative data analysis, explore its methods, and provide real-life examples showcasing its power in uncovering insights.
What is Qualitative Data Analysis?
Qualitative data analysis is a systematic process of examining non-numerical data to extract meaning, patterns, and insights.
In contrast to quantitative analysis, which focuses on numbers and statistical metrics, the qualitative study focuses on the qualitative aspects of data, such as text, images, audio, and videos. It seeks to understand every aspect of human experiences, perceptions, and behaviors by examining the data’s richness.
Companies frequently conduct this analysis on customer feedback. You can collect qualitative data from reviews, complaints, chat messages, interactions with support centers, customer interviews, case notes, or even social media comments. This kind of data holds the key to understanding customer sentiments and preferences in a way that goes beyond mere numbers.
Importance of Qualitative Data Analysis
Qualitative data analysis plays a crucial role in your research and decision-making process across various disciplines. Let’s explore some key reasons that underline the significance of this analysis:
In-Depth Understanding
It enables you to explore complex and nuanced aspects of a phenomenon, delving into the ‘how’ and ‘why’ questions. This method provides you with a deeper understanding of human behavior, experiences, and contexts that quantitative approaches might not capture fully.
Contextual Insight
You can use this analysis to give context to numerical data. It will help you understand the circumstances and conditions that influence participants’ thoughts, feelings, and actions. This contextual insight becomes essential for generating comprehensive explanations.
Theory Development
You can generate or refine hypotheses via qualitative data analysis. As you analyze the data attentively, you can form hypotheses, concepts, and frameworks that will drive your future research and contribute to theoretical advances.
Participant Perspectives
When performing qualitative research, you can highlight participant voices and opinions. This approach is especially useful for understanding marginalized or underrepresented people, as it allows them to communicate their experiences and points of view.
Exploratory Research
The analysis is frequently used at the exploratory stage of your project. It assists you in identifying important variables, developing research questions, and designing quantitative studies that will follow.
Types of Qualitative Data
When conducting qualitative research, you can use several qualitative data collection methods , and here you will come across many sorts of qualitative data that can provide you with unique insights into your study topic. These data kinds add new views and angles to your understanding and analysis.
Interviews and Focus Groups
Interviews and focus groups will be among your key methods for gathering qualitative data. Interviews are one-on-one talks in which participants can freely share their thoughts, experiences, and opinions.
Focus groups, on the other hand, are discussions in which members interact with one another, resulting in dynamic exchanges of ideas. Both methods provide rich qualitative data and direct access to participant perspectives.
Observations and Field Notes
Observations and field notes are another useful sort of qualitative data. You can immerse yourself in the research environment through direct observation, carefully documenting behaviors, interactions, and contextual factors.
These observations will be recorded in your field notes, providing a complete picture of the environment and the behaviors you’re researching. This data type is especially important for comprehending behavior in their natural setting.
Textual and Visual Data
Textual and visual data include a wide range of resources that can be qualitatively analyzed. Documents, written narratives, and transcripts from various sources, such as interviews or speeches, are examples of textual data.
Photographs, films, and even artwork provide a visual layer to your research. These forms of data allow you to investigate what is spoken and the underlying emotions, details, and symbols expressed by language or pictures.
When to Choose Qualitative Data Analysis over Quantitative Data Analysis
As you begin your research journey, understanding why the analysis of qualitative data is important will guide your approach to understanding complex events. If you analyze qualitative data, it will provide new insights that complement quantitative methodologies, which will give you a broader understanding of your study topic.
It is critical to know when to use qualitative analysis over quantitative procedures. You can prefer qualitative data analysis when:
- Complexity Reigns: When your research questions involve deep human experiences, motivations, or emotions, qualitative research excels at revealing these complexities.
- Exploration is Key: Qualitative analysis is ideal for exploratory research. It will assist you in understanding a new or poorly understood topic before formulating quantitative hypotheses.
- Context Matters: If you want to understand how context affects behaviors or results, qualitative data analysis provides the depth needed to grasp these relationships.
- Unanticipated Findings: When your study provides surprising new viewpoints or ideas, qualitative analysis helps you to delve deeply into these emerging themes.
- Subjective Interpretation is Vital: When it comes to understanding people’s subjective experiences and interpretations, qualitative data analysis is the way to go.
You can make informed decisions regarding the right approach for your research objectives if you understand the importance of qualitative analysis and recognize the situations where it shines.
Qualitative Data Analysis Methods and Examples
Exploring various qualitative data analysis methods will provide you with a wide collection for making sense of your research findings. Once the data has been collected, you can choose from several analysis methods based on your research objectives and the data type you’ve collected.
There are five main methods for analyzing qualitative data. Each method takes a distinct approach to identifying patterns, themes, and insights within your qualitative data. They are:
Method 1: Content Analysis
Content analysis is a methodical technique for analyzing textual or visual data in a structured manner. In this method, you will categorize qualitative data by splitting it into manageable pieces and assigning the manual coding process to these units.
As you go, you’ll notice ongoing codes and designs that will allow you to conclude the content. This method is very beneficial for detecting common ideas, concepts, or themes in your data without losing the context.
Steps to Do Content Analysis
Follow these steps when conducting content analysis:
- Collect and Immerse: Begin by collecting the necessary textual or visual data. Immerse yourself in this data to fully understand its content, context, and complexities.
- Assign Codes and Categories: Assign codes to relevant data sections that systematically represent major ideas or themes. Arrange comparable codes into groups that cover the major themes.
- Analyze and Interpret: Develop a structured framework from the categories and codes. Then, evaluate the data in the context of your research question, investigate relationships between categories, discover patterns, and draw meaning from these connections.
Benefits & Challenges
There are various advantages to using content analysis:
- Structured Approach: It offers a systematic approach to dealing with large data sets and ensures consistency throughout the research.
- Objective Insights: This method promotes objectivity, which helps to reduce potential biases in your study.
- Pattern Discovery: Content analysis can help uncover hidden trends, themes, and patterns that are not always obvious.
- Versatility: You can apply content analysis to various data formats, including text, internet content, images, etc.
However, keep in mind the challenges that arise:
- Subjectivity: Even with the best attempts, a certain bias may remain in coding and interpretation.
- Complexity: Analyzing huge data sets requires time and great attention to detail.
- Contextual Nuances: Content analysis may not capture all of the contextual richness that qualitative data analysis highlights.
Example of Content Analysis
Suppose you’re conducting market research and looking at customer feedback on a product. As you collect relevant data and analyze feedback, you’ll see repeating codes like “price,” “quality,” “customer service,” and “features.” These codes are organized into categories such as “positive reviews,” “negative reviews,” and “suggestions for improvement.”
According to your findings, themes such as “price” and “customer service” stand out and show that pricing and customer service greatly impact customer satisfaction. This example highlights the power of content analysis for obtaining significant insights from large textual data collections.
Method 2: Thematic Analysis
Thematic analysis is a well-structured procedure for identifying and analyzing recurring themes in your data. As you become more engaged in the data, you’ll generate codes or short labels representing key concepts. These codes are then organized into themes, providing a consistent framework for organizing and comprehending the substance of the data.
The analysis allows you to organize complex narratives and perspectives into meaningful categories, which will allow you to identify connections and patterns that may not be visible at first.
Steps to Do Thematic Analysis
Follow these steps when conducting a thematic analysis:
- Code and Group: Start by thoroughly examining the data and giving initial codes that identify the segments. To create initial themes, combine relevant codes.
- Code and Group: Begin by engaging yourself in the data, assigning first codes to notable segments. To construct basic themes, group comparable codes together.
- Analyze and Report: Analyze the data within each theme to derive relevant insights. Organize the topics into a consistent structure and explain your findings, along with data extracts that represent each theme.
Thematic analysis has various benefits:
- Structured Exploration: It is a method for identifying patterns and themes in complex qualitative data.
- Comprehensive knowledge: Thematic analysis promotes an in-depth understanding of the complications and meanings of the data.
- Application Flexibility: This method can be customized to various research situations and data kinds.
However, challenges may arise, such as:
- Interpretive Nature: Interpreting qualitative data in thematic analysis is vital, and it is critical to manage researcher bias.
- Time-consuming: The study can be time-consuming, especially with large data sets.
- Subjectivity: The selection of codes and topics might be subjective.
Example of Thematic Analysis
Assume you’re conducting a thematic analysis on job satisfaction interviews. Following your immersion in the data, you assign initial codes such as “work-life balance,” “career growth,” and “colleague relationships.” As you organize these codes, you’ll notice themes develop, such as “Factors Influencing Job Satisfaction” and “Impact on Work Engagement.”
Further investigation reveals the tales and experiences included within these themes and provides insights into how various elements influence job satisfaction. This example demonstrates how thematic analysis can reveal meaningful patterns and insights in qualitative data.
Method 3: Narrative Analysis
The narrative analysis involves the narratives that people share. You’ll investigate the histories in your data, looking at how stories are created and the meanings they express. This method is excellent for learning how people make sense of their experiences through narrative.
Steps to Do Narrative Analysis
The following steps are involved in narrative analysis:
- Gather and Analyze: Start by collecting narratives, such as first-person tales, interviews, or written accounts. Analyze the stories, focusing on the plot, feelings, and characters.
- Find Themes: Look for recurring themes or patterns in various narratives. Think about the similarities and differences between these topics and personal experiences.
- Interpret and Extract Insights: Contextualize the narratives within their larger context. Accept the subjective nature of each narrative and analyze the narrator’s voice and style. Extract insights from the tales by diving into the emotions, motivations, and implications communicated by the stories.
There are various advantages to narrative analysis:
- Deep Exploration: It lets you look deeply into people’s personal experiences and perspectives.
- Human-Centered: This method prioritizes the human perspective, allowing individuals to express themselves.
However, difficulties may arise, such as:
- Interpretive Complexity: Analyzing narratives requires dealing with the complexities of meaning and interpretation.
- Time-consuming: Because of the richness and complexities of tales, working with them can be time-consuming.
Example of Narrative Analysis
Assume you’re conducting narrative analysis on refugee interviews. As you read the stories, you’ll notice common themes of toughness, loss, and hope. The narratives provide insight into the obstacles that refugees face, their strengths, and the dreams that guide them.
The analysis can provide a deeper insight into the refugees’ experiences and the broader social context they navigate by examining the narratives’ emotional subtleties and underlying meanings. This example highlights how narrative analysis can reveal important insights into human stories.
Method 4: Grounded Theory Analysis
Grounded theory analysis is an iterative and systematic approach that allows you to create theories directly from data without being limited by pre-existing hypotheses. With an open mind, you collect data and generate early codes and labels that capture essential ideas or concepts within the data.
As you progress, you refine these codes and increasingly connect them, eventually developing a theory based on the data. Grounded theory analysis is a dynamic process for developing new insights and hypotheses based on details in your data.
Steps to Do Grounded Theory Analysis
Grounded theory analysis requires the following steps:
- Initial Coding: First, immerse yourself in the data, producing initial codes that represent major concepts or patterns.
- Categorize and Connect: Using axial coding, organize the initial codes, which establish relationships and connections between topics.
- Build the Theory: Focus on creating a core category that connects the codes and themes. Regularly refine the theory by comparing and integrating new data, ensuring that it evolves organically from the data.
Grounded theory analysis has various benefits:
- Theory Generation: It provides a one-of-a-kind opportunity to generate hypotheses straight from data and promotes new insights.
- In-depth Understanding: The analysis allows you to deeply analyze the data and reveal complex relationships and patterns.
- Flexible Process: This method is customizable and ongoing, which allows you to enhance your research as you collect additional data.
However, challenges might arise with:
- Time and Resources: Because grounded theory analysis is a continuous process, it requires a large commitment of time and resources.
- Theoretical Development: Creating a grounded theory involves a thorough understanding of qualitative data analysis software and theoretical concepts.
- Interpretation of Complexity: Interpreting and incorporating a newly developed theory into existing literature can be intellectually hard.
Example of Grounded Theory Analysis
Assume you’re performing a grounded theory analysis on workplace collaboration interviews. As you open code the data, you will discover notions such as “communication barriers,” “team dynamics,” and “leadership roles.” Axial coding demonstrates links between these notions, emphasizing the significance of efficient communication in developing collaboration.
You create the core “Integrated Communication Strategies” category through selective coding, which unifies new topics.
This theory-driven category serves as the framework for understanding how numerous aspects contribute to effective team collaboration. This example shows how grounded theory analysis allows you to generate a theory directly from the inherent nature of the data.
Method 5: Discourse Analysis
Discourse analysis focuses on language and communication. You’ll look at how language produces meaning and how it reflects power relations, identities, and cultural influences. This strategy examines what is said and how it is said; the words, phrasing, and larger context of communication.
The analysis is precious when investigating power dynamics, identities, and cultural influences encoded in language. By evaluating the language used in your data, you can identify underlying assumptions, cultural standards, and how individuals negotiate meaning through communication.
Steps to Do Discourse Analysis
Conducting discourse analysis entails the following steps:
- Select Discourse: For analysis, choose language-based data such as texts, speeches, or media content.
- Analyze Language: Immerse yourself in the conversation, examining language choices, metaphors, and underlying assumptions.
- Discover Patterns: Recognize the dialogue’s reoccurring themes, ideologies, and power dynamics. To fully understand the effects of these patterns, put them in their larger context.
There are various advantages of using discourse analysis:
- Understanding Language: It provides an extensive understanding of how language builds meaning and influences perceptions.
- Uncovering Power Dynamics: The analysis reveals how power dynamics appear via language.
- Cultural Insights: This method identifies cultural norms, beliefs, and ideologies stored in communication.
However, the following challenges may arise:
- Complexity of Interpretation: Language analysis involves navigating multiple levels of nuance and interpretation.
- Subjectivity: Interpretation can be subjective, so controlling researcher bias is important.
- Time-Intensive: Discourse analysis can take a lot of time because careful linguistic study is required in this analysis.
Example of Discourse Analysis
Consider doing discourse analysis on media coverage of a political event. You notice repeating linguistic patterns in news articles that depict the event as a conflict between opposing parties. Through deconstruction, you can expose how this framing supports particular ideologies and power relations.
You can illustrate how language choices influence public perceptions and contribute to building the narrative around the event by analyzing the speech within the broader political and social context. This example shows how discourse analysis can reveal hidden power dynamics and cultural influences on communication.
How to do Qualitative Data Analysis with the QuestionPro Research suite?
QuestionPro is a popular survey and research platform that offers tools for collecting and analyzing qualitative and quantitative data. Follow these general steps for conducting qualitative data analysis using the QuestionPro Research Suite:
- Collect Qualitative Data: Set up your survey to capture qualitative responses. It might involve open-ended questions, text boxes, or comment sections where participants can provide detailed responses.
- Export Qualitative Responses: Export the responses once you’ve collected qualitative data through your survey. QuestionPro typically allows you to export survey data in various formats, such as Excel or CSV.
- Prepare Data for Analysis: Review the exported data and clean it if necessary. Remove irrelevant or duplicate entries to ensure your data is ready for analysis.
- Code and Categorize Responses: Segment and label data, letting new patterns emerge naturally, then develop categories through axial coding to structure the analysis.
- Identify Themes: Analyze the coded responses to identify recurring themes, patterns, and insights. Look for similarities and differences in participants’ responses.
- Generate Reports and Visualizations: Utilize the reporting features of QuestionPro to create visualizations, charts, and graphs that help communicate the themes and findings from your qualitative research.
- Interpret and Draw Conclusions: Interpret the themes and patterns you’ve identified in the qualitative data. Consider how these findings answer your research questions or provide insights into your study topic.
- Integrate with Quantitative Data (if applicable): If you’re also conducting quantitative research using QuestionPro, consider integrating your qualitative findings with quantitative results to provide a more comprehensive understanding.
Qualitative data analysis is vital in uncovering various human experiences, views, and stories. If you’re ready to transform your research journey and apply the power of qualitative analysis, now is the moment to do it. Book a demo with QuestionPro today and begin your journey of exploration.
LEARN MORE FREE TRIAL
MORE LIKE THIS
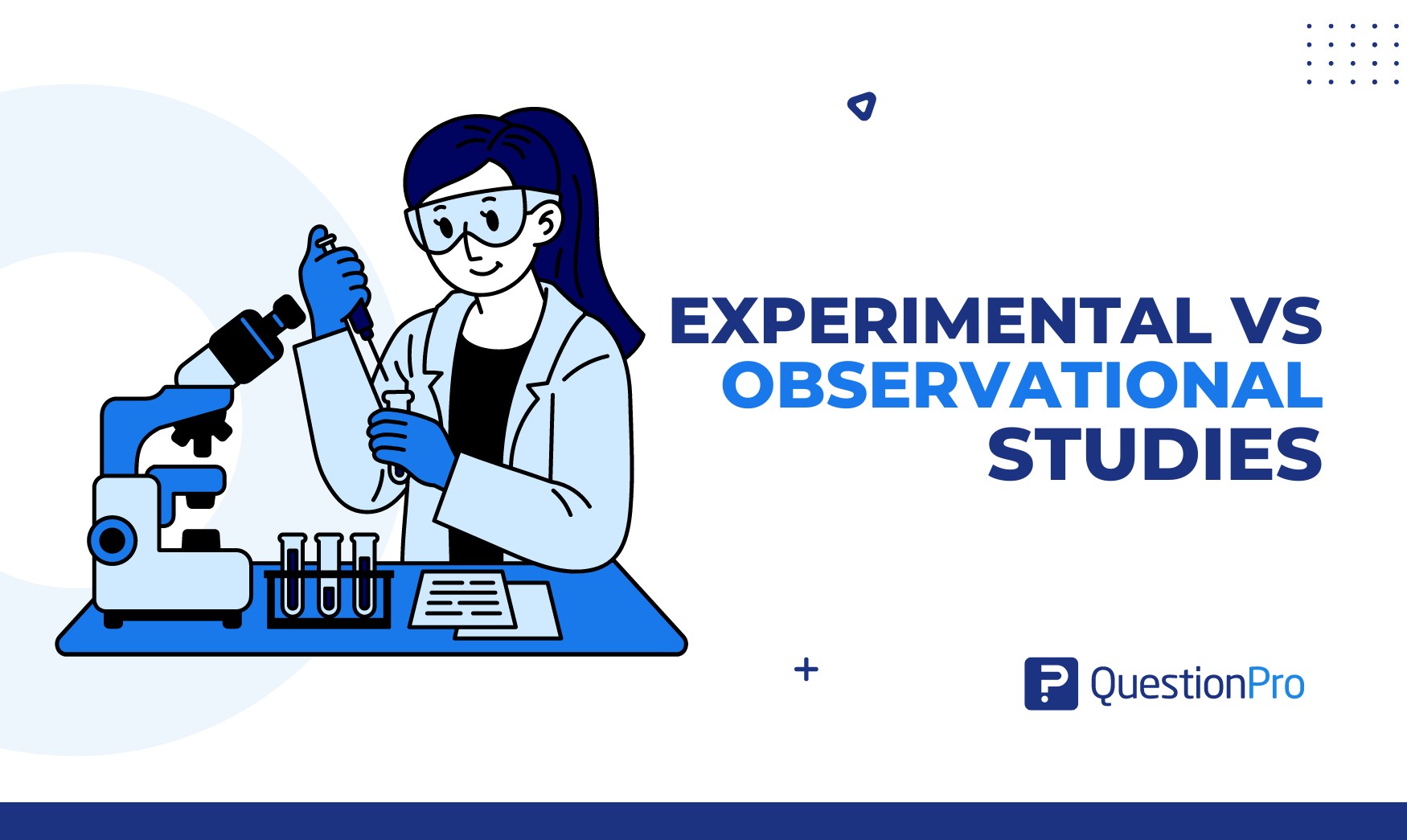
Experimental vs Observational Studies: Differences & Examples
Sep 5, 2024
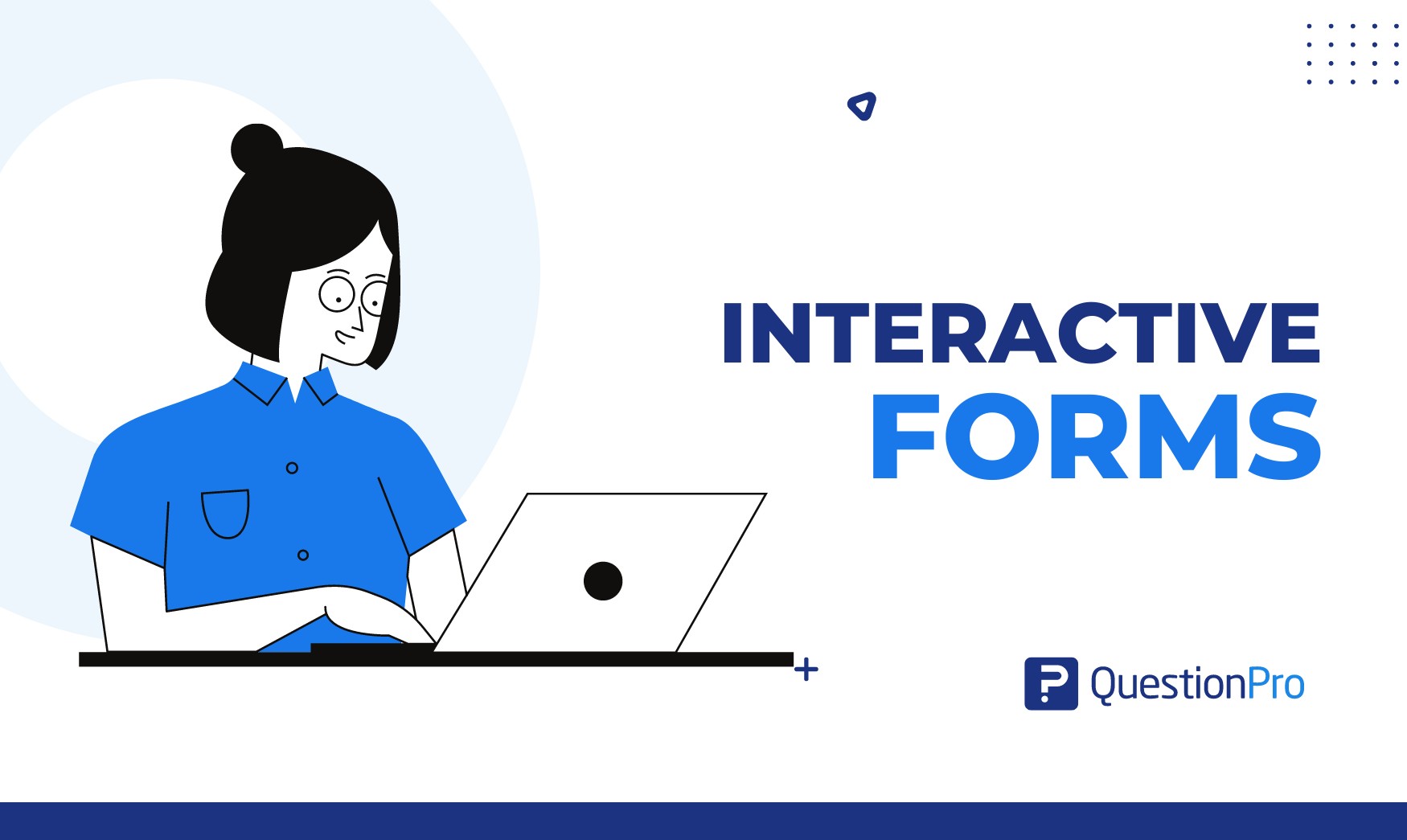
Interactive Forms: Key Features, Benefits, Uses + Design Tips
Sep 4, 2024
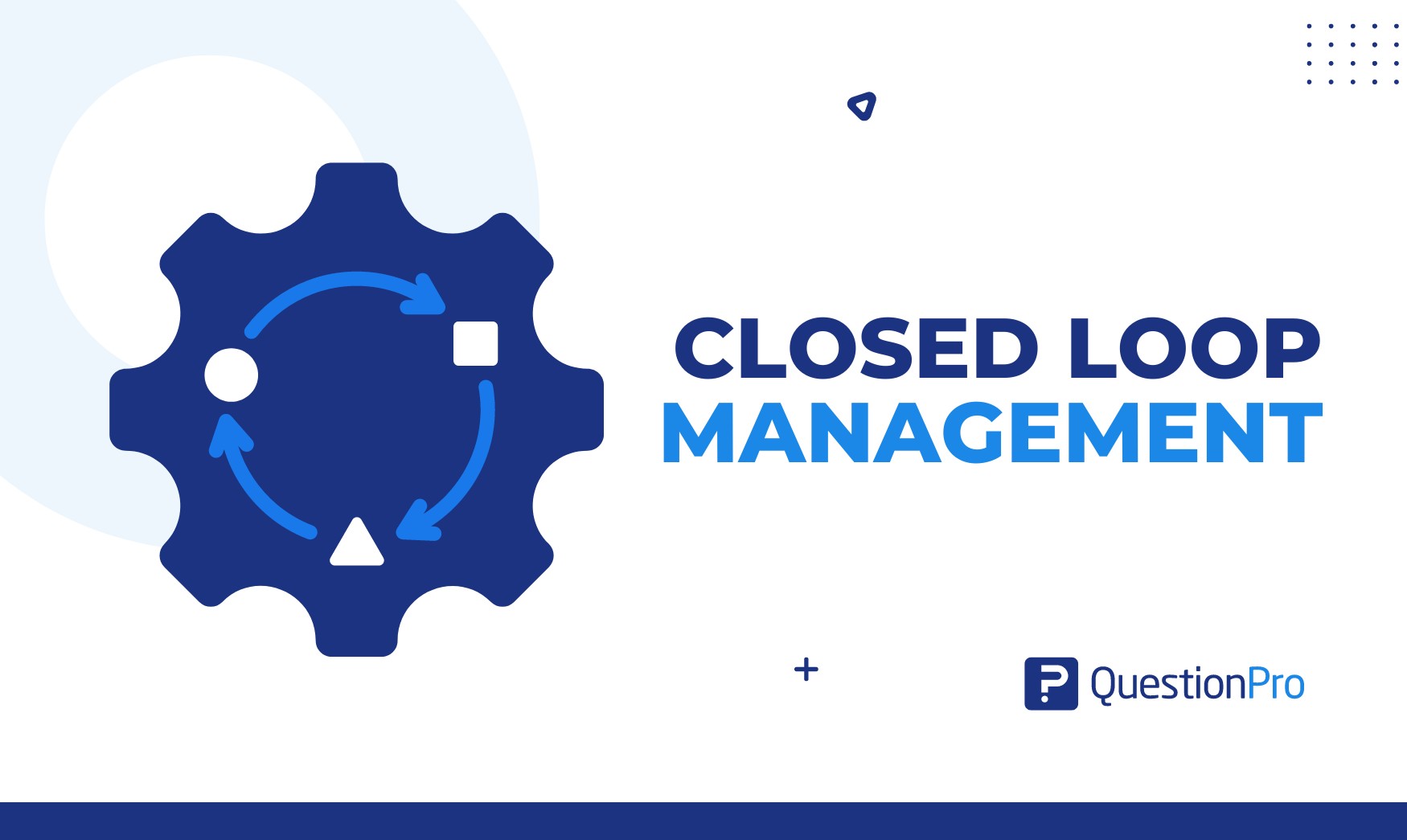
Closed-Loop Management: The Key to Customer Centricity
Sep 3, 2024
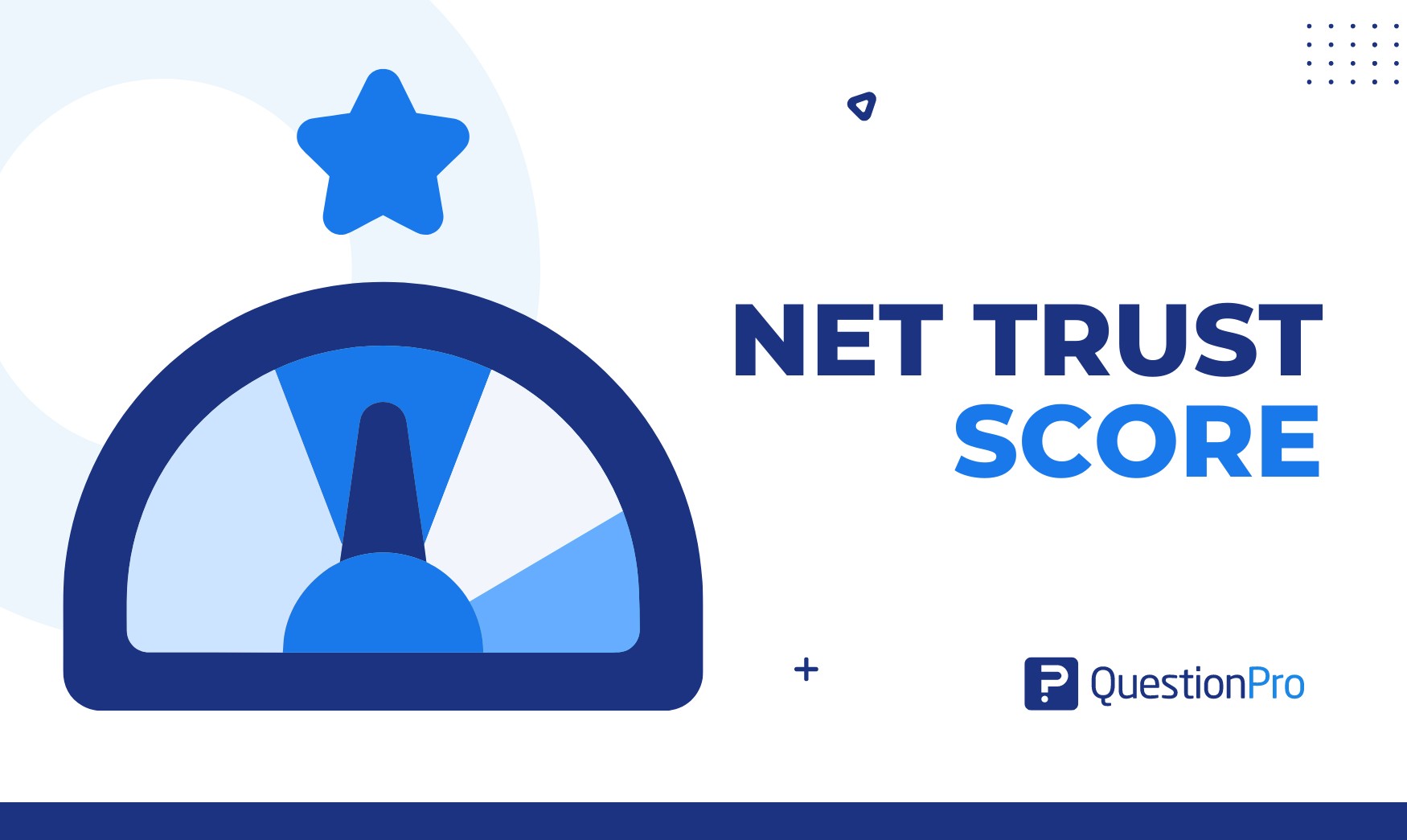
Net Trust Score: Tool for Measuring Trust in Organization
Sep 2, 2024
Other categories
- Academic Research
- Artificial Intelligence
- Assessments
- Brand Awareness
- Case Studies
- Communities
- Consumer Insights
- Customer effort score
- Customer Engagement
- Customer Experience
- Customer Loyalty
- Customer Research
- Customer Satisfaction
- Employee Benefits
- Employee Engagement
- Employee Retention
- Friday Five
- General Data Protection Regulation
- Insights Hub
- Life@QuestionPro
- Market Research
- Mobile diaries
- Mobile Surveys
- New Features
- Online Communities
- Question Types
- Questionnaire
- QuestionPro Products
- Release Notes
- Research Tools and Apps
- Revenue at Risk
- Survey Templates
- Training Tips
- Tuesday CX Thoughts (TCXT)
- Uncategorized
- What’s Coming Up
- Workforce Intelligence

An official website of the United States government
The .gov means it’s official. Federal government websites often end in .gov or .mil. Before sharing sensitive information, make sure you’re on a federal government site.
The site is secure. The https:// ensures that you are connecting to the official website and that any information you provide is encrypted and transmitted securely.
- Publications
- Account settings
Preview improvements coming to the PMC website in October 2024. Learn More or Try it out now .
- Advanced Search
- Journal List
- Indian J Anaesth
- v.60(9); 2016 Sep
Basic statistical tools in research and data analysis
Zulfiqar ali.
Department of Anaesthesiology, Division of Neuroanaesthesiology, Sheri Kashmir Institute of Medical Sciences, Soura, Srinagar, Jammu and Kashmir, India
S Bala Bhaskar
1 Department of Anaesthesiology and Critical Care, Vijayanagar Institute of Medical Sciences, Bellary, Karnataka, India
Statistical methods involved in carrying out a study include planning, designing, collecting data, analysing, drawing meaningful interpretation and reporting of the research findings. The statistical analysis gives meaning to the meaningless numbers, thereby breathing life into a lifeless data. The results and inferences are precise only if proper statistical tests are used. This article will try to acquaint the reader with the basic research tools that are utilised while conducting various studies. The article covers a brief outline of the variables, an understanding of quantitative and qualitative variables and the measures of central tendency. An idea of the sample size estimation, power analysis and the statistical errors is given. Finally, there is a summary of parametric and non-parametric tests used for data analysis.
INTRODUCTION
Statistics is a branch of science that deals with the collection, organisation, analysis of data and drawing of inferences from the samples to the whole population.[ 1 ] This requires a proper design of the study, an appropriate selection of the study sample and choice of a suitable statistical test. An adequate knowledge of statistics is necessary for proper designing of an epidemiological study or a clinical trial. Improper statistical methods may result in erroneous conclusions which may lead to unethical practice.[ 2 ]
Variable is a characteristic that varies from one individual member of population to another individual.[ 3 ] Variables such as height and weight are measured by some type of scale, convey quantitative information and are called as quantitative variables. Sex and eye colour give qualitative information and are called as qualitative variables[ 3 ] [ Figure 1 ].

Classification of variables
Quantitative variables
Quantitative or numerical data are subdivided into discrete and continuous measurements. Discrete numerical data are recorded as a whole number such as 0, 1, 2, 3,… (integer), whereas continuous data can assume any value. Observations that can be counted constitute the discrete data and observations that can be measured constitute the continuous data. Examples of discrete data are number of episodes of respiratory arrests or the number of re-intubations in an intensive care unit. Similarly, examples of continuous data are the serial serum glucose levels, partial pressure of oxygen in arterial blood and the oesophageal temperature.
A hierarchical scale of increasing precision can be used for observing and recording the data which is based on categorical, ordinal, interval and ratio scales [ Figure 1 ].
Categorical or nominal variables are unordered. The data are merely classified into categories and cannot be arranged in any particular order. If only two categories exist (as in gender male and female), it is called as a dichotomous (or binary) data. The various causes of re-intubation in an intensive care unit due to upper airway obstruction, impaired clearance of secretions, hypoxemia, hypercapnia, pulmonary oedema and neurological impairment are examples of categorical variables.
Ordinal variables have a clear ordering between the variables. However, the ordered data may not have equal intervals. Examples are the American Society of Anesthesiologists status or Richmond agitation-sedation scale.
Interval variables are similar to an ordinal variable, except that the intervals between the values of the interval variable are equally spaced. A good example of an interval scale is the Fahrenheit degree scale used to measure temperature. With the Fahrenheit scale, the difference between 70° and 75° is equal to the difference between 80° and 85°: The units of measurement are equal throughout the full range of the scale.
Ratio scales are similar to interval scales, in that equal differences between scale values have equal quantitative meaning. However, ratio scales also have a true zero point, which gives them an additional property. For example, the system of centimetres is an example of a ratio scale. There is a true zero point and the value of 0 cm means a complete absence of length. The thyromental distance of 6 cm in an adult may be twice that of a child in whom it may be 3 cm.
STATISTICS: DESCRIPTIVE AND INFERENTIAL STATISTICS
Descriptive statistics[ 4 ] try to describe the relationship between variables in a sample or population. Descriptive statistics provide a summary of data in the form of mean, median and mode. Inferential statistics[ 4 ] use a random sample of data taken from a population to describe and make inferences about the whole population. It is valuable when it is not possible to examine each member of an entire population. The examples if descriptive and inferential statistics are illustrated in Table 1 .
Example of descriptive and inferential statistics

Descriptive statistics
The extent to which the observations cluster around a central location is described by the central tendency and the spread towards the extremes is described by the degree of dispersion.
Measures of central tendency
The measures of central tendency are mean, median and mode.[ 6 ] Mean (or the arithmetic average) is the sum of all the scores divided by the number of scores. Mean may be influenced profoundly by the extreme variables. For example, the average stay of organophosphorus poisoning patients in ICU may be influenced by a single patient who stays in ICU for around 5 months because of septicaemia. The extreme values are called outliers. The formula for the mean is

where x = each observation and n = number of observations. Median[ 6 ] is defined as the middle of a distribution in a ranked data (with half of the variables in the sample above and half below the median value) while mode is the most frequently occurring variable in a distribution. Range defines the spread, or variability, of a sample.[ 7 ] It is described by the minimum and maximum values of the variables. If we rank the data and after ranking, group the observations into percentiles, we can get better information of the pattern of spread of the variables. In percentiles, we rank the observations into 100 equal parts. We can then describe 25%, 50%, 75% or any other percentile amount. The median is the 50 th percentile. The interquartile range will be the observations in the middle 50% of the observations about the median (25 th -75 th percentile). Variance[ 7 ] is a measure of how spread out is the distribution. It gives an indication of how close an individual observation clusters about the mean value. The variance of a population is defined by the following formula:

where σ 2 is the population variance, X is the population mean, X i is the i th element from the population and N is the number of elements in the population. The variance of a sample is defined by slightly different formula:

where s 2 is the sample variance, x is the sample mean, x i is the i th element from the sample and n is the number of elements in the sample. The formula for the variance of a population has the value ‘ n ’ as the denominator. The expression ‘ n −1’ is known as the degrees of freedom and is one less than the number of parameters. Each observation is free to vary, except the last one which must be a defined value. The variance is measured in squared units. To make the interpretation of the data simple and to retain the basic unit of observation, the square root of variance is used. The square root of the variance is the standard deviation (SD).[ 8 ] The SD of a population is defined by the following formula:

where σ is the population SD, X is the population mean, X i is the i th element from the population and N is the number of elements in the population. The SD of a sample is defined by slightly different formula:

where s is the sample SD, x is the sample mean, x i is the i th element from the sample and n is the number of elements in the sample. An example for calculation of variation and SD is illustrated in Table 2 .
Example of mean, variance, standard deviation

Normal distribution or Gaussian distribution
Most of the biological variables usually cluster around a central value, with symmetrical positive and negative deviations about this point.[ 1 ] The standard normal distribution curve is a symmetrical bell-shaped. In a normal distribution curve, about 68% of the scores are within 1 SD of the mean. Around 95% of the scores are within 2 SDs of the mean and 99% within 3 SDs of the mean [ Figure 2 ].

Normal distribution curve
Skewed distribution
It is a distribution with an asymmetry of the variables about its mean. In a negatively skewed distribution [ Figure 3 ], the mass of the distribution is concentrated on the right of Figure 1 . In a positively skewed distribution [ Figure 3 ], the mass of the distribution is concentrated on the left of the figure leading to a longer right tail.

Curves showing negatively skewed and positively skewed distribution
Inferential statistics
In inferential statistics, data are analysed from a sample to make inferences in the larger collection of the population. The purpose is to answer or test the hypotheses. A hypothesis (plural hypotheses) is a proposed explanation for a phenomenon. Hypothesis tests are thus procedures for making rational decisions about the reality of observed effects.
Probability is the measure of the likelihood that an event will occur. Probability is quantified as a number between 0 and 1 (where 0 indicates impossibility and 1 indicates certainty).
In inferential statistics, the term ‘null hypothesis’ ( H 0 ‘ H-naught ,’ ‘ H-null ’) denotes that there is no relationship (difference) between the population variables in question.[ 9 ]
Alternative hypothesis ( H 1 and H a ) denotes that a statement between the variables is expected to be true.[ 9 ]
The P value (or the calculated probability) is the probability of the event occurring by chance if the null hypothesis is true. The P value is a numerical between 0 and 1 and is interpreted by researchers in deciding whether to reject or retain the null hypothesis [ Table 3 ].
P values with interpretation

If P value is less than the arbitrarily chosen value (known as α or the significance level), the null hypothesis (H0) is rejected [ Table 4 ]. However, if null hypotheses (H0) is incorrectly rejected, this is known as a Type I error.[ 11 ] Further details regarding alpha error, beta error and sample size calculation and factors influencing them are dealt with in another section of this issue by Das S et al .[ 12 ]
Illustration for null hypothesis

PARAMETRIC AND NON-PARAMETRIC TESTS
Numerical data (quantitative variables) that are normally distributed are analysed with parametric tests.[ 13 ]
Two most basic prerequisites for parametric statistical analysis are:
- The assumption of normality which specifies that the means of the sample group are normally distributed
- The assumption of equal variance which specifies that the variances of the samples and of their corresponding population are equal.
However, if the distribution of the sample is skewed towards one side or the distribution is unknown due to the small sample size, non-parametric[ 14 ] statistical techniques are used. Non-parametric tests are used to analyse ordinal and categorical data.
Parametric tests
The parametric tests assume that the data are on a quantitative (numerical) scale, with a normal distribution of the underlying population. The samples have the same variance (homogeneity of variances). The samples are randomly drawn from the population, and the observations within a group are independent of each other. The commonly used parametric tests are the Student's t -test, analysis of variance (ANOVA) and repeated measures ANOVA.
Student's t -test
Student's t -test is used to test the null hypothesis that there is no difference between the means of the two groups. It is used in three circumstances:

where X = sample mean, u = population mean and SE = standard error of mean

where X 1 − X 2 is the difference between the means of the two groups and SE denotes the standard error of the difference.
- To test if the population means estimated by two dependent samples differ significantly (the paired t -test). A usual setting for paired t -test is when measurements are made on the same subjects before and after a treatment.
The formula for paired t -test is:

where d is the mean difference and SE denotes the standard error of this difference.
The group variances can be compared using the F -test. The F -test is the ratio of variances (var l/var 2). If F differs significantly from 1.0, then it is concluded that the group variances differ significantly.
Analysis of variance
The Student's t -test cannot be used for comparison of three or more groups. The purpose of ANOVA is to test if there is any significant difference between the means of two or more groups.
In ANOVA, we study two variances – (a) between-group variability and (b) within-group variability. The within-group variability (error variance) is the variation that cannot be accounted for in the study design. It is based on random differences present in our samples.
However, the between-group (or effect variance) is the result of our treatment. These two estimates of variances are compared using the F-test.
A simplified formula for the F statistic is:

where MS b is the mean squares between the groups and MS w is the mean squares within groups.
Repeated measures analysis of variance
As with ANOVA, repeated measures ANOVA analyses the equality of means of three or more groups. However, a repeated measure ANOVA is used when all variables of a sample are measured under different conditions or at different points in time.
As the variables are measured from a sample at different points of time, the measurement of the dependent variable is repeated. Using a standard ANOVA in this case is not appropriate because it fails to model the correlation between the repeated measures: The data violate the ANOVA assumption of independence. Hence, in the measurement of repeated dependent variables, repeated measures ANOVA should be used.
Non-parametric tests
When the assumptions of normality are not met, and the sample means are not normally, distributed parametric tests can lead to erroneous results. Non-parametric tests (distribution-free test) are used in such situation as they do not require the normality assumption.[ 15 ] Non-parametric tests may fail to detect a significant difference when compared with a parametric test. That is, they usually have less power.
As is done for the parametric tests, the test statistic is compared with known values for the sampling distribution of that statistic and the null hypothesis is accepted or rejected. The types of non-parametric analysis techniques and the corresponding parametric analysis techniques are delineated in Table 5 .
Analogue of parametric and non-parametric tests

Median test for one sample: The sign test and Wilcoxon's signed rank test
The sign test and Wilcoxon's signed rank test are used for median tests of one sample. These tests examine whether one instance of sample data is greater or smaller than the median reference value.
This test examines the hypothesis about the median θ0 of a population. It tests the null hypothesis H0 = θ0. When the observed value (Xi) is greater than the reference value (θ0), it is marked as+. If the observed value is smaller than the reference value, it is marked as − sign. If the observed value is equal to the reference value (θ0), it is eliminated from the sample.
If the null hypothesis is true, there will be an equal number of + signs and − signs.
The sign test ignores the actual values of the data and only uses + or − signs. Therefore, it is useful when it is difficult to measure the values.
Wilcoxon's signed rank test
There is a major limitation of sign test as we lose the quantitative information of the given data and merely use the + or – signs. Wilcoxon's signed rank test not only examines the observed values in comparison with θ0 but also takes into consideration the relative sizes, adding more statistical power to the test. As in the sign test, if there is an observed value that is equal to the reference value θ0, this observed value is eliminated from the sample.
Wilcoxon's rank sum test ranks all data points in order, calculates the rank sum of each sample and compares the difference in the rank sums.
Mann-Whitney test
It is used to test the null hypothesis that two samples have the same median or, alternatively, whether observations in one sample tend to be larger than observations in the other.
Mann–Whitney test compares all data (xi) belonging to the X group and all data (yi) belonging to the Y group and calculates the probability of xi being greater than yi: P (xi > yi). The null hypothesis states that P (xi > yi) = P (xi < yi) =1/2 while the alternative hypothesis states that P (xi > yi) ≠1/2.
Kolmogorov-Smirnov test
The two-sample Kolmogorov-Smirnov (KS) test was designed as a generic method to test whether two random samples are drawn from the same distribution. The null hypothesis of the KS test is that both distributions are identical. The statistic of the KS test is a distance between the two empirical distributions, computed as the maximum absolute difference between their cumulative curves.
Kruskal-Wallis test
The Kruskal–Wallis test is a non-parametric test to analyse the variance.[ 14 ] It analyses if there is any difference in the median values of three or more independent samples. The data values are ranked in an increasing order, and the rank sums calculated followed by calculation of the test statistic.
Jonckheere test
In contrast to Kruskal–Wallis test, in Jonckheere test, there is an a priori ordering that gives it a more statistical power than the Kruskal–Wallis test.[ 14 ]
Friedman test
The Friedman test is a non-parametric test for testing the difference between several related samples. The Friedman test is an alternative for repeated measures ANOVAs which is used when the same parameter has been measured under different conditions on the same subjects.[ 13 ]
Tests to analyse the categorical data
Chi-square test, Fischer's exact test and McNemar's test are used to analyse the categorical or nominal variables. The Chi-square test compares the frequencies and tests whether the observed data differ significantly from that of the expected data if there were no differences between groups (i.e., the null hypothesis). It is calculated by the sum of the squared difference between observed ( O ) and the expected ( E ) data (or the deviation, d ) divided by the expected data by the following formula:

A Yates correction factor is used when the sample size is small. Fischer's exact test is used to determine if there are non-random associations between two categorical variables. It does not assume random sampling, and instead of referring a calculated statistic to a sampling distribution, it calculates an exact probability. McNemar's test is used for paired nominal data. It is applied to 2 × 2 table with paired-dependent samples. It is used to determine whether the row and column frequencies are equal (that is, whether there is ‘marginal homogeneity’). The null hypothesis is that the paired proportions are equal. The Mantel-Haenszel Chi-square test is a multivariate test as it analyses multiple grouping variables. It stratifies according to the nominated confounding variables and identifies any that affects the primary outcome variable. If the outcome variable is dichotomous, then logistic regression is used.
SOFTWARES AVAILABLE FOR STATISTICS, SAMPLE SIZE CALCULATION AND POWER ANALYSIS
Numerous statistical software systems are available currently. The commonly used software systems are Statistical Package for the Social Sciences (SPSS – manufactured by IBM corporation), Statistical Analysis System ((SAS – developed by SAS Institute North Carolina, United States of America), R (designed by Ross Ihaka and Robert Gentleman from R core team), Minitab (developed by Minitab Inc), Stata (developed by StataCorp) and the MS Excel (developed by Microsoft).
There are a number of web resources which are related to statistical power analyses. A few are:
- StatPages.net – provides links to a number of online power calculators
- G-Power – provides a downloadable power analysis program that runs under DOS
- Power analysis for ANOVA designs an interactive site that calculates power or sample size needed to attain a given power for one effect in a factorial ANOVA design
- SPSS makes a program called SamplePower. It gives an output of a complete report on the computer screen which can be cut and paste into another document.
It is important that a researcher knows the concepts of the basic statistical methods used for conduct of a research study. This will help to conduct an appropriately well-designed study leading to valid and reliable results. Inappropriate use of statistical techniques may lead to faulty conclusions, inducing errors and undermining the significance of the article. Bad statistics may lead to bad research, and bad research may lead to unethical practice. Hence, an adequate knowledge of statistics and the appropriate use of statistical tests are important. An appropriate knowledge about the basic statistical methods will go a long way in improving the research designs and producing quality medical research which can be utilised for formulating the evidence-based guidelines.
Financial support and sponsorship
Conflicts of interest.
There are no conflicts of interest.
Business growth
Business tips
What is data analysis? Examples and how to get started
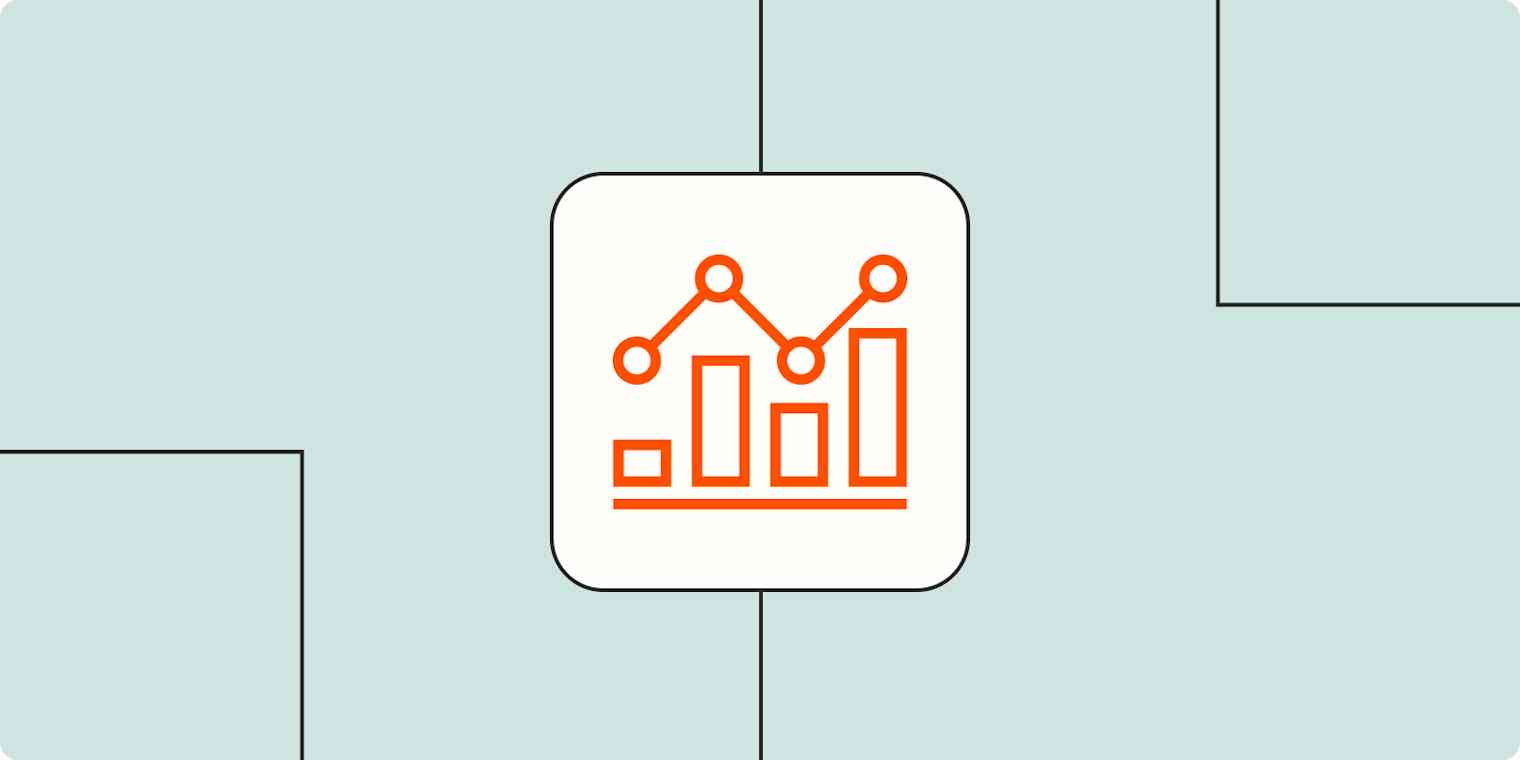
Even with years of professional experience working with data, the term "data analysis" still sets off a panic button in my soul. And yes, when it comes to serious data analysis for your business, you'll eventually want data scientists on your side. But if you're just getting started, no panic attacks are required.
Table of contents:
Quick review: What is data analysis?
Data analysis is the process of examining, filtering, adapting, and modeling data to help solve problems. Data analysis helps determine what is and isn't working, so you can make the changes needed to achieve your business goals.
Keep in mind that data analysis includes analyzing both quantitative data (e.g., profits and sales) and qualitative data (e.g., surveys and case studies) to paint the whole picture. Here are two simple examples (of a nuanced topic) to show you what I mean.
An example of quantitative data analysis is an online jewelry store owner using inventory data to forecast and improve reordering accuracy. The owner looks at their sales from the past six months and sees that, on average, they sold 210 gold pieces and 105 silver pieces per month, but they only had 100 gold pieces and 100 silver pieces in stock. By collecting and analyzing inventory data on these SKUs, they're forecasting to improve reordering accuracy. The next time they order inventory, they order twice as many gold pieces as silver to meet customer demand.
An example of qualitative data analysis is a fitness studio owner collecting customer feedback to improve class offerings. The studio owner sends out an open-ended survey asking customers what types of exercises they enjoy the most. The owner then performs qualitative content analysis to identify the most frequently suggested exercises and incorporates these into future workout classes.
Why is data analysis important?
Here's why it's worth implementing data analysis for your business:
Understand your target audience: You might think you know how to best target your audience, but are your assumptions backed by data? Data analysis can help answer questions like, "What demographics define my target audience?" or "What is my audience motivated by?"
Inform decisions: You don't need to toss and turn over a decision when the data points clearly to the answer. For instance, a restaurant could analyze which dishes on the menu are selling the most, helping them decide which ones to keep and which ones to change.
Adjust budgets: Similarly, data analysis can highlight areas in your business that are performing well and are worth investing more in, as well as areas that aren't generating enough revenue and should be cut. For example, a B2B software company might discover their product for enterprises is thriving while their small business solution lags behind. This discovery could prompt them to allocate more budget toward the enterprise product, resulting in better resource utilization.
Identify and solve problems: Let's say a cell phone manufacturer notices data showing a lot of customers returning a certain model. When they investigate, they find that model also happens to have the highest number of crashes. Once they identify and solve the technical issue, they can reduce the number of returns.
Types of data analysis (with examples)
There are five main types of data analysis—with increasingly scary-sounding names. Each one serves a different purpose, so take a look to see which makes the most sense for your situation. It's ok if you can't pronounce the one you choose.
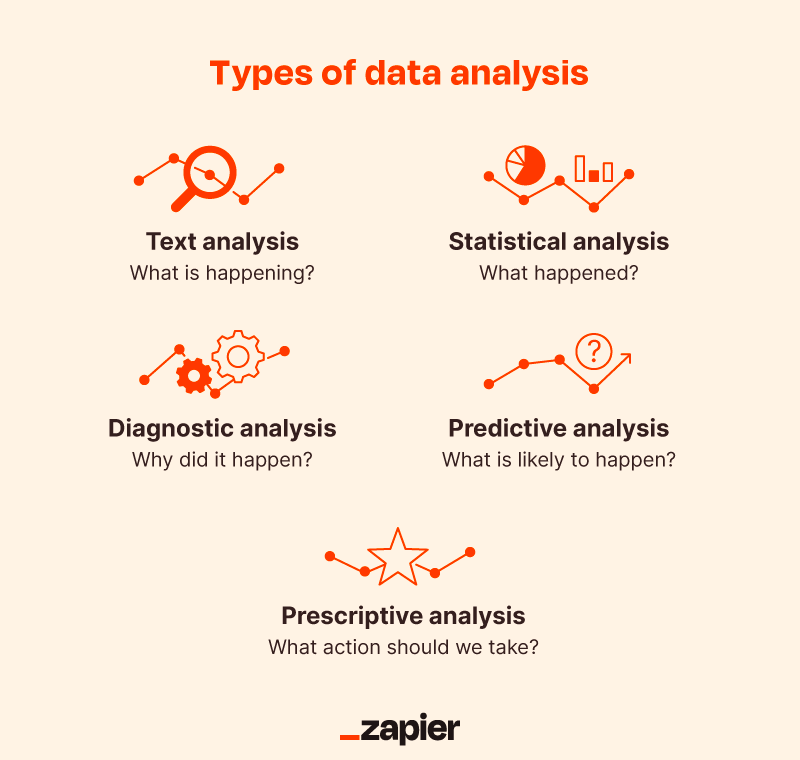
Text analysis: What is happening?
Here are a few methods used to perform text analysis, to give you a sense of how it's different from a human reading through the text:
Word frequency identifies the most frequently used words. For example, a restaurant monitors social media mentions and measures the frequency of positive and negative keywords like "delicious" or "expensive" to determine how customers feel about their experience.
Language detection indicates the language of text. For example, a global software company may use language detection on support tickets to connect customers with the appropriate agent.
Keyword extraction automatically identifies the most used terms. For example, instead of sifting through thousands of reviews, a popular brand uses a keyword extractor to summarize the words or phrases that are most relevant.
Statistical analysis: What happened?
Statistical analysis pulls past data to identify meaningful trends. Two primary categories of statistical analysis exist: descriptive and inferential.
Descriptive analysis
Here are a few methods used to perform descriptive analysis:
Measures of frequency identify how frequently an event occurs. For example, a popular coffee chain sends out a survey asking customers what their favorite holiday drink is and uses measures of frequency to determine how often a particular drink is selected.
Measures of central tendency use mean, median, and mode to identify results. For example, a dating app company might use measures of central tendency to determine the average age of its users.
Measures of dispersion measure how data is distributed across a range. For example, HR may use measures of dispersion to determine what salary to offer in a given field.
Inferential analysis
Inferential analysis uses a sample of data to draw conclusions about a much larger population. This type of analysis is used when the population you're interested in analyzing is very large.
Here are a few methods used when performing inferential analysis:
Hypothesis testing identifies which variables impact a particular topic. For example, a business uses hypothesis testing to determine if increased sales were the result of a specific marketing campaign.
Regression analysis shows the effect of independent variables on a dependent variable. For example, a rental car company may use regression analysis to determine the relationship between wait times and number of bad reviews.
Diagnostic analysis: Why did it happen?
Diagnostic analysis, also referred to as root cause analysis, uncovers the causes of certain events or results.
Here are a few methods used to perform diagnostic analysis:
Time-series analysis analyzes data collected over a period of time. A retail store may use time-series analysis to determine that sales increase between October and December every year.
Correlation analysis determines the strength of the relationship between variables. For example, a local ice cream shop may determine that as the temperature in the area rises, so do ice cream sales.
Predictive analysis: What is likely to happen?
Predictive analysis aims to anticipate future developments and events. By analyzing past data, companies can predict future scenarios and make strategic decisions.
Here are a few methods used to perform predictive analysis:
Decision trees map out possible courses of action and outcomes. For example, a business may use a decision tree when deciding whether to downsize or expand.
Prescriptive analysis: What action should we take?
The highest level of analysis, prescriptive analysis, aims to find the best action plan. Typically, AI tools model different outcomes to predict the best approach. While these tools serve to provide insight, they don't replace human consideration, so always use your human brain before going with the conclusion of your prescriptive analysis. Otherwise, your GPS might drive you into a lake.
Here are a few methods used to perform prescriptive analysis:
Algorithms are used in technology to perform specific tasks. For example, banks use prescriptive algorithms to monitor customers' spending and recommend that they deactivate their credit card if fraud is suspected.
Data analysis process: How to get started
The actual analysis is just one step in a much bigger process of using data to move your business forward. Here's a quick look at all the steps you need to take to make sure you're making informed decisions.
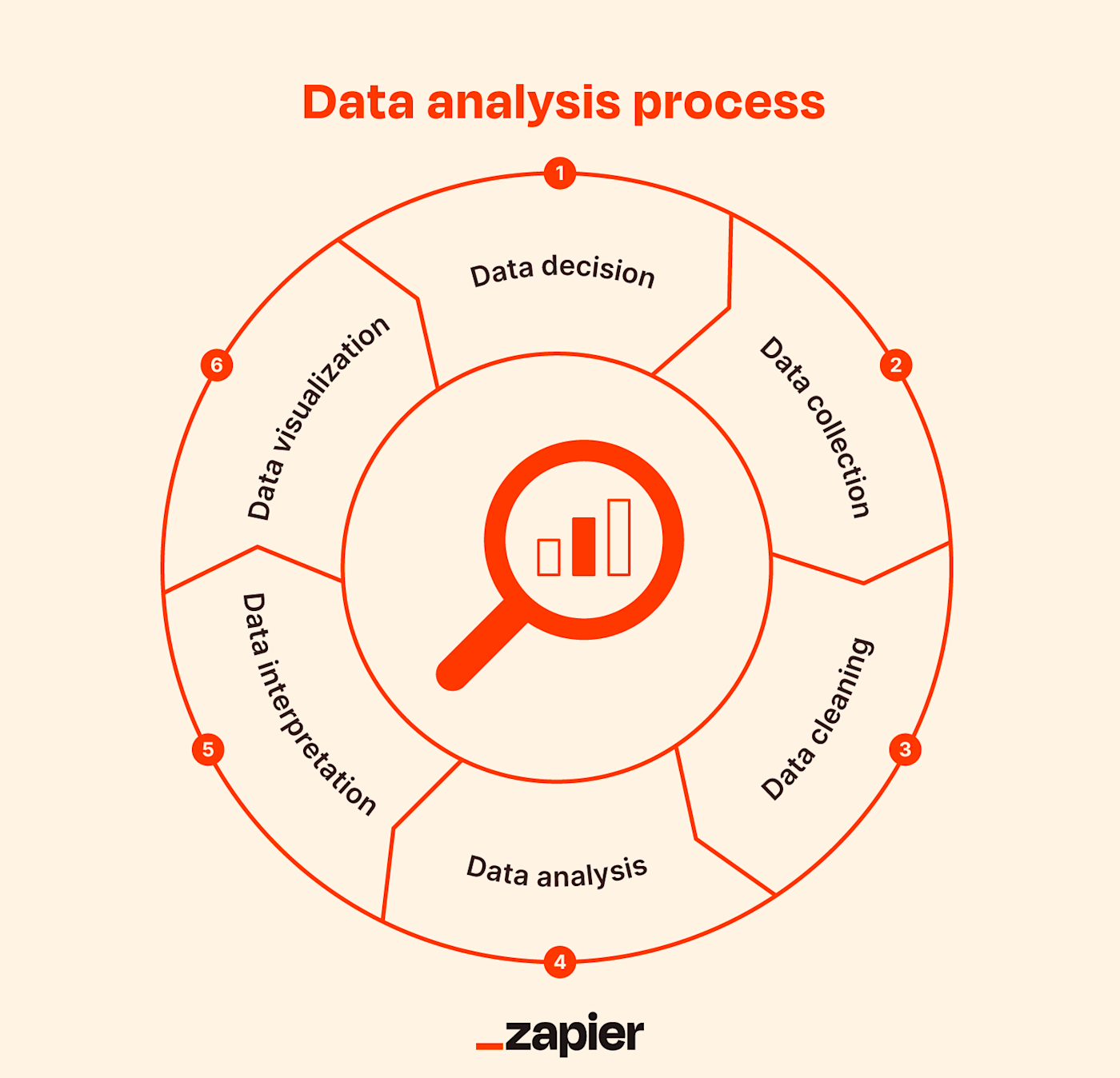
Data decision
As with almost any project, the first step is to determine what problem you're trying to solve through data analysis.
Make sure you get specific here. For example, a food delivery service may want to understand why customers are canceling their subscriptions. But to enable the most effective data analysis, they should pose a more targeted question, such as "How can we reduce customer churn without raising costs?"
Data collection
Next, collect the required data from both internal and external sources.
Internal data comes from within your business (think CRM software, internal reports, and archives), and helps you understand your business and processes.
External data originates from outside of the company (surveys, questionnaires, public data) and helps you understand your industry and your customers.
Data cleaning
Data can be seriously misleading if it's not clean. So before you analyze, make sure you review the data you collected. Depending on the type of data you have, cleanup will look different, but it might include:
Removing unnecessary information
Addressing structural errors like misspellings
Deleting duplicates
Trimming whitespace
Human checking for accuracy
Data analysis
Now that you've compiled and cleaned the data, use one or more of the above types of data analysis to find relationships, patterns, and trends.
Data analysis tools can speed up the data analysis process and remove the risk of inevitable human error. Here are some examples.
Spreadsheets sort, filter, analyze, and visualize data.
Structured query language (SQL) tools manage and extract data in relational databases.
Data interpretation
After you analyze the data, you'll need to go back to the original question you posed and draw conclusions from your findings. Here are some common pitfalls to avoid:
Correlation vs. causation: Just because two variables are associated doesn't mean they're necessarily related or dependent on one another.
Confirmation bias: This occurs when you interpret data in a way that confirms your own preconceived notions. To avoid this, have multiple people interpret the data.
Small sample size: If your sample size is too small or doesn't represent the demographics of your customers, you may get misleading results. If you run into this, consider widening your sample size to give you a more accurate representation.
Data visualization
Automate your data collection, frequently asked questions.
Need a quick summary or still have a few nagging data analysis questions? I'm here for you.
What are the five types of data analysis?
The five types of data analysis are text analysis, statistical analysis, diagnostic analysis, predictive analysis, and prescriptive analysis. Each type offers a unique lens for understanding data: text analysis provides insights into text-based content, statistical analysis focuses on numerical trends, diagnostic analysis looks into problem causes, predictive analysis deals with what may happen in the future, and prescriptive analysis gives actionable recommendations.
What is the data analysis process?
The data analysis process involves data decision, collection, cleaning, analysis, interpretation, and visualization. Every stage comes together to transform raw data into meaningful insights. Decision determines what data to collect, collection gathers the relevant information, cleaning ensures accuracy, analysis uncovers patterns, interpretation assigns meaning, and visualization presents the insights.
What is the main purpose of data analysis?
In business, the main purpose of data analysis is to uncover patterns, trends, and anomalies, and then use that information to make decisions, solve problems, and reach your business goals.
Related reading:
This article was originally published in October 2022 and has since been updated with contributions from Cecilia Gillen. The most recent update was in September 2023.
Get productivity tips delivered straight to your inbox
We’ll email you 1-3 times per week—and never share your information.
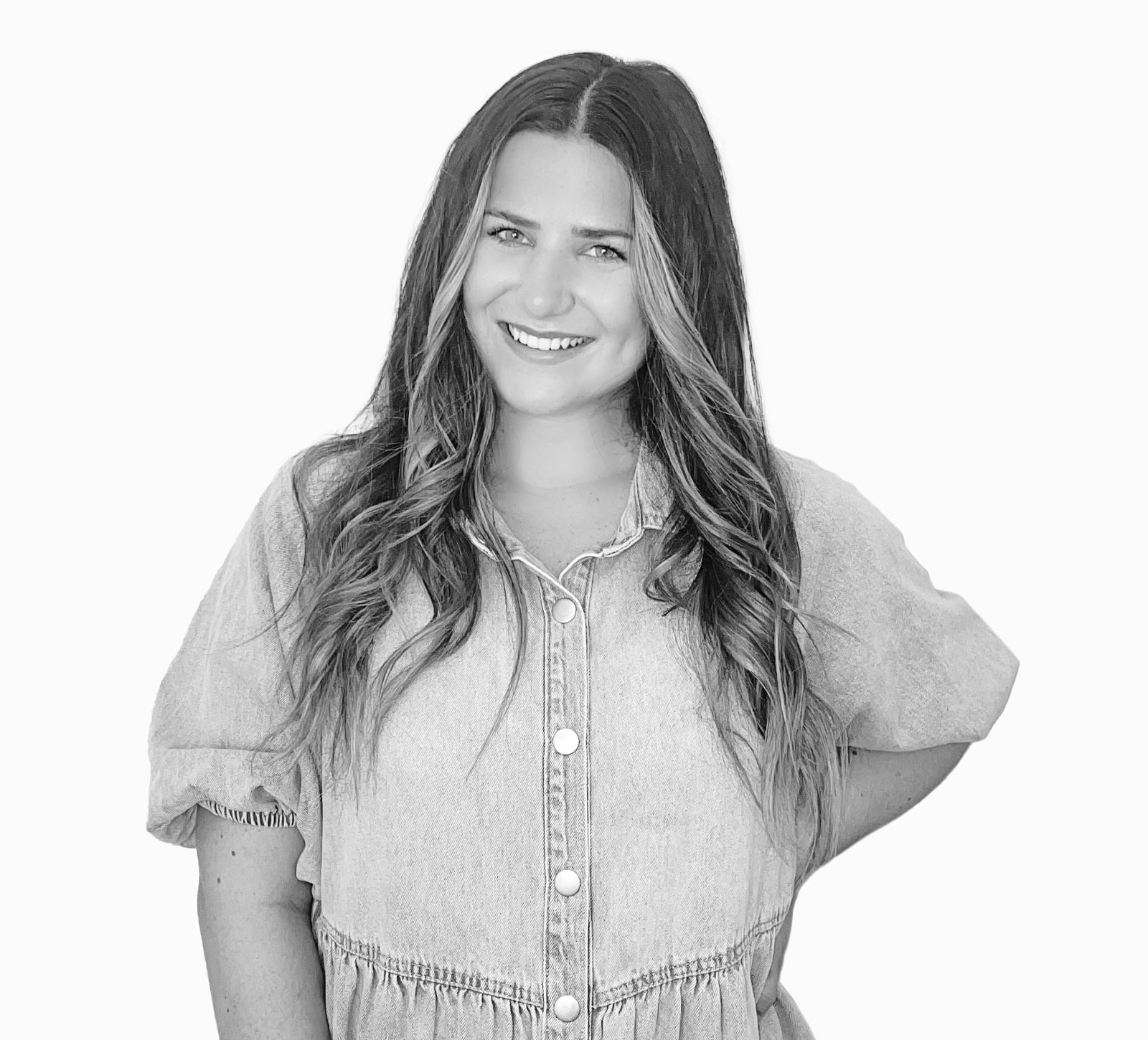
Shea Stevens
Shea is a content writer currently living in Charlotte, North Carolina. After graduating with a degree in Marketing from East Carolina University, she joined the digital marketing industry focusing on content and social media. In her free time, you can find Shea visiting her local farmers market, attending a country music concert, or planning her next adventure.
- Data & analytics
- Small business
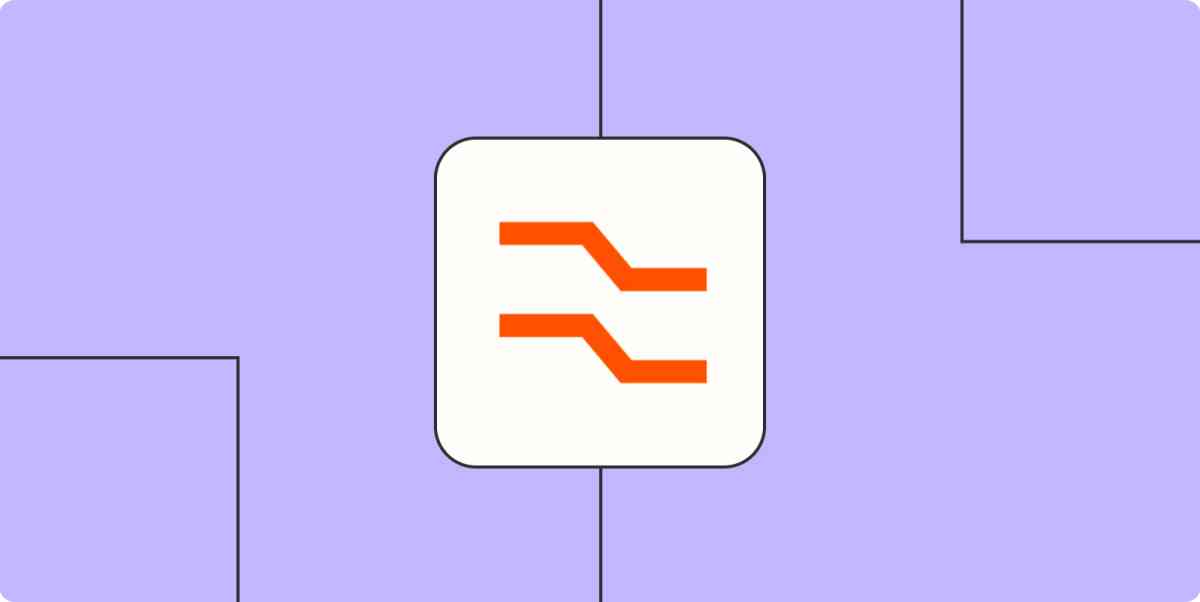
Data extraction is the process of taking actionable information from larger, less structured sources to be further refined or analyzed. Here's how to do it.
Related articles
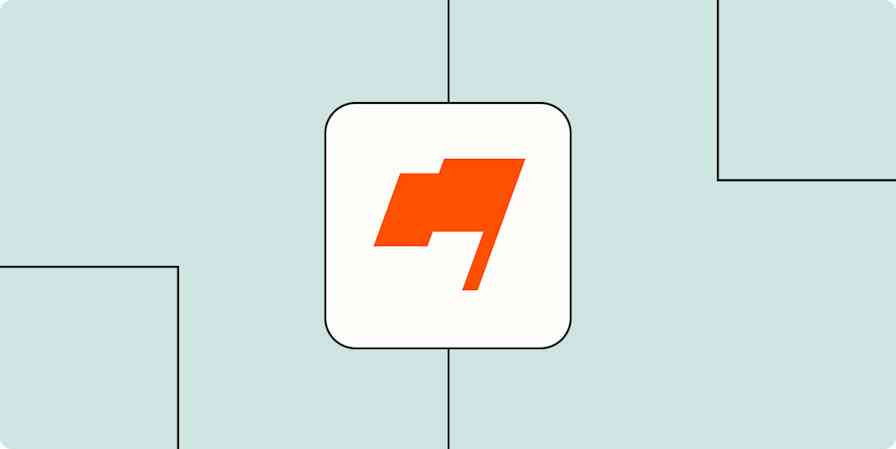
Project milestones for improved project management
Project milestones for improved project...
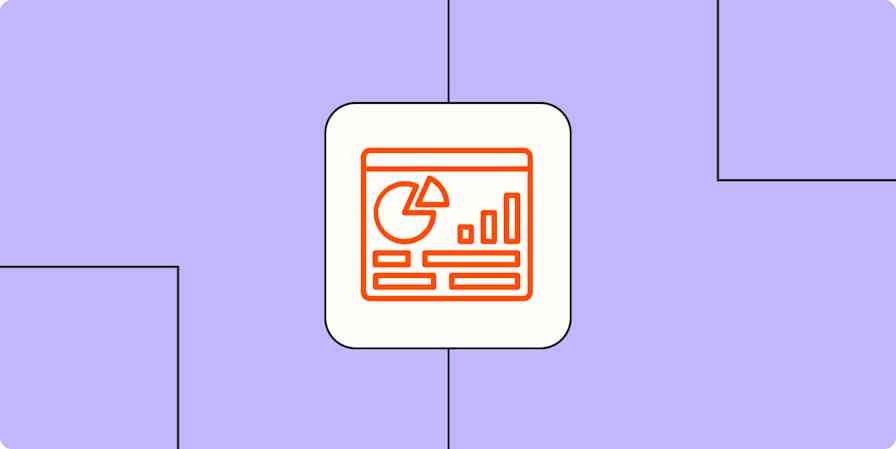
14 data visualization examples to captivate your audience
14 data visualization examples to captivate...
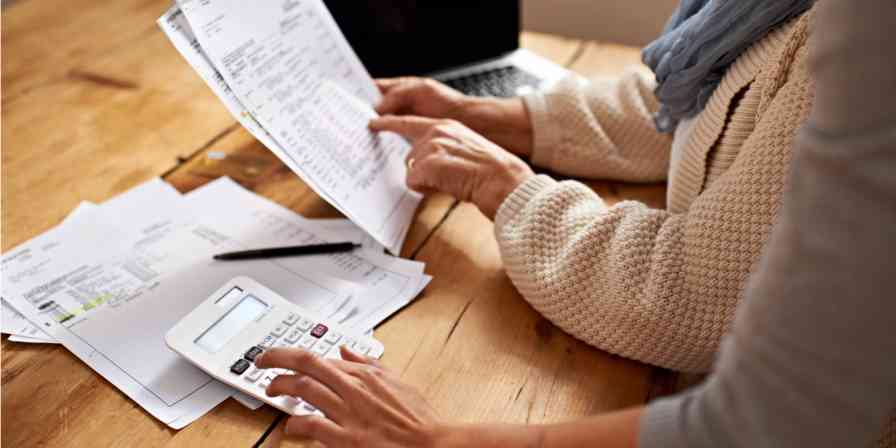
61 best businesses to start with $10K or less
61 best businesses to start with $10K or...
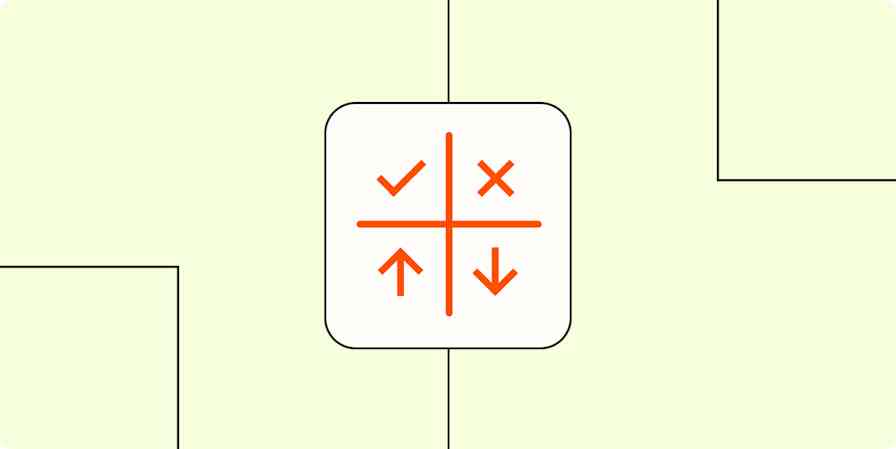
SWOT analysis: A how-to guide and template (that won't bore you to tears)
SWOT analysis: A how-to guide and template...
Improve your productivity automatically. Use Zapier to get your apps working together.
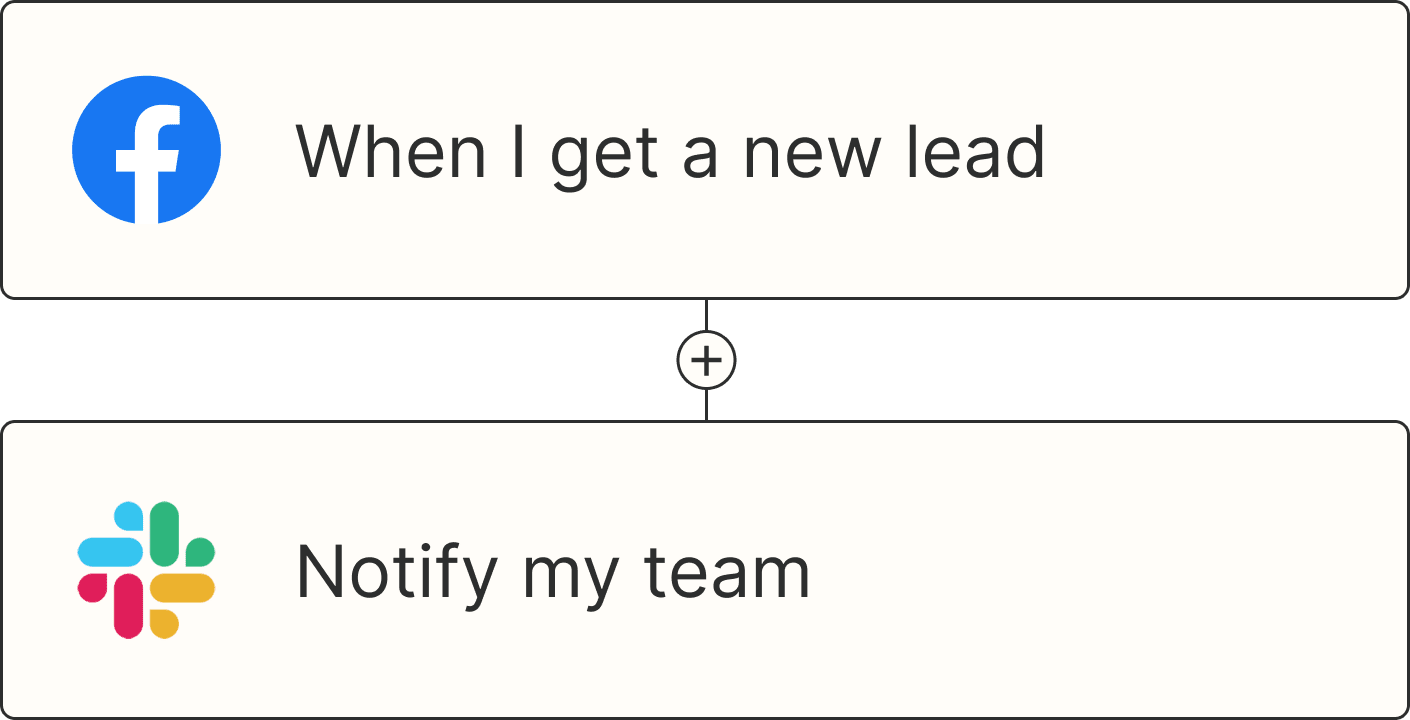
Pardon Our Interruption
As you were browsing something about your browser made us think you were a bot. There are a few reasons this might happen:
- You've disabled JavaScript in your web browser.
- You're a power user moving through this website with super-human speed.
- You've disabled cookies in your web browser.
- A third-party browser plugin, such as Ghostery or NoScript, is preventing JavaScript from running. Additional information is available in this support article .
To regain access, please make sure that cookies and JavaScript are enabled before reloading the page.
- Open access
- Published: 02 September 2024
Causal associations of hypothyroidism with frozen shoulder: a two-sample bidirectional Mendelian randomization study
- Bin Chen 1 ,
- Zheng-hua Zhu 1 ,
- Qing Li 2 ,
- Zhi-cheng Zuo 1 &
- Kai-long Zhou 1
BMC Musculoskeletal Disorders volume 25 , Article number: 693 ( 2024 ) Cite this article
Metrics details
Many studies have investigated the association between hypothyroidism and frozen shoulder, but their findings have been inconsistent. Furthermore, earlier research has been primarily observational, which may introduce bias and does not establish a cause-and-effect relationship. To ascertain the causal association, we performed a two-sample bidirectional Mendelian randomization (MR) analysis.
We obtained data on “Hypothyroidism” and “Frozen Shoulder” from Summary-level Genome-Wide Association Studies (GWAS) datasets that have been published. The information came from European population samples. The primary analysis utilized the inverse-variance weighted (IVW) method. Additionally, a sensitivity analysis was conducted to assess the robustness of the results.
We ultimately chose 39 SNPs as IVs for the final analysis. The results of the two MR methods we utilized in the investigation indicated that a possible causal relationship between hypothyroidism and frozen shoulder. The most significant analytical outcome demonstrated an odds ratio (OR) of 1.0577 (95% Confidence Interval (CI):1.0057–1.1123), P = 0.029, using the IVW approach. Furthermore, using the MR Egger method as a supplementary analytical outcome showed an OR of 1.1608 (95% CI:1.0318–1.3060), P = 0.017. Furthermore, the results of our sensitivity analysis indicate that there is no heterogeneity or pleiotropy in our MR analysis. In the reverse Mendelian analysis, no causal relationship was found between frozen shoulders and hypothyroidism.
Our MR analysis suggests that there may be a causal relationship between hypothyroidism and frozen shoulder.
Peer Review reports
Frozen shoulder, also known as adhesive capsulitis, is a common shoulder condition. Patients with frozen shoulder usually experience severe shoulder pain and diffuse shoulder stiffness, which is usually progressive and can lead to severe limitations in daily activities, especially with external rotation of the shoulder joint [ 1 ]. The incidence of the disease is difficult to ascertain because of its insidious onset and the fact that many patients do not choose to seek medical attention. It is estimated to affect about 2% to 5% of the population, with women affected more commonly than men (1.6:1.0) [ 2 , 3 ]. The peak occurrence of frozen shoulder is typically between the ages of 40 and 60, with a positive family history present in around 9.5% of cases [ 4 ]. However, the underlying etiology and pathophysiology of frozen shoulder remains unclear.
The prevalence of frozen shoulder has been reported to be higher in certain diseases such as dyslipidemia [ 5 ], diabetes [ 6 , 7 ], and thyroid disorders [ 4 , 8 ]. The relationship between diabetes and frozen shoulder has been established through epidemiological studies [ 9 , 10 , 11 ]. However, the relationship between thyroid disease and frozen shoulder remains unclear. Thyroid disorders include hyperthyroidism, hypothyroidism, thyroiditis, subclinical hypothyroidism, and others. Previously, some studies reported the connection between frozen shoulders and thyroid dysfunction. However, the conclusions of these studies are not consistent [ 4 , 12 , 13 , 14 , 15 , 16 ]. In addition, these studies are primarily observational and susceptible to confounding variables. Traditional observational studies can only obtain correlations, not exact causal relationships [ 17 ].
MR is a technique that utilizes genetic variants as instrumental variables (IVs) of exposure factors to determine the causal relationship between exposure factors and outcomes [ 17 , 18 ]. MR operates similarly to a randomized controlled trial as genetic variants adhere to Mendelian inheritance patterns and are randomly distributed in the population [ 19 ]. Moreover, alleles remain fixed between individuals and are not influenced by the onset or progression of disease. Consequently, causal inferences derived from MR analyses are less susceptible to confounding and reverse causality biases [ 20 , 21 ]. And with the growing number of GWAS data published by large consortia, MR studies can provide reliable results with a sufficient sample size [ 22 ]. In this study, we performed a two-sample bidirectional MR analysis to evaluate the causal relationship between hypothyroidism and frozen shoulder.
Study design description
The bidirectional MR design, which examines the relationship between hypothyroidism and frozen shoulder, is succinctly outlined in Fig. 1 . Using summary data from Genome-Wide Association Studies (GWAS) datasets, we conducted two MR analyses to explore the potential reciprocal association between hypothyroidism and frozen shoulder. In the reverse MR analyses, Frozen Shoulder was considered as the exposure and Hypothyroidism as the outcome, while the forward MR analyses focused on Hypothyroidism as the exposure. Figure 1 illustrates the key assumptions of the MR analysis.
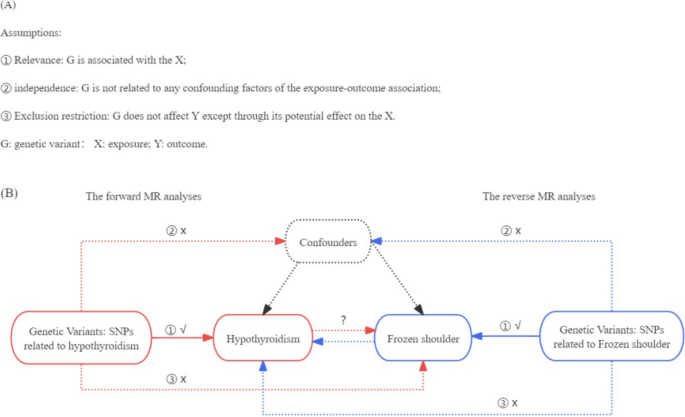
Description of the study design in this bidirectional MR study. A MR analyses depend on three core assumptions. B Research design sketches
Data source
Genetic variants associated with Hypothyroidism were extracted from published Summary-level GWAS datasets provided by the FinnGen Consortium, using the “Hypothyroidism” phenotype in this study. The GWAS included 16380353 subjects, including 22997 cases and 175475 controls. Data for Frozen Shoulder were obtained from the GWAS, which was derived from a European sample [ 23 ]. The frozen shoulder was defined based on the occurrence of one or more International Classification of Disease, 10th Revision (ICD10) codes (as shown in the supplementary material). Our MR study was conducted using publicly available studies or shared datasets and therefore did not require additional ethical statements or consent.
Selection of IV
For MR studies to yield reliable results, they must adhere to three fundamental assumptions [ 24 ], Regarding the IV selection, the following statements hold true: (1) IVs exhibit substantial correlation with exposure factors; (2) IVs do not directly impact outcomes but influence outcomes through exposure; (3) IVs are not correlated with any confounding factors that could influence exposure and outcome. Firstly, we selected single‐nucleotide polymorphisms (SNPs) from the European GWAS that met the genome-wide significance criterion ( p < 5 × 10 –8 ) and were associated with the exposure of interest as potential SNPs. Subsequently, we excluded any selected SNPs that linkage disequilibrium (LD) using the clump function (r 2 = 0.001, kb = 10000). Furthermore, palindromic SNPs and ambiguous SNPs were excluded. These excluded SNPs were not included in subsequent analyses. To evaluate weak instrumental variable effects, we utilized the F-statistic, considering genetic variants with an F-statistic < 10 as weak IVs and excluding them. Then for the second assumption, we needed to manually remove SNPs associated with outcome ( p < 5 × 10 –8 ). For the third assumption, “ IVs are not correlated with any confounding factors that could influence exposure and outcome,” implying that the IVs chosen should not have horizontal pleiotropy. The final set of SNPs meeting these criteria were utilized as IVs in the subsequent MR analysis.
MR analysis
In this study, we evaluated the relationship between hypothyroidism and frozen shoulder using two different MR methods: IVW [ 25 ] and MR-Egger regression [ 26 ]. The Wald ratio for each IV will be meta-analyzed using the IVW approach to investigate the causal relationship. In contrast to the MR-Egger technique, which remains functional even in the presence of invalid IVs, the IVW method assumes that all included genetic variants are valid instrumental variables. Furthermore, MR-Egger incorporates an intercept term to examine potential pleiotropy. If this intercept term equals 0 ( P > 0.05), the results of the MR-Egger regression model closely align with those obtained from IVW; However, if the intercept term deviates significantly from 0 ( P < 0.05), it suggests possible horizontal pleiotropy associated with these IVs. MR-Egger employed as estimation method alongside IVW. Although less efficient, these approaches can provide reliable predictions across a broader range of scenarios.
Sensitivity analysis
We performed a sensitivity analysis to investigate potential horizontal pleiotropy and heterogeneity in our study, aiming to demonstrate the robustness of our findings. Cochran’s Q test was employed to identify possible heterogeneity. Cochran’s Q statistic assessed genetic variant heterogeneity while considering significance at p < 0.05 level and I 2 > 25% as an indication of heterogeneity. on the results, we generated funnel plots. MR-Egger intercept tests were then utilized to estimate horizontal pleiotropy (with presence of an intercept and horizontal pleiotropy considered when p < 0.05). Additionally, a leave-one-out to determine if causality depended on or was influenced by any specific SNP. All statistical analyses were performed using the “TwoSampleMR” packages in R (version 3.6.3, www.r-project.org/ ) [ 27 ].
Instrumental variables
We ultimately chose 39 SNPs as IVs for the final analysis after going through the aforementioned screening process. All IVs had an F-statistic > 10, indicating a low probability of weak IV bias. Comprehensive information on each IV can be found in Appendix 1 .
Mendelian randomization results
According to the outcomes of the two MR techniques we employed for our analysis, hypothyroidism increases the risk factors for developing frozen shoulder. Specifically, as shown in the results of Table 1 , the primary analytical outcome using the IVW method revealed an OR of 1.0577 (95% CI:1.0057–1.1123), P = 0.029. Additionally, employing the MR Egger method secondary analytical outcome resulted in an OR of 1.1608 (95% CI:1.0318–1.3060), P = 0.017. Furthermore, scatter plots (Fig. 2 ) and forest plots (Fig. 3 ) were generated based on the findings of this MR study.
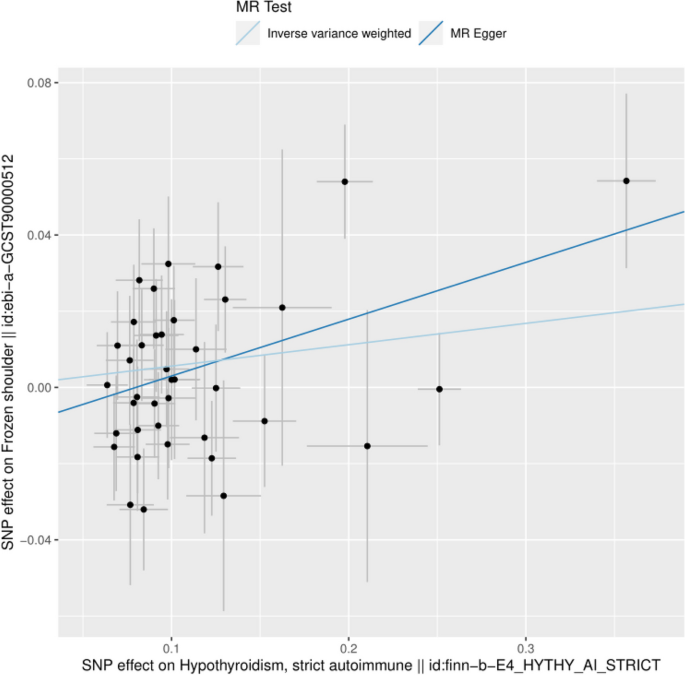
Scatterplot of MR analysis
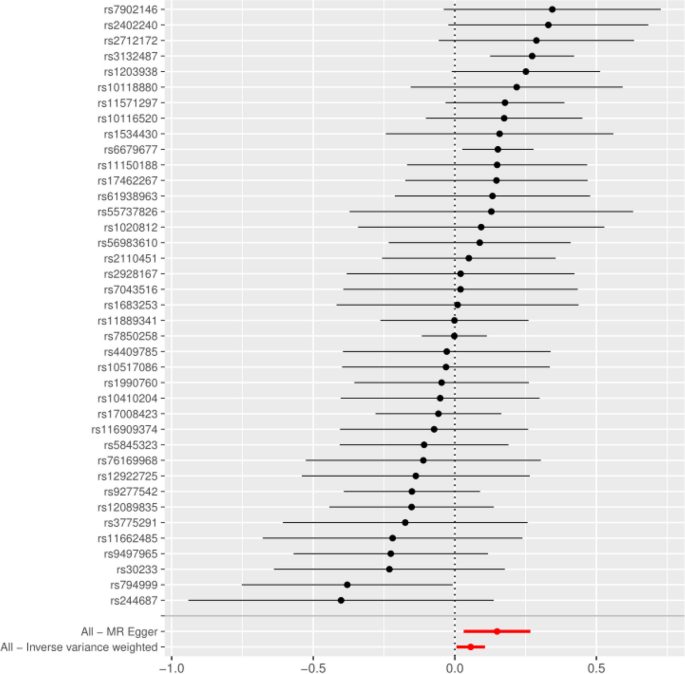
Forest plot of MR analysis
Heterogeneity and sensitivity test
The heterogeneity of causal estimates obtained for each SNP reflects their variability. A lower level of heterogeneity indicates higher reliability of MR estimates. To further validate the dependability of the results, we conducted a sensitivity analysis to examine the heterogeneity in MR. The funnel plots we created are displayed in Fig. 4 together with the results of Cochran’s Q test (Table 2 ), which revealed no heterogeneity in IVs. Additionally, the MR-Egger intercept test results (p = 0.0968) indicated no presence of pleiotropy in our data. Furthermore, the outcomes leave-one-out test demonstrated that causation remained independent and unaffected by any specific SNP (Fig. 5 ).
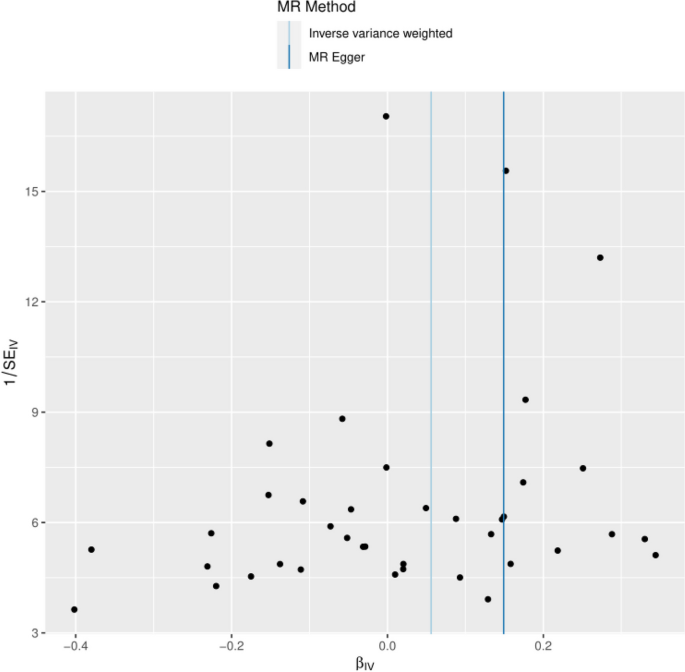
Funnel plot to assess heterogeneity
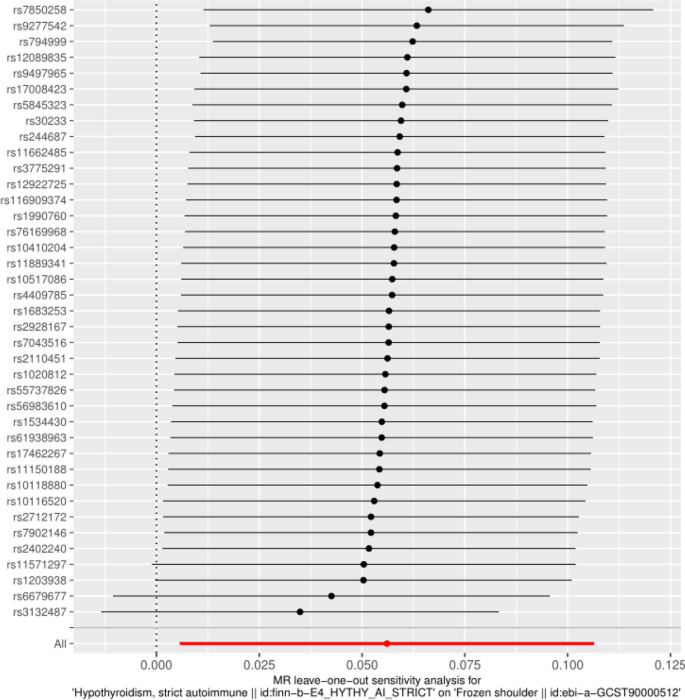
Sensitivity analysis by the leave-one-out method
Reverse Mendelian randomization analysis
In the reverse two-sample MR analysis, frozen shoulder was chosen as the exposure factor, and hypothyroidism as the outcome factor. The same threshold was set, and chain imbalance was eliminated. Finally, four SNPs were included as IVs in the reverse MR analysis. None of the four results from the MR analysis support a causal relationship between genetic susceptibility to frozen shoulder and the risk of hypothyroidism, as shown in Table 3 .
The frequent shoulder ailment known as frozen shoulder is characterized by joint pain and dysfunction. It has a significant negative impact on patient’s quality of life and increases the financial strain on families and society. Frozen shoulder can be caused by various factors, with thyroid disorders being one of them, although the exact causal relationship between them remains unclear.
There is considerable debate over whether hypothyroidism enhances the prevalence of frozen shoulder in the population. Results from Carina Cohen et al. [ 4 ] indicate that thyroid disorders, particularly hypothyroidism and the presence of benign thyroid nodules, significantly contribute to the risk of developing frozen shoulder. These factors increase the likelihood of acquiring the condition by 2.69 times [ 4 ]. A case–control study conducted in China revealed that thyroid disease is associated with an elevated risk of developing frozen shoulder [ 14 ]. Hyung Bin Park et al. also discovered a notable association between subclinical hypothyroidism and frozen shoulder [ 16 ]. Consistent with previous studies, a case–control study from Brazil reported that patients with hypothyroidism were more likely to be diagnosed with frozen shoulder than comparable patients [ 28 ]. However, there are some inconsistencies. Kiera Kingston et al. [ 13 ] discovered hypothyroidism in 8.1% of individuals with adhesive capsulitis; however, this rate was lower than the 10.3% identified in the control population [ 13 ]. Hyung et al. concluded that there was no association between them [ 15 ]. Studies by Chris et al. also questioned the relationship between heart disease, high cholesterol and thyroid disease and frozen shoulder [ 29 ]. All of these studies, we discovered, had poor scores on the evidence-based medicine scale, were vulnerable to a wide range of confounding variables, and carried a number of significant risks of bias. Additionally, conventional observational studies only provide correlations rather than precise causal links.
To overcome this shortcoming, we performed the MR analysis. The results of the two MR methods examined in this study suggest a possible causal relationship between hypothyroidism and frozen shoulder. Importantly, no substantial heterogeneity or pleiotropy was observed in these findings. Our conclusions are similar to those of Deng et al. [ 30 ]. However, our study conducted a reverse Mendelian randomization analysis and had a larger sample size. Several mechanisms may underlie this association. First, fibrosis plays a crucial role in the movement disorders associated with frozen shoulder. Hypothyroidism impairs the synthesis and breakdown of collagen, elastic fibers, and polysaccharides within soft tissues, resulting in tissue edema and fibrosis, contributing to the development of frozen shoulder [ 31 ]. Second, hypothyroidism influences various signaling pathways including growth factors, the extracellular matrix, and calcium signaling, which can impact the differentiation and functionality of osteocytes, leading to bone degeneration and subsequently progressing to frozen shoulder [ 32 ]. Third, hypothyroidism can result in reduced nerve conduction velocity, nerve fiber degeneration, and neuritis, subsequently compromising the sensory and motor functions of nerves and elevating the risk of developing frozen shoulder [ 33 ]. The outcomes of the MR analysis can be used to screen potential risk factors in advance. Accordingly, people with hypothyroidism are more likely to develop frozen shoulder. It is suggested that clinicians should pay attention to the patients with shoulder discomfort when treating hypothyroidism, and provide some ideas for early intervention, which is beneficial to the prognosis of patients.
Our research has some advantages. Firstly, by employing the MR approach, confounding factors and reverse causality were carefully controlled, at least to a large extent. Secondly, our study relied on data derived from previously published GWAS studies, which boasted a substantial sample size and encompassed numerous genetic variants. Moreover, it is worth mentioning that we also used different methods to estimate the impacts, which improves the reliability of our results. However, our MR study still has limitations. First, there may be unobserved pleiotropy beyond vertical pleiotropy. In addition, the samples for this study were all from the European population. Research results based on race may limit their generalizations to other populations. Therefore, large-scale, multi ethnic clinical and basic research may be needed to validate these issues.
With the help of two Mendelian randomization studies, we found that there may be a causal relationship between hypothyroidism and frozen shoulder, and hypothyroidism may be associated with an increased risk of frozen shoulder. However, the exact mechanism remains to be elucidated. More research is required to investigate the underlying mechanisms of this causal relationship.
Availability of data and materials
The datasets used and/or analysed during the current study available from the corresponding author on reasonable request.
Abbreviations
- Mendelian randomization
Genome-Wide Association Studies
Inverse-Variance Weighted
Confidence Interval
Instrumental Variables
Single‐Nucleotide Polymorphisms
Linkage Disequilibrium
Neviaser AS, Neviaser RJ. Adhesive capsulitis of the shoulder. J Am Acad Orthop Surg. 2011;19(9):536–42. https://doi.org/10.5435/00124635-201109000-00004 .
Article PubMed Google Scholar
Hand C, Clipsham K, Rees JL, Carr AJ. Long-term outcome of frozen shoulder. J Shoulder Elbow Surg. 2008;17(2):231–6. https://doi.org/10.1016/j.jse.2007.05.009 .
Hsu JE, Anakwenze OA, Warrender WJ, Abboud JA. Current review of adhesive capsulitis. J Shoulder Elbow Surg. 2011;20(3):502–14. https://doi.org/10.1016/j.jse.2010.08.023 .
Cohen C, Tortato S, Silva OBS, Leal MF, Ejnisman B, Faloppa F. Association between Frozen Shoulder and Thyroid Diseases: Strengthening the Evidences. Rev Bras Ortop (Sao Paulo). 2020;55(4):483–9. https://doi.org/10.1055/s-0039-3402476 .
Sung CM, Jung TS, Park HB. Are serum lipids involved in primary frozen shoulder? A case-control study. J Bone Joint Surg Am. 2014;96(21):1828–33. https://doi.org/10.2106/jbjs.m.00936 .
Huang YP, Fann CY, Chiu YH, Yen MF, Chen LS, Chen HH, et al. Association of diabetes mellitus with the risk of developing adhesive capsulitis of the shoulder: a longitudinal population-based followup study. Arthritis Care Res (Hoboken). 2013;65(7):1197–202. https://doi.org/10.1002/acr.21938 .
Arkkila PE, Kantola IM, Viikari JS, Rönnemaa T. Shoulder capsulitis in type I and II diabetic patients: association with diabetic complications and related diseases. Ann Rheum Dis. 1996;55(12):907–14. https://doi.org/10.1136/ard.55.12.907 .
Article CAS PubMed PubMed Central Google Scholar
Bowman CA, Jeffcoate WJ, Pattrick M, Doherty M. Bilateral adhesive capsulitis, oligoarthritis and proximal myopathy as presentation of hypothyroidism. Br J Rheumatol. 1988;27(1):62–4. https://doi.org/10.1093/rheumatology/27.1.62 .
Article CAS PubMed Google Scholar
Ramirez J. Adhesive capsulitis: diagnosis and management. Am Fam Physician. 2019;99(5):297–300.
PubMed Google Scholar
Wagner S, Nørgaard K, Willaing I, Olesen K, Andersen HU. Upper-extremity impairments in type 1 diabetes: results from a controlled nationwide study. Diabetes Care. 2023;46(6):1204–8. https://doi.org/10.2337/dc23-0063 .
Juel NG, Brox JI, Brunborg C, Holte KB, Berg TJ. Very High prevalence of frozen shoulder in patients with type 1 diabetes of ≥45 years’ duration: the dialong shoulder study. Arch Phys Med Rehabil. 2017;98(8):1551–9. https://doi.org/10.1016/j.apmr.2017.01.020 .
Huang SW, Lin JW, Wang WT, Wu CW, Liou TH, Lin HW. Hyperthyroidism is a risk factor for developing adhesive capsulitis of the shoulder: a nationwide longitudinal population-based study. Sci Rep. 2014;4:4183. https://doi.org/10.1038/srep04183 .
Kingston K, Curry EJ, Galvin JW, Li X. Shoulder adhesive capsulitis: epidemiology and predictors of surgery. J Shoulder Elbow Surg. 2018;27(8):1437–43. https://doi.org/10.1016/j.jse.2018.04.004 .
Li W, Lu N, Xu H, Wang H, Huang J. Case control study of risk factors for frozen shoulder in China. Int J Rheum Dis. 2015;18(5):508–13. https://doi.org/10.1111/1756-185x.12246 .
Park HB, Gwark JY, Jung J, Jeong ST. Association between high-sensitivity C-reactive protein and idiopathic adhesive capsulitis. J Bone Joint Surg Am. 2020;102(9):761–8. https://doi.org/10.2106/jbjs.19.00759 .
Park HB, Gwark JY, Jung J, Jeong ST. Involvement of inflammatory lipoproteinemia with idiopathic adhesive capsulitis accompanying subclinical hypothyroidism. J Shoulder Elbow Surg. 2022;31(10):2121–7. https://doi.org/10.1016/j.jse.2022.03.003 .
Lawlor DA, Harbord RM, Sterne JA, Timpson N, Davey Smith G. Mendelian randomization: using genes as instruments for making causal inferences in epidemiology. Stat Med. 2008;27(8):1133–63. https://doi.org/10.1002/sim.3034 .
Smith GD, Ebrahim S. ‘Mendelian randomization’: can genetic epidemiology contribute to understanding environmental determinants of disease? Int J Epidemiol. 2003;32(1):1–22. https://doi.org/10.1093/ije/dyg070 .
He Y, Zheng C, He MH, Huang JR. The causal relationship between body mass index and the risk of osteoarthritis. Int J Gen Med. 2021;14:2227–37. https://doi.org/10.2147/ijgm.s314180 .
Article PubMed PubMed Central Google Scholar
Evans DM, Davey Smith G. Mendelian randomization: new applications in the coming age of hypothesis-free causality. Annu Rev Genomics Hum Genet. 2015;16:327–50. https://doi.org/10.1146/annurev-genom-090314-050016 .
Burgess S, Butterworth A, Malarstig A, Thompson SG. Use of Mendelian randomisation to assess potential benefit of clinical intervention. BMJ. 2012;345:e7325. https://doi.org/10.1136/bmj.e7325 .
Li MJ, Liu Z, Wang P, Wong MP, Nelson MR, Kocher JP, et al. GWASdb v2: an update database for human genetic variants identified by genome-wide association studies. Nucleic Acids Res. 2016;44(D1):D869–76. https://doi.org/10.1093/nar/gkv1317 .
Green HD, Jones A, Evans JP, Wood AR, Beaumont RN, Tyrrell J, et al. A genome-wide association study identifies 5 loci associated with frozen shoulder and implicates diabetes as a causal risk factor. PLoS Genet. 2021;17(6):e1009577. https://doi.org/10.1371/journal.pgen.1009577 .
Burgess S, Davey Smith G, Davies NM, Dudbridge F, Gill D, Glymour MM, et al. Guidelines for performing Mendelian randomization investigations: update for summer 2023. Wellcome Open Res. 2019;4:186. https://doi.org/10.12688/wellcomeopenres.15555.3 .
Burgess S, Butterworth A, Thompson SG. Mendelian randomization analysis with multiple genetic variants using summarized data. Genet Epidemiol. 2013;37(7):658–65. https://doi.org/10.1002/gepi.21758 .
Bowden J, Del Greco MF, Minelli C, Davey Smith G, Sheehan NA, Thompson JR. Assessing the suitability of summary data for two-sample Mendelian randomization analyses using MR-Egger regression: the role of the I2 statistic. Int J Epidemiol. 2016;45(6):1961–1974. https://doi.org/10.1093/ije/dyw220 .
Hemani G, Zheng J, Elsworth B, Wade KH, Haberland V, Baird D, et al. The MR-Base platform supports systematic causal inference across the human phenome . Elife. 2018;7. https://doi.org/10.7554/eLife.34408 .
Schiefer M, Teixeira PFS, Fontenelle C, Carminatti T, Santos DA, Righi LD, et al. Prevalence of hypothyroidism in patients with frozen shoulder. J Shoulder Elbow Surg. 2017;26(1):49–55. https://doi.org/10.1016/j.jse.2016.04.026 .
Smith CD, White WJ, Bunker TD. The associations of frozen shoulder in patients requiring arthroscopic capsular release. Should Elb. 2012;4(2):87–9. https://doi.org/10.1111/j.1758-5740.2011.00169.x .
Article Google Scholar
Deng G, Wei Y. The causal relationship between hypothyroidism and frozen shoulder: A two-sample Mendelian randomization. Medicine (Baltimore). 2023;102(43):e35650. https://doi.org/10.1097/md.0000000000035650 .
Pandey V, Madi S. Clinical guidelines in the management of frozen shoulder: an update! Indian J Orthop. 2021;55(2):299–309. https://doi.org/10.1007/s43465-021-00351-3 .
Zhu S, Pang Y, Xu J, Chen X, Zhang C, Wu B, et al. Endocrine regulation on bone by thyroid. Front Endocrinol (Lausanne). 2022;13:873820. https://doi.org/10.3389/fendo.2022.873820 .
Baksi S, Pradhan A. Thyroid hormone: sex-dependent role in nervous system regulation and disease. Biol Sex Differ. 2021;12(1):25. https://doi.org/10.1186/s13293-021-00367-2 .
Download references
Acknowledgements
Not applicable.
This study was supported by the Project of State Key Laboratory of Radiation Medicine and Protection, Soochow University (No. GZK12023047).
Author information
Authors and affiliations.
Department of Orthopaedics, The Second Affiliated Hospital of Soochow University, Suzhou, China
Bin Chen, Zheng-hua Zhu, Zhi-cheng Zuo & Kai-long Zhou
State Key Laboratory of Radiation Medicine and Protection, Soochow University, Suzhou, 215123, China
You can also search for this author in PubMed Google Scholar
Contributions
BC: designed research, performed research, collected data, analyzed data, wrote paper. Zh Z, QL and Zc Z: collected data and verification results. Kl Z: designed research and revised article.
Corresponding author
Correspondence to Kai-long Zhou .
Ethics declarations
Ethics approval and consent to participate.
Because the study was based on a public database, did not involve animal or human studies, and was available in the form of open access and anonymous data, Institutional Review Board approval was not required.
Consent for publication
Competing interests.
The authors declare no competing interests.
Additional information
Publisher’s note.
Springer Nature remains neutral with regard to jurisdictional claims in published maps and institutional affiliations.
Supplementary Information
Supplementary material 1., supplementary material 2., rights and permissions.
Open Access This article is licensed under a Creative Commons Attribution-NonCommercial-NoDerivatives 4.0 International License, which permits any non-commercial use, sharing, distribution and reproduction in any medium or format, as long as you give appropriate credit to the original author(s) and the source, provide a link to the Creative Commons licence, and indicate if you modified the licensed material. You do not have permission under this licence to share adapted material derived from this article or parts of it. The images or other third party material in this article are included in the article’s Creative Commons licence, unless indicated otherwise in a credit line to the material. If material is not included in the article’s Creative Commons licence and your intended use is not permitted by statutory regulation or exceeds the permitted use, you will need to obtain permission directly from the copyright holder. To view a copy of this licence, visit http://creativecommons.org/licenses/by-nc-nd/4.0/ .
Reprints and permissions
About this article
Cite this article.
Chen, B., Zhu, Zh., Li, Q. et al. Causal associations of hypothyroidism with frozen shoulder: a two-sample bidirectional Mendelian randomization study. BMC Musculoskelet Disord 25 , 693 (2024). https://doi.org/10.1186/s12891-024-07826-y
Download citation
Received : 03 October 2023
Accepted : 28 August 2024
Published : 02 September 2024
DOI : https://doi.org/10.1186/s12891-024-07826-y
Share this article
Anyone you share the following link with will be able to read this content:
Sorry, a shareable link is not currently available for this article.
Provided by the Springer Nature SharedIt content-sharing initiative
- Frozen shoulder
- Hypothyroidism
BMC Musculoskeletal Disorders
ISSN: 1471-2474
- General enquiries: [email protected]
- Open access
- Published: 04 September 2024
In-depth analysis of Bt cotton adoption: farmers' opinions, genetic landscape, and varied perspectives—a case study from Pakistan
- Shahzad Rahil ORCID: orcid.org/0000-0002-4111-5037 1 ,
- Jamil Shakra 1 ,
- Chaudhry Urooj Fatima 1 ,
- Rahman Sajid Ur 1 &
- Iqbal Muhammad Zaffar 1 , 2
Journal of Cotton Research volume 7 , Article number: 31 ( 2024 ) Cite this article
Metrics details
Bt technology has played significant role in controlling bollworms and increasing cotton yield in earlier days of its introduction, a subsequent decline in yield became apparent over time. This decline may be attributed to various environmental factors, pest dynamics, or combination of both. Therefore, the present biophysical survey and questionnaire were designed to evaluate the impact of Bt cotton on bollworms management and its effect on reducing spray costs, targeting farmers with varied landholdings and educational backgrounds. Additionally, data on farmers' cultivated varieties and the prevalence of bollworms and sucking insects in their fields were recorded. Subsequently, about eleven thousand cotton samples from farmer fields were tested for Cry1Ac , Cry2Ab and Vip3A genes by strip test.
In this analysis, 83% of the farmers planting approved varieties believe that Bt technology control bollworms, while 17% hold contradictory views. Similarly, among farmers cultivating unapproved varieties, 77% agree on effectiveness of Bt technology against bollworms, while 23% disagree. On the other hand, 67% of farmers planting approved varieties believe that Bt technology does not reduce spray costs, while 33% agree with the effectiveness. Similarly, 78% of farmers cultivating unapproved varieties express doubt regarding its role to reduce spray costs, while 22% are in favour of this notion. Differences in opinions on the effectiveness of Bt cotton in controlling bollworms and reducing spray cost between farmers planting unapproved and approved varieties may stem from several factors. One major cause is the heavy infestation of sucking insects, which is probably due to the narrow genetic variation of the cultivated varieties. Additionally, the widespread cultivation of unapproved varieties (21.67%) is also an important factor to cause different opinions on the effectiveness of Bt cotton.
Based on our findings, we propose that the ineffective control of pests on cotton crop may be attributed to large scale cultivation of unapproved varieties and non-inclusion of double and triple transgene technologies in country’s sowing plan. On the basis of our findings, we suggest cotton breeders, regulatory bodies and legislative bodies to discourage the cultivation of unapproved varieties and impure seed. Moreover, the adoption of double and triple Bt genes in cottons with a broad genetic variation could facilitate the revival of the cotton industry, and presenting a promising way forward.
Cotton ( Gossypium hirsutum L.) is an important fibre crop also known as ‘White Gold’ (Ali et al. 2020 ; Jarwar et al. 2019 ). Pakistan earns major part of foreign exchange from cotton crop which contributes significantly towards economy. Pakistan is the 5 th largest cotton producer and 3 rd larger cotton consuming country in the world. It is an important crop for both agriculture and textile industries, and contributes about 0.6% of GDP and 3.1% of value addition in agriculture sector (Ministry of Finance, Government of Pakistan 2023 ). Over the time, cotton production in Pakistan has declined, due to seed adulteration, ineffective use of fertilizers and pesticides, labour mismanagement, unfavourable weather conditions, and irregular input supplies (Ali et al. 2019 ).
Since the introduction of synthetic insecticides, cotton producers relied heavily on those products to control insect pests. Certain factors i.e., insect resistance, secondary pest outbreaks, and pest resurgences cause an increasing application of synthetic insecticides (Trapero et al. 2016 ). The bollworms ( Heliothis and Helicoverpa spp.) and sucking insects ( Bemisia tabaci , Empoasca spp.) developed resistance to traditional pesticides during the 1990’s (Spielman et al. 2017 ). Afterwards, genetically modified (GM) cotton expressing Bacillus thuringiensis (Bt) toxin was introduced to control lepidopteran pests (Jamil et al., 2021a , b ). Resultantly, bollworms which have developed resistance against insecticides were effectively controlled and pesticide use was significantly reduced (Ahmad et al. 2019 ).
First official approval for general cultivation of Bt cotton in Pakistan was granted in 2010 by National Biosafety Committee within the Pakistan Environmental Protection Agency. However, substantial evidence shows cultivation of Bt cotton at farmers field prior to its official approval (Ahmad et al. 2021 ; Almas et al. 2023 ; Razzaq et al. 2021 ), which are Cry1Ac (first-generation cry gene) based and are primarily resistant to lepidopteran pests. In the earlier days of its introduction, the adoption of Bt technology led to a notable surge in cotton production, increasing from 8.7 million bales in 1999 to 14.61 million bales during 2004–2005, within just five to six years period (Rehman et al. 2019 ). Initially, both approved Bt varieties and unapproved ones showed inconsistent and potentially ineffective transgene expression due to the ineffective regulatory system overseeing commercialization of transgenic variety, releasing of new variety and distribution of seed of approved varieties (Ahmad et al. 2019 ). These loopholes in the system, combined with the challenges farmers face in visually assessing varieties genuineness and seed quality during purchase have contributed to the proliferation of spurious or low-quality seeds (Ali et al. 2019 ; Spielman et al. 2017 ).
Now, the area under Bt cotton cultivation is shrinking and the yield is decreased due to increased insect pest infestations (Arshad et al. 2021 ) owing to field evolved resistance in insects (Jaleel et al. 2020 ; Lei et al. 2021 ). Technologically advanced countries like the USA have addressed this issue of insect resistance development by adopting non-Bt cotton refuge systems and pyramiding multiple toxin genes ( Cry1Ac , Cry2Ab , and Vip3A ). However, in developing countries like China, India, and Pakistan, similar strategies were not effectively implemented, causing the field-evolved resistance in bollworms to proliferate (Jamil et al., 2021a , b ; Karthik et al. 2021 ). Another issue faced by farmers planted Bt cotton is increased infestation of sucking pests due to reduced use of pesticides (Ali et al. 2019 ; Shekhawat and Hasmi 2023 ). Hence, it is believed that interplay of various factors i.e. increased insect pest infestation, field evolved resistance, cultivation of unapproved and substandard seeds and adverse weather conditions result in huge loss of cotton production from 14 million bales in 2004–2005 to 4.91 million bales in 2023 (Ministry of Finance, Government of Pakistan 2023 ).
Keeping in view of the above mentioned facts, a survey was designed to evaluate the impact of Bt technology on cotton production across fifteen core cotton growing districts of Punjab, Pakistan and to understand the multifaceted factors affecting cotton production and to find out the root cause of declining of cotton production. In total 400 farmers possessing various landholding and educational background were surveyed to document their views on Bt. cotton's efficacy against bollworms and spray cost. Additionally, 10,986 cotton samples were tested at farmer’s field through strip tests to assess the purity of cotton varieties with respect to Bt ( Cry1Ac , Cry2Ab and Vip3A) genes.
Present study was conducted at Agricultural Biotechnology Research Institute, Ayub Agricultural Research Institute, Faisalabad 38000, Punjab, Pakistan.
Survey site
The survey was carried out in core cotton growing area of Pakistan, Punjab province. The Punjab is further divided into 36 administrative units called “districts” that vary significantly in cotton production. Out of 36 districts, fifteen were selected, i.e. Faisalabad, Toba Tek Singh, Sahiwal, Pakpattan, Multan, Lodhran, Khanewal, Vehari, Muzaffargarh, Layyah, D.G. Khan, Rajanpur, Bahawalpur, R.Y. Khan and Bahawalnagar, on the basis of acreage under cotton cultivation as outlined in AMIS.PK ( http://www.amis.pk/Agristatistics/DistrictWise/DistrictWiseData.aspx ). Subsequently, 400 farmer fields were selected from all “Tehsils” (sub-administrative unit) with various landholdings and diverse educational backgrounds particularly in the regions with intensive Bt cotton cultivation. The GPS coordinates of each farmer’s location was recorded using Latitude-Longitude App (Financept) and listed in Table 1 .
Survey questionnaire
A structured questionnaire, comprising of six questions was designed to collect data regarding farmer’s demographic factors, farmers' landholdings and viewpoint about effectiveness of Bt technology in controlling cotton bollworms. The questions were: 1) farmers landholding, classified as small (0–10 acres), medium (11–50 acres) or large (above 50 acres). 2) farmers educational background, stratified into uneducated, below matric, matric, bachelor degree, and masters or above qualifications. 3) the efficacy of Bt cotton in controlling bollworms (Yes, No). 4) the role of Bt technology in reducing the frequency of pesticide sprays and respective pesticide cost to farmers (Yes, No). 5) the variety cultivated by farmers (Table S1). 6) insect infestations of i.e. jassid, whitefly, aphid, thrips, mites, American bollworm (AB) and pink bollworm (PB) (low, medium and high). Infestation levels (low, medium, or high) were based on the economic threshold level (ETL) of each insect species. Infestations below the ETL were classified as "low", those comparable to the ETL as "medium", and those exceeding the ETL as "high". The reference point ETLs for various insect species were as follows: jassid (1 nymph or adult per leaf), whitefly (5 adults per leaf), thrips (8–10 adults per leaf), mites (2 adults per leaf), aphid (20 aphids per leaf), AB (4–5 eggs and larvae per 100 plants), and PB (8% infested bolls) (Ali et al. 2019 ; Razaq et al. 2019 ; Rehman et al. 2019 ).
Molecular analysis of Cry1Ac , Cry2Ab and Vip3A genes
Molecular analysis was performed through strip test for detection and identification of transgenes at four hundred farmer fields, and a total of 10986 samples were tested. At each field, a minimum of 25 samples were collected and tested, at least 10 samples were tested for each variety. Consequently, depending on the number of varieties cultivated by the farmers, more than 25 samples were tested in some fields. The strip tests were performed using QuickStix combo kits (EnviroLogix), which are equipped with built-in antibody coatings for the detection of Cry1Ac , Cry2Ab , and Vip3A transgenes. The procedure for strip test involved pressing the cap of a disposable eppendorf tube onto two leaves to obtain double leaf disc (weighing approximately 20 mg). Subsequently, the leaf samples were finely grinded with the help of disposable pestle by rubbing against the walls of eppendorf tube after adding 0.5 mL of 1X EB2 extraction buffer. The leaf extract and extraction buffer were homogenized by thorough mixing, ensuring the components were evenly combined for accurate and reliable downstream analysis. Following that, the QuickStix combo strips were dipped in eppendorf tube containing leaf extract with arrow pointing downward. After 10 min incubation, bands were developed on strips through antigen-antibody reaction and strips were analysed for the presence of final bands, and results were recorded (Jamil et al., 2021a , b ).
Data analysis
Frequency analysis of Cry1Ac , Cry2Ab , and Vip3A genes was performed using the “ dplyr ” package to streamline data manipulation and summarization. District-wise opinion of farmers on bollworm management and spray cost reduction were analysed using " tidyverse " functions. The association between Bt. technology adoption, farmers' landholding, and education was studied through a heatmap using the " heatmap.2 " function of " gplots " package in R software. The data regarding varieties cultivated by farmers in each district was analysed using stacked bar-chart illustrated by " ggplot2 " package of R software. Lastly, insect pest infestation data was analysed using " dplyr " and " ggplot2 " packages (Ross et al. 2017 ) and Chi-square (χ 2 ) test was performed to check the associations between different qualitative variables using “ chisq.test ” function in R software.
Survey design and farmers demographics
The purposive sampling technique was used assessing the viewpoint of farmers having diverse landholdings and differential educational backgrounds. Landholdings varied among districts showing distinct distribution of farmers with small, medium and large landholdings (Table 1 ). Notably, the highest proportion of large landholders was found in Sahiwal (43%) followed by Faisalabad (33%), Dera Ghazi Khan (31%) and Rajanpur (29%) district. In terms of medium landholders, district Rahim Yar Khan had the highest (74%), while district Layyah had the lowest (35%) proportions. Among small landholders, district Layyah displayed the highest (50%) while district Sahiwal having the lowest (7%) ratio. Overall, 60% of the farmers have medium, 18% owned small and 22% possessed large landholdings (Table 1 ).
Similarly, variability was observed among farmers on the basis of academic background (Table 2 ). The majority of farmers have completed matric (53%), 22% of farmers were below matric (22%), 12% farmers had bachelor degree, 7% farmers had master degree or above qualifications, and merely 6% farmers were uneducated. The district Sahiwal has highest ratio of uneducated farmers (22%) while highest proportion of farmers with below matric qualification was observed in district Dera Ghazi Khan (39%). Besides Dera Ghazi Khan, all other analysed districts have higher proportion of farmers with matric qualification, specifically, district Toba Tek Singh exhibited highest proportion (100%) followed by Pakpattan (70%). Furthermore, district Bahawalpur and district Layyah exhibited highest proportion of farmers among bachelor degree holders (25%) and master degree or above qualification (30%), respectively (Table 2 ).
Genetic landscape and cultivation patterns of varieties
The varieties planted at farmer fields were noted and verified based on tags issues by Federal Seed Certification and Registration Department (FSC&RD). The varieties planted at farmer fields were compared with the database of approved varieties from the government to identify the approved or unapproved variety. Overall, unapproved varieties were cultivated extensively covering significant area (21.67%). Moreover among approved varieties the top cultivating were IUB-13 (15.22%), BS-15 (12.61%), FH-142 (8.26%) and FH-Lalazar (8.04%). The lowest cultivated variety was MNH-886 (3.45%). In total of 7.27% area were cultivated with other approved varieties. The top 3 area cultivated unapproved cotton varieties were Bahawalnagar (40.73%), Layyah (38.24%), and Bahawalpur (32.40%). Conversely, unapproved variety was not found in Pakpattan or Toba Tek Singh (Fig. 1 ).
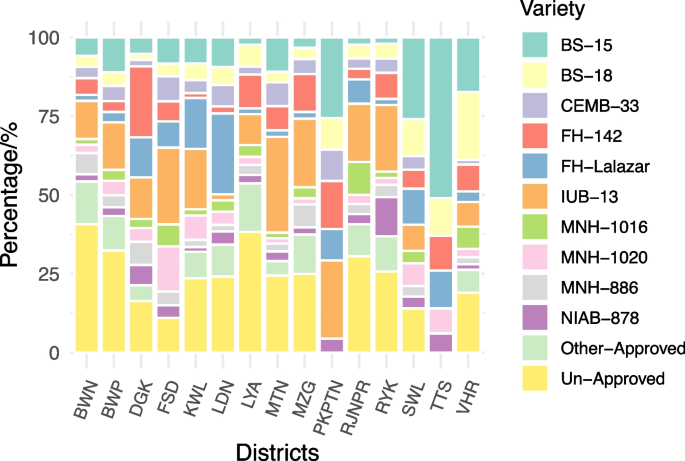
Stacked bar-chart showcasing varietal diversity across fifteen cotton growing districts of cotton belt of Punjab, Pakistan; BWN; Bahawalnagar, BWP; Bahawalpur, DGK; Dera Ghazi Khan, FSD; Faisalabad, KWL; Khanewal, LDN; Lodhran, LYA; Layyah, MTN; Multan, MZG; Muzaffargarh, PKPTN; Pakpattan, RJNPR; Rajanpur, RYK; Rahim Yar Khan, SWL; Sahiwal, TTS; Toba Tek Singh, VHR; Vehari
Analysing region-specific cultivation of varieties, it was observed that IUB-13 was the most cultivated variety in Bahawalnagar (12.09%), Bahawalpur (15.10%), Khanewal (19.18%), Multan (30.41%), Muzaffargarh (21.74%), Faisalabad (24.33%), Rahim Yar Khan (21.10%), and Rajanpur (18.49%). FH-142 was the preferred variety in DG Khan (22.51%) and Layyah (10.66%). FH-Lalazar was most commonly cultivated in Lodhran district (25.64%), while BS-18 dominated in Vehari (21.59%). Additionally, BS-15 was prominently cultivated in Toba Tek Singh (51%), Sahiwal (26.00%), and Pakpattan district (25.60%). Toba Tek Singh and Pakpattan districts have least diversity of cultivated varieties (Fig. 1 ).
Biochemical testing of Bt cotton
To understand the genetic landscape of cultivated varieties with respect to transgenes, strip tests were performed for detection and identification of Cry1Ac , Cry2Ab and Vip3A genes. Across fifteen districts, a total of 10,986 cotton samples were tested. The Cry1Ac gene was presented in varying degrees, with highest occurrence (100%) in district Lodhran, Sahiwal, Pakpattan and Toba Tek Singh. Other districts, such as Khanewal, Bahawalpur, Bahawalnagar, Faisalabad, Layyah, Multan, Rajanpur, Rahim Yar Khan and Vehari also reported more than 80% of Cry1Ac gene in farmer fields. In contrast, Dera Ghazi Khan and Muzaffargarh districts displayed relatively lower percentage of Cry1Ac gene, 69% and 78%, respectively (Table 3 ).
The Cry2Ab gene exhibited a relatively low (9%) percentage throughout the survey area, its frequency ranged from 0% in Pakpattan to 15% in Layyah and Toba Tek Singh districts. The frequency of Cry2Ab gene was no more than 10% in Bahawalnagar, Bahawalpur, Faisalabad, Khanewal, Lodhran, Muzaffargarh, Multan, Pakpattan, Rajanpur, Rahim Yar Khan and Sahiwal districts. Further, the third Bt gene Vip3A, which has broad spectrum resistance against lepidopteron pests, was not found in a single tested sample throughout the survey area. In summary, Cry2Ab gene was found throughout the cotton cultivation regions, except Pakpattan, but the percentage was much lower than Cry1Ac gene (Table 3 ).
Pest dynamics at farmers’ field
The pest counting was performed in the survey area for major cotton pests, i.e., AB, PB, whitefly, aphid, jassid, thrips, and mites. The PB infestation was medium level in more than 50% farmers' fields in most districts except Bahawalnagar, Der Ghazi Khan, Khanewal, Pakpattan and Vehari. Lodhran and Toba Tek Singh recorded 50% of field with low level of PB, whereas Pakpattan and Vehari recorded high level PB invasions at more than 50% fields. In case of AB, Lodhran, Muzaffargarh, Pakpattan and Toba Tek Singh exhibited low AB level at all fields. However, in Bahawalpur and Layyah, 14% and 20% fields experienced medium outbreak of AB, respectively. Notably, in Faisalabad, Layyah and Sahiwal districts, high infestation of AB was observed at 12%, 10% and 7% fields, respectively. On an average, 93% of fields from all survey regions observed low AB outbreak (Table 4 ).
The whitefly remains the predominant insect throughout the survey area with high outbreaks at 68% fields on the average. Five districts including Dera Ghazi Khan, Faisalabad, Muzaffargarh, Rajanpur, and Toba Tek Singh were whitefly hotspot areas, with all survey fields recorded high outbreak. Other districts like Bahawalpur, Khanewal, Multan, Layyah, Rahim Yar Khan, and Sahiwal exhibited diverse infestation patterns. Although aphid is one of the most concerned pest, 77% farmer fields reported low outbreak, particularly in Faisalabad, Sahiwal, Pakpattan, and Toba Tek Singh districts with all observed field recorded as low infestation. On the other hand, 64% fields at Bahawalpur and 44% at Muzaffargarh recorded medium level and 28% fields at district Rahim Yar Khan recorded high level outbreak (Table 4 ).
Apart from whitefly and aphid, jassid was another alarming threat in cotton production, showing high level of invasion at 62% farmer fields. The jassid outbreak in all fields of district Faisalabad, Muzaffargarh, Pakpattan, Rajanpur, Sahiwal, and Toba Tek Singh was at high level. The jassid infestation was also high in more than 70% fields of Bahawalnagar, Dera Ghazi Khan, and Vehari districts. The pest counting of mites revealed low infestation at 62% observed fields. Faisalabad and Pakpattan districts had low infestations in all fields, whereas Dera Ghazi Khan and Muzaffargarh districts recorded medium outbreak at 73% and 86% fields, and 50% fields of Toba Tek Singh recorded as high mites outbreak. The thrips outbreak was high at 60% farmer fields on the average, Bahawalpur, Khanewal, Lodhran, Muzaffargarh, Rajanpur, Toba Tek Singh and Vehari were recorded as high outbreak in 78%, 78%, 75%, 74%, 89%, 100% and 69% fields, respectively while all fileds in Faisalabad showed medium outbreak of thrips (Table 4 ).
Chi-square test on the associations between different factors
The Chi-square (χ 2 ) test was performed to check the association of 17 pairs of factors as detailed in Table 5 . The association of transgene with varieties was non-significant, which means the type of Bt cotton either single or double transgenic cultivars is not showing distinct correlation with the approved or unapproved varieties. Moreover, farmer’s education and landholding have no impact on transgene adoption. Furthermore, association of transgene was also non-significant with thrips which indicates that thrips equally affect non-Bt, single Bt gene, or double Bt gene cotton. However, association of transgene with AB, PB, whitefly, aphid, jassid and mite infestation was significant. This indicates that AB and PB attack vary with transgene and these are interlinked. Similarly, whitefly, aphid, jassid and mites infestation also vary on non-Bt., single and double gene Bt. cotton varieties (Table 5 , S2).
Likewise, association of varieties with AB, aphid and mite was non-significant which reveals that there is no statistical difference of AB, aphid and mite infestation between approved and unapproved varieties. On the contrary, association of varieties with PB, whitefly, jassid and thrips was significant, indicating that infestation of PB, whitefly, jassid and thrips vary among approved and unapproved varieties (Table 5 ).
Farmer’s opinion on Bt cotton
The farmer’s viewpoint on efficiency of Bt technology in controlling bollworms and reducing spray cost in cotton crop was analysed and it was observed that 83% of farmers cultivating approved varieties, believed in Bt cotton's effectiveness against bollworms, while 17% hold the contrary belief. However, variation exists in different district, i.e. farmers from Bahawalpur, Faisalabad, Rahim Yar Khan, and Sahiwal unanimously agreed (100%) on Bt cotton's effectiveness against bollworms. But 50% farmers in Bahawalnagar, 33% in Toba Tek Singh and some in other districts cultivating unapproved varieties were not convinced. On the other hand, 77% of farmer cultivated unapproved varieties have faith in Bt cotton usefulness for controlling bollworms, whereas 23% expressed disbelief. All farmers cultivating unapproved varieties in Bahawalpur, Faisalabad, Rahim Yar Khan, Sahiwal and Toba Tek Singh districts unanimously believed that Bt cotton is effective against bollworms. In contrary to that, 67% farmers in Multan, 43% in Dera Ghazi Khan, 37% each in Layyah and Muzaffargarh, 33% each in Lodhran and Rajanpur, 31% in Vehari, 23% in Bahawalnagar, and 8% in Khanewal cultivating unapproved varieties are not convinced about this claim. It is evident that farmers cultivating approved varieties express higher confidence in the effectiveness of bollworm control by Bt technology as compared to those cultivating unapproved varieties (Table 6 ).
Similarly, examining the impact of Bt cotton on spray cost reduction revealed a complex scenario. Among farmers planting approved varieties, 33% believed that Bt technology has reduced spray costs, while majority (67%) disagree. Particularly, farmers in districts Bahawalnagar, Dera Ghazi Khan, Faisalabad, Muzaffargarh, Rajanpur, Toba Tek Singh and Vehari unanimously disagreed that Bt technology reduced the spray costs, while all farmers at Bahawalpur, Rahim Yar Khan and Sahiwal have opposite views. Likewise, among farmers cultivating unapproved varieties, 22% express confidence in reducing spray costs by introduction of Bt technology, while 78% hold opposite perspective. In Pakpattan and Bahawalpur 100% of farmers growing unapproved varieties believe in reduction of spray costs, while in Multan, Faisalabad, Khanewal, Layyah, Lodhran, Muzaffargarh, Rajanpur, Sahiwal, Toba Tek Singh and Vehari, all farmers disagreed with this notion. Overall, the analysis highlighted diverse opinions among farmers about the impact of Bt cotton on spray cost reduction (Table 6 ).
In the midst of the changing agricultural technology and the persistent challenges faced by cotton farmers, our study delves into the dynamics surrounding the adoption and effectiveness of Bt cotton technology. With a focus on bollworm management and spray cost reduction, our research navigates through the perceptions and practices of farmers with diverse educational backgrounds and landholdings and revealed main factors affecting cotton farming. We unravel the complexities underlying farmer beliefs, technological advancements, and regulatory frameworks, aiming to chart a course towards sustainable solutions for the revitalization of the cotton crop.
We have approached farmers from all cotton growing districts of the Punjab with diverse backgrounds, i.e. possessing varying landholdings (Table 1 ) and different educational backgrounds (Table 2 ) to increase the reliability of the results (O'Connell et al. 2022 ). The farmers have been inquired about effectiveness of Bt technology against cotton bollworms and its impact on spray cost. Overall, 60% of the farmers have medium landholdings, 22% farmers owned large landholdings and 18% farmers possessed small landholdings (Table 1 ). Likewise, from education perspective, 53% farmers have matric, 22% farmers are below matric, 12% and 7% farmers have bachelor degree and master degree or above qualifications, whereas 6% farmers were uneducated (Table 2 ) representing a mixed population from each strata of education background and landholdings to obtain meaningful information (Swami and Parthasarathy 2020 ).
These farmers' opinion have been bifurcated into two categories based on cultivation of approved and unapproved varieties. The viewpoint of 83% of farmers cultivating approved varieties is that Bt cotton has controlled the bollworms effectively and 17% have opposite opinion. But among those cultivating unapproved varieties, 77% farmers think that bollworms have been controlled after introduction of Bt cotton and 23% farmers have opposite views (Table 6 ). These findings agrees with the study that both approved and unapproved varieties have significant Bt toxin protein level to control bollworms effectively (Spielman et al. 2017 ). Given that AB and PB infestation are dependent on transgenes (Table 5 ) and have an antagonistic relationship (Table S2), and considering that nearly all cultivated varieties (either approved or unapproved) were transgenic (Table S1), the use of these transgenic varieties is likely the primary factor in controlling bollworms (Kashif et al. 2022 ). Moreover, according to a previous study, unapproved varieties are as effective in controlling bollworms as approved varieties, both expressing transgenes at levels lethal to pests (Cheema et al. 2016 ). However, Jamil et al. ( 2021a , b ) have contradictory viewpoint and believe that, unapproved varieties are the leading cause of resistance due to low Bt. toxin level which providing ideal environment for field evolved resistance (Ahmad et al. 2019 ).
In the earlier years of Bt cotton introduction, farmers were largely convinced about its efficiency to control bollworm invasions as reported in different geographies (Gore et al. 2002 ; Kranthi et al. 2005 ) and Pakistan (Arshad et al. 2009 ). However, with the passage of time, without adoption of some levels of refuge plants (plantation of 10% non-Bt crop as refuge) fields have evolved resistance in bollworms (Shahid et al. 2021 ). The situation was further aggravated due to least or no adoption of double ( Cry1Ac and Cry2Ab ) and triple transgene ( Cry1Ac , Cry2Ab and Vip3A ) technologies (Table 3 ). The double and triple transgene cotton have broad-spectrum resistance by different mode of action and corresponding receptor sites in insect gut (Chen et al. 2017 ; Llewellyn et al. 2007 ). Particularly, the Vip3A gene provides broad-spectrum resistance by encoding Bt toxin that disrupts the digestive system upon ingestion, ultimately leading to insect death. Unlike Cry1Ac , Vip3A gene acts through a different mode of action, making it effective against pests that may have developed resistance to Cry1Ac . This diversity in toxin mechanisms helps enhance the overall efficacy of Bt cotton in managing pest populations and reducing crop damage (Chen et al. 2017 ). Some countries swiftly adopted double and triple gene technologies in the cultivation plan, while Pakistan continues to rely solely on the initially introduced single gene ( Cry1Ac ) Bt cotton, which result in the development of resistance in the field (Tabashnik et al. 2013 ; Tabashnik and Carrière 2017 ).
Analysis of farmers' perspective about the efficacy of Bt technology in reducing spray costs has revealed that more than 50% farmers from both categories (planting approved or unapproved varieties) believe that spray cost has not been reduced upon introduction of Bt technology. Specifically, 33% of farmers cultivating approved varieties affirmed that Bt technology effectively reduces spray costs, while 67% hold a contrary viewpoint. Conversely, among farmers planting unapproved varieties, a higher percentage (78%) of farmers have expressed suspicion regarding the effectiveness of Bt cotton in reducing spray costs, with only 22% supporting this notion (Table 6 ). Farmers hold different views on the effectiveness of Bt cotton against bollworms and its impact on spray costs. Majority of farmers claimed that Bt cotton has successfully controlled bollworms, while they also believe that the introduction of Bt cotton has not reduced spray costs. This is attributed to the increased pressure from sucking insect pests such as whitefly, aphid, jassid, thrips, and mites (Table 4 ), which has led to higher spray costs instead of the anticipated reduction. The sucking pest pressure has been increased after introduction of Bt genotypes owing to the low adaptation to local agro ecological conditions (Lu et al. 2022 ) and narrow genetic base (Jamil et al., 2021a , b ). Therefore, these varieties are more vulnerable to sucking pests compared to earlier genetically diverse varieties, thereby necessitats frequent pesticide spray and nullifys the anticipated reduction in spray costs (Arshad et al. 2009 ).
One significant factor influence farmers' believe on Bt technology is large scale cultivation of unapproved varieties (21.67% area). Particularly, in Bahawalnagar, Layyah and Bahawalpur districts (Fig. 1 ). This may be a leading cause in building farmers' perceptions about Bt. cotton's inefficiency to control bollworms and reducing spray costs, reflecting mismanagement rather than inherent flaws in the technology. Because, during the formal varietal approval process, varieties are passed through certain checks, i.e., disease & insect resistance, adaptability to different geographies, response to different climatic factors and genetic diversity from cultivated varieties (Ahmad et al. 2023a , b ; Iftikhar et al. 2019 ). However, if a variety escape through this process and reach farmers field merely on the basis of high yield, it may be susceptible to bollworms and sucking insects (Kranthi and Stone 2020 ). Furthermore, approved varieties may also have mixing of non-Bt seed as reported in one of our previous study (Jamil et al., 2021a , b ), supressing their genetic potential. Perhaps, all the factors explained above, underscores a deficiency on the part of cotton breeders (both public and private sectors) and regulatory bodies (such as FSC&RD), as they have not effectively regulated the supply of unapproved varieties to farmers, lacking proper check and legislative measures (Shahzad et al. 2022 ).
Different opinions among farmers on the effectiveness of Bt cotton may partly be due to cultivation of unapproved varieties. Moreover, least adoption of double and triple transgene technologies and excessive outbreaks of sucking insects particularly whitefly, jassid and thrips exacerbated the situation. To mitigate these challenges, concerted efforts from cotton breeders and regulatory bodies are imperative. Moreover, there is a need to promote and disseminate the latest Bt cotton technologies particularly Cry2Ab and Vip3A genes among farmers on large scale for dissemination of broad-spectrum resistance against bollworms.
Availability of data and materials
All data generated or analysed during this study are included in this published article.
Ahmad S, Cheema HMN, Khan AA, et al. Resistance status of Helicoverpa armigera against Bt cotton in Pakistan. Transgenic Res. 2019;28:199–212. https://doi.org/10.1007/s11248-019-00114-9 .
Article CAS PubMed Google Scholar
Ahmad J, Zulkiffal M, Anwar J, et al. MH-21, a novel high-yielding and rusts resistant bread wheat variety for irrigated areas of Punjab Pakistan. SABRAO J Breed Genet. 2023a;55(3):749–59. https://doi.org/10.54910/sabrao2023.55.3.13 .
Article Google Scholar
Ahmad J, Rehman A, Ahmad N, et al. Dilkash-20: a newly approved wheat variety recommended for Punjab, Pakistan with supreme yielding potential and disease resistance. SABRAO J Breed Genet. 2023b;55(2):298–308. https://doi.org/10.54910/sabrao2023.55.2.3 .
Ahmad S, Shahzad R, Jamil S, et al. Regulatory aspects, risk assessment, and toxicity associated with RNAi and CRISPR methods. In: Abd-Elsalam KA, Lim K, et al., editors. CRISPR and RNAi systems. Cambridge: Elsevier Inc.; 2021. p. 687–721. https://doi.org/10.1016/B978-0-12-821910-2.00013-8 .
Ali M, Kundu S, Alam M, et al. Selection of genotypes and contributing characters to improve seed cotton yield of upland cotton ( Gossypium hirsutum L.). Asian Res J Agri. 2020;13(1):31–41. https://doi.org/10.9734/arja/2020/v13i130095 .
Ali MA, Farooq J, Batool A, et al. Cotton production in Pakistan. In: Jabran K, Chauhan BS, et al., editors. Cotton production. Hoboken: John Wiley & Sons Ltd; 2019. p. 249–76. https://doi.org/10.1002/9781119385523.ch12 .
Almas HI, Azhar MT, Atif RM, et al. Adaptation of genetically modified crops in Pakistan. In: Nawaz MA, Chung G, Tsatsakis AM, et al., editors. GMOs and political stance. Cambridge: Elsevier Inc; 2023. p. 93–114. https://doi.org/10.1016/B978-0-12-823903-2.00002-0 .
Arshad M, Suhail A, Gogi MD, et al. Farmers’ perceptions of insect pests and pest management practices in Bt cotton in the Punjab, Pakistan. Int J Pest Manage. 2009;55(1):1–10. https://doi.org/10.1080/09670870802419628 .
Arshad A, Raza MA, Zhang Y, et al. Impact of climate warming on cotton growth and yields in China and Pakistan: a regional perspective. Agriculture. 2021;11(2):97. https://doi.org/10.3390/agriculture11020097 .
Article CAS Google Scholar
Cheema H, Khan A, Khan M, et al. Assessment of Bt cotton genotypes for the Cry1Ac transgene and its expression. J Agri Sci. 2016;154(1):109–17. https://doi.org/10.1017/S0021859615000325 .
Chen WB, Lu GQ, Cheng HM, et al. Transgenic cotton coexpressing Vip3A and Cry1Ac has a broad insecticidal spectrum against lepidopteran pests. J Invertebr Pathol. 2017;149:59–65. https://doi.org/10.1016/j.jip.2017.08.001 .
Gore J, Leonard B, Church G, et al. Behavior of bollworm (Lepidoptera: Noctuidae) larvae on genetically engineered cotton. J Econ Entomol. 2002;95(4):763–9. https://doi.org/10.1603/0022-0493-95.4.763 .
Ministry of Finance, Government of Pakistan. Agriculture. In: Economic survey of Pakistan. Ministry of Finance, Government of Pakistan. 2023. p. 19–30.
Iftikhar MS, Talha GM, Shahzad R, et al. Early response of cotton ( Gossypium hirsutum L.) genotype against drought stress. Inter J Biosci. 2019;14(2):537–44. https://doi.org/10.12692/ijb/14.2.536-543 .
Jaleel W, Saeed S, Naqqash MN, et al. Effects of temperature on baseline susceptibility and stability of insecticide resistance against Plutella xylostella (Lepidoptera: Plutellidae) in the absence of selection pressure. Saudi J Biol Sci. 2020;27(1):1–5. https://doi.org/10.1016/j.sjbs.2019.03.004 .
Jamil S, Shahzad R, Rahman SU, et al. The level of Cry1Ac endotoxin and its efficacy against H. armigera in Bt cotton at large scale in Pakistan. GM Crops & Food. 2021a;12(1):1–17. https://doi.org/10.1080/21645698.2020.1799644 .
Jamil S, Shahzad R, Iqbal MZ, et al. DNA fingerprinting and genetic diversity assessment of GM cotton genotypes for protection of plant breeders rights. Int J Agric Biol. 2021b;25(4):768–76. https://doi.org/10.17957/IJAB/15.1728 .
Jarwar AH, Wang X, Iqbal MS, et al. Genetic divergence on the basis of principal component, correlation and cluster analysis of yield and quality traits in cotton cultivars. Pak J Bot. 2019;51(3):1143–8. https://doi.org/10.30848/PJB2019-3(38) .
Karthik K, Negi J, Rathinam M, et al. Exploitation of novel Bt ICPs for the management of Helicoverpa armigera (Hübner) in cotton ( Gossypium hirsutum L.): a transgenic approach. Front Microbial. 2021;12:661212. https://doi.org/10.3389/fmicb.2021.661212 .
Kashif N, Cheema HMN, Khan AA, et al. Expression profiling of transgenes ( Cry1Ac and Cry2A ) in cotton genotypes under different genetic backgrounds. J Integr Agric. 2022;21(10):2818–32. https://doi.org/10.1016/j.jia.2022.07.033 .
Kranthi KR, Stone GD. Long-term impacts of Bt cotton in India. Nat Plants. 2020;6(3):188–96. https://doi.org/10.1038/s41477-020-0750-z .
Kranthi K, Dhawad C, Naidu S, et al. Bt-cotton seed as a source of Bacillus thuringiensis insecticidal Cry1Ac toxin for bioassays to detect and monitor bollworm resistance to Bt-cotton. Curr Sci. 2005;88(5):796–800. https://www.jstor.org/stable/24111269 .
CAS Google Scholar
Lei Y, Jaleel W, Shahzad MF, et al. Effect of constant and fluctuating temperature on the circadian foraging rhythm of the red imported fire ant, Solenopsis invicta Buren (Hymenoptera: Formicidae). Saudi J Biol Sci. 2021;28(1):64–72. https://doi.org/10.1016/j.sjbs.2020.08.032 .
Article PubMed Google Scholar
Li H, Wu KM, Yang XR, et al. Trend of occurrence of cotton bollworm and control efficacy of Bt cotton in cotton planting region of southern Xinjiang. Sci Agric Sin. 2006;39(1):199–205. https://www.cabidigitallibrary.org/doi/full/10.5555/20073100228 .
Google Scholar
Llewellyn DJ, Mares CL, Fitt GP. Field performance and seasonal changes in the efficacy against Helicoverpa armigera (Hübner) of transgenic cotton expressing the insecticidal protein vip3A. Agric for Entomol. 2007;9(2):93–101. https://doi.org/10.1111/j.1461-9563.2007.00332.x .
Lu Y, Wyckhuys KA, Yang L, et al. Bt cotton area contraction drives regional pest resurgence, crop loss, and pesticide use. Plant Biotechnol J. 2022;20(2):390–8. https://doi.org/10.1111/pbi.13721 .
O’Connell C, Osmond D. Why soil testing is not enough: a mixed methods study of farmer nutrient management decision-making among US producers. J Environ Manage. 2022;314:115027. https://doi.org/10.1016/j.jenvman.2022.115027 .
Razaq M, Mensah R, Athar HUR. Insect pest management in cotton. In: Jabran K, Chauhan BS, editors. Cotton production. Hoboken: John Wiley & Sons Ltd; 2019. p. 85–107. https://doi.org/10.1002/9781119385523.ch5 .
Razzaq A, Zafar MM, ALI A, et al. Cotton germplasm improvement and progress in Pakistan. J Cotton Res. 2021;4(1):1–14. https://doi.org/10.1186/s42397-020-00077-x .
Rehman A, Jingdong L, Chandio AA, et al. Economic perspectives of cotton crop in Pakistan: a time series analysis (1970–2015)(Part 1). J Saudi Soc Agric Sci. 2019;18(1):49–54. https://doi.org/10.1016/j.jssas.2016.12.005 .
Ross Z, Wickham H, Robinson D. Declutter your R workflow with tidy tools. PeerJ Preprints. 2017;5:e3180v1. https://doi.org/10.7287/peerj.preprints.3180v1 .
Shahid MR, Farooq M, Shakeel M, et al. Need for growing non-Bt cotton refugia to overcome Bt resistance problem in targeted larvae of the cotton bollworms, Helicoverpa armigera and Pectinophora gossypiella . Egypt J Biol Pest Co. 2021;31:1–8. https://doi.org/10.1186/s41938-021-00384-8 .
Shahzad K, Mubeen I, Zhang M, et al. Progress and perspective on cotton breeding in Pakistan. J Cotton Res. 2022;5:29. https://doi.org/10.1186/s42397-022-00137-4 .
Shekhawat SS, Hasmi SK. Safety and benefits of Bt and Bt cotton: factures, refute, and allegations. In: Shekhawat SS, Irsad, Hasmi SK, editors. Genetic engineering. New York: Apple Academic Press; 2023. p. 23–52. https://www.taylorfrancis.com/chapters/edit/10.1201/9781003378273-2 .
Spielman DJ, Zaidi F, Zambrano P, et al. What are farmers really planting? Measuring the presence and effectiveness of Bt cotton in Pakistan. PLoS One. 2017;12(5):e0176592. https://doi.org/10.1371/journal.pone.0176592 .
Article CAS PubMed PubMed Central Google Scholar
Swami D, Parthasarathy D. A multidimensional perspective to farmers’ decision making determines the adaptation of the farming community. J Environ Manage. 2020;264:110487. https://doi.org/10.1016/j.jenvman.2020.110487 .
Tabashnik BE, Carrière Y. Surge in insect resistance to transgenic crops and prospects for sustainability. Nat Biotechnol. 2017;35(10):926–35. https://doi.org/10.1038/nbt.3974 .
Tabashnik BE, Brévault T, Carrière Y, et al. Insect resistance to Bt crops: lessons from the first billion acres. Nat Biotechnol. 2013;31(6):510–21. https://doi.org/10.1038/nbt.2597 .
Trapero C, Wilson IW, Stiller WN, et al. Enhancing integrated pest management in GM cotton systems using host plant resistance. Front Plant Sci. 2016;7:500. https://doi.org/10.3389/fpls.2016.00500 .
Article PubMed PubMed Central Google Scholar
Download references
Acknowledgements
The authors are thankful to Dr. Shakeel Ahmad, Seed Center, Ministry of Environment, Water and Agriculture, Riyadh, Dr. Muqadas Aleem, Department of Plant Breeding and Genetics, University of Agriculture, Faisalabad, Dr. Waseem Akbar, Maize and Millets Research Institute, Sahiwal for spending significant time on improvement of the technical aspect of our article and Mr. Ahmad Shehzad, Lab Assistant to assist in biophysical survey. Furthermore, Punjab Agriculture Research Board (PARB) for provision of funds for carrying out this study under Grant No. PARB 890.
This work was supported by Punjab Agriculture Research Board, Grant numbers PARB No. 890. Author S.J., S.U.R. and M.Z.I. has received research support from Punjab Agriculture Board.
Author information
Authors and affiliations.
Genetically Modified Organisms Development and Testing Laboratory, Agricultural Biotechnology Research Institute, Ayub Agricultural Research Institute, Faisalabad, Punjab, 38000, Pakistan
Shahzad Rahil, Jamil Shakra, Chaudhry Urooj Fatima, Rahman Sajid Ur & Iqbal Muhammad Zaffar
Centre of Excellence for Olive Research and Trainings (CEFORT), Barani, Agricultural Research Institute, Chakwal, Punjab, Pakistan
Iqbal Muhammad Zaffar
You can also search for this author in PubMed Google Scholar
Contributions
Shahzad R, Jamil S, Rahman SU, and Iqbal MZ Conceived and designed the analysis; Shahzad R, Jamil S, and Chaudhry UF Collected the data; Shahzad R Chaudhry UF and Jamil S Contributed data or analysis tools; Shahzad R and Chaudhry UF Performed the analysis; Shahzad R and Chaudhry UF wrote the paper; Jamil S, Rahman SU, and Iqbal MZ proofread the manuscript. All authors read and approved the final version of the manuscript.
Corresponding author
Correspondence to Shahzad Rahil .
Ethics declarations
Ethics approval and consent to participate.
Not applicable.
Consent for publication
Competing interests.
The authors declare that they have no competing interests.
Supplementary Information
Supplementary table s1. list of varieties cultivated at farmer fields along with their transgene and approval status., 42397_2024_191_moesm2_esm.docx.
Supplementary Table S2. Frequency Table showing the interaction between cotton type (BT and Non-BT) and various pest infestations, including American bollworm (AB), pink bollworm (PB), whitefly, aphid, jassid, and mite.
Rights and permissions
Open Access This article is licensed under a Creative Commons Attribution 4.0 International License, which permits use, sharing, adaptation, distribution and reproduction in any medium or format, as long as you give appropriate credit to the original author(s) and the source, provide a link to the Creative Commons licence, and indicate if changes were made. The images or other third party material in this article are included in the article's Creative Commons licence, unless indicated otherwise in a credit line to the material. If material is not included in the article's Creative Commons licence and your intended use is not permitted by statutory regulation or exceeds the permitted use, you will need to obtain permission directly from the copyright holder. To view a copy of this licence, visit http://creativecommons.org/licenses/by/4.0/ .
Reprints and permissions
About this article
Cite this article.
Shahzad, R., Jamil, S., Chaudhry, U.F. et al. In-depth analysis of Bt cotton adoption: farmers' opinions, genetic landscape, and varied perspectives—a case study from Pakistan. J Cotton Res 7 , 31 (2024). https://doi.org/10.1186/s42397-024-00191-0
Download citation
Received : 21 January 2024
Accepted : 18 July 2024
Published : 04 September 2024
DOI : https://doi.org/10.1186/s42397-024-00191-0
Share this article
Anyone you share the following link with will be able to read this content:
Sorry, a shareable link is not currently available for this article.
Provided by the Springer Nature SharedIt content-sharing initiative
- Cry1Ac, Cry2Ab
- Farmer’s perception
- Purposive sampling
- Sucking insects
- Unapproved varieties
Journal of Cotton Research
ISSN: 2523-3254
- Submission enquiries: [email protected]
- General enquiries: [email protected]

IMAGES
VIDEO
COMMENTS
Data Analysis in Research: Types & Methods
Learn what data analysis is, how to do it, and why it's important for various fields and industries. Explore the data analysis process, types of data analysis, and recommended courses to get started on Coursera.
Data Analysis Techniques In Research - Methods, Tools & ...
Learn how to plan, collect, and analyze data using statistical methods. This guide covers hypotheses, research design, sampling, descriptive and inferential statistics, and examples.
A practical guide to data analysis in general literature reviews
Learn what data analysis is, how to apply it to different types of questions, and what skills you need to get started. Explore the data analysis process, types of data analysis, and examples with Coursera courses and certificates.
Learn about the most common qualitative data analysis methods, such as content analysis, narrative analysis, discourse analysis and more. See how to apply them to your research project with examples and resources.
It is important to know w hat kind of data you are planning to collect or analyse as this w ill. affect your analysis method. A 12 step approach to quantitative data analysis. Step 1: Start with ...
Learn what data analysis is, how it is done, and why it is important for various fields and industries. Explore the different types of data analysis, such as descriptive, diagnostic, predictive, and prescriptive, and see examples of each type.
Explanatory Analysis, Infer ential Analys is, Predictive Analysis, Expla natory Analysis, Causal Analysis and. Mechanistic Analysis, Statistical Analysis. I. DATA ANALYSIS AND DATA PREPARATION ...
Quantitative Data Analysis Methods & Techniques 101
Learn about the definition, process, types, methods, tools, and applications of data analysis in research. Find out how to collect, clean, analyze, interpret, and communicate data using various techniques and software.
Types of Data Analysis: A Guide
Qualitative Data Analysis: What is it, Methods + Examples
Basic statistical tools in research and data analysis - PMC
Learning to Do Qualitative Data Analysis: A Starting Point
Learn what data analysis is, why it's important, and how to use it for your business. Explore five types of data analysis with examples and tips for each.
Explore four types of data analysis with examples. Data analysis is the practice of working with data to glean useful information, which can then be used to make informed decisions. "It is a capital mistake to theorize before one has data. Insensibly, one begins to twist facts to suit theories, instead of theories to suit facts," Sherlock ...
Data Analysis in Research | Methods, Techniques & ...
The aim of this paper is to equip readers with an understanding of the principles of qualitative data analysis and offer a practical example of how analysis might be undertaken in an interview-based study. Qualitative research is a generic term that refers to a group of methods, and ways of collecting and analysing data that are interpretative or explanatory in nature and focus on meaning ...
18 2 Practical Data Analysis: An Example. Fig. 2.1 A histogram for the distribution of the value of attribute age using 8 bins. Fig. 2.2 A histogram for the distribution of the value of attribute ...
Learn how to extract meaning from data and make better decisions with data analysis. Explore the data analysis process, different types of data analysis, and examples of how to apply them in various scenarios.
The sample design is the framework used that defines is the group of individuals who will participate in the research. Researchers have to decide how to select a sample that is representative. A good sample size is one that accurately represents the population and allows for reliable statistical analysis. Larger sample sizes are typically better because they reduce the likelihood of sampling ...
A deeper dive into the comparison between the processed post-coital samples and male reference sample suggests that the data support a clean "single source" assignment to the male subject for sperm fractions extracted from samples collected up to and including 12 h PC (i.e., Samples 1-7), while samples collected 24, 48, or 72 h after ...
Qualitative data analysis is. concerned with transforming raw data by searching, evaluating, recogni sing, cod ing, mapping, exploring and describing patterns, trends, themes an d categories in ...
Many studies have investigated the association between hypothyroidism and frozen shoulder, but their findings have been inconsistent. Furthermore, earlier research has been primarily observational, which may introduce bias and does not establish a cause-and-effect relationship. To ascertain the causal association, we performed a two-sample bidirectional Mendelian randomization (MR) analysis.
Molecular analysis of Cry1Ac, Cry2Ab and Vip3A genes. Molecular analysis was performed through strip test for detection and identification of transgenes at four hundred farmer fields, and a total of 10986 samples were tested. At each field, a minimum of 25 samples were collected and tested, at least 10 samples were tested for each variety.