Penn State University Libraries
Empirical research in the social sciences and education.
- What is Empirical Research and How to Read It
- Finding Empirical Research in Library Databases
- Designing Empirical Research
- Ethics, Cultural Responsiveness, and Anti-Racism in Research
- Citing, Writing, and Presenting Your Work

Contact the Librarian at your campus for more help!

Introduction: What is Empirical Research?
Empirical research is based on observed and measured phenomena and derives knowledge from actual experience rather than from theory or belief.
How do you know if a study is empirical? Read the subheadings within the article, book, or report and look for a description of the research "methodology." Ask yourself: Could I recreate this study and test these results?
Key characteristics to look for:
- Specific research questions to be answered
- Definition of the population, behavior, or phenomena being studied
- Description of the process used to study this population or phenomena, including selection criteria, controls, and testing instruments (such as surveys)
Another hint: some scholarly journals use a specific layout, called the "IMRaD" format, to communicate empirical research findings. Such articles typically have 4 components:
- Introduction: sometimes called "literature review" -- what is currently known about the topic -- usually includes a theoretical framework and/or discussion of previous studies
- Methodology: sometimes called "research design" -- how to recreate the study -- usually describes the population, research process, and analytical tools used in the present study
- Results: sometimes called "findings" -- what was learned through the study -- usually appears as statistical data or as substantial quotations from research participants
- Discussion: sometimes called "conclusion" or "implications" -- why the study is important -- usually describes how the research results influence professional practices or future studies
Reading and Evaluating Scholarly Materials
Reading research can be a challenge. However, the tutorials and videos below can help. They explain what scholarly articles look like, how to read them, and how to evaluate them:
- CRAAP Checklist A frequently-used checklist that helps you examine the currency, relevance, authority, accuracy, and purpose of an information source.
- IF I APPLY A newer model of evaluating sources which encourages you to think about your own biases as a reader, as well as concerns about the item you are reading.
- Credo Video: How to Read Scholarly Materials (4 min.)
- Credo Tutorial: How to Read Scholarly Materials
- Credo Tutorial: Evaluating Information
- Credo Video: Evaluating Statistics (4 min.)
- Credo Tutorial: Evaluating for Diverse Points of View
- Next: Finding Empirical Research in Library Databases >>
- Last Updated: Aug 13, 2024 3:16 PM
- URL: https://guides.libraries.psu.edu/emp
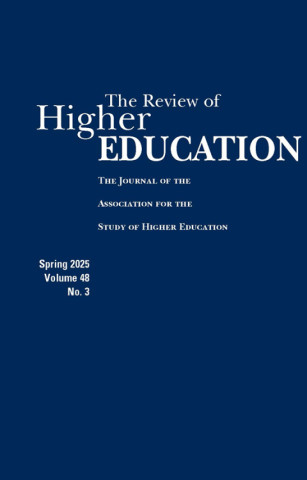
The Review of Higher Education
Penny A. Pasque, The Ohio State University; Thomas F. Nelson Laird, Indiana University, Bloomington
Journal Details
The Review of Higher Education is interested in empirical research studies, empirically-based historical and theoretical articles, and scholarly reviews and essays that move the study of colleges and universities forward. The most central aspect of RHE is the saliency of the subject matter to other scholars in the field as well as its usefulness to academic leaders and public policymakers. Manuscripts submitted for RHE need to extend the literature in the field of higher education and may connect across fields and disciplines when relevant. Selection of articles for publication is based solely on the merits of the manuscripts with regards to conceptual or theoretical frameworks, methodological accurateness and suitability, and/or the clarity of ideas and gathered facts presented. Additionally, our publications center around issues within US Higher Education and any manuscript that we send for review must have clear implications for US Higher Education.
Guidelines for Contributors
Manuscripts should be typed, serif or san serif text as recommended by APA 7th edition (e.g., 11-point Calibri, 11-point Arial, and 10-point Lucida Sans Unicode, 12-point Times New Roman, 11-point Georgia, 10-point Computer Modern) double-spaced throughout, including block quotes and references. Each page should be numbered on the top right side of the page consecutively and include a running head. Please supply the title of your submission, an abstract of 100 or fewer words, and keywords as the first page of your manuscript submission (this page does not count towards your page limit). The names, institutional affiliations, addresses, phone numbers, email addresses and a short biography of authors should appear on a separate cover page to aid proper masking during the review process. Initial and revised submissions should not run more than 32 pages (excluding abstract, keywords, and references; including tables, figures and appendices). Authors should follow instructions in the 7th edition Publication Manual of the American Psychological Association; any manuscripts not following all APA guidelines will not be reviewed. Please do not change fonts, spacing, or margins or use style formatting features at any point in the manuscript except for tables. All tables should be submitted in a mutable format (i.e. not a fixed image). Please upload your manuscript as a word document. All supporting materials (i.e., tables, figures, appendices) should be editable in the manuscript or a separate word document (i.e., do not embedded tables or figures). For a fixed image, please upload a separate high-resolution JPEG.
Authors should use their best judgment when masking citations. Masking some or all citations that include an author’s name can help prevent reviewers from knowing the identities of the authors. However, in certain circumstances masking citations is unnecessary or could itself reveal the identities of manuscript authors. Because authors are in the best position to know when masking citations will be effective, the editorial team will generally defer to them for these decisions.
Manuscripts are to be submitted in Word online at mc.manuscriptcentral.com/rhe . (If you have not previously registered on this website, click on the “Register here” link to create a new account.) Once you log on, click on the “Author Center” link and then follow the printed instructions to submit your manuscript.
The term “conflict of interest” means any financial or other interest which conflicts with the work of the individual because it (1) could significantly impair the individual’s objectivity or (2) could create an unfair advantage for any person or organization. We recommend all authors review and adhere to the ASHE Conflict of Interest Policy before submitting any and all work. Please refer to the policy at ashe.ws/ashe_coi
Please note that The Review of Higher Education does not require potential contributors to pay an article submission fee in order to be considered for publication. Any other website that purports to be affiliated with the Journal and that requires you to pay an article submission fee is fraudulent. Do not provide payment information. Instead, we ask that you contact the RHE editorial office at [email protected] or William Breichner the Journals Publisher at the Johns Hopkins University Press [email protected] .
Author Checklist for New Submissions
Page Limit. Manuscripts should not go over 32 pages (excluding abstract, keywords, and references; including tables, figures and appendices.)
Masked Review. All author information (i.e., name, affiliation, email, phone number, address) should appear on a separate cover page of the manuscript. The manuscript should have no indication of authorship. Any indication of authorship will result in your manuscript being unsubmitted.
Formatting. Manuscripts should be typed, serif or san serif text as recommended by APA 7th edition (e.g., 11-point Calibri, 11-point Arial, and 10-point Lucida Sans Unicode, 12-point Times New Roman, 11-point Georgia, 10-point Computer Modern), double-spaced throughout, including block quotes and references, and each page should be numbered on the top right side of the page consecutively. Authors should follow instructions in the 7th edition Publication Manual of the American Psychological Association; this includes running heads, heading levels, spacing, margins, etc.. Any manuscripts not following APA 7th edition will be unsubmitted. [Please note, the RHE editorial team recommends 12-pt Times New Roman font to ensure proper format conversion within the ScholarOne system.]
Abstract. All manuscripts must include an abstract of 100 words or fewer, and keywords as the first page of your manuscript submission (this page does not count towards your page limit).
Author Note. An Author’s note may include Land Acknowledgments, Disclosure Statement (i.e., funding sources), or other acknowledgments. This should appear on your title page (not in the masked manuscript).
Tables. All tables should be editable. Tables may be uploaded in the manuscript itself or in a separate word document. All tables must be interpretable by readers without the reference to the manuscript. Do not duplicate information from the manuscript into tables. Tables must present additional information from what has already been stated in the manuscript.
Figures. Figures should be editable in the manuscript or a separate word document (i.e., no embedded tables). For fixed images, please upload high-resolution JPEGs separately.
References. The reference page should follow 7th edition APA guidelines and be double spaced throughout (reference pages do not count toward your page limit).
Appendices. Appendices should generally run no more than 3 manuscript pages.
Additional Checklist for Revised Submissions
Revised manuscripts should follow the checklist above, with the following additional notes:
Page Limit. Revised manuscripts should stay within the page limit for new submissions (32 pages). However, we do realize that this is not always possible, and we may allow for a couple of extra pages for your revisions. Extensions to your page length will be subject to editor approval upon resubmission, but may not exceed 35 pages (excluding abstract, keywords, and references).
- Author Response to Reviewer Comments. At the beginning of your revised manuscript file, please include a separate masked statement that indicates fully [1] all changes that have been made in response to the reviewer and editor suggestions and the pages on which those changes may be found in the revised manuscript and [2] those reviewer and editor suggestions that are not addressed in the revised manuscript and a rationale for why you think such revisions are not necessary. This can be in the form of a table or text paragraphs and must appear at the front of your revised manuscript document. Your response to reviewer and editor comments will not count toward your manuscript page limit. Please note that, because you will be adding your response to the reviewer and editor feedback to the beginning of your submission, this may change the page numbers of your document unless you change the pagination and start your manuscript itself on page 1. The choice is yours but either way, please ensure that you reference the appropriate page numbers within your manuscript in these responses. Additionally, when you submit your revised manuscript, there will be a submission box labeled “Author Response to Decision Letter”. You are not required to duplicate information already provided in the manuscript, but instead may use this to send a note to the reviewer team (e.g., an anonymous cover letter or note of appreciation for feedback). Please maintain anonymity throughout the review process by NOT including your name or by masking any potentially identifying information when providing your response to the reviewer's feedback (both in documents and the ScholarOne system).
Editorial Correspondence
Please address all correspondence about submitting articles (no subscriptions, please) to one or both of the following editors:
Dr. Penny A. Pasque, PhD Editor, Review of Higher Education 341 C Ramseyer Hall 29 W. Woodruff Avenue The Ohio State University Columbus, OH 43210 email: [email protected]
Dr. Thomas F. Nelson Laird, PhD Editor, Review of Higher Education 201 North Rose Avenue Indiana University School of Education Bloomington, IN 47405-100 email: [email protected]
Submission Policy
RHE publishes original works that are not available elsewhere. We ask that all manuscripts submitted to our journal for review are not published, in press or submitted to other journals while under our review. Additionally, reprints and translations of previously published articles will not be accepted.
Type of Preliminary Review
RHE utilizes a collaborative review process that requires several members of the editorial team to ensure that submitted manuscripts are suitable before being sent out for masked peer-review. Members of this team include a Editor, Associate Editor and Managing Editors. Managing Editors complete an initial review of manuscripts to ensure authors meet RHE ’s Author Guidelines and work with submitting authors to address preliminary issues and concerns (i.e., APA formatting). Editors and Associate Editors work together to decide whether it should be sent out for review and select appropriate reviewers for the manuscript.
Type of Review
When a manuscript is determined as suitable for review by the collaborative decision of the editorial team, Editors and/or Associate Editors will assign reviewers. Both the authors’ and reviewers’ are masked throughout the review and decision process.
Criteria for Review
Criteria for review include, but are not limited to, the significance of the topic to higher education, completeness of the literature review, appropriateness of the research methods or historical analysis, and the quality of the discussion concerning the implications of the findings for theory, research, and practice. In addition, we look for the congruence of thought and approach throughout the manuscript components.
Type of Revisions Process
Some authors will receive a “Major Revision” or “Minor Revision” decision. Authors who receive such decisions are encouraged to carefully attend to reviewer’s comments and recommendations and resubmit their revised manuscripts for another round of reviews. When submitting their revised manuscripts, authors are asked to include a response letter and indicate how they have responded to reviewer comments and recommendations. In some instances, authors may be asked to revise and resubmit a manuscript more than once.
Review Process Once Revised
Revised manuscripts are sent to the reviewers who originally made comments and recommendations regarding the manuscript, whenever possible. We rely on our editorial board and ad-hoc reviewers who volunteer their time and we give those reviewers a month to provide thorough feedback. Please see attached pdf for a visual representation of the RHE workflow .
Timetable (approx.)
- Managing Editor Technical Checks – 1-3 days
- Editor reviews and assigns manuscript to Associate Editors – 3-5 days
- Associate Editor reviews and invites reviewers – 3-5 days
- Reviewer comments due – 30 days provided for reviews
- Associate Editor makes a recommendation – 5-7 days
- Editor makes decision – 5-7 days
- If R&R, authors revise and resubmit manuscript – 90 days provided for revisions
- Repeat process above until manuscript is accepted or rejected -
Type of review for book reviews
Book reviews are the responsibility of the associate editor of book reviews. Decisions about acceptance of a book review are made by that associate editor.
The Hopkins Press Journals Ethics and Malpractice Statement can be found at the ethics-and-malpractice page.
The Review of Higher Education expects all authors to review and adhere to ASHE’s Conflict of Interest Policy before submitting any and all work. The term “conflict of interest” means any financial or other interest which conflicts with the work of the individual because it (1) could significantly impair the individual’s objectivity or (2) could create an unfair advantage for any person or organization. Please refer to the policy at ashe.ws/ashe_coi .
Guidelines for Book Reviews
RHE publishes book reviews of original research, summaries of research, or scholarly thinking in book form. We do not publish reviews of books or media that would be described as expert opinion or advice for practitioners.
The journal publishes reviews of current books, meaning books published no more than 12 months prior to submission to the associate editor in charge of book reviews.
If you want to know whether the RHE would consider a book review before writing it, you may email the associate editor responsible for book reviews with the citation for the book.
Reviewers should have scholarly expertise in the higher education research area they are reviewing.
Graduate students are welcome to co-author book reviews, but with faculty or seasoned research professionals as first authors.
Please email the review to the associate editor in charge of book reviews (Timothy Reese Cain, [email protected] ), who will work through necessary revisions with you if your submission is accepted for publishing.
In general, follow the APA Publication Manual, 7th edition.
Provide a brief but clear description and summary of the contents so that the reader has a good idea of the scope and organization of the book. This is especially important when reviewing anthologies that include multiple sections with multiple authors.
Provide an evaluation of the book, both positive and negative points. What has been done well? Not so well? For example the following are some questions that you can address (not exclusively), as appropriate:
What are the important contributions that this book makes?
What contributions could have been made, but were not made?
What arguments or claims were problematic, weak, etc.?
How is the book related to, how does it supplement, or how does it complicate current work on the topic?
To which audience(s) will this book be most helpful?
How well has the author achieved their stated goals?
Use quotations efficiently to provide a flavor of the writing style and/or statements that are particularly helpful in illustrating the author(s) points.
If you cite any other published work, please provide a complete reference.
Please include a brief biographical statement immediately after your name, usually title and institution. Follow the same format for co authored reviews. The first author is the contact author.
Please follow this example for the headnote of the book(s) you are reviewing: Stefan M. Bradley. Upending the Ivory Tower: Civil Rights, Black Power, and the Ivy League. New York: New York University Press, 2018. 465 pp. $35. ISBN 97814798739999.
Our preferred length is 2,000–2,500 words in order for authors to provide a complete, analytical, review. Reviews of shorter books may not need to be of that length.
The term “conflict of interest” means any financial or other interest which conflicts with the work of the individual because it (1) could significantly impair the individual’s objectivity or (2) could create an unfair advantage for any person or organization. We recommend all book reviewers read and adhere to the ASHE Conflict of Interest Policy before submitting any and all work. Please refer to the policy at ashe.ws/ashe_coi
NOTE: If the Editor has sent a book to an author for review, but the author is unable to complete the review within a reasonable timeframe, we would appreciate the return of the book as soon as possible; thanks for your understanding.
Please send book review copies to the contact above. Review copies received by the Johns Hopkins University Press office will be discarded.
Penny A. Pasque, The Ohio State University
Thomas F. Nelson Laird, Indiana University-Bloomington
Associate Editors
Angela Boatman, Boston College
Timothy Reese Cain (including Book Reviews), University of Georgia
Milagros Castillo-Montoya, University of Connecticut
Tania D. Mitchell, University of Minnesota
Chrystal A. George Mwangi George Mason University
Federick Ngo, University of Nevada, Las Vegas
Managing Editors
Miras Sharipov Indiana University Bloomington
Monica Quezada Barrera, The Ohio State University
Editorial Board
Sonja Ardoin, Clemson University
Peter Riley Bahr, University of Michigan
Vicki Baker, Albion College
Allison BrckaLorenz, Indiana University Bloomington
Nolan L. Cabrera, The University of Arizona
Brendan Cantwell, Michigan State University
Rozana Carducci, Elon University
Deborah Faye Carter, Claremont Graduate University
Ashley Clayton, Louisiana State University
Regina Deil-Amen, The University of Arizona
Jennifer A. Delaney, University of Illinois Urbana Champaign
Erin E. Doran, Iowa State University
Antonio Duran, Arizona State University
Michelle M. Espino, University of Maryland
Claudia García-Louis, University of Texas, San Antonio
Deryl Hatch-Tocaimaza, University of Nebraska-Lincoln
Nicholas Hillman, University of Wisconsin-Madison
Cindy Ann Kilgo, Indiana University-Bloomington
Judy Marquez Kiyama, University of Arizona
Román Liera, Montclair State University
Angela Locks, California State University, Long Beach
Demetri L. Morgan, Loyola University Chicago
Rebecca Natow, Hofstra University
Z Nicolazzo, The University of Arizona
Elizabeth Niehaus, University of Nebraska-Lincoln
Robert T. Palmer, Howard University
Rosemary Perez, University of Michigan
OiYan Poon, Spencer Foundation
Kelly Rosinger, The Pennsylvania State University
Vanessa Sansone, The University of Texas at San Antonio
Tricia Seifert, Montana State University
Barrett Taylor, University of North Texas
Annemarie Vaccaro, University of Rhode Island
Xueli Wang, University of Wisconsin-Madison
Stephanie Waterman, University of Toronto
Rachelle Winkle-Wagner, University of Wisconsin-Madison
Association for the Study of Higher Education Board of Directors
The Review of Higher Education is the journal of Association for the Study Higher Education (ASHE) and follows the ASHE Bylaws and Statement on Diversity.
ASHE Board of Directors
Abstracting & Indexing Databases
- Current Contents
- Web of Science
- Dietrich's Index Philosophicus
- IBZ - Internationale Bibliographie der Geistes- und Sozialwissenschaftlichen Zeitschriftenliteratur
- Internationale Bibliographie der Rezensionen Geistes- und Sozialwissenschaftlicher Literatur
- Academic Search Alumni Edition, 9/1/2003-
- Academic Search Complete, 9/1/2003-
- Academic Search Elite, 9/1/2003-
- Academic Search Premier, 9/1/2003-
- Current Abstracts, 9/1/2003-
- Education Research Complete, 3/1/1997-
- Education Research Index, Sep.2003-
- Education Source, 3/1/1997-
- Educational Administration Abstracts, 3/1/1991-
- ERIC (Education Resources Information Center), 1977-
- MLA International Bibliography (Modern Language Association)
- Poetry & Short Story Reference Center, 3/1/1997-
- PsycINFO, 2001-, dropped
- Russian Academy of Sciences Bibliographies
- TOC Premier (Table of Contents), 9/1/2003-
- Scopus, 1996-
- Gale Academic OneFile
- Gale OneFile: Educator's Reference Complete, 12/2001-
- Higher Education Abstracts (Online)
- ArticleFirst, vol.15, no.3, 1992-vol.35, no.2, 2011
- Electronic Collections Online, vol.20, no.1, 1996-vol.35, no.2, 2011
- Periodical Abstracts, v.26, n.4, 2003-v.33, n.3, 2010
- PsycFIRST, vol.24, no.3, 2001-vol.33, no.1, 2009
- Personal Alert (E-mail)
- Education Collection, 7/1/2003-
- Education Database, 7/1/2003-
- Health Research Premium Collection, 7/1/2003-
- Hospital Premium Collection, 7/1/2003-
- Periodicals Index Online, 1/1/1981-7/1/2000
- Professional ProQuest Central, 07/01/2003-
- ProQuest 5000, 07/01/2003-
- ProQuest 5000 International, 07/01/2003-
- ProQuest Central, 07/01/2003-
- Psychology Database, 7/1/2003-
- Research Library, 07/01/2003-
- Social Science Premium Collection, 07/01/2003-
- Educational Research Abstracts Online
- Research into Higher Education Abstracts (Online)
- Studies on Women and Gender Abstracts (Online)
Abstracting & Indexing Sources
- Contents Pages in Education (Ceased) (Print)
- Family Index (Ceased) (Print)
- Psychological Abstracts (Ceased) (Print)
Source: Ulrichsweb Global Serials Directory.
2.9 (2023) 3.2 (Five-Year Impact Factor) 0.00184 (Eigenfactor™ Score) Rank in Category (by Journal Impact Factor): 72 of 756 journals, in “Education & Educational Research”
© Clarivate Analytics 2024
Published quarterly
Readers include: Scholars, academic leaders, administrators, public policy makers involved in higher education, and all members of the Association for the Study of Higher Education (ASHE)
Print circulation: 761
Print Advertising Rates
Full Page: (4.75 x 7.5") - $450.00
Half Page: (4.75 x 3.5") - $338.00
2 Page Spread - $675.00
Print Advertising Deadlines
September Issue – July 15
December Issue – October 15
March Issue – January 15
June Issue – April 15
Online Advertising Rates (per month)
Promotion (400x200 pixels) – $338.00
Online Advertising Deadline
Online advertising reservations are placed on a month-to-month basis.
All online ads are due on the 20th of the month prior to the reservation.
General Advertising Info
For more information on advertising or to place an ad, please visit the Advertising page.
eTOC (Electronic Table of Contents) alerts can be delivered to your inbox when this or any Hopkins Press journal is published via your ProjectMUSE MyMUSE account. Visit the eTOC instructions page for detailed instructions on setting up your MyMUSE account and alerts.
Also of Interest
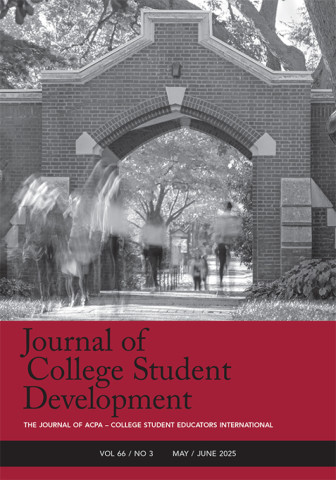
Vasti Torres, Indiana University
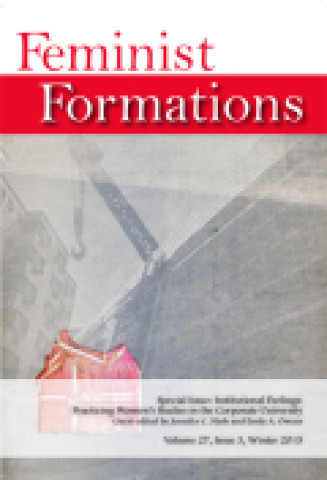
Patti Duncan, Oregon State University
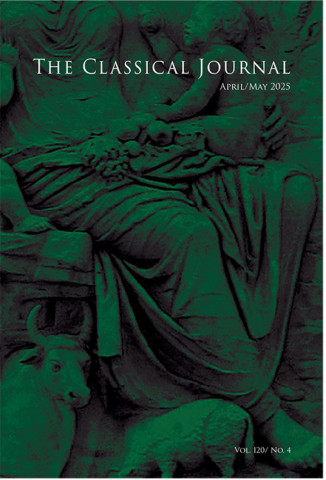
Georgia L. Irby, College of William & Mary
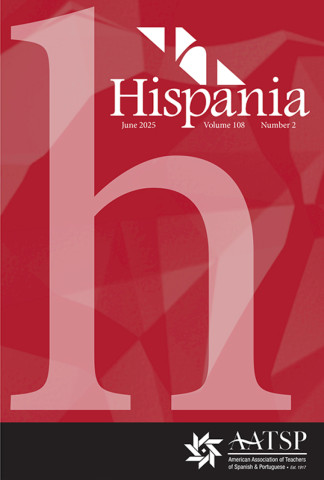
Benjamin Fraser, The University of Arizona
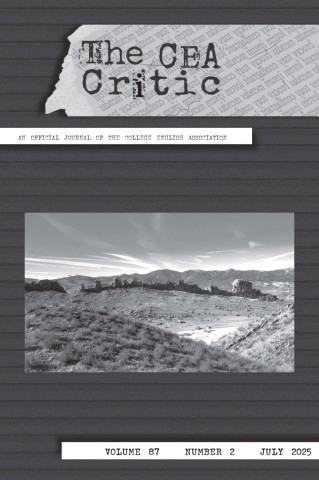
Jeraldine Kraver, University of Northern Colorado; Peter Kratzke, University of Colorado, Boulder
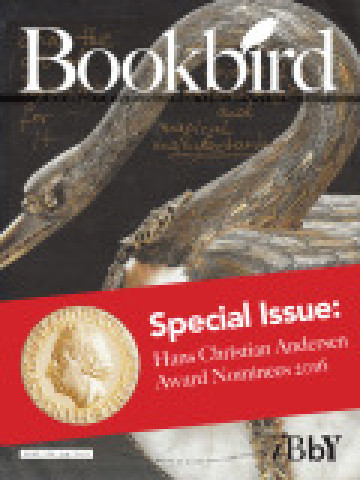
Chrysogonus Siddha Malilang, Malmö University, Sweden
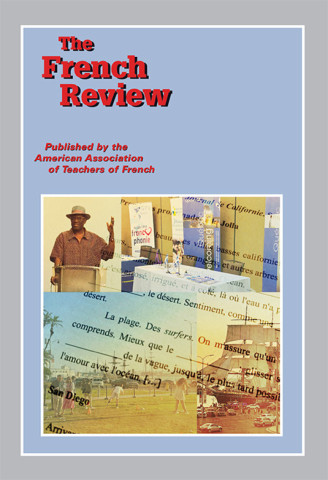
Carine Bourget, University of Arizona
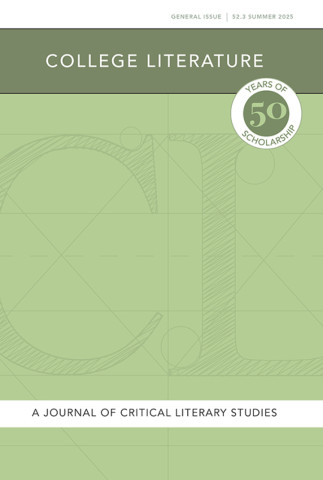
Megan Corbin, West Chester University
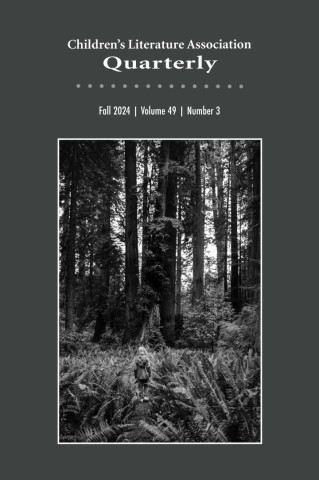
Joseph Michael Sommers, Central Michigan University
Hopkins Press Journals
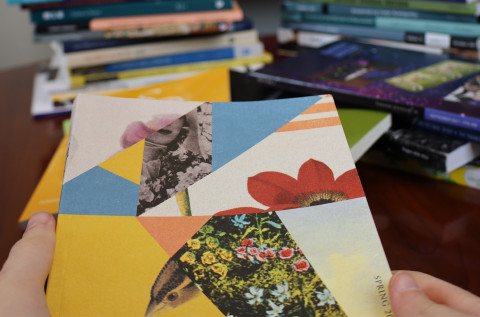

Journal of Diversity in Higher Education
- Read this journal
- Read free articles
- Journal snapshot
- Advertising information
Journal scope statement
APA and the National Association of Diversity Officers in Higher Education (NADOHE) have joined together to publish the Journal of Diversity in Higher Education .
Multidisciplinary in perspective, this bimonthly journal offers insights into theory and research that can help guide the efforts of institutions of higher education in the pursuit of inclusive excellence.
Journal of Diversity in Higher Education largely publishes empirical research focused on issues related to diversity, equity, and inclusion in post-secondary environments. Our manuscripts address the experiences and outcomes of individuals from underrepresented and underserved communities, focusing on institutional barriers and challenges, patterns of access and achievement, and the impact of engaging with diverse students, faculty, and administrators.
We are also interested in work that explores issues related to teaching and learning, policy development and implementation, and leadership and organizational change in diverse learning environments. We are committed to publishing work that supports efforts to transform institutions, inspire colleagues, engage campus, governmental, and private sector leaders, and articulate culturally competent outcomes.
Equity, diversity, and inclusion
Journal of Diversity in Higher Education supports equity, diversity, and inclusion (EDI) in its practices. More information on these initiatives is available under EDI Efforts .
Calls for papers
- General call for papers
Practice briefs
Editor’s choice.
One article from each issue of Journal of Diversity in Higher Education will be highlighted as an “ Editor’s Choice ” article. Selection is based on the recommendations of the associate editors, the paper’s potential impact to the field, the distinction of expanding the contributors to, or the focus of, the science, or its discussion of an important future direction for science. Editor’s Choice articles are featured alongside articles from other APA published journals in a bi-weekly newsletter and are temporarily made freely available to newsletter subscribers.
Author and editor spotlights
Explore journal highlights : free article summaries, editor interviews and editorials, journal awards, mentorship opportunities, and more.
Prior to submission, please carefully read and follow the submission guidelines detailed below. Manuscripts that do not conform to the submission guidelines may be returned without review.
To submit to the editorial office of Chris Linder, please submit manuscripts electronically (.rtf, .pdf, or .doc) through the Manuscript Submission Portal.
Prepare manuscripts according to the Publication Manual of the American Psychological Association using the 7 th edition. Manuscripts may be copyedited for bias-free language (see Chapter 5 of the Publication Manual ). APA Style and Grammar Guidelines for the 7 th edition are available.
Submit Manuscript
Chris Linder, PhD Associate Professor, Higher Education Department of Educational Leadership & Policy Director, McCluskey Center for Violence Prevention University of Utah Salt Lake City, UT Email
In addition to addresses and phone numbers, please supply email addresses and fax numbers for use by the editorial office and later by the production office. The majority of correspondence between the editorial office and authors is handled by email, so a valid email address is important to the timely flow of communication during the editorial process.
Manuscripts for the Journal of Diversity in Higher Education can vary in length based on the type below. Manuscripts should be concise but of sufficient length to ensure theoretical and/or methodological competence.
The Journal publishes full length articles that share empirical research that supports efforts to transform institutions; inspire colleagues; engage campus, governmental, and private sector leaders; and articulate culturally competent outcomes. We also publish practice briefs that present empirically-based, conceptually framed recommendations addressing pressing and persistent problems faced by practitioners in their daily work. We do not publish book reviews or critical essays at this time.
Manuscript types
Journal of Diversity in Higher Education will publish two types of articles. All page limits are exclusive of tables, figures, and references:
- full length articles (20 – 35 double-spaced pages maximum) based on empirical research that makes major theoretical contributions and has practical implications
- practice briefs (6 – 10 double-spaced pages maximum) that leverage existing theory and research to inform efforts to address real-world problems and provide actionable recommendations for practitioners.
The Journal of Diversity in Higher Education publishes practice briefs as a way to accomplish the journal’s mission to advance scholarship that promotes institutional transformation and inclusive excellence. Their length and specificity make them an ideal resource for policymakers, practitioners, and institutional leaders. As scholarly pieces that translate and advance research, practice briefs are meant to foster dialogue and connection among scholarship and practice. The journal is especially interested in pieces co-authored by practitioners and researchers.
Reference the call for practice briefs for full instructions and guidelines for the structure and submission of practice briefs.
Manuscript preparation
Prepare manuscripts according to the Publication Manual of the American Psychological Association using the 7th edition. Manuscripts may be copyedited for bias-free language (see Chapter 5 of the Publication Manual ).
Review APA's Journal Manuscript Preparation Guidelines before submitting your article.
Double-space all copy. Other formatting instructions, as well as instructions on preparing tables, figures, references, metrics, and abstracts, appear in the Manual . Additional guidance on APA Style is available on the APA Style website .
If your manuscript was mask reviewed, please ensure that the final version for production includes a byline and full author note for typesetting.
Authors should review the updated APA Style Journal Article Reporting Standards (JARS) for quantitative, qualitative, and mixed methods research. These standards offer ways to improve transparency in reporting to ensure that readers have the information necessary to evaluate the quality of the research and to facilitate collaboration and replication.
The new JARS
- Recommend the division of hypotheses, analyses, and conclusions into primary, secondary, and exploratory groupings to allow for a full understanding of quantitative analyses presented in a manuscript and to enhance reproducibility;
- Offer modules for authors reporting on N-of-1 designs, replications, clinical trials, longitudinal studies, and observational studies, as well as the analytic methods of structural equation modeling and Bayesian analysis;
- Include guidelines on reporting on registration (including making protocols public); participant characteristics, including demographic characteristics; inclusion and exclusion criteria; psychometric characteristics of outcome measures and other variables; and planned data diagnostics and analytic strategy.
For further resources, including flowcharts, review the APA Style Journal Article Reporting Standards (JARS) website.
Below are additional instructions regarding the preparation of display equations, computer code, and tables.
Display equations
We strongly encourage you to use MathType (third-party software) or Equation Editor 3.0 (built into pre-2007 versions of Word) to construct your equations, rather than the equation support that is built into Word 2007 and Word 2010. Equations composed with the built-in Word 2007/Word 2010 equation support are converted to low-resolution graphics when they enter the production process and must be rekeyed by the typesetter, which may introduce errors.
To construct your equations with MathType or Equation Editor 3.0:
- Go to the Text section of the Insert tab and select Object.
- Select MathType or Equation Editor 3.0 in the drop-down menu.
If you have an equation that has already been produced using Microsoft Word 2007 or 2010 and you have access to the full version of MathType 6.5 or later, you can convert this equation to MathType by clicking on MathType Insert Equation. Copy the equation from Microsoft Word and paste it into the MathType box. Verify that your equation is correct, click File, and then click Update. Your equation has now been inserted into your Word file as a MathType Equation.
Use Equation Editor 3.0 or MathType only for equations or for formulas that cannot be produced as Word text using the Times or Symbol font.
Computer code
Because altering computer code in any way (e.g., indents, line spacing, line breaks, page breaks) during the typesetting process could alter its meaning, we treat computer code differently from the rest of your article in our production process. To that end, we request separate files for computer code.
In online supplemental material
We request that runnable source code be included as supplemental material to the article. For more information, visit Supplementing Your Article With Online Material .
In the text of the article
If you would like to include code in the text of your published manuscript, please submit a separate file with your code exactly as you want it to appear, using Courier New font with a type size of 8 points. We will make an image of each segment of code in your article that exceeds 40 characters in length. (Shorter snippets of code that appear in text will be typeset in Courier New and run in with the rest of the text.) If an appendix contains a mix of code and explanatory text, please submit a file that contains the entire appendix, with the code keyed in 8-point Courier New.
Use Word's insert table function when you create tables. Using spaces or tabs in your table will create problems when the table is typeset and may result in errors.
Academic writing and English language editing services
Authors who feel that their manuscript may benefit from additional academic writing or language editing support prior to submission are encouraged to seek out such services at their host institutions, engage with colleagues and subject matter experts, and/or consider several vendors that offer discounts to APA authors .
Please note that APA does not endorse or take responsibility for the service providers listed. It is strictly a referral service.
Use of such service is not mandatory for publication in an APA journal. Use of one or more of these services does not guarantee selection for peer review, manuscript acceptance, or preference for publication in any APA journal.
Submitting supplemental materials
APA can place supplemental materials online, available via the published article in the PsycArticles ® database. Please see Supplementing Your Article With Online Material for more details.
Abstract and keywords
All manuscripts must include an abstract containing a maximum of 250 words typed on a separate page. After the abstract, please supply up to five keywords or brief phrases.
List references in alphabetical order. Each listed reference should be cited in text, and each text citation should be listed in the references section.
Examples of basic reference formats:
Journal article
McCauley, S. M., & Christiansen, M. H. (2019). Language learning as language use: A cross-linguistic model of child language development. Psychological Review , 126 (1), 1–51. https://doi.org/10.1037/rev0000126
Authored book
Brown, L. S. (2018). Feminist therapy (2nd ed.). American Psychological Association. https://doi.org/10.1037/0000092-000
Chapter in an edited book
Balsam, K. F., Martell, C. R., Jones. K. P., & Safren, S. A. (2019). Affirmative cognitive behavior therapy with sexual and gender minority people. In G. Y. Iwamasa & P. A. Hays (Eds.), Culturally responsive cognitive behavior therapy: Practice and supervision (2nd ed., pp. 287–314). American Psychological Association. https://doi.org/10.1037/0000119-012
Preferred formats for graphics files are TIFF and JPG, and preferred format for vector-based files is EPS. Graphics downloaded or saved from web pages are not acceptable for publication. Multipanel figures (i.e., figures with parts labeled a, b, c, d, etc.) should be assembled into one file. When possible, please place symbol legends below the figure instead of to the side.
- All color line art and halftones: 300 DPI
- Black and white line tone and gray halftone images: 600 DPI
Line weights
- Color (RGB, CMYK) images: 2 pixels
- Grayscale images: 4 pixels
- Stroke weight: 0.5 points
APA offers authors the option to publish their figures online in color without the costs associated with print publication of color figures.
The same caption will appear on both the online (color) and print (black and white) versions. To ensure that the figure can be understood in both formats, authors should add alternative wording (e.g., “the red (dark gray) bars represent”) as needed.
For authors who prefer their figures to be published in color both in print and online, original color figures can be printed in color at the editor's and publisher's discretion provided the author agrees to pay:
- $900 for one figure
- An additional $600 for the second figure
- An additional $450 for each subsequent figure
Permissions
Authors of accepted papers must obtain and provide to the editor on final acceptance all necessary permissions to reproduce in print and electronic form any copyrighted work, including test materials (or portions thereof), photographs, and other graphic images (including those used as stimuli in experiments).
On advice of counsel, APA may decline to publish any image whose copyright status is unknown.
- Download Permissions Alert Form (PDF, 13KB)
Publication policies
For full details on publication policies, including use of Artificial Intelligence tools, please see APA Publishing Policies .
APA policy prohibits an author from submitting the same manuscript for concurrent consideration by two or more publications.
See also APA Journals ® Internet Posting Guidelines .
APA requires authors to reveal any possible conflict of interest in the conduct and reporting of research (e.g., financial interests in a test or procedure, funding by pharmaceutical companies for drug research).
- Download Full Disclosure of Interests Form (PDF, 41KB)
Ethical Principles
It is a violation of APA Ethical Principles to publish "as original data, data that have been previously published" (Standard 8.13).
In addition, APA Ethical Principles specify that "after research results are published, psychologists do not withhold the data on which their conclusions are based from other competent professionals who seek to verify the substantive claims through reanalysis and who intend to use such data only for that purpose, provided that the confidentiality of the participants can be protected and unless legal rights concerning proprietary data preclude their release" (Standard 8.14).
APA expects authors to adhere to these standards. Specifically, APA expects authors to have their data available throughout the editorial review process and for at least 5 years after the date of publication.
Authors are required to state in writing that they have complied with APA ethical standards in the treatment of their sample, human or animal, or to describe the details of treatment.
- Download Certification of Compliance With APA Ethical Principles Form (PDF, 26KB)
The APA Ethics Office provides the full Ethical Principles of Psychologists and Code of Conduct electronically on its website in HTML, PDF, and Word format. You may also request a copy by emailing or calling the APA Ethics Office (202-336-5930). You may also read "Ethical Principles," December 1992, American Psychologist , Vol. 47, pp. 1597–1611.
Other information
See APA’s Publishing Policies page for more information on publication policies, including information on author contributorship and responsibilities of authors, author name changes after publication, the use of generative artificial intelligence, funder information and conflict-of-interest disclosures, duplicate publication, data publication and reuse, and preprints.
Visit the Journals Publishing Resource Center for more resources for writing, reviewing, and editing articles for publishing in APA journals.
Chris Linder, PhD University of Utah, United States
Associate editors
Erin Doran, EdD Iowa State University, United States
Patton O. Garriott, PhD University of Denver, United States
Chrystal George Mwangi, PhD George Washington University, United States
Deryl Hatch-Tocaimaza, PhD University of Nebraska–Lincoln, United States
Editorial board
Frim Ampaw, EdD Morgan State University, United States
Matthew Bahnson, PhD Purdue University, United States
Veronica Jones Baldwin, PhD University of North Texas, United States
Jessica C. Bennett, PhD University of Maryland, United States
Ginny Boss, PhD University of Georgia, United States
Kaleb L. Briscoe, PhD University of Oklahoma, United States
Christopher Broadhurst, PhD University of New Orleans, United States
Derrick Brooms, PhD University of Tennessee Knoxville, United States
Kirsten Brown, PhD Edgewood College, United States
Jessica Belue Buckley, PhD University of Louisville , United States
Beth E. Bukoski, PhD Virginia Commonwealth University, United States
Jorge Burmicky, PhD Howard University, United States
W. Carson Byrd, PhD University of Michigan , United States
Germán A. Cadenas, PhD Lehigh University, United States
D. Chase J. Catalano, EdD Virginia Tech, United States
Tabbye Maria Chavous, PhD University of Michigan, United States
Mun Yuk Chin, PhD University of Nebraska–Lincoln, United States
Mary M. Chittooran, PhD Saint Louis University, United States
D. Anthony Clark, PhD Arizona State University, United States
Joan Collier, PhD Rutgers University, United States
Felecia Commodore, PhD Old Dominion University, United States
Marcela G. Cuellar, PhD University of California, Davis, United States
Bradley R. Curs, PhD University of Missouri, United States
Kun Dai, PhD The Chinese University of Hong Kong, Hong Kong
Charles H.F. Davis III, PhD University of Michigan, United States
Chayla Haynes Davison, PhD Texas A&M University, United States
J. Michael Denton, PhD University of South Florida, United States
Kari J. Dockendorff, PhD Colorado State University , United States
Antonio Duran, PhD Arizona State University, United States
Carlton J. Fong Texas State University, United States
Zak Foste, PhD University of Kansas , United States
Nichole M. Garcia, PhD Rutgers University, United States
Stacey D. Garrett, PhD Appalachian State University, United States
Leslie D. Gonzales, EdD Michigan State University, United States
Niah S. Grimes, PhD Morgan State University, United States
Kayon A. Hall, PhD Kent State University, United States
Ebelia Hernandez, PhD Rutgers University, United States
Tara D. Hudson, PhD Kent State University, United States
Sarah Socorro Hurtado, PhD University of Denver, United States
Dimpal Jain, PhD California State University, Northridge, United States
Rashné Jehangir, PhD University of Minnesota, United States
Jarrel T. Johnson, PhD University of Utah, United States
Jennifer M. Johnson, PhD Temple University, United Sates
Nadeeka Karunaratne, PhD University of Utah, United States
Cindy Ann Kilgo, PhD Indiana University, United States
Young K. Kim, PhD Azusa Pacific University, United States
Katie Koo, PhD University of Georgia, United States
Melanie N. Lee, PhD University of Utah, United States
Lucy A. LePeau, PhD Indiana University, United States
Luis Antonio Leyva, PhD Vanderbilt University, United States
Jameson D. Lopez, PhD University of Arizona, United States
Carol Lundberg, PhD California State University, Fullerton , United States
Ty C. McNamee, PhD The University of Mississippi, United States
Darris R. Means, PhD University of Pittsburgh, United States
Steve D. Mobley Jr., PhD Morgan State University, United States
Susana M. Muñoz, PhD Colorado State University–Fort Collins, United States
Brett Ranon Nachman, PhD University of Arkansas , United States
Bach Mai Dolly Nguyen, PhD Oregon State University, United States
Mike Hoa Nguyen, PhD New York University, United States
Sarah Nightingale, PhD Eastern Connecticut State University , United States
Gudrun Nyunt, PhD Northern Illinois University, United States
Wilson Kwamogi Okello, PhD Pennsylvania State University, United States
Oscar E. Patrón, PhD Indiana University, United States
Rosemary J. Perez, PhD University of Michigan, United States
Trang Pham, PhD Singapore University of Social Sciences, United States
Meghan J. Pifer, PhD University of Louisville, United States
Raechele L. Pope, EdD University of Buffalo, United States
Paul G. Rubin, PhD University of Utah, United States
Nicole Alia Salis Reyes, EdD University of Hawaiʻi at Mānoa, United States
Elizabeth E. Sparks, PhD Lynch School, Boston College (retired), United States Dian D. Squire, PhD Northern Arizona University, United States
Terah J. Stewart, PhD Iowa State University, United States
V. Thandi Sulé, PhD Oakland University, United States
Tenisha Tevis, PhD Oregon State University, United States
Jason K. Wallace, PhD Mississippi State University, United States
LaWanda W. M. Ward, JD, PhD Pennsylvania State University, United States
Stephanie J. Waterman, PhD University of Toronto, Canada
Melvin A. Whitehead, PhD Binghamton University, State University of New York, United States
Melanie M. Wilcox, PhD Augusta University, United States
Kerrie G. Wilkins-Yel, PhD University of Massachusetts Boston, United States
Brit Williams, PhD University of Vermont, United States
Michael Steven Williams, PhD University of Missouri, United States
Roger L. Worthington, PhD University of Maryland, College Park, United States
Raquel Wright-Mair, PhD Rowan University, United States
Christina W. Yao, PhD University of South Carolina, United States
Fanny Yeung, PhD California State University, East Bay , United States
Varaxy Yi, PhD California State University, Fresno , United States
Jing Yu, PhD University of Wisconsin-Madison , United States
Ximena U. Zúñiga, PhD University of Massachusetts Amherst, United States
Abstracting and indexing services providing coverage of Journal of Diversity in Higher Education
- Cabell's Directory of Publishing Opportunities in Psychology
- Current Contents: Social & Behavioral Sciences
- Education Source
- Journal Citations Report: Social Sciences Edition
- Social Sciences Citation Index
- Supplemental Index
- TOC Premier
Special issue of APA's Journal of Diversity in Higher Education, Vol. 9, No. 3, September 2016. The articles offer important insights into diversity-related concerns and how students may serve as agents of campus change as well as broader social change.
Special issue of APA's Journal of Diversity in Higher Education, Vol. 8, No. 3, September 2015. Includes articles about cultures of work-life balance, work-life satisfaction, career-life balance for women of color; and international faculty.
Special issue of APA's Journal of Diversity in Higher Education, Vol. 1, No. 4, December 2008. Articles examine the methods, findings, and implications of a multitude of campus climate research studies; report psychometric investigations on the measurement of unique dimensions of campus climate; and provide a conceptual framework for the assessment of campus climate that has been used at a large number of institutions.
Journal equity, diversity, and inclusion statement
Inclusive study designs.
- Collaborative research models
- Diverse samples
Definitions and further details on inclusive study designs are available on the Journals EDI homepage .
Inclusive reporting standards
- Bias-free language and community-driven language guidelines (required)
- Reflexivity (recommended)
- Positionality statements (recommended)
- Impact statements (recommended)
- Participant sample descriptions (recommended)
- Sample justifications (recommended)
- Inclusive reference lists (recommended)
More information on this journal’s reporting standards is listed under the submission guidelines tab .
Other EDI offerings
Masked peer review.
This journal offers masked peer review (where both the authors’ and reviewers’ identities are not known to the other). Research has shown that masked peer review can help reduce implicit bias against traditionally female names or early-career scientists with smaller publication records (Budden et al., 2008; Darling, 2015).
Recruitment
The journal practices intentional recruitment of individuals from diverse identity groups and cultural backgrounds as reviewers and editorial board members.
- Guidelines for reviewing manuscripts
Editor Spotlight
- Read an interview with Chris Linder, PhD
From APA Journals Article Spotlight ®
- Finding your people and your space on the internet: The web as a source of support for trans students of color
- How do recent college graduates navigate ideological bubbles? Findings from a longitudinal qualitative study
- The chief diversity officer
- A closer look at diversity: Understanding the place of race post-affirmative action
Journal Alert
Sign up to receive email alerts on the latest content published.
Welcome! Thank you for subscribing.
Subscriptions and access
- Pricing and individual access
- APA PsycArticles database
Calls for Papers
Access options
- APA publishing resources
- Educators and students
- Editor resource center
APA Publishing Insider
APA Publishing Insider is a free monthly newsletter with tips on APA Style, open science initiatives, active calls for papers, research summaries, and more.
Social media
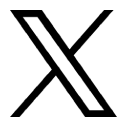
Contact Journals
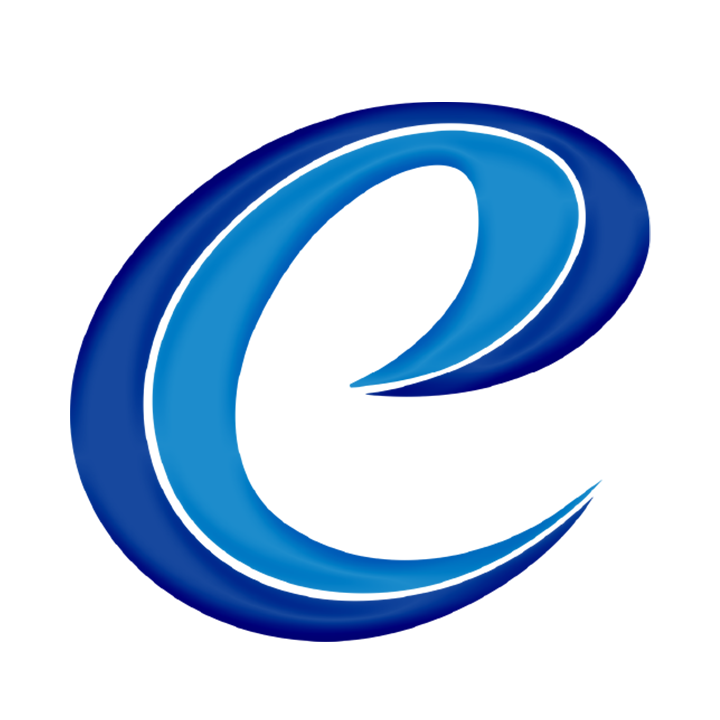
Exploring the Impact of Institutional Context on Experiential Education in Higher Education The importance of institutional culture, faculty relationships, and a home on the org chart.
Article sidebar, main article content.
In revisiting the Society for Experiential Education’s eight principles of good practice, members of the most recent cohort of the SEE fellows program explored the impact of institutional context on experiential education in higher education. Previous research outlines campus and institutional structures where experiential education takes place. Our group leveraged a scholar-practitioner frame and drew on action research methods to explore the experiences of each individual's context and experiential education structure. The findings are organized around four themes and three principles of good practice. Our team’s inquiry reveals that experiential education’s location and structure within the organization may impact how EE is valued and executed. The findings suggest that within higher education contexts the praxis of experiential education is directly connected to its institutional location, the institutional culture of DEI, its positioning as a value proposition, and the faculty relationships that can support it. The implications of this work showcase areas for consideration when institutionalizing experiential education in higher education and provide an opportunity for institutions to restructure where experiential education is positioned within the institution.
Article Details

This work is licensed under a Creative Commons Attribution 4.0 International License .
Elisa Avila, Texas A&M University – San Antonio
Elisa Avila is the Experiential and Service-Learning Coordinator at Texas A&M University – San Antonio. Her work ranges from planning the logistics of service-learning partnerships and supporting students as they schedule service, to providing ad-hoc reflection sessions, offering consulting support, and facilitating workshops with faculty. She loves facilitating conversations about teaching and equity, especially creating space for instructors to blend DEIB principles with the Kolb Learning Cycle. Elisa’s sociological research focuses on community, race, and environment (both built and natural). As a scholar-practitioner, she hopes to bridge the gap between the social contexts of higher education and the practicalities of supporting student success through experiential education. Elisa has an MS in Sociology from the University of Wisconsin-Madison, an MA in Social Sciences from the University of Chicago, and a BA in History and Sociology from Tulane University.
Rebekah Harriger, Harrisburg University of Science & Technology
Rebekah Harriger serves as the Senior Director of Career Services & Experiential Learning at Harrisburg University of Science and Technology. Rebekah’s passion for higher education began during her time at Shippensburg University. Through participation in the John L. Grove College of Business Internship Program, it ignited a desire for Rebekah to pursue a career in higher education specifically within internship program management and career and professional development. Through Rebekah’s work, she hopes by fostering the skills and confidence necessary to participate in internships and applied projects, these experiential learning opportunities will help to create a pathway to STEM careers. She was awarded by the Society for Experiential Education as the recipient of the Experiential Education Rising Leader Award in 2021. Rebekah holds a Bachelor of Science degree in Business Administration from Shippensburg University, and a Master of Arts in Higher Education from Geneva College and is a recent graduate from the Society for Experiential Education’s Experiential Education Academy in 2021.
Dennis McCunney, East Carolina University
Dennis McCunney serves as the Director of Intercultural Affairs and adjunct faculty member of ECU’s Leadership Studies Minor and Master of Public Administration Program. Dr. McCunney earned his PhD in Higher Education Administration from Morgan State University, and his dissertation focus was on the formation of student culture around civic engagement, leadership and activism. Dr. McCunney has presented his research in various arenas, including the Global Service-Learning Summit, Leadership Educators Institute, NASPA Annual Conference, and the Engagement Scholarship Consortium. Recent publications include articles in AAC&U’s Diversity & Democracy, New Directions in Higher Education, Metropolitan Universities Journal and Journal of Applied Research in Higher Education. His professional and research interests include public service and leadership development, organizational development, intercultural and global learning, and campus-community partnerships. He serves on the leadership team for the NASPA Spirituality and Religion Knowledge Community and serves as a board member for Amizade, a nonprofit that organizes safe, sustainable, and collaborative global learning experiences.
Benjamin Trager, University of Wisconsin - Milwaukee
Ben Trager holds a PhD in Urban Education from UWM and currently serves as the Director of Experiential Learning and Community Engagement at the University of Wisconsin - Milwaukee. As the director, Ben develops and supports university-wide vision and practices related to experiential learning, community-based learning, community partnerships, and co-curricular service. His scholarly and practical interests include the varied and changing purposes of higher education, the intersections of service-learning, internships, and other high impact practices, and participatory research methods. Ben has published work in The Michigan Journal of Community Service Learning and regularly presents at regional, national, and international conferences focused on education, community engagement, qualitative research, and educational philosophy.
- Research article
- Open access
- Published: 24 April 2023
Artificial intelligence in higher education: the state of the field
- Helen Crompton ORCID: orcid.org/0000-0002-1775-8219 1 , 3 &
- Diane Burke 2
International Journal of Educational Technology in Higher Education volume 20 , Article number: 22 ( 2023 ) Cite this article
115k Accesses
155 Citations
67 Altmetric
Metrics details
This systematic review provides unique findings with an up-to-date examination of artificial intelligence (AI) in higher education (HE) from 2016 to 2022. Using PRISMA principles and protocol, 138 articles were identified for a full examination. Using a priori, and grounded coding, the data from the 138 articles were extracted, analyzed, and coded. The findings of this study show that in 2021 and 2022, publications rose nearly two to three times the number of previous years. With this rapid rise in the number of AIEd HE publications, new trends have emerged. The findings show that research was conducted in six of the seven continents of the world. The trend has shifted from the US to China leading in the number of publications. Another new trend is in the researcher affiliation as prior studies showed a lack of researchers from departments of education. This has now changed to be the most dominant department. Undergraduate students were the most studied students at 72%. Similar to the findings of other studies, language learning was the most common subject domain. This included writing, reading, and vocabulary acquisition. In examination of who the AIEd was intended for 72% of the studies focused on students, 17% instructors, and 11% managers. In answering the overarching question of how AIEd was used in HE, grounded coding was used. Five usage codes emerged from the data: (1) Assessment/Evaluation, (2) Predicting, (3) AI Assistant, (4) Intelligent Tutoring System (ITS), and (5) Managing Student Learning. This systematic review revealed gaps in the literature to be used as a springboard for future researchers, including new tools, such as Chat GPT.
A systematic review examining AIEd in higher education (HE) up to the end of 2022.
Unique findings in the switch from US to China in the most studies published.
A two to threefold increase in studies published in 2021 and 2022 to prior years.
AIEd was used for: Assessment/Evaluation, Predicting, AI Assistant, Intelligent Tutoring System, and Managing Student Learning.
Introduction
The use of artificial intelligence (AI) in higher education (HE) has risen quickly in the last 5 years (Chu et al., 2022 ), with a concomitant proliferation of new AI tools available. Scholars (viz., Chen et al., 2020 ; Crompton et al., 2020 , 2021 ) report on the affordances of AI to both instructors and students in HE. These benefits include the use of AI in HE to adapt instruction to the needs of different types of learners (Verdú et al., 2017 ), in providing customized prompt feedback (Dever et al., 2020 ), in developing assessments (Baykasoğlu et al., 2018 ), and predict academic success (Çağataylı & Çelebi, 2022 ). These studies help to inform educators about how artificial intelligence in education (AIEd) can be used in higher education.
Nonetheless, a gap has been highlighted by scholars (viz., Hrastinski et al., 2019 ; Zawacki-Richter et al., 2019 ) regarding an understanding of the collective affordances provided through the use of AI in HE. Therefore, the purpose of this study is to examine extant research from 2016 to 2022 to provide an up-to-date systematic review of how AI is being used in the HE context.
Artificial intelligence has become pervasive in the lives of twenty-first century citizens and is being proclaimed as a tool that can be used to enhance and advance all sectors of our lives (Górriz et al., 2020 ). The application of AI has attracted great interest in HE which is highly influenced by the development of information and communication technologies (Alajmi et al., 2020 ). AI is a tool used across subject disciplines, including language education (Liang et al., 2021 ), engineering education (Shukla et al., 2019 ), mathematics education (Hwang & Tu, 2021 ) and medical education (Winkler-Schwartz et al., 2019 ),
Artificial intelligence
The term artificial intelligence is not new. It was coined in 1956 by McCarthy (Cristianini, 2016 ) who followed up on the work of Turing (e.g., Turing, 1937 , 1950 ). Turing described the existence of intelligent reasoning and thinking that could go into intelligent machines. The definition of AI has grown and changed since 1956, as there has been significant advancements in AI capabilities. A current definition of AI is “computing systems that are able to engage in human-like processes such as learning, adapting, synthesizing, self-correction and the use of data for complex processing tasks” (Popenici et al., 2017 , p. 2). The interdisciplinary interest from scholars from linguistics, psychology, education, and neuroscience who connect AI to nomenclature, perceptions and knowledge in their own disciplines could create a challenge when defining AI. This has created the need to create categories of AI within specific disciplinary areas. This paper focuses on the category of AI in Education (AIEd) and how AI is specifically used in higher educational contexts.
As the field of AIEd is growing and changing rapidly, there is a need to increase the academic understanding of AIEd. Scholars (viz., Hrastinski et al., 2019 ; Zawacki-Richter et al., 2019 ) have drawn attention to the need to increase the understanding of the power of AIEd in educational contexts. The following section provides a summary of the previous research regarding AIEd.
Extant systematic reviews
This growing interest in AIEd has led scholars to investigate the research on the use of artificial intelligence in education. Some scholars have conducted systematic reviews to focus on a specific subject domain. For example, Liang et. al. ( 2021 ) conducted a systematic review and bibliographic analysis the roles and research foci of AI in language education. Shukla et. al. ( 2019 ) focused their longitudinal bibliometric analysis on 30 years of using AI in Engineering. Hwang and Tu ( 2021 ) conducted a bibliometric mapping analysis on the roles and trends in the use of AI in mathematics education, and Winkler-Schwartz et. al. ( 2019 ) specifically examined the use of AI in medical education in looking for best practices in the use of machine learning to assess surgical expertise. These studies provide a specific focus on the use of AIEd in HE but do not provide an understanding of AI across HE.
On a broader view of AIEd in HE, Ouyang et. al. ( 2022 ) conducted a systematic review of AIEd in online higher education and investigated the literature regarding the use of AI from 2011 to 2020. The findings show that performance prediction, resource recommendation, automatic assessment, and improvement of learning experiences are the four main functions of AI applications in online higher education. Salas-Pilco and Yang ( 2022 ) focused on AI applications in Latin American higher education. The results revealed that the main AI applications in higher education in Latin America are: (1) predictive modeling, (2) intelligent analytics, (3) assistive technology, (4) automatic content analysis, and (5) image analytics. These studies provide valuable information for the online and Latin American context but not an overarching examination of AIEd in HE.
Studies have been conducted to examine HE. Hinojo-Lucena et. al. ( 2019 ) conducted a bibliometric study on the impact of AIEd in HE. They analyzed the scientific production of AIEd HE publications indexed in Web of Science and Scopus databases from 2007 to 2017. This study revealed that most of the published document types were proceedings papers. The United States had the highest number of publications, and the most cited articles were about implementing virtual tutoring to improve learning. Chu et. al. ( 2022 ) reviewed the top 50 most cited articles on AI in HE from 1996 to 2020, revealing that predictions of students’ learning status were most frequently discussed. AI technology was most frequently applied in engineering courses, and AI technologies most often had a role in profiling and prediction. Finally, Zawacki-Richter et. al. ( 2019 ) analyzed AIEd in HE from 2007 to 2018 to reveal four primary uses of AIEd: (1) profiling and prediction, (2) assessment and evaluation, (3) adaptive systems and personalization, and (4) intelligent tutoring systems. There do not appear to be any studies examining the last 2 years of AIEd in HE, and these authors describe the rapid speed of both AI development and the use of AIEd in HE and call for further research in this area.
Purpose of the study
The purpose of this study is in response to the appeal from scholars (viz., Chu et al., 2022 ; Hinojo-Lucena et al., 2019 ; Zawacki-Richter et al., 2019 ) to research to investigate the benefits and challenges of AIEd within HE settings. As the academic knowledge of AIEd HE finished with studies examining up to 2020, this study provides the most up-to-date analysis examining research through to the end of 2022.
The overarching question for this study is: what are the trends in HE research regarding the use of AIEd? The first two questions provide contextual information, such as where the studies occurred and the disciplines AI was used in. These contextual details are important for presenting the main findings of the third question of how AI is being used in HE.
In what geographical location was the AIEd research conducted, and how has the trend in the number of publications evolved across the years?
What departments were the first authors affiliated with, and what were the academic levels and subject domains in which AIEd research was being conducted?
Who are the intended users of the AI technologies and what are the applications of AI in higher education?
A PRISMA systematic review methodology was used to answer three questions guiding this study. PRISMA principles (Page et al., 2021 ) were used throughout the study. The PRISMA extension Preferred Reporting Items for Systematic Reviews and Meta-Analysis for Protocols (PRISMA-P; Moher et al., 2015 ) were utilized in this study to provide an a priori roadmap to conduct a rigorous systematic review. Furthermore, the Preferred Reporting Items for Systematic Reviews and Meta-Analysis (PRISMA principles; Page et al., 2021 ) were used to search, identify, and select articles to be included in the research were used for searching, identifying, and selecting articles, then in how to read, extract, and manage the secondary data gathered from those studies (Moher et al., 2015 , PRISMA Statement, 2021 ). This systematic review approach supports an unbiased synthesis of the data in an impartial way (Hemingway & Brereton, 2009 ). Within the systematic review methodology, extracted data were aggregated and presented as whole numbers and percentages. A qualitative deductive and inductive coding methodology was also used to analyze extant data and generate new theories on the use of AI in HE (Gough et al., 2017 ).
The research begins with the search for the research articles to be included in the study. Based on the research question, the study parameters are defined including the search years, quality and types of publications to be included. Next, databases and journals are selected. A Boolean search is created and used for the search of those databases and journals. Once a set of publications are located from those searches, they are then examined against an inclusion and exclusion criteria to determine which studies will be included in the final study. The relevant data to match the research questions is then extracted from the final set of studies and coded. This method section is organized to describe each of these methods with full details to ensure transparency.
Search strategy
Only peer-reviewed journal articles were selected for examination in this systematic review. This ensured a level of confidence in the quality of the studies selected (Gough et al., 2017 ). The search parameters narrowed the search focus to include studies published in 2016 to 2022. This timeframe was selected to ensure the research was up to date, which is especially important with the rapid change in technology and AIEd.
The data retrieval protocol employed an electronic and a hand search. The electronic search included educational databases within EBSCOhost. Then an additional electronic search was conducted of Wiley Online Library, JSTOR, Science Direct, and Web of Science. Within each of these databases a full text search was conducted. Aligned to the research topic and questions, the Boolean search included terms related to AI, higher education, and learning. The Boolean search is listed in Table 1 . In the initial test search, the terms “machine learning” OR “intelligent support” OR “intelligent virtual reality” OR “chatbot” OR “automated tutor” OR “intelligent agent” OR “expert system” OR “neural network” OR “natural language processing” were used. These were removed as they were subcategories of terms found in Part 1 of the search. Furthermore, inclusion of these specific AI terms resulted in a large number of computer science courses that were focused on learning about AI and not the use of AI in learning.
Part 2 of the search ensured that articles involved formal university education. The terms higher education and tertiary were both used to recognize the different terms used in different countries. The final Boolean search was “Artificial intelligence” OR AI OR “smart technologies” OR “intelligent technologies” AND “higher education” OR tertiary OR graduate OR undergraduate. Scholars (viz., Ouyang et al., 2022 ) who conducted a systematic review on AIEd in HE up to 2020 noted that they missed relevant articles from their study, and other relevant journals should intentionally be examined. Therefore, a hand search was also conducted to include an examination of other journals relevant to AIEd that may not be included in the databases. This is important as the field of AIEd is still relatively new, and journals focused on this field may not yet be indexed in databases. The hand search included: The International Journal of Learning Analytics and Artificial Intelligence in Education, the International Journal of Artificial Intelligence in Education, and Computers & Education: Artificial Intelligence.
Electronic and hand searches resulted in 371 articles for possible inclusion. The search parameters within the electronic database search narrowed the search to articles published from 2016 to 2022, per-reviewed journal articles, and duplicates. Further screening was conducted manually, as each of the 138 articles were reviewed in full by two researchers to examine a match against the inclusion and exclusion criteria found in Table 2 .
The inter-rater reliability was calculated by percentage agreement (Belur et al., 2018 ). The researchers reached a 95% agreement for the coding. Further discussion of misaligned articles resulted in a 100% agreement. This screening process against inclusion and exclusion criteria resulted in the exclusion of 237 articles. This included the duplicates and those removed as part of the inclusion and exclusion criteria, see Fig. 1 . Leaving 138 articles for inclusion in this systematic review.
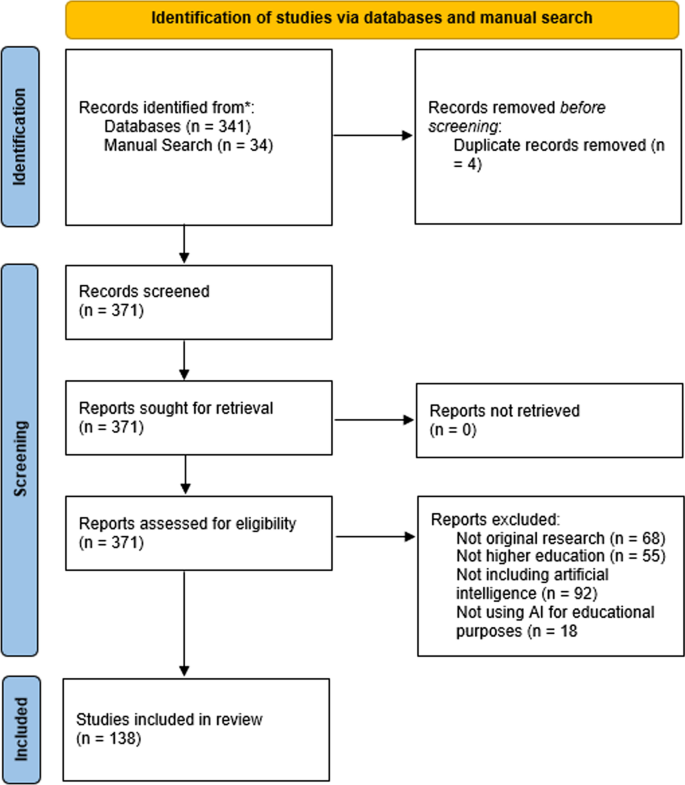
(From: Page et al., 2021 )
PRISMA flow chart of article identification and screening
The 138 articles were then coded to answer each of the research questions using deductive and inductive coding methods. Deductive coding involves examining data using a priori codes. A priori are pre-determined criteria and this process was used to code the countries, years, author affiliations, academic levels, and domains in the respective groups. Author affiliations were coded using the academic department of the first author of the study. First authors were chosen as that person is the primary researcher of the study and this follows past research practice (e.g., Zawacki-Richter et al., 2019 ). Who the AI was intended for was also coded using the a priori codes of Student, Instructor, Manager or Others. The Manager code was used for those who are involved in organizational tasks, e.g., tracking enrollment. Others was used for those not fitting the other three categories.
Inductive coding was used for the overarching question of this study in examining how the AI was being used in HE. Researchers of extant systematic reviews on AIEd in HE (viz., Chu et al., 2022 ; Zawacki-Richter et al., 2019 ) often used an a priori framework as researchers matched the use of AI to pre-existing frameworks. A grounded coding methodology (Strauss & Corbin, 1995 ) was selected for this study to allow findings of the trends on AIEd in HE to emerge from the data. This is important as it allows a direct understanding of how AI is being used rather than how researchers may think it is being used and fitting the data to pre-existing ideas.
Grounded coding process involved extracting how the AI was being used in HE from the articles. “In vivo” (Saldana, 2015 ) coding was also used alongside grounded coding. In vivo codes are when codes use language directly from the article to capture the primary authors’ language and ensure consistency with their findings. The grounded coding design used a constant comparative method. Researchers identified important text from articles related to the use of AI, and through an iterative process, initial codes led to axial codes with a constant comparison of uses of AI with uses of AI, then of uses of AI with codes, and codes with codes. Codes were deemed theoretically saturated when the majority of the data fit with one of the codes. For both the a priori and the grounded coding, two researchers coded and reached an inter-rater percentage agreement of 96%. After discussing misaligned articles, a 100% agreement was achieved.

Findings and discussion
The findings and discussion section are organized by the three questions guiding this study. The first two questions provide contextual information on the AIEd research, and the final question provides a rigorous investigation into how AI is being used in HE.
RQ1. In what geographical location was the AIEd research conducted, and how has the trend in the number of publications evolved across the years?
The 138 studies took place across 31 countries in six of seven continents of the world. Nonetheless, that distribution was not equal across continents. Asia had the largest number of AIEd studies in HE at 41%. Of the seven countries represented in Asia, 42 of the 58 studies were conducted in Taiwan and China. Europe, at 30%, was the second largest continent and had 15 countries ranging from one to eight studies a piece. North America, at 21% of the studies was the continent with the third largest number of studies, with the USA producing 21 of the 29 studies in that continent. The 21 studies from the USA places it second behind China. Only 1% of studies were conducted in South America and 2% in Africa. See Fig. 2 for a visual representation of study distribution across countries. Those continents with high numbers of studies are from high income countries and those with low numbers have a paucity of publications in low-income countries.
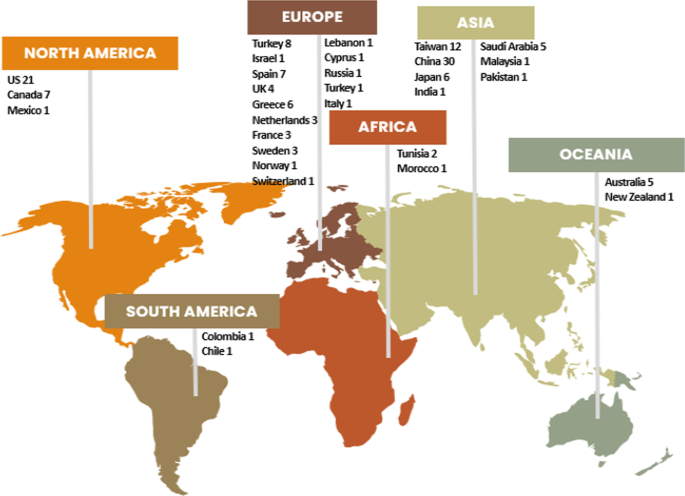
Geographical distribution of the AIEd HE studies
Data from Zawacki-Richter et. al.’s ( 2019 ) 2007–2018 systematic review examining countries found that the USA conducted the most studies across the globe at 43 out of 146, and China had the second largest at eleven of the 146 papers. Researchers have noted a rapid trend in Chinese researchers publishing more papers on AI and securing more patents than their US counterparts in a field that was originally led by the US (viz., Li et al., 2021 ). The data from this study corroborate this trend in China leading in the number of AIEd publications.
With the accelerated use of AI in society, gathering data to examine the use of AIEd in HE is useful in providing the scholarly community with specific information on that growth and if it is as prolific as anticipated by scholars (e.g., Chu et al., 2022 ). The analysis of data of the 138 studies shows that the trend towards the use of AIEd in HE has greatly increased. There is a drop in 2019, but then a great rise in 2021 and 2022; see Fig. 3 .
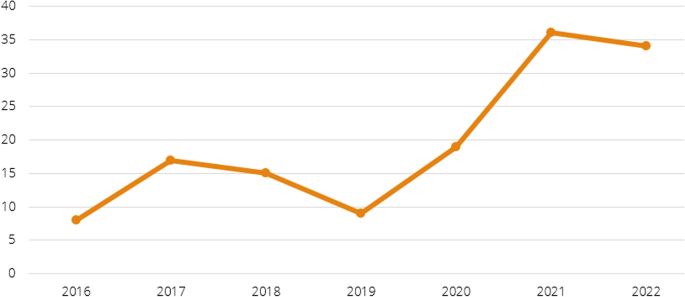
Chronological trend in AIEd in HE
Data on the rise in AIEd in HE is similar to the findings of Chu et. al. ( 2022 ) who noted an increase from 1996 to 2010 and 2011–2020. Nonetheless Chu’s parameters are across decades, and the rise is to be anticipated with a relatively new technology across a longitudinal review. Data from this study show a dramatic rise since 2020 with a 150% increase from the prior 2 years 2020–2019. The rise in 2021 and 2022 in HE could have been caused by the vast increase in HE faculty having to teach with technology during the pandemic lockdown. Faculty worldwide were using technologies, including AI, to explore how they could continue teaching and learning that was often face-to-face prior to lockdown. The disadvantage of this rapid adoption of technology is that there was little time to explore the possibilities of AI to transform learning, and AI may have been used to replicate past teaching practices, without considering new strategies previously inconceivable with the affordances of AI.
However, in a further examination of the research from 2021 to 2022, it appears that there are new strategies being considered. For example, Liu et. al.’s, 2022 study used AIEd to provide information on students’ interactions in an online environment and examine their cognitive effort. In Yao’s study in 2022, he examined the use of AI to determine student emotions while learning.
RQ2. What departments were the first authors affiliated with, and what were the academic levels and subject domains in which AIEd research was being conducted?
Department affiliations
Data from the AIEd HE studies show that of the first authors were most frequently from colleges of education (28%), followed by computer science (20%). Figure 4 presents the 15 academic affiliations of the authors found in the studies. The wide variety of affiliations demonstrate the variety of ways AI can be used in various educational disciplines, and how faculty in diverse areas, including tourism, music, and public affairs were interested in how AI can be used for educational purposes.
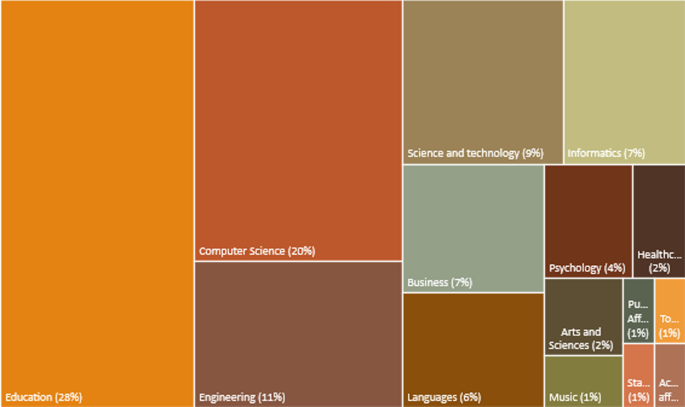
Research affiliations
In an extant AIED HE systematic review, Zawacki-Richter et. al.’s ( 2019 ) named their study Systematic review of research on artificial intelligence applications in higher education—where are the educators? In this study, the authors were keen to highlight that of the AIEd studies in HE, only six percent were written by researchers directly connected to the field of education, (i.e., from a college of education). The researchers found a great lack in pedagogical and ethical implications of implementing AI in HE and that there was a need for more educational perspectives on AI developments from educators conducting this work. It appears from our data that educators are now showing greater interest in leading these research endeavors, with the highest affiliated group belonging to education. This may again be due to the pandemic and those in the field of education needing to support faculty in other disciplines, and/or that they themselves needed to explore technologies for their own teaching during the lockdown. This may also be due to uptake in professors in education becoming familiar with AI tools also driven by a societal increased attention. As the focus of much research by education faculty is on teaching and learning, they are in an important position to be able to share their research with faculty in other disciplines regarding the potential affordances of AIEd.
Academic levels
The a priori coding of academic levels show that the majority of studies involved undergraduate students with 99 of the 138 (72%) focused on these students. This was in comparison to the 12 of 138 (9%) for graduate students. Some of the studies used AI for both academic levels: see Fig. 5
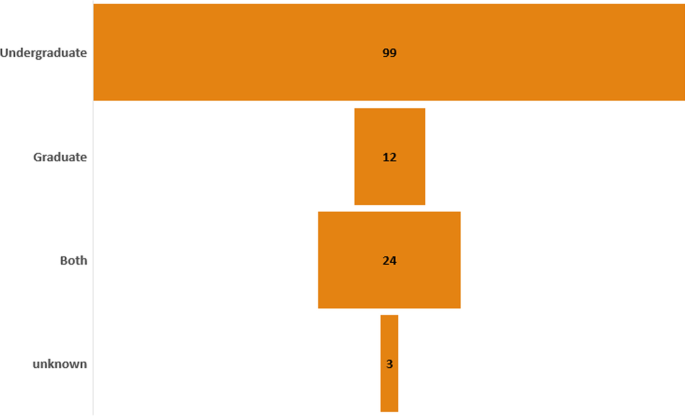
Academic level distribution by number of articles
This high percentage of studies focused on the undergraduate population was congruent with an earlier AIED HE systematic review (viz., Zawacki-Richter et al., 2019 ) who also reported student academic levels. This focus on undergraduate students may be due to the variety of affordances offered by AIEd, such as predictive analytics on dropouts and academic performance. These uses of AI may be less required for graduate students who already have a record of performance from their undergraduate years. Another reason for this demographic focus can also be convenience sampling, as researchers in HE typically has a much larger and accessible undergraduate population than graduates. This disparity between undergraduates and graduate populations is a concern, as AIEd has the potential to be valuable in both settings.
Subject domains
The studies were coded into 14 areas in HE; with 13 in a subject domain and one category of AIEd used in HE management of students; See Fig. 6 . There is not a wide difference in the percentages of top subject domains, with language learning at 17%, computer science at 16%, and engineering at 12%. The management of students category appeared third on the list at 14%. Prior studies have also found AIEd often used for language learning (viz., Crompton et al., 2021 ; Zawacki-Richter et al., 2019 ). These results are different, however, from Chu et. al.’s ( 2022 ) findings that show engineering dramatically leading with 20 of the 50 studies, with other subjects, such as language learning, appearing once or twice. This study appears to be an outlier that while the searches were conducted in similar databases, the studies only included 50 studies from 1996 to 2020.
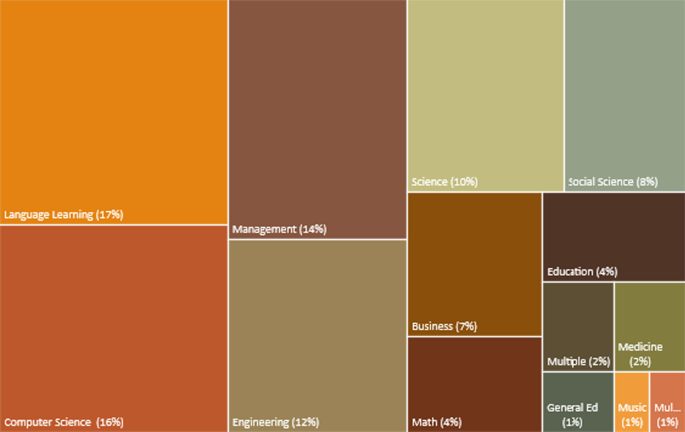
Subject domains of AIEd in HE
Previous scholars primarily focusing on language learning using AI for writing, reading, and vocabulary acquisition used the affordances of natural language processing and intelligent tutoring systems (e.g., Liang et al., 2021 ). This is similar to the findings in studies with AI used for automated feedback of writing in a foreign language (Ayse et al., 2022 ), and AI translation support (Al-Tuwayrish, 2016 ). The large use of AI for managerial activities in this systematic review focused on making predictions (12 studies) and then admissions (three studies). This is positive to see this use of AI to look across multiple databases to see trends emerging from data that may not have been anticipated and cross referenced before (Crompton et al., 2022 ). For example, to examine dropouts, researchers may consider examining class attendance, and may not examine other factors that appear unrelated. AI analysis can examine all factors and may find that dropping out is due to factors beyond class attendance.
RQ3. Who are the intended users of the AI technologies and what are the applications of AI in higher education?
Intended user of AI
Of the 138 articles, the a priori coding shows that 72% of the studies focused on Students, followed by a focus on Instructors at 17%, and Managers at 11%, see Fig. 7 . The studies provided examples of AI being used to provide support to students, such as access to learning materials for inclusive learning (Gupta & Chen, 2022 ), provide immediate answers to student questions, self-testing opportunities (Yao, 2022 ), and instant personalized feedback (Mousavi et al., 2020 ).
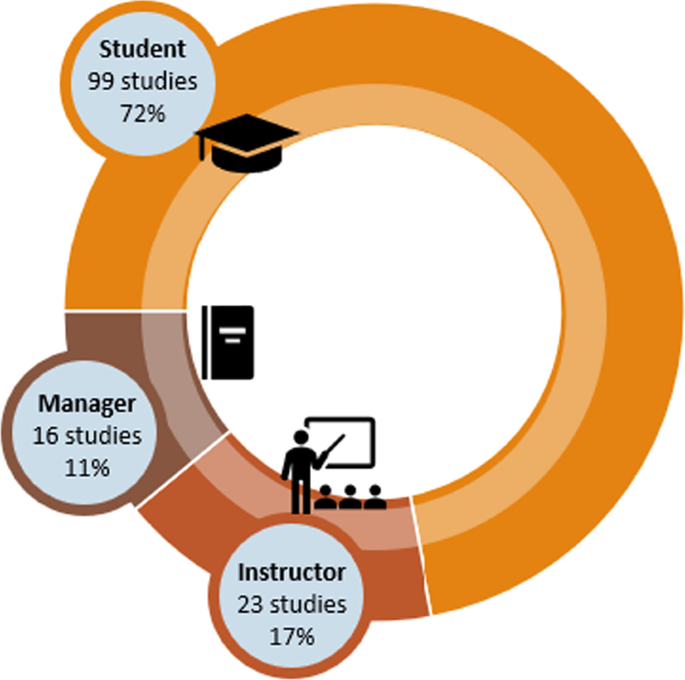
Intended user
The data revealed a large emphasis on students in the use of AIEd in HE. This user focus is different from a recent systematic review on AIEd in K-12 that found that AIEd studies in K-12 settings prioritized teachers (Crompton et al., 2022 ). This may appear that HE uses AI to focus more on students than in K-12. However, this large number of student studies in HE may be due to the student population being more easily accessibility to HE researchers who may study their own students. The ethical review process is also typically much shorter in HE than in K-12. Therefore, the data on the intended focus should be reviewed while keeping in mind these other explanations. It was interesting that Managers were the lowest focus in K-12 and also in this study in HE. AI has great potential to collect, cross reference and examine data across large datasets that can allow data to be used for actionable insight. More focus on the use of AI by managers would tap into this potential.
How is AI used in HE
Using grounded coding, the use of AIEd from each of the 138 articles was examined and six major codes emerged from the data. These codes provide insight into how AI was used in HE. The five codes are: (1) Assessment/Evaluation, (2) Predicting, (3) AI Assistant, (4) Intelligent Tutoring System (ITS), and (5) Managing Student Learning. For each of these codes there are also axial codes, which are secondary codes as subcategories from the main category. Each code is delineated below with a figure of the codes with further descriptive information and examples.
Assessment/evaluation
Assessment and Evaluation was the most common use of AIEd in HE. Within this code there were six axial codes broken down into further codes; see Fig. 8 . Automatic assessment was most common, seen in 26 of the studies. It was interesting to see that this involved assessment of academic achievement, but also other factors, such as affect.
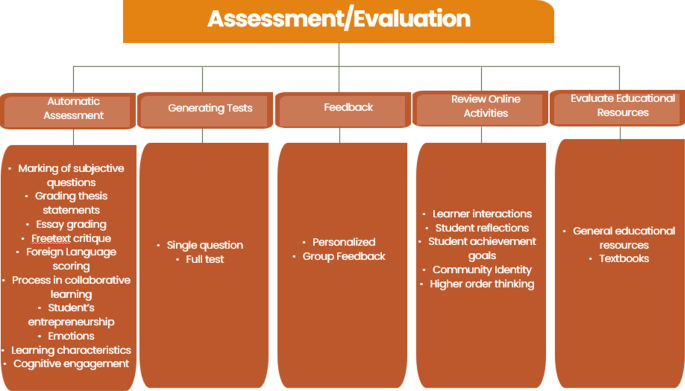
Codes and axial codes for assessment and evaluation
Automatic assessment was used to support a variety of learners in HE. As well as reducing the time it takes for instructors to grade (Rutner & Scott, 2022 ), automatic grading showed positive use for a variety of students with diverse needs. For example, Zhang and Xu ( 2022 ) used automatic assessment to improve academic writing skills of Uyghur ethnic minority students living in China. Writing has a variety of cultural nuances and in this study the students were shown to engage with the automatic assessment system behaviorally, cognitively, and affectively. This allowed the students to engage in self-regulated learning while improving their writing.
Feedback was a description often used in the studies, as students were given text and/or images as feedback as a formative evaluation. Mousavi et. al. ( 2020 ) developed a system to provide first year biology students with an automated personalized feedback system tailored to the students’ specific demographics, attributes, and academic status. With the unique feature of AIEd being able to analyze multiple data sets involving a variety of different students, AI was used to assess and provide feedback on students’ group work (viz., Ouatik et al., 2021 ).
AI also supports instructors in generating questions and creating multiple question tests (Yang et al., 2021 ). For example, (Lu et al., 2021 ) used natural language processing to create a system that automatically created tests. Following a Turing type test, researchers found that AI technologies can generate highly realistic short-answer questions. The ability for AI to develop multiple questions is a highly valuable affordance as tests can take a great deal of time to make. However, it would be important for instructors to always confirm questions provided by the AI to ensure they are correct and that they match the learning objectives for the class, especially in high value summative assessments.
The axial code within assessment and evaluation revealed that AI was used to review activities in the online space. This included evaluating student’s reflections, achievement goals, community identity, and higher order thinking (viz., Huang et al., 2021 ). Three studies used AIEd to evaluate educational materials. This included general resources and textbooks (viz., Koć‑Januchta et al., 2022 ). It is interesting to see the use of AI for the assessment of educational products, rather than educational artifacts developed by students. While this process may be very similar in nature, this shows researchers thinking beyond the traditional use of AI for assessment to provide other affordances.
Predicting was a common use of AIEd in HE with 21 studies focused specifically on the use of AI for forecasting trends in data. Ten axial codes emerged on the way AI was used to predict different topics, with nine focused on predictions regarding students and the other on predicting the future of higher education. See Fig. 9 .
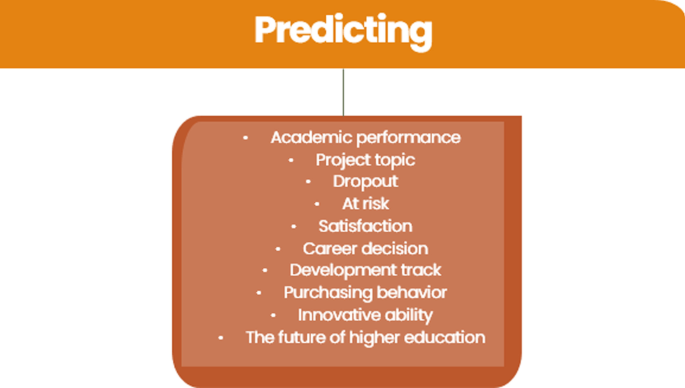
Predicting axial codes
Extant systematic reviews on HE highlighted the use of AIEd for prediction (viz., Chu et al., 2022 ; Hinojo-Lucena et al., 2019 ; Ouyang et al., 2022 ; Zawacki-Richter et al., 2019 ). Ten of the articles in this study used AI for predicting academic performance. Many of the axial codes were often overlapping, such as predicting at risk students, and predicting dropouts; however, each provided distinct affordances. An example of this is the study by Qian et. al. ( 2021 ). These researchers examined students taking a MOOC course. MOOCs can be challenging environments to determine information on individual students with the vast number of students taking the course (Krause & Lowe, 2014 ). However, Qian et al., used AIEd to predict students’ future grades by inputting 17 different learning features, including past grades, into an artificial neural network. The findings were able to predict students’ grades and highlight students at risk of dropping out of the course.
In a systematic review on AIEd within the K-12 context (viz., Crompton et al., 2022 ), prediction was less pronounced in the findings. In the K-12 setting, there was a brief mention of the use of AI in predicting student academic performance. One of the studies mentioned students at risk of dropping out, but this was immediately followed by questions about privacy concerns and describing this as “sensitive”. The use of prediction from the data in this HE systematic review cover a wide range of AI predictive affordances. students Sensitivity is still important in a HE setting, but it is positive to see the valuable insight it provides that can be used to avoid students failing in their goals.
AI assistant
The studies evaluated in this review indicated that the AI Assistant used to support learners had a variety of different names. This code included nomenclature such as, virtual assistant, virtual agent, intelligent agent, intelligent tutor, and intelligent helper. Crompton et. al. ( 2022 ), described the difference in the terms to delineate the way that the AI appeared to the user. For example, if there was an anthropomorphic presence to the AI, such as an avatar, or if the AI appeared to support via other means, such as text prompt. The findings of this systematic review align to Crompton et. al.’s ( 2022 ) descriptive differences of the AI Assistant. Furthermore, this code included studies that provide assistance to students, but may not have specifically used the word assistance. These include the use of chatbots for student outreach, answering questions, and providing other assistance. See Fig. 10 for the axial codes for AI Assistant.
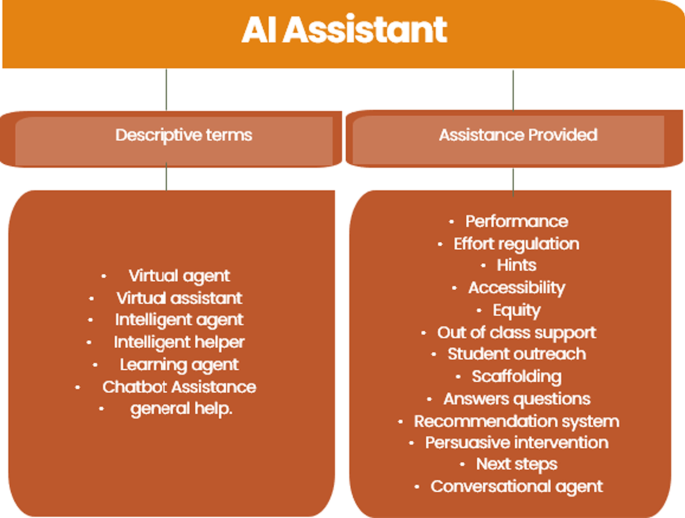
AI assistant axial codes
Many of these assistants offered multiple supports to students, such as Alex , the AI described as a virtual change agent in Kim and Bennekin’s ( 2016 ) study. Alex interacted with students in a college mathematics course by asking diagnostic questions and gave support depending on student needs. Alex’s support was organized into four stages: (1) goal initiation (“Want it”), (2) goal formation (“Plan for it”), (3) action control (“Do it”), and (4) emotion control (“Finish it”). Alex provided responses depending on which of these four areas students needed help. These messages supported students with the aim of encouraging persistence in pursuing their studies and degree programs and improving performance.
The role of AI in providing assistance connects back to the seminal work of Vygotsky ( 1978 ) and the Zone of Proximal Development (ZPD). ZPD highlights the degree to which students can rapidly develop when assisted. Vygotsky described this assistance often in the form of a person. However, with technological advancements, the use of AI assistants in these studies are providing that support for students. The affordances of AI can also ensure that the support is timely without waiting for a person to be available. Also, assistance can consider aspects on students’ academic ability, preferences, and best strategies for supporting. These features were evident in Kim and Bennekin’s ( 2016 ) study using Alex.
Intelligent tutoring system
The use of Intelligent Tutoring Systems (ITS) was revealed in the grounded coding. ITS systems are adaptive instructional systems that involve the use of AI techniques and educational methods. An ITS system customizes educational activities and strategies based on student’s characteristics and needs (Mousavinasab et al., 2021 ). While ITS may be an anticipated finding in AIED HE systematic reviews, it was interesting that extant reviews similar to this study did not always describe their use in HE. For example, Ouyang et. al. ( 2022 ), included “intelligent tutoring system” in search terms describing it as a common technique, yet ITS was not mentioned again in the paper. Zawacki-Richter et. al. ( 2019 ) on the other hand noted that ITS was in the four overarching findings of the use of AIEd in HE. Chu et. al. ( 2022 ) then used Zawacki-Richter’s four uses of AIEd for their recent systematic review.
In this systematic review, 18 studies specifically mentioned that they were using an ITS. The ITS code did not necessitate axial codes as they were performing the same type of function in HE, namely, in providing adaptive instruction to the students. For example, de Chiusole et. al. ( 2020 ) developed Stat-Knowlab, an ITS that provides the level of competence and best learning path for each student. Thus Stat-Knowlab personalizes students’ learning and provides only educational activities that the student is ready to learn. This ITS is able to monitor the evolution of the learning process as the student interacts with the system. In another study, Khalfallah and Slama ( 2018 ) built an ITS called LabTutor for engineering students. LabTutor served as an experienced instructor in enabling students to access and perform experiments on laboratory equipment while adapting to the profile of each student.
The student population in university classes can go into the hundreds and with the advent of MOOCS, class sizes can even go into the thousands. Even in small classes of 20 students, the instructor cannot physically provide immediate unique personalize questions to each student. Instructors need time to read and check answers and then take further time to provide feedback before determining what the next question should be. Working with the instructor, AIEd can provide that immediate instruction, guidance, feedback, and following questioning without delay or becoming tired. This appears to be an effective use of AIEd, especially within the HE context.
Managing student learning
Another code that emerged in the grounded coding was focused on the use of AI for managing student learning. AI is accessed to manage student learning by the administrator or instructor to provide information, organization, and data analysis. The axial codes reveal the trends in the use of AI in managing student learning; see Fig. 11 .
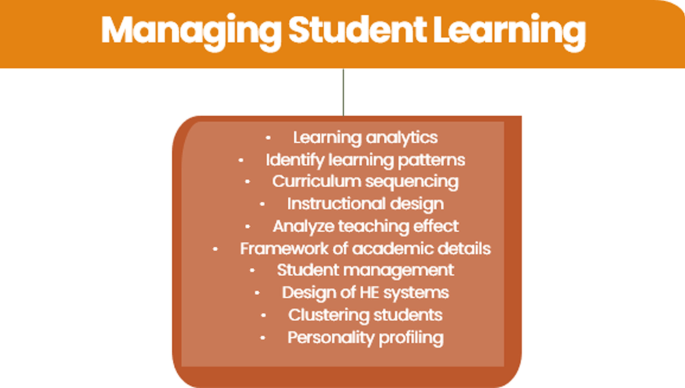
Learning analytics was an a priori term often found in studies which describes “the measurement, collection, analysis and reporting of data about learners and their contexts, for purposes of understanding and optimizing learning and the environments in which it occurs” (Long & Siemens, 2011 , p. 34). The studies investigated in this systematic review were across grades and subject areas and provided administrators and instructors different types of information to guide their work. One of those studies was conducted by Mavrikis et. al. ( 2019 ) who described learning analytics as teacher assistance tools. In their study, learning analytics were used in an exploratory learning environment with targeted visualizations supporting classroom orchestration. These visualizations, displayed as screenshots in the study, provided information such as the interactions between the students, goals achievements etc. These appear similar to infographics that are brightly colored and draw the eye quickly to pertinent information. AI is also used for other tasks, such as organizing the sequence of curriculum in pacing guides for future groups of students and also designing instruction. Zhang ( 2022 ) described how designing an AI teaching system of talent cultivation and using the digital affordances to establish a quality assurance system for practical teaching, provides new mechanisms for the design of university education systems. In developing such a system, Zhang found that the stability of the instructional design, overcame the drawbacks of traditional manual subjectivity in the instructional design.
Another trend that emerged from the studies was the use of AI to manage student big data to support learning. Ullah and Hafiz ( 2022 ) lament that using traditional methods, including non-AI digital techniques, asking the instructor to pay attention to every student’s learning progress is very difficult and that big data analysis techniques are needed. The ability to look across and within large data sets to inform instruction is a valuable affordance of AIEd in HE. While the use of AIEd to manage student learning emerged from the data, this study uncovered only 19 studies in 7 years (2016–2022) that focused on the use of AIEd to manage student data. This lack of the use was also noted in a recent study in the K-12 space (Crompton et al., 2022 ). In Chu et. al.’s ( 2022 ) study examining the top 50 most cited AIEd articles, they did not report the use of AIEd for managing student data in the top uses of AIEd HE. It would appear that more research should be conducted in this area to fully explore the possibilities of AI.
Gaps and future research
From this systematic review, six gaps emerged in the data providing opportunities for future studies to investigate and provide a fuller understanding of how AIEd can used in HE. (1) The majority of the research was conducted in high income countries revealing a paucity of research in developing countries. More research should be conducted in these developing countries to expand the level of understanding about how AI can enhance learning in under-resourced communities. (2) Almost 50% of the studies were conducted in the areas of language learning, computer science and engineering. Research conducted by members from multiple, different academic departments would help to advance the knowledge of the use of AI in more disciplines. (3) This study revealed that faculty affiliated with schools of education are taking an increasing role in researching the use of AIEd in HE. As this body of knowledge grows, faculty in Schools of Education should share their research regarding the pedagogical affordances of AI so that this knowledge can be applied by faculty across disciplines. (4) The vast majority of the research was conducted at the undergraduate level. More research needs to be done at the graduate student level, as AI provides many opportunities in this environment. (5) Little study was done regarding how AIEd can assist both instructors and managers in their roles in HE. The power of AI to assist both groups further research. (6) Finally, much of the research investigated in this systematic review revealed the use of AIEd in traditional ways that enhance or make more efficient current practices. More research needs to focus on the unexplored affordances of AIEd. As AI becomes more advanced and sophisticated, new opportunities will arise for AIEd. Researchers need to be on the forefront of these possible innovations.
In addition, empirical exploration is needed for new tools, such as ChatGPT that was available for public use at the end of 2022. With the time it takes for a peer review journal article to be published, ChatGPT did not appear in the articles for this study. What is interesting is that it could fit with a variety of the use codes found in this study, with students getting support in writing papers and instructors using Chat GPT to assess students work and with help writing emails or descriptions for students. It would be pertinent for researchers to explore Chat GPT.
Limitations
The findings of this study show a rapid increase in the number of AIEd studies published in HE. However, to ensure a level of credibility, this study only included peer review journal articles. These articles take months to publish. Therefore, conference proceedings and gray literature such as blogs and summaries may reveal further findings not explored in this study. In addition, the articles in this study were all published in English which excluded findings from research published in other languages.
In response to the call by Hinojo-Lucena et. al. ( 2019 ), Chu et. al. ( 2022 ), and Zawacki-Richter et. al. ( 2019 ), this study provides unique findings with an up-to-date examination of the use of AIEd in HE from 2016 to 2022. Past systematic reviews examined the research up to 2020. The findings of this study show that in 2021 and 2022, publications rose nearly two to three times the number of previous years. With this rapid rise in the number of AIEd HE publications, new trends have emerged.
The findings show that of the 138 studies examined, research was conducted in six of the seven continents of the world. In extant systematic reviews showed that the US led by a large margin in the number of studies published. This trend has now shifted to China. Another shift in AIEd HE is that while extant studies lamented the lack of focus on professors of education leading these studies, this systematic review found education to be the most common department affiliation with 28% and computer science coming in second at 20%. Undergraduate students were the most studied students at 72%. Similar to the findings of other studies, language learning was the most common subject domain. This included writing, reading, and vocabulary acquisition. In examination of who the AIEd was intended for, 72% of the studies focused on students, 17% instructors, and 11% managers.
Grounded coding was used to answer the overarching question of how AIEd was used in HE. Five usage codes emerged from the data: (1) Assessment/Evaluation, (2) Predicting, (3) AI Assistant, (4) Intelligent Tutoring System (ITS), and (5) Managing Student Learning. Assessment and evaluation had a wide variety of purposes, including assessing academic progress and student emotions towards learning, individual and group evaluations, and class based online community assessments. Predicting emerged as a code with ten axial codes, as AIEd predicted dropouts and at-risk students, innovative ability, and career decisions. AI Assistants were specific to supporting students in HE. These assistants included those with an anthropomorphic presence, such as virtual agents and persuasive intervention through digital programs. ITS systems were not always noted in extant systematic reviews but were specifically mentioned in 18 of the studies in this review. ITS systems in this study provided customized strategies and approaches to student’s characteristics and needs. The final code in this study highlighted the use of AI in managing student learning, including learning analytics, curriculum sequencing, instructional design, and clustering of students.
The findings of this study provide a springboard for future academics, practitioners, computer scientists, policymakers, and funders in understanding the state of the field in AIEd HE, how AI is used. It also provides actionable items to ameliorate gaps in the current understanding. As the use AIEd will only continue to grow this study can serve as a baseline for further research studies in the use of AIEd in HE.
Availability of data and materials
The datasets used and/or analysed during the current study are available from the corresponding author on reasonable request.
Alajmi, Q., Al-Sharafi, M. A., & Abuali, A. (2020). Smart learning gateways for Omani HEIs towards educational technology: Benefits, challenges and solutions. International Journal of Information Technology and Language Studies, 4 (1), 12–17.
Google Scholar
Al-Tuwayrish, R. K. (2016). An evaluative study of machine translation in the EFL scenario of Saudi Arabia. Advances in Language and Literary Studies, 7 (1), 5–10.
Ayse, T., & Nil, G. (2022). Automated feedback and teacher feedback: Writing achievement in learning English as a foreign language at a distance. The Turkish Online Journal of Distance Education, 23 (2), 120–139. https://doi.org/10.7575/aiac.alls.v.7n.1p.5
Article Google Scholar
Baykasoğlu, A., Özbel, B. K., Dudaklı, N., Subulan, K., & Şenol, M. E. (2018). Process mining based approach to performance evaluation in computer-aided examinations. Computer Applications in Engineering Education, 26 (5), 1841–1861. https://doi.org/10.1002/cae.21971
Belur, J., Tompson, L., Thornton, A., & Simon, M. (2018). Interrater reliability in systematic review methodology: Exploring variation in coder decision-making. Sociological Methods & Research, 13 (3), 004912411887999. https://doi.org/10.1177/0049124118799372
Çağataylı, M., & Çelebi, E. (2022). Estimating academic success in higher education using big five personality traits, a machine learning approach. Arab Journal Scientific Engineering, 47 , 1289–1298. https://doi.org/10.1007/s13369-021-05873-4
Chen, L., Chen, P., & Lin, Z. (2020). Artificial intelligence in education: A review. IEEE Access, 8 , 75264–75278. https://doi.org/10.1109/ACCESS.2020.2988510
Chu, H., Tu, Y., & Yang, K. (2022). Roles and research trends of artificial intelligence in higher education: A systematic review of the top 50 most-cited articles. Australasian Journal of Educational Technology, 38 (3), 22–42. https://doi.org/10.14742/ajet.7526
Cristianini, N. (2016). Intelligence reinvented. New Scientist, 232 (3097), 37–41. https://doi.org/10.1016/S0262-4079(16)31992-3
Crompton, H., Bernacki, M. L., & Greene, J. (2020). Psychological foundations of emerging technologies for teaching and learning in higher education. Current Opinion in Psychology, 36 , 101–105. https://doi.org/10.1016/j.copsyc.2020.04.011
Crompton, H., & Burke, D. (2022). Artificial intelligence in K-12 education. SN Social Sciences, 2 , 113. https://doi.org/10.1007/s43545-022-00425-5
Crompton, H., Jones, M., & Burke, D. (2022). Affordances and challenges of artificial intelligence in K-12 education: A systematic review. Journal of Research on Technology in Education . https://doi.org/10.1080/15391523.2022.2121344
Crompton, H., & Song, D. (2021). The potential of artificial intelligence in higher education. Revista Virtual Universidad Católica Del Norte, 62 , 1–4. https://doi.org/10.35575/rvuen.n62a1
de Chiusole, D., Stefanutti, L., Anselmi, P., & Robusto, E. (2020). Stat-Knowlab. Assessment and learning of statistics with competence-based knowledge space theory. International Journal of Artificial Intelligence in Education, 30 , 668–700. https://doi.org/10.1007/s40593-020-00223-1
Dever, D. A., Azevedo, R., Cloude, E. B., & Wiedbusch, M. (2020). The impact of autonomy and types of informational text presentations in game-based environments on learning: Converging multi-channel processes data and learning outcomes. International Journal of Artificial Intelligence in Education, 30 (4), 581–615. https://doi.org/10.1007/s40593-020-00215-1
Górriz, J. M., Ramírez, J., Ortíz, A., Martínez-Murcia, F. J., Segovia, F., Suckling, J., Leming, M., Zhang, Y. D., Álvarez-Sánchez, J. R., Bologna, G., Bonomini, P., Casado, F. E., Charte, D., Charte, F., Contreras, R., Cuesta-Infante, A., Duro, R. J., Fernández-Caballero, A., Fernández-Jover, E., … Ferrández, J. M. (2020). Artificial intelligence within the interplay between natural and artificial computation: Advances in data science, trends and applications. Neurocomputing, 410 , 237–270. https://doi.org/10.1016/j.neucom.2020.05.078
Gough, D., Oliver, S., & Thomas, J. (2017). An introduction to systematic reviews (2nd ed.). Sage.
Gupta, S., & Chen, Y. (2022). Supporting inclusive learning using chatbots? A chatbot-led interview study. Journal of Information Systems Education, 33 (1), 98–108.
Hemingway, P. & Brereton, N. (2009). In Hayward Medical Group (Ed.). What is a systematic review? Retrieved from http://www.medicine.ox.ac.uk/bandolier/painres/download/whatis/syst-review.pdf
Hinojo-Lucena, F., Arnaz-Diaz, I., Caceres-Reche, M., & Romero-Rodriguez, J. (2019). A bibliometric study on its impact the scientific literature. Education Science . https://doi.org/10.3390/educsci9010051
Hrastinski, S., Olofsson, A. D., Arkenback, C., Ekström, S., Ericsson, E., Fransson, G., Jaldemark, J., Ryberg, T., Öberg, L.-M., Fuentes, A., Gustafsson, U., Humble, N., Mozelius, P., Sundgren, M., & Utterberg, M. (2019). Critical imaginaries and reflections on artificial intelligence and robots in postdigital K-12 education. Postdigital Science and Education, 1 (2), 427–445. https://doi.org/10.1007/s42438-019-00046-x
Huang, C., Wu, X., Wang, X., He, T., Jiang, F., & Yu, J. (2021). Exploring the relationships between achievement goals, community identification and online collaborative reflection. Educational Technology & Society, 24 (3), 210–223.
Hwang, G. J., & Tu, Y. F. (2021). Roles and research trends of artificial intelligence in mathematics education: A bibliometric mapping analysis and systematic review. Mathematics, 9 (6), 584. https://doi.org/10.3390/math9060584
Khalfallah, J., & Slama, J. B. H. (2018). The effect of emotional analysis on the improvement of experimental e-learning systems. Computer Applications in Engineering Education, 27 (2), 303–318. https://doi.org/10.1002/cae.22075
Kim, C., & Bennekin, K. N. (2016). The effectiveness of volition support (VoS) in promoting students’ effort regulation and performance in an online mathematics course. Instructional Science, 44 , 359–377. https://doi.org/10.1007/s11251-015-9366-5
Koć-Januchta, M. M., Schönborn, K. J., Roehrig, C., Chaudhri, V. K., Tibell, L. A. E., & Heller, C. (2022). “Connecting concepts helps put main ideas together”: Cognitive load and usability in learning biology with an AI-enriched textbook. International Journal of Educational Technology in Higher Education, 19 (11), 11. https://doi.org/10.1186/s41239-021-00317-3
Krause, S. D., & Lowe, C. (2014). Invasion of the MOOCs: The promise and perils of massive open online courses . Parlor Press.
Li, D., Tong, T. W., & Xiao, Y. (2021). Is China emerging as the global leader in AI? Harvard Business Review. https://hbr.org/2021/02/is-china-emerging-as-the-global-leader-in-ai
Liang, J. C., Hwang, G. J., Chen, M. R. A., & Darmawansah, D. (2021). Roles and research foci of artificial intelligence in language education: An integrated bibliographic analysis and systematic review approach. Interactive Learning Environments . https://doi.org/10.1080/10494820.2021.1958348
Liu, S., Hu, T., Chai, H., Su, Z., & Peng, X. (2022). Learners’ interaction patterns in asynchronous online discussions: An integration of the social and cognitive interactions. British Journal of Educational Technology, 53 (1), 23–40. https://doi.org/10.1111/bjet.13147
Long, P., & Siemens, G. (2011). Penetrating the fog: Analytics in learning and education. Educause Review, 46 (5), 31–40.
Lu, O. H. T., Huang, A. Y. Q., Tsai, D. C. L., & Yang, S. J. H. (2021). Expert-authored and machine-generated short-answer questions for assessing students learning performance. Educational Technology & Society, 24 (3), 159–173.
Mavrikis, M., Geraniou, E., Santos, S. G., & Poulovassilis, A. (2019). Intelligent analysis and data visualization for teacher assistance tools: The case of exploratory learning. British Journal of Educational Technology, 50 (6), 2920–2942. https://doi.org/10.1111/bjet.12876
Moher, D., Shamseer, L., Clarke, M., Ghersi, D., Liberati, A., Petticrew, M., Shekelle, P., & Stewart, L. (2015). Preferred reporting items for systematic review and meta-analysis protocols (PRISMA-P) 2015 statement. Systematic Reviews, 4 (1), 1–9. https://doi.org/10.1186/2046-4053-4-1
Mousavi, A., Schmidt, M., Squires, V., & Wilson, K. (2020). Assessing the effectiveness of student advice recommender agent (SARA): The case of automated personalized feedback. International Journal of Artificial Intelligence in Education, 31 (2), 603–621. https://doi.org/10.1007/s40593-020-00210-6
Mousavinasab, E., Zarifsanaiey, N., Kalhori, S. R. N., Rakhshan, M., Keikha, L., & Saeedi, M. G. (2021). Intelligent tutoring systems: A systematic review of characteristics, applications, and evaluation methods. Interactive Learning Environments, 29 (1), 142–163. https://doi.org/10.1080/10494820.2018.1558257
Ouatik, F., Ouatikb, F., Fadlic, H., Elgoraria, A., Mohadabb, M. E. L., Raoufia, M., et al. (2021). E-Learning & decision making system for automate students assessment using remote laboratory and machine learning. Journal of E-Learning and Knowledge Society, 17 (1), 90–100. https://doi.org/10.20368/1971-8829/1135285
Ouyang, F., Zheng, L., & Jiao, P. (2022). Artificial intelligence in online higher education: A systematic review of empirical research from 2011–2020. Education and Information Technologies, 27 , 7893–7925. https://doi.org/10.1007/s10639-022-10925-9
Page, M. J., McKenzie, J. E., Bossuyt, P. M., Boutron, I., Hoffmann, T., Mulrow, C., Shamseer, L., Tetzlaff, J. M., Akl, E. A., Brennan, S. E., Chou, R., Glanville, J., Grimshaw, J. M., Hróbjartsson, A., Lalu, M. M., Li, T., Loder, E. W., Mayo-Wilson, E., McDonald, S., … Moher, D. (2021). The PRISMA 2020 statement: An updated guideline for reporting systematic reviews. British Medical Journal . https://doi.org/10.1136/bmj.n71
Popenici, S. A. D., & Kerr, S. (2017). Exploring the impact of artificial intelligence on teaching and learning in higher education. Research and Practice in Technology Enhanced Learning, 12 (22), 1–13. https://doi.org/10.1186/s41039-017-0062-8
PRISMA Statement. (2021). PRISMA endorsers. PRISMA statement website. http://www.prisma-statement.org/Endorsement/PRISMAEndorsers
Qian, Y., Li, C.-X., Zou, X.-G., Feng, X.-B., Xiao, M.-H., & Ding, Y.-Q. (2022). Research on predicting learning achievement in a flipped classroom based on MOOCs by big data analysis. Computer Applied Applications in Engineering Education, 30 , 222–234. https://doi.org/10.1002/cae.22452
Rutner, S. M., & Scott, R. A. (2022). Use of artificial intelligence to grade student discussion boards: An exploratory study. Information Systems Education Journal, 20 (4), 4–18.
Salas-Pilco, S., & Yang, Y. (2022). Artificial Intelligence application in Latin America higher education: A systematic review. International Journal of Educational Technology in Higher Education, 19 (21), 1–20. https://doi.org/10.1186/S41239-022-00326-w
Saldana, J. (2015). The coding manual for qualitative researchers (3rd ed.). Sage.
Shukla, A. K., Janmaijaya, M., Abraham, A., & Muhuri, P. K. (2019). Engineering applications of artificial intelligence: A bibliometric analysis of 30 years (1988–2018). Engineering Applications of Artificial Intelligence, 85 , 517–532. https://doi.org/10.1016/j.engappai.2019.06.010
Strauss, A., & Corbin, J. (1995). Grounded theory methodology: An overview. In N. K. Denzin & Y. S. Lincoln (Eds.), Handbook of qualitative research (pp. 273–285). Sage.
Turing, A. M. (1937). On computable numbers, with an application to the Entscheidungs problem. Proceedings of the London Mathematical Society, 2 (1), 230–265.
Turing, A. M. (1950). Computing machinery and intelligence. Mind, 59 , 443–460.
MathSciNet Google Scholar
Ullah, H., & Hafiz, M. A. (2022). Exploring effective classroom management strategies in secondary schools of Punjab. Journal of the Research Society of Pakistan, 59 (1), 76.
Verdú, E., Regueras, L. M., Gal, E., et al. (2017). Integration of an intelligent tutoring system in a course of computer network design. Educational Technology Research and Development, 65 , 653–677. https://doi.org/10.1007/s11423-016-9503-0
Vygotsky, L. S. (1978). Mind and society: The development of higher psychological processes . Harvard University Press.
Winkler-Schwartz, A., Bissonnette, V., Mirchi, N., Ponnudurai, N., Yilmaz, R., Ledwos, N., Siyar, S., Azarnoush, H., Karlik, B., & Del Maestro, R. F. (2019). Artificial intelligence in medical education: Best practices using machine learning to assess surgical expertise in virtual reality simulation. Journal of Surgical Education, 76 (6), 1681–1690. https://doi.org/10.1016/j.jsurg.2019.05.015
Yang, A. C. M., Chen, I. Y. L., Flanagan, B., & Ogata, H. (2021). Automatic generation of cloze items for repeated testing to improve reading comprehension. Educational Technology & Society, 24 (3), 147–158.
Yao, X. (2022). Design and research of artificial intelligence in multimedia intelligent question answering system and self-test system. Advances in Multimedia . https://doi.org/10.1155/2022/2156111
Zawacki-Richter, O., Marín, V. I., Bond, M., & Gouverneur, F. (2019). Systematic review of research on artificial intelligence applications in higher education—Where are the educators? International Journal of Educational Technology in Higher Education, 16 (1), 1–27. https://doi.org/10.1186/s41239-019-0171-0
Zhang, F. (2022). Design and application of artificial intelligence technology-driven education and teaching system in universities. Computational and Mathematical Methods in Medicine . https://doi.org/10.1155/2022/8503239
Zhang, Z., & Xu, L. (2022). Student engagement with automated feedback on academic writing: A study on Uyghur ethnic minority students in China. Journal of Multilingual and Multicultural Development . https://doi.org/10.1080/01434632.2022.2102175
Download references
Acknowledgements
The authors would like to thank Mildred Jones, Katherina Nako, Yaser Sendi, and Ricardo Randall for data gathering and organization.
Author information
Authors and affiliations.
Department of Teaching and Learning, Old Dominion University, Norfolk, USA
Helen Crompton
ODUGlobal, Norfolk, USA
Diane Burke
RIDIL, ODUGlobal, Norfolk, USA
You can also search for this author in PubMed Google Scholar
Contributions
HC: Conceptualization; Data curation; Project administration; Formal analysis; Methodology; Project administration; original draft; and review & editing. DB: Conceptualization; Data curation; Project administration; Formal analysis; Methodology; Project administration; original draft; and review & editing. Both authors read and approved this manuscript.
Corresponding author
Correspondence to Helen Crompton .
Ethics declarations
Competing interests.
The authors declare that they have no competing interests.
Additional information
Publisher's note.
Springer Nature remains neutral with regard to jurisdictional claims in published maps and institutional affiliations.
Rights and permissions
Open Access This article is licensed under a Creative Commons Attribution 4.0 International License, which permits use, sharing, adaptation, distribution and reproduction in any medium or format, as long as you give appropriate credit to the original author(s) and the source, provide a link to the Creative Commons licence, and indicate if changes were made. The images or other third party material in this article are included in the article's Creative Commons licence, unless indicated otherwise in a credit line to the material. If material is not included in the article's Creative Commons licence and your intended use is not permitted by statutory regulation or exceeds the permitted use, you will need to obtain permission directly from the copyright holder. To view a copy of this licence, visit http://creativecommons.org/licenses/by/4.0/ .
Reprints and permissions
About this article
Cite this article.
Crompton, H., Burke, D. Artificial intelligence in higher education: the state of the field. Int J Educ Technol High Educ 20 , 22 (2023). https://doi.org/10.1186/s41239-023-00392-8
Download citation
Received : 30 January 2023
Accepted : 23 March 2023
Published : 24 April 2023
DOI : https://doi.org/10.1186/s41239-023-00392-8
Share this article
Anyone you share the following link with will be able to read this content:
Sorry, a shareable link is not currently available for this article.
Provided by the Springer Nature SharedIt content-sharing initiative
- Artificial Intelligence
- Systematic review
- Higher education
Empirical Research in Vocational Education and Training
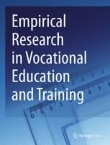
Call for Papers: Dropouts in vocational and professional education
A decreasing attractiveness of vocational education, particularly in payment and quality, causes higher dropout rates while hitting ongoing demographic changes resulting in extensive skill shortages for many regions. Therefore, tackling the internationally high dropout rates is of utmost political and scientific interest. This thematic issue contributes to the conceptualization, analysis, and prevention of vocational and professional dropouts by bringing together current research that progresses to a deeper processual understanding and empirical modelling of dropouts.
Call for Papers: Authentic Technology-based Learning and Testing Environments in Vocational Education and Training
In the course of an increasing digitalization of the working world, everyday work activities are becoming more and more technology-based. Therefore, technology-based learning and assessment is of particular importance in vocational education and training (VET) as it provides authentic tasks and tools and learning opportunities in controlled environments.
Reducing ethnic minority youth’s disadvantage in accessing vocational training
Call for Papers: Promoting financial literacy in Vocational Education and Training
Financial literacy is as an increasingly important skill. It varies within and across age and population groups, but overall deficits must be assumed. In order to remedy this problem and to promote financial literacy effectively, research is needed that focuses on the development and evaluation of support measures be it in the context of initial vocational education or continuous vocational education and training.
- Most accessed
- Collections
Different dropout directions in vocational education and training: the role of the initiating party and trainees’ reasons for dropping out
Authors: Christian Michaelis and Stefanie Findeisen
Compromises in occupational choice and premature termination of vocational education and training: gender type, prestige, and occupational interests in focus
Authors: Melanie Fischer-Browne, Lea Ahrens, Corinna Kleinert and Brigitte Schels
The discourse on technological literacy: exploring visual representations enabled by the visual cultures of four Swedish vocational education and training programmes
Authors: Andreas Larsson, Emelie Fälton and Karin Stolpe
A network model of stress contagion: evidence from the vocational classroom
Authors: Tobias Kärner, Livia Shkoza and Winfried Pohlmeier
Examining career and technical education (CTE) pathways in historically Black colleges and universities (HBCUs)
Authors: Kevin M. Williams, Vinetha Belur and Zhitong Yang
Most recent articles RSS
View all articles
Technical and vocational education and training (TVET) for young people in low- and middle-income countries: a systematic review and meta-analysis
Authors: Janice S Tripney and Jorge G Hombrados
A study of carpenter apprentices’ spatial skills
Authors: Sebastien Cuendet, Jessica Dehler-Zufferey, Christoph Arn, Engin Bumbacher and Pierre Dillenbourg
Vocational training in India: determinants of participation and effect on wages
Authors: Rishi Kumar, Shravanth Mandava and Venkata Sandeep Gopanapalli
Measurement of vocational competences: an analysis of the structure and reliability of current assessment practices in economic domains
Authors: Esther Winther and Viola Katharina Klotz
Guiding workplace learning in vocational education and training: a literature review
Authors: Susanna Mikkonen, Laura Pylväs, Heta Rintala, Petri Nokelainen and Liisa Postareff
Most accessed articles RSS
Dropouts in vocational and professional education Empirical Research in Vocational Education and Training Edited By: Viola Deutscher, Stefanie Findeisen, Christian Michaelis
Exploring resilience in vocational and professional contexts Empirical Research in Vocational Education and Training Edited by: Carmela Aprea, Susan Beltman, Viviana Sappa,
Authentic Technology-based Learning and Testing Environments in Vocational Education and Training Empirical Research in Vocational Education and Training Edited by: Eveline Wuttke, Susan Seeber, Jürgen Seifried, Andreas Rausch
The Economics of Vocational Education and Training Empirical Research in Vocational Education and Training Edited by : Prof. Dr. Samuel Mühlemann and Prof. Dr. Harald Pfeifer, Maastricht University, Netherlands and BIBB, Germany
Test motivation and test conditions in domain-specific assessments in VET Empirical Research in Vocational Education and Training Edited by : Jun. Prof. Dr. Kristina Kögler, Ass.-Prof. Dr. Christoph Helm, Dr. Alexander Robitzsch
Assessing Financial Literacy as a Basis for Designing and Evaluating Interventions in Vocational and Adult Education and Training Empirical Research in Vocational Education and Training Edited by : Prof. Dr. Carmela Aprea, Prof. Dr. Eveline Wuttke, Prof. Dr. Bärbel Fürstenau
Transitions - Research with longitudinal data Empirical Research in Vocational Education and Training Transitions of adolescents and young adults within the educational system and the labor market. Edited by Prof. Dr. Stephan Schumann, Prof. Dr. Susan Seeber, Prof. Dr. Kurt Häfeli Collection published: 1 June 2016
Technology-based assessment of domain-specific work-related competences Empirical Research in Vocational Education and Training A broader understanding of competence that goes beyond knowledge reproduction in traditional testing and measurement of outcomes of educational efforts. Edited by Prof. Dr. Eveline Wuttke, Prof. Dr. Jürgen Seifried, Dr. Andreas Rausch
Thematic series Assessment of Domain-specific Professional Competencies Empirical Research in Vocational Education and Training Edited by Prof Olga Zlatkin-Troitschanskaia, Prof Richard Shavelson Collection published: 12 February 2015
Check out our new infographic
To learn the top reasons why you should publish your next open access research article with us.
- Discover thematic series
Call for Papers: Exploring resilience in vocational and professional contexts
ERVET welcomes papers that brings together current research that progresses the emerging field of resilience in vocational and professional contexts, in order to expand our understanding of how resilience can be conceptualised and measured in these contexts, to provide evidence in terms of effective interventions to build and sustain resilience, especially in times of crisis. Read more and submit your paper.
ERVET is indexed by ESCI and SCOPUS
Empirical Research in Vocational Training and Education has been accepted by the Emerging Sources Citation Indext (ESCI) and is indexed by SCOPUS with an annual CiteScore of 3.4.
Aims and scope
The main focus of this journal is to provide a platform for original empirical investigations in the field of professional, vocational and technical education , comparing the effectiveness, efficiency and equity of different vocational education systems at the school, company and systemic level. The journal fills a gap in the existing literature focusing on empirically-oriented academic research and stimulating the interest in strengthening the vocational part of the educational system , both at the basic and higher education level.
Contributions published in this journal cast new light on a broad range of topics related to teaching and learning in vocational education, such as pedagogy practices, apprenticeship, competence development, validation of test instruments and assessment, professional challenges with certain labour-market conditions, and classroom experience. Offering evidence and reports from basic education and training to continuous or adult learning, Empirical Research in Vocational Education and Training will appeal not only to academics and researchers in the field of vocational education, but also to policy-makers, policy planners and administrators, school-leaders and trainers.
Latest Thematic Series: The Economics of Vocational Education and Training
The labour markets of many industrialised countries are facing extensive technological and demographic changes. Digitalisation, networking, and robotics all transform the world of work and with it the demand for skills and qualifications. Vocational education and training (VET) is often valued for its ability to help young people transition smoothly from education to employment. Despite an increasing amount of research in this area, many questions in the field of the Economics of VET remain unanswered.
SpringerNature supports ORCID in this journal
SpringerNature supports the ORCID (Open Researcher and Contributor ID) identifier, which is an ID that uniquely attaches your identity to your research work. The result: no more confusion because another researcher has the same or a similar name. You get the credit for your work.
Book series
- Editorial Board
- Sign up for article alerts and news from this journal
Annual Journal Metrics
Citation Impact 2023 Journal Impact Factor: 1.6 5-year Journal Impact Factor: 1.4 Source Normalized Impact per Paper (SNIP): 1.352 SCImago Journal Rank (SJR): 0.503 Speed 2023 Submission to first editorial decision (median days): 20 Submission to acceptance (median days): 213 Usage 2023 Downloads: 279,678 Altmetric mentions: 20
- More about our metrics
This journal is indexed by
- Emerging Sources Citation Index (ESCI)
- Google Scholar
- EBSCO Discovery Service
- EBSCO Education Source
- OCLC WorldCat Discovery Service
- ProQuest Central
- ProQuest Education Database
- ProQuest Social Science Collection
- ProQuest-ExLibris Summon
- UGC-CARE List (India)
- TD Net Discovery Service
- ProQuest-ExLibris Primo
- Norwegian Register for Scientific Journals and Series
Society affiliation
- Arnold B. Bakker ORCID: orcid.org/0000-0003-1489-1847 1 , 2 &
- Karina Mostert 2
781 Accesses
Explore all metrics
This article reviews the literature on student well-being (burnout and engagement) and their relationships with study demands and resources, student behaviors (proactive and self-undermining study behaviors), and student outcomes in higher education. Building on research that used Job Demands–Resources and Study Demands–Resources models to investigate student well-being, we develop the Study Demands–Resources (SD–R) theory to delineate the various processes, mechanisms, and behaviors involved in student burnout and engagement. Study demands and resources have unique and combined effects on higher education students’ well-being. In addition, students can influence their own well-being and study-related outcomes by either proactively optimizing their study demands and resources or displaying self-undermining behaviors that can adversely affect their study environment. We discuss several avenues for future research, including (a) rigorous tests of SD–R propositions; (b) trait versus state effects in SD–R theory; (c) the impact of the higher education climate and lecturer influence; and (d) an expanded SD–R theory.
Similar content being viewed by others
Study Demands-Resources Model of Student Engagement and Burnout
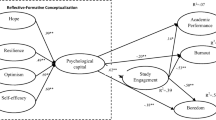
How psychological capital is related to academic performance, burnout, and boredom? The mediating role of study engagement
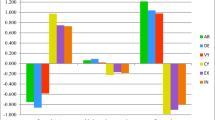
Identifying higher education students’ profiles of academic engagement and burnout and analysing their predictors and outcomes
Explore related subjects.
- Artificial Intelligence
Avoid common mistakes on your manuscript.
Higher education students are confronted with a range of demands, such as coursework deadlines, group assignments, financial problems, and exams. Such demands necessitate time management, coordination, and focused attention. Over time, study demands require considerable cognitive, emotional, and physical effort, which may drain psychological resources and lead to study anxiety, fatigue, and even burnout (Gusy et al., 2016 ; Madigan & Curran, 2021 ; Salmela-Aro & Upadyaya, 2014 ). However, students may also encounter various resources while studying, including support and constructive feedback from lecturers, social support from family and friends, and development opportunities (Bakker et al., 2015 ). Such resources help students manage their demands, facilitate student engagement (Gusy et al., 2016 ), and guide goal-oriented behaviors. Resources are inherently motivating because they satisfy basic psychological needs, such as the needs for autonomy, relatedness, and competence (Vansteenkiste et al., 2009 ).
To better understand the factors influencing student well-being, educational psychologists have adopted the Job Demands–Resources (JD–R) model (Demerouti et al., 2001 ) that originated in an organizational context. JD–R theory is a comprehensive, well-established, and widely utilized theory to measure and explain well-being in organizational contexts empirically (Bakker, Demerouti, & Sanz-Vergel, 2023 ; Bakker, Hetland, et al., 2023 ; Bakker, Xanthopoulou, & Demerouti, 2023 ), offers insights from both positive and negative well-being perspectives, and integrates various job stress and motivational perspectives (Bakker, Demerouti, & Sanz-Vergel, 2023 ; Demerouti et al., 2001 ). The JD–R model categorizes characteristics of the work environment into demands (facets of work that cost effort and instigate a health impairment process) and resources (facets of work that help cope with demands, give meaning, and fuel a motivational process) (Bakker & Demerouti, 2007 ; Schaufeli & Bakker, 2004 ).
Over the past 15 years, numerous studies have provided evidence that the university environment can similarly be organized into study demands and resources, which evoke parallel health impairment and motivational processes among students (e.g., Calderwood & Gabriel, 2017 ; Cho et al., 2023 ; Clements & Kamau, 2018 ; Salanova et al., 2010 ; Wilson & Sheetz, 2010 ; Wolff et al., 2014 ). Thus, building on the JD–R framework, several scholars have proposed and tested Study Demands–Resources (SD–R) models tailored to the higher education context (e.g., Gusy et al., 2016 ; Jagodics & Szabó, 2023 ; Lesener et al., 2020 ; Martin & Collie, 2022 ; Mokgele & Rothmann, 2014 ; Salmela-Aro et al., 2022 ).
In this position paper, we rely on recent formulations of JD–R theory (Bakker et al., 2014 ; Bakker & Demerouti, 2024 ; Bakker, Demerouti, & Sanz-Vergel, 2023 ; Demerouti & Bakker, 2023 ) to systematically delineate the various processes, mechanisms, and study behaviors (proactive behaviors and self-undermining) associated with student burnout and engagement. We build on and strengthen existing SD–R models and review literature on higher education students’ demands and resources. In addition, we discuss how study demands and resources impact student well-being and achievement and the implications for optimizing the university experience.
Although the experiences of students in the higher education environment are not exactly the same as those of employees, there are several similarities between studying and working. Like employees, students need to engage in organized, structured, and compulsory activities, like attending classes, working on group assignments, and studying for exams. In addition, like work activities, study activities are goal-oriented and evaluated, and have important implications for one’s career (Salanova et al., 2010 ). We align empirical evidence for the Study Demands–Resources (SD–R) model with new developments in JD–R theory that build on and strengthen existing research (Gusy et al., 2016 ; Jagodics & Szabó, 2023 ; Lesener et al., 2020 ; Salmela-Aro et al., 2022 ) and focus on the higher education context, although SD–R theory may also be relevant for other educational contexts (e.g., primary school and high school) (Salmela-Aro & Upadyaya, 2014 ).
We aim to make the following contributions to the educational psychology literature. We systematically integrate JD–R principles and propositions based on the existing student well-being literature and build on and strengthen current SD–R models to inform a sound, holistic SD–R theory for the higher education context. First, we integrate the various causes and consequences of two types of student well-being: burnout and engagement. Second, we explain the roles of study demands and resources. Why, how, and when do study demands result in strain and burnout? What is the function of study resources such as autonomy, recognition, and social support? How do study demands and resources have a combined impact on student well-being? We integrate the buffer and boost hypotheses in SD–R theory. Third, we look at the role of personal resources, such as self-efficacy, optimism, and resiliency. How do such beliefs and cognitions influence student burnout and engagement? We explain how personal resources may result in new study resources, and how personal resources statistically interact with study demands and resources. Fourth, we discuss proactive self-enhancing study behaviors such as study crafting and playful study design, as well as reactive self-undermining behaviors. We describe how these behaviors may result in gain and loss spirals of study events and experiences, respectively. Fifth, we discuss the underlying psychological processes linking study demands and resources and student burnout and engagement to individual and higher education outcomes. Specifically, we show how student burnout and engagement mediate the relationship between antecedents and outcomes. Finally, we make several recommendations for future research and practice.
Study Demands–Resources Theory
An important building block of SD–R theory is that the features of the study environment can be categorized as either a demand or a resource. Following this logic and the findings of previous JD–R and SD–R models, SD–R theory proposes that higher education students’ experiences can be categorized as being demanding or resourceful. Study demands require effort and may, therefore, consume considerable physical, emotional, and cognitive energy and capacity. These demands encompass challenges that facilitate learning (e.g., intricate assignments) as well as hindrances that thwart progress (e.g., ambiguous tasks that create uncertainty) (Salmela-Aro et al., 2022 ). In contrast, study resources play a functional role in helping students achieve their academic goals and are instrumental in helping the student studying, regulating study demands, and motivating students to grow, learn, and progress while studying. These resources frequently comprise multilayered factors that assist students’ learning and engagement (Salmela-Aro et al., 2022 ). In our review, we will examine two distinct processes outlined in JD–R and SD–R models (Demerouti et al., 2001 ; Lesener et al., 2020 ; Salmela-Aro et al., 2022 ; Schaufeli & Bakker, 2004 ): the health impairment process and the motivational process. In the organizational context, these processes have demonstrated notable and disparate negative and positive impacts on well-being. The health impairment process is associated with adverse health outcomes, while the motivational process is linked to positive outcomes (Bakker, Demerouti, & Sanz-Vergel, 2023 ).
A second building block of SD–R theory is student well-being with the opposing states of student burnout and engagement. Here, as with JD–R theory, SD–R theory outlines that students may either feel exhausted and be cynical about their studies or rather the opposite, i.e., feel vigorous and enthusiastic. The third building block concerns student behaviors. SD–R theory proposes that certain study environments trigger reactive and maladaptive study behaviors that can undermine effective studying, whereas other study environments trigger proactive and adaptive study behaviors that facilitate effective studying. The proposed SD–R theory also includes feedback loops and is graphically depicted in Fig. 1 . In what follows, we discuss each of these building blocks in more detail while reviewing the available evidence in the educational literature. We start with discussing student well-being.
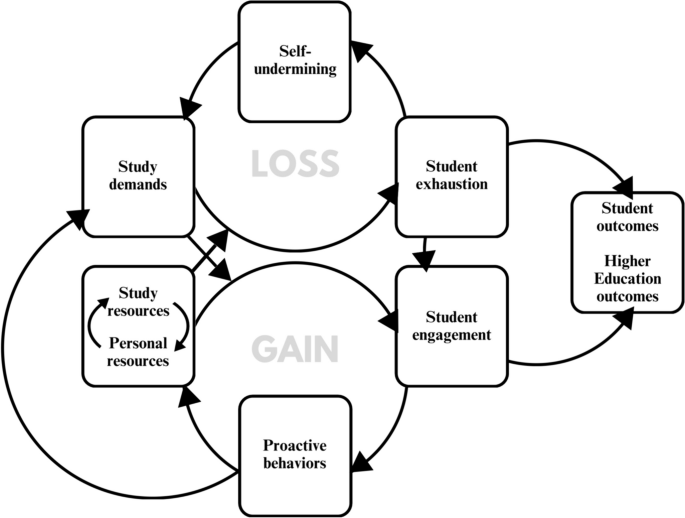
The Study Demands–Resources model
Student Well-Being
During the past two decades, student well-being has received considerable attention (for meta-analyses, see Bücker et al., 2018 ; Kaya & Erdem, 2021 ). However, since scholars have used a wide range of student well-being definitions and indicators, it is challenging to get a good overview of the potential predictors and outcomes of student well-being. In the present paper, we focus on two specific types of student well-being: student burnout and student engagement.
Student Burnout
Student burnout refers to feeling exhausted because of study demands, expressing a cynical, detached attitude toward one’s studies, and feeling incompetent as a student (Schaufeli et al., 2002 ). Burned-out students experience chronic mental, emotional, or physical exhaustion due to the many demands they face while studying. They often feel disconnected or cynical about their classes and suffer from reduced academic efficacy, may skip classes, or may not complete assignments. Student burnout has been linked to several unfavorable outcomes, including depressive symptoms (Cheng et al., 2020 ), increased use of substances such as alcohol and cannabis (Allen et al., 2022 ), suicidal ideation (Dyrbye et al., 2008 ), class absenteeism (Seibert et al., 2017 ), and dropping out (Bumbacco & Scharfe, 2023 ). Consequently, burnout is a predictor of impaired academic achievement (Madigan & Curran, 2021 ).
Student Engagement
Student engagement is defined as a positive, fulfilling, study-related psychological state characterized by vigor, dedication, and absorption (Salmela-Aro & Read, 2017 ; Schaufeli et al., 2002 ). Engaged students display mental resilience while studying and perseverance in the face of challenges and difficulties (Finn & Zimmer, 2012 ; Hu, 2010 ). In addition, they exhibit a strong commitment to their studies, experiencing a sense of excitement, enthusiasm, and focus (Pekrun & Linnenbrink-Garcia, 2012 ; Schaufeli et al., 2002 ). Engaged students show active learning behaviors and receive better grades (Bakker et al., 2015 ; Schaufeli et al., 2002 ), and low levels of academic withdrawal (Tuominen-Soini & Salmela-Aro, 2014 ). In addition, student engagement predicts various long-term positive outcomes, such as persistence in educational pathways (Öz & Boyacı, 2021 ) and better job possibilities (Ma & Bennett, 2021 ). Engaged students are also more likely to start an entrepreneurial career (e.g., Liu, Gorgievski, et al., 2023 ). Moreover, both student burnout and engagement are the consequence of a unique combination of study demands and resources, which are discussed next.
Study Demands and Resources
Higher education institutions serve as a transformative space where students develop invaluable skills and can gain life-changing opportunities. Engaging in tertiary education means gaining new experiences, meeting new people, and learning a passion for your subject. On days with lectures and tutorial meetings, there are opportunities to learn new things and have interesting conversations with professors and fellow students. The days students prepare for an exam may demand focused reading, intensive information processing, and dealing with interruptions. Moreover, in some countries, students may encounter various other demands, including transportation problems, limited access to technology, poor housing, unsafe living conditions, financial struggles, and difficulty adjusting to the higher education environment (Haverila et al., 2020 ; Martin et al., 2023 ; Martin & Collie, 2022 ).
Studying is also a social activity. Students may be asked to collaborate with their peers when writing papers, preparing presentations, or creating podcasts — which provides an opportunity for an enjoyable and fulfilling experience. However, collaborating may also mean dealing with interpersonal conflicts, for example, when finding out that a group member engages in social loafing and exerts little effort to contribute to the group task. As a final example, students may enhance their academic experience by participating in extracurricular activities, joining study associations, planning study visits to organizations, or inviting experts to give interesting talks.
Research indicates that students from higher social classes typically have access to more resources such as academic materials, financial support, family assistance, and developmental opportunities than their peers from lower social classes (Munir et al., 2023 ; Van Zyl, 2016 ). These resources may enable them to better navigate and manage their demands and reduce study stress, facilitating engagement and study success. In contrast, students from lower social classes often face a larger range of demands, including academic unpreparedness for higher education, difficulties in commuting to campus, challenges in adapting to new social circles (resulting in lower levels of peer support), and being enrolled in courses that were not their preferred choice (Van Zyl, 2016 ). These demands may intensify the perceived academic workload and stress levels, making it more difficult to succeed.
The activities and events students encounter in their study life seem countless and manifold. Following JD–R theory and previous SD–R models (e.g., Lesener et al., 2020 ; Salmela-Aro et al., 2022 ; Salmela-Aro & Upadyaya, 2014 ), SD–R theory proposes that the characteristics of study life can be distinguished into two categories, namely study demands and resources. We define study demands as all the facets of studying that cost effort and, therefore, expend physical, emotional, and mental energy (cf. Bakker, Demerouti, & Sanz-Vergel, 2023 ). Study demands may manifest in diverse forms, such as a high pace and volume of study work and cognitive challenges (Cilliers et al., 2018 ). Students may also face time constraints (Lesener et al., 2020 ), conflicting deadlines (Martin et al., 2023 ), and learning obstacles (Martin et al., 2021 ).
In contrast, study resources are defined as all the aspects of studying that have motivating potential, can buffer the impact of study demands, and facilitate growth and learning (cf. Demerouti et al., 2001 ; Demerouti & Bakker, 2023 ). Specifically, resources tailored to studying can manifest as study resources (e.g., having competent lecturers, access to books and study materials, study facilitators, and mentors) and university resources (e.g., classrooms, library and computer facilities, good infrastructure, and an atmosphere creating a sense of belonging). Resources specific to studying may include learning support (Martin et al., 2021 ), autonomy and sense of control (Collie et al., 2015 ), family and friend support (Cilliers et al., 2018 ), developmental and growth opportunities (Cilliers et al., 2018 ; Lesener et al., 2020 ), lecturer support (Cilliers et al., 2018 ; Kulikowski et al., 2019 ), and role clarity (Lesener et al., 2020 ) among others.
Proposition 1: Study characteristics can be modeled using two distinctive categories, namely study demands and study resources.
Another central proposition in SD–R theory is that study demands and resources have unique effects on student burnout and engagement. Research on such effects within the work context has provided consistent evidence for two processes: (a) a health impairment process triggered by excessive job demands and (b) a motivational process triggered by job resources (Lesener et al., 2019 ). The health impairment process refers to the impact of demands on physical health complaints through fatigue, anxiety, and other strains. In contrast, the motivational process refers to the impact of resources on creativity and task performance through the experience of engagement (vigor, dedication, absorption) (Bakker, Demerouti, & Sanz-Vergel, 2023 ; Schaufeli & Bakker, 2004 ).
Research among students has also provided evidence for these dual processes. For instance, study demands have been shown to deplete students’ energy levels (Cilliers et al., 2018 ; Jagodics & Szabó, 2023 ) and negatively affect their physical and psychological well-being (Gusy et al., 2016 ; Mokgele & Rothmann, 2014 ). Kaggwa et al. ( 2021 ) recently highlighted that the demands prevalent in the higher education context can potentially lead to burnout symptoms, ultimately resulting in negative student outcomes such as procrastination, decreased life and study satisfaction, and intention to drop out (Turhan et al., 2022 ). Research has also demonstrated a clear link between escalated levels of student burnout and mental health disorders (e.g., depression; Jackson et al., 2016 ) as well as reduced academic performance (Madigan & Curran, 2021 ). Thus, consistent with the health impairment process proposed by JD–R theory (Bakker, Demerouti, & Sanz-Vergel, 2023 ), study demands are de-energizing to students, and lead to health problems and unfavorable study outcomes.
While study demands are positively associated with strain and student burnout, study resources are more clearly positively associated with positive aspects of student well-being, including student engagement (Gusy et al., 2016 ; Robins et al., 2015 ). Indeed, several studies underscore the importance of study resources in shaping student motivation and performance. Resources like support from lecturers and peers have been demonstrated to enhance aspects of student well-being such as life satisfaction and engagement (Mokgele & Rothmann, 2014 ). Bellini et al. ( 2022 ) further suggest that a resourceful study environment facilitates students’ learning goals. When students perceived an abundance of study resources, their engagement and motivation to study were significantly heightened (Liu, Gorgievski, et al., 2023 ).
Bakker et al. ( 2015 ) followed students for three consecutive weeks in which they attended six tutorial group meetings. They found that in the weeks that students had access to more study resources (autonomy, social support, opportunities to learn about new topics, and positive feedback), they were more energized and enthusiastic about their studies (i.e., more engaged). During these weeks, students exhibited increased engagement in tutorial meetings, actively participating in problem-solving brainstorms and posing critical questions. In contrast to study demands, study resources, therefore, have the potential to trigger the motivational process in students (Bakker, Demerouti, & Sanz-Vergel, 2023 ). Lesener et al. ( 2020 ) found support for the health impairment and motivational processes in a sample of 5660 university students. Their findings showed that study demands were positively associated with student burnout and that student burnout mediated the link between study demands and students’ life satisfaction. They also found support for the motivational process, where study resources were positively related to life satisfaction through student engagement.
Proposition 2: Study demands and resources instigate two different processes, a health impairment process, and a motivational process.
The third proposition in SD–R theory is that study demands and resources have a combined impact on student well-being and outcomes. According to Bakker, Demerouti, and Sanz-Vergel ( 2023 ), there are two ways in which demands and resources interact and have an impact on well-being. The buffer hypothesis states that study resources such as social support and constructive feedback alleviate or buffer the positive influence of study demands on strain. Thus, buffer effects refer to the protective role of resources in alleviating the adverse consequences of high study demands. For example, when students face demanding coursework and interpersonal conflicts, certain study resources, such as time control and support from fellow students, can act as buffers to diminish the negative impact on their well-being. Aloia and McTigue ( 2019 ) found evidence for a buffer effect in their study among college students in the USA. Specifically, they found that the impact of study demands (e.g., workload, and pressures to perform) on psychological well-being was weakened when students had access to an abundance of study resources (supportive informational and emotional communication). In addition, research by Naylor ( 2022 ) suggested that a study environment rich in resources (e.g., teacher autonomy support and interesting coursework) can compensate for study demands such as study load and financial stress. They also showed that students who had access to more study resources reported lower levels of burnout, anxiety, and depression in the face of high study demands.
The boost hypothesis states that challenging study demands can amplify or boost the positive impact of study resources on engagement and performance (cf. Bakker et al., 2014 ). Particularly when students are confronted with complex study tasks and deadlines, they will benefit most from lecturer support and constructive feedback. Hospel and Galand ( 2016 ) found evidence for a boost effect by showing that students were more emotionally engaged (e.g., curious, interested, enthusiastic) in the lessons when teachers combined high study demands (i.e., high expectations, strong guidance) with study resources in the form of autonomy and support. When students had numerous opportunities to take initiative and when their perspectives and feelings were well acknowledged, study demands positively influenced positive emotional engagement and negatively influenced negative emotional engagement. However, the demands × resources interaction term showed only marginal, mainly nonsignificant, effects on cognitive and behavioral engagement. We refer to Salmela-Aro et al. ( 2022 ) for a further review of synergistic relationships among study demands and resources in the SD–R model.
Proposition 3: Study demands and resources have a multiplicative, combined impact on student well-being.
The Role of Personal Resources
Personal resources refer to individuals’ beliefs in their ability to control and impact their environment successfully (Hobfoll et al., 2018 ; Xanthopoulou et al., 2009 ). Examples are self-efficacy, optimism, hope, and resiliency (also referred to as psychological capital; Luthans et al., 2013 ). In the organizational context, several studies have demonstrated the importance of personal resources for employee outcomes (e.g., Bakker & Van Wingerden, 2021 ; Knight et al., 2017 ). Moreover, research suggests that individuals who have more personal resources also have access to more environmental resources (e.g., Xanthopoulou et al., 2009 ). These findings suggest that when individuals believe they can influence their environment successfully, they are more likely to gain more environmental resources (e.g., autonomy, social support, feedback), which helps them feel engaged and perform well (Bakker, Demerouti, & Sanz-Vergel, 2023 ).
Over the past decade, research suggests that personal resources are also important for student well-being and learning outcomes. Spanish and Portuguese students who reported many personal resources (optimism, self-efficacy, resilience, hope) were more engaged in their studies and demonstrated a higher grade point average than students with few personal resources (Martínez et al., 2019 ). Similarly, Vietnamese business students with higher personal resources reported greater happiness and higher quality of university life (Tho, 2023 ). Personal resources such as hope and self-efficacy were most important for students who had only limited access to social and organizational resources and vice versa (Junça Silva et al., 2022 ). This suggests that personal resources can compensate for a lack of study resources. Finally, a scoping review of Theron ( 2022 ) showed that personal resources (e.g., self-confidence, self-efficacy) and personal skills (e.g., talent for learning, time management skills) help students navigate challenges, achieving goals, and enhancing their well-being.
Is there any evidence that students with more personal resources also gain more study resources over time? Even though Bakker et al. ( 2015 ) did not test the causal relationship between personal and study resources, they did find that on the days students had access to more personal resources, they reported more study resources, and vice versa. Robayo-Tamayo et al. ( 2020 ) investigated the influence of early-day personal resources on end-of-day student engagement through study resources. They used a 5-day quantitative diary study and showed that on the days students felt more self-efficacious and curious, they mobilized more social support from their professors and peers, increasing their engagement.
Lee et al. ( 2022 ) argued that social support from peers and teachers (study resources) and self-compassion (i.e., being mindful and kind to oneself — a personal resource) would be reciprocally related. Although the design of their study could not test (reversed) causal effects, they did find a positive link between social support and self-compassion. In addition, both resources were positively related to academic engagement and negatively related to academic burnout. Finally, it can be argued that students who believe in themselves and think they have control over their study environment are more likely to proactively ask for resources from others. Indeed, Tho ( 2023 ) showed that students with more personal resources (hope, optimism, self-efficacy, and resilience) were more likely to engage in study crafting, i.e., they took the personal initiative to increase their social and structural study resources.
Proposition 4: Personal resources such as optimism, self-efficacy, hope, and resilience have a reciprocal relationship with study resources.
SD–R theory further proposes that, like study resources, personal resources can moderate the negative impact of study demands on student well-being. In the organizational context, several studies have shown that personal resources alleviate the impact of job demands on well-being (e.g., Bakker & Sanz-Vergel, 2013 ; Demirović Bajrami et al., 2022 ). However, in the context of higher education, most research has focused on mediating effects, with limited literature available that provides evidence for a moderating effect of personal resources.
In one of the few studies available, ‘t Mannetje et al. ( 2021 ) used interviews to explore the personal resources high-achieving honors students rely on to perform well in a demanding academic environment. This study across three Dutch universities showed that several personal resources, including self-direction, inquiry-mindedness, and perseverance, were crucial for achieving academic success and helped students cope with the demands they faced. Further, a recent study by Martin et al. ( 2023 ) investigated the roles of self-perceived adaptability and fluid reasoning, which both can be classified as personal resources in an educational setting. The authors hypothesized that students with higher levels of adaptability and fluid reasoning would be less prone to experiencing the adverse effects of a mandated lockdown, which was considered a study demand. Results showed that fluid reasoning (but not adaptability) buffered the unfavorable effects of the lockdown on self-efficacy. In line with JD–R and SD–R models, a boost effect was also observed, indicating that fluid reasoning was a more important resource with a stronger impact on self-efficacy when the demands were high rather than low (Martin et al., 2023 ).
Proposition 5: Similar to study resources, personal resources moderate the impact of study demands on student well-being.
Proactive Self-Enhancing Study Behaviors Producing Gain Spirals
The previous section has shown that study demands and resources have unique as well as multiplicative effects on student well-being. A critical insight in JD–R theory is that individuals do not merely respond to the characteristics of their environment, but rather may take initiative to actively influence this environment (Bakker, 2017 ; Demerouti & Bakker, 2024 ). Accordingly, engaged individuals are motivated to proactively shape the design of their tasks and social environment. This phenomenon is called crafting — the proactive adjustments individuals make in their tasks and social relationships (Wrzesniewski & Dutton, 2001 ) or more broadly in their demands and resources (Tims & Bakker, 2010 ) to enhance the meaning of their activities and to create a better fit between their personal abilities, preferences, and the situation. Several reviews and meta-analyses have shown that job crafting has a range of positive consequences in the organizational context, including increased engagement, meaningfulness, task performance, and prosocial behavior (Demerouti & Bakker, 2024 ; Lazazzara et al., 2020 ; Rudolph et al., 2017 ). Thus, individuals who proactively increase their challenging demands (e.g., start a new project), reduce their hindrance demands (e.g., reduce workload and negative interactions with others), and/or actively increase their resources (e.g., ask for feedback, seek support) are more likely to feel energized and enthusiastic about their work, and consequently perform better (Demerouti & Bakker, 2024 ), creating a positive and upward spiral.
In SD–R theory, we propose that students may also engage in proactive self-enhancing behaviors (e.g., study crafting), to optimize their study environment, engagement, and performance. They are more likely to do so when they feel well and are enthusiastic about their studies (see Fig. 1 ). By taking the personal initiative to influence their study environment, students can sustain their engagement and create an upward spiral of resources, engagement, and study performance (Llorens et al., 2007 ). Bindl and Parker ( 2011 ) have defined such proactive behavior as “self-initiated, anticipatory action aimed at changing either the situation or oneself.” (p. 567). Examples in the study context include taking the initiative to set clear study goals, proactive problem-solving, and proactively using one’s strengths and improving one’s deficits. Students who proactively build a network during their studies are more likely to approach each other for help when needed, and adapt to university life (Brouwer & Engels, 2022 ). Moreover, students’ characteristics and behaviors influence other students’ academic performance and social outcomes — known as peer effects (Cao et al., 2024 ; Yeung & Nguyen-Hoang, 2016 ).
Recent research has shown that students engage in feedback-seeking behaviors, study crafting, and playful study design and that such behaviors can have favorable consequences for student engagement and outcomes. Using a weekly diary design, Körner et al. ( 2021 ) investigated study crafting among higher education students. Findings showed that weekly study resources (decision latitude, social support from lecturers, and support from fellow students) were positively related to weekly study crafting via weekly student engagement. Thus, study resources fostered energy and enthusiasm in students, which, in turn, made them more likely to proactively increase their study challenges, try to learn new things, and ask lecturers for feedback about their performance.
Tho ( 2023 ) investigated the consequences of study crafting (asking for feedback, taking on extra study tasks) among a large sample of Vietnamese undergraduate business students. Results of this study showed that study crafting was an important determinant of satisfaction with study life, when students believed that they had control over their study environment. Particularly when students’ personal resources (e.g., hope, optimism) were high, study crafting was positively related to indicators of happiness and satisfaction with study life. In a similar vein, Mülder et al. ( 2022 ) conducted a study among almost 3000 German university students and found that study crafting was positively related to well-being. Students who proactively improved their study demands and resources were more engaged with their studies, were less exhausted, and reported higher overall well-being (e.g., quality of life, satisfaction with health and personal relationships).
Luu and Vo ( 2020 ) conducted a study among medical students and their teachers. They used observations and video recordings of medical teachers’ authentic leadership (e.g., observations of self-awareness and relational transparency) and student reports of study crafting. The results showed that teacher authentic leadership was positively related to student crafting behaviors. Thus, when teachers were more authentic, students were more likely to proactively seek study challenges and resources. Postema et al. ( 2022 ) conducted a study among Dutch student-athletes and investigated possible spillover effects of study crafting to the sports domain. Consistent with an enrichment perspective, results showed that on the days students engaged in study crafting (increasing challenges, increasing resources, and cognitive crafting), they experienced higher levels of activated positive affect (e.g., feeling inspired, excited, alert) and increased student engagement. Positive affect also had a spillover effect on the sports domain: students who experienced more positive emotions because of study crafting showed better training performance as evident from self- and coach-ratings.
Körner et al. ( 2023 ) evaluated a study crafting intervention’s effectiveness by randomly assigning students to a training or control group. Study crafting and student engagement and exhaustion were assessed before and after the intervention. Results showed that students learned to optimize their study demands and resources (i.e., study crafting), and this increased their levels of student engagement and decreased their levels of exhaustion.
Liu, Zhang, et al. ( 2023 ) investigated another proactive study behavior called playful study design — a cognitive-behavioral approach to study tasks through which tasks and/or activities are redesigned to be more fun and more challenging (Scharp et al., 2023 ). They used a day reconstruction method and collected data from university students across five consecutive days. The results showed that playful study design fostered the daily experiences of flow and flourishing, particularly under difficult conditions (when students often ruminated about COVID-19). In another study, Wang et al. ( 2023 ) investigated the impact of weekly playful study design on student engagement and goal attainment. This study showed that students were highly engaged and successful in achieving their goals when they redesigned their study tasks to be more playful. For example, by guessing the hypothesized outcomes, segmenting tasks to increase the challenge of studying, or by using cognitive mind maps for summarizing the literature, students increased their own engagement and performed better. The effects were strongest for students who were high (vs. low) in proactive personality — they benefitted most from using playful study design. These findings are consistent with JD–R theory and previous findings in the work context showing that job crafting and playful work design have favorable effects on engagement, creativity, and performance (Bakker, Demerouti, & Sanz-Vergel, 2023 ; Bakker & Scharp, 2024 ; Oprea et al., 2019 ).
Proposition 6: Students proactively optimize their own study demands and resources through study crafting and playful study design.
Proposition 7: Student engagement can instigate a gain spiral of proactive, self-enhancing study behaviors, (study and personal) resources, and optimal study demands.
Reactive Self-Undermining Study Behaviors Producing Loss Spirals
Whereas proactive study behaviors play an important role in the gain spiral of SD–R theory, self-undermining behaviors play an important role in the loss spiral (see Fig. 1 ). Self-undermining refers to certain undesirable behaviors in response to stress, creating obstacles (Bakker & Wang, 2020 ). One example is that a student experiencing strain because of an upcoming exam and accompanying high study demands may feel upset and irritated and creates interpersonal conflicts with other students. Students may also have trouble concentrating and, therefore, create a backlog in their study tasks. Self-undermining behaviors create hindrances or new, additional demands that add up to the demands that already exist (Bakker & Costa, 2014 ).
Widlund et al. ( 2021 ) used an accelerated longitudinal study design to investigate differences in Finnish adolescents’ developmental trajectories of school burnout and engagement and their associations with students’ progression in mathematics performance and educational aspirations. One of the trajectory profiles the authors identified was that of declining academic well-being (low and declining engagement, high and increasing burnout). Students in this group started with high performance and aspirations, but they progressed at a slower rate in mathematics and lowered their aspirations over time. Widlund and her colleagues explained these findings by self-undermining behaviors. They argued that self-undermining may have taken the form of poor communication (cf. Bakker & Wang, 2020 ), which reduced the availability of study resources. Students with elevated stress levels may have fallen behind in their studies, and possibly created conflict with peers and teachers because of their own feelings of irritation and impatience. This, in turn, creates more demands over time (Bakker & Costa, 2014 ).
Previous research has provided evidence for such a loss spiral by revealing a reciprocal relationship between school stress and students’ perceived conflicts with teachers (Kiuru et al., 2020 ). In their weekly diary study among German higher education students, Körner et al. ( 2021 ) found that in the weeks students faced higher study demands (time pressure, overload, complex study tasks), they were more likely to feel emotionally exhausted and consequently more likely to show self-undermining behaviors. Particularly in the weeks students faced complex assignments and needed to process a lot of information, they were drained by their studies and reported a backlog in their study tasks, more mistakes, and poorer communication (i.e., self-undermining). Jia et al. ( 2021 ) conducted a study on self-handicapping among Chinese medical students during the COVID-19 crisis. Self-handicapping shows some conceptual overlap with self-undermining. It refers to the process of finding or creating barriers to achieving successful study performance — with the aim of safeguarding one’s sense of self-competence (Jones & Berglas, 1978 ). The results of this study showed that students who experienced higher levels of academic stress (e.g., nervousness and anxiety for the final exams) were more likely to procrastinate and consequently showed more self-handicapping behaviors (e.g., drinking alcohol and deliberately losing learning materials).
Research has also shown that procrastination is predictive of future stress, through maladaptive coping (Sirois & Kitner, 2015 ). Tice and Baumeister ( 1997 ) assert that procrastination is a self-undermining behavior pattern characterized by short-term benefits (such as rest), but long-term costs (such as exhaustion). Their study examined the occurrence and effects of procrastination on physical symptoms and stress, among a small sample of higher education students. The findings showed that at the beginning of the semester, students who procrastinated reported lower levels of stress and fewer illnesses compared to students who did not procrastinate, indicating short-term benefits. However, toward the end of the term, students who procrastinated reported higher stress levels and more illnesses, as well as lower academic performance. This supports the idea of a loss spiral in which students who show self-undermining behaviors (creating a backlog, avoidance coping) create more stress over time.
A recent study was conducted among a large sample of German university students to investigate the relationship between academic procrastination and learning-related anxiety and hope. The study was conducted with 6-week intervals at the beginning, middle, and end of the academic semester (Gadosey et al., 2023 ). The results showed that academic procrastination at the start of the academic semester predicted learning-related anxiety and low levels of learning-related hope during the middle of the semester, which, in turn, resulted in even more procrastination toward the end of the semester. These findings suggest that higher tendencies to procrastinate could lead to low levels of hope over time and that students may end up in a spiral of more self-undermining behavior. Conversely, the study reports that lower tendencies to procrastinate may lead to increasing levels of hope, which relates to the gain spirals mentioned earlier.
These results are consistent with previous findings in an organizational context. For example, Bakker and Wang ( 2020 ) showed in a series of studies that individuals who were exposed to higher job demands felt more exhausted and were more likely to engage in self-undermining behaviors. Using a weekly diary design, Bakker, Xanthopoulou, and Demerouti ( 2023 ) argued and found that weekly emotional demands and workload were predictive of weekly burnout complaints, and indirectly predictive of self-undermining and dysfunctional coping (avoidance and passive coping). These effects were stronger for individuals who already scored relatively high (vs. low) on chronic burnout at the start of the study. Providing additional evidence for a loss cycle, Roczniewska and Bakker ( 2021 ) found that employees who felt lower on energetic resources at the start of the day were more likely to engage in self-undermining behaviors and less likely to engage in job crafting, which consequently undermined their daily functioning.
Proposition 8: Study demands and strain may lead to reactive, maladaptive self-regulation cognitions and behaviors (self-undermining).
Proposition 9: Study-related strain can instigate a loss spiral of self-undermining and study demands.
Student and Higher Education Outcomes
Study characteristics, like job characteristics, can have an important impact on student burnout and engagement and indirectly influence student and higher education outcomes. Student burnout and engagement are positioned as two central well-being constructs in SD–R theory because of their significant impact on student behaviors as well as student and higher education outcomes (see Fig. 1 ).
Research has shown that demands and resources directly relate to key student outcomes. A study among higher education students found that hindering study demands (e.g., high workload, inadequate comprehension in classes) adversely affected students’ academic achievement (i.e., GPA scores). In contrast, challenging study demands (e.g., perception of the degree of work difficulty in classes) showed a positive relationship with students’ academic achievement and were negatively related to students’ hours of withdrawal or disengagement (Travis et al., 2020 ). Study resources have a positive impact on student outcomes. For instance, resources such as student support (instructional, peer, and technical support) were shown to have a positive impact on students’ satisfaction with their study course (Lee et al., 2011 ), while support from family and friends directly affected students’ academic achievement (Saeed et al., 2023 ).
Research among students has also provided evidence of burnout and engagement’s unique and differential effects on various key outcomes. Such outcomes include, but are not limited to, academic performance (e.g., GPA score) (Schreiber & Yu, 2016 ), life satisfaction (Lesener et al., 2020 ), intention to drop out and satisfaction with studies (Álvarez-Pérez et al., 2021 ; Mostert & Pienaar, 2020 ), psychological well-being (Chaudhry et al., 2024 ), students’ likelihood of being satisfied with the higher education experience, and pursuing postgraduate studies (Öz & Boyacı, 2021 ).
However, the relationship between student burnout, engagement, and student outcomes is not necessarily direct or linear. Rather, it is a result of the dynamic interplay of different factors, influenced by both antecedents and outcomes as outlined in the health impairment and the motivational processes. Öz and Boyacı ( 2021 ) conducted a study to examine the association between student engagement and outcomes. Their findings showed that engagement explained variance in students’ GPA scores and increased the likelihood that students were satisfied with their experience at the university, as well as the likelihood that students pursued a postgraduate degree. This is consistent with the idea that activated positive emotions like energy and enthusiasm encourage active involvement with goal pursuits and with the environment (Lyubomirsky et al., 2005 ).
In addition, various studies have shown that student burnout and engagement can act as mediators between study-related antecedents and outcomes in SD–R theory (cf. Fig. 1 ). For example, a study among part-time employed students (Laughman et al., 2016 ) investigated the relationship between work–school conflict and job outcomes. Their findings showed that work–school conflict predicted work outcomes and that burnout mediated these effects. Similarly, Chaudhry et al. ( 2024 ) provided evidence for the mediating effect of student engagement. Their study among management students investigated the relationship between various types of student support and psychological well-being. Their findings showed that academic engagement partially mediated the relationship between a positive internal team environment, family support, and psychological well-being. Moreover, academic engagement fully mediated the relationship between institutional support and psychological well-being.
Körner et al. ( 2021 ) specifically investigated the mediating role of engagement and exhaustion in the relationship between study characteristics and study crafting and self-undermining behaviors among students. Their findings revealed a positive relationship between study resources and study crafting mediated by engagement, as well as a positive relationship between study demands and self-undermining mediated by exhaustion. Another recent survey among medical students found that students with a high risk of burnout tend to have a lower academic performance rate (Ilić & Ilić, 2023 ). Interestingly, this study also found that students with higher GPAs tended to have a higher risk of burnout — highlighting the intricate dynamics between these relationships.
We conclude that when students feel full of energy and are really enthusiastic about their studies (i.e., engaged), they are able to invest considerable cognitive and energetic resources in their studies. Consistent with this perspective, SD–R theory proposes that engaged students are more likely to be proactive (e.g., engage in study crafting, playful work design, and strengths use) and function better. In contrast, when students feel exhausted and cynical about their studies (i.e., burnout), they do not have the psychological resources to invest effort in their studies. As a consequence, they may start to undermine themselves and enter a loss spiral. This has negative implications for their own performance and for the higher education institution at large. Therefore, using previous SD–R models and theory, we may predict student and higher education outcomes, including academic performance, class attendance, learning activities, active participation, and inclination to drop out (Bakker et al., 2015 ; Loyens et al., 2007 ).
Proposition 10: Study demands and resources are directly related to student and higher education outcomes and indirectly related through the mediation of student burnout and engagement.
Recommendations for Research
Now that we have formulated SD–R theory, it is important to set an agenda for future research. Rigorous testing of the propositions put forward in this article is needed. SD–R theory (graphically depicted in Fig. 1 ) can be used to guide such research. Studies could test health impairment versus motivational processes and investigate whether the two processes are indeed unique and predict different outcomes. For example, SD–R theory predicts that study demands are most predictive of physical health and class absence, whereas study resources are most predictive of grades and university dropout. In addition, research should test statistical interactions between study demands and study resources. Are time pressure, interpersonal conflicts, and complex assignments less stressful if students have access to an abundance of study resources (e.g., support from professors, career opportunities) and personal resources (e.g., optimism, self-efficacy)? Do study resources such as study skills workshops and extracurricular activities particularly have a positive influence on engagement and performance when study challenges are high? Another stream of research may test the loss and gain spirals proposed in SD–R theory (Bakker, Demerouti, & Sanz-Vergel, 2023 ; Bakker, Xanthopoulou, & Demerouti, 2023 ). Are students with burnout complaints more likely to show self-undermining and procrastination, and does this lead to a further increase in study demands? Are students high in engagement more likely to craft their studies and to proactively optimize their demands and resources leading to more engagement? Does a playful approach of study tasks and assignments facilitate persistence and help students deal with daily hindrance demands (e.g., repetitive study tasks, financial uncertainty, higher education bureaucracy)?
It should be noted that various pathways in SD–R theory are reciprocal, implying that scholars can use most variables as predictors and outcomes. Thus, next to using study demands as predictors of strain and self-undermining, study demands could be used as outcomes of strain and self-undermining. Similarly, study resources can be modeled and tested as predictors and outcomes of student engagement and study crafting. In short, testing the basic hypotheses in SD–R theory has just started, and we need a range of new studies to further establish its validity. Studies may apply longitudinal research designs with months or years between the assessments, or “shortitudinal” designs (Dormann & Griffin, 2015 ) with daily or weekly assessments so that causal and reversed causal effects can be modeled. Shortitudinal studies collect data over short periods of time, typically a few days or weeks with frequent assessments conducted daily. They allow examining changes in variables over time and capture short-term fluctuations in student experiences and behaviors. We also need rigorous qualitative research to explore the various study demands and resources students are exposed to and to explore their unique experiences in various higher education settings.
Future research may also extend SD–R theory and explore new avenues. Here, we briefly discuss three possible research directions, namely (a) trait versus state effects in SD–R theory; (b) the impact of the higher education climate and lecturer influence; and (c) an expanded SD–R theory.
Traits Versus States in SD–R Theory
Scholars in educational psychology have typically relied on self-report questionnaires and cross-sectional and longitudinal research designs to investigate student well-being and performance. In these studies, the person is the unit of analysis and the statistical analyses are based on differences between persons (e.g., their personalities, or their typical (“trait-like”) study environment, well-being, and behaviors). An underlying assumption in these studies is that the investigated constructs have some stability over time. However, students’ experiences and behaviors may fluctuate considerably over short periods of time, for example, as a function of daily discussions with peers and professors, participation in group coursework, and engagement in extracurricular activities (Bakker et al., 2015 ; Doerksen et al., 2014 ; Xue et al., 2022 ). Such short-term fluctuations (“states”) can be studied using daily diary designs in which not the person, but the situation is the unit of analysis. Diary studies enable researchers to capture “life as it is lived” (Bolger et al., 2003 , p. 597). For example, with the experience sampling method, students may be asked to fill out a brief questionnaire on their smartphone every time they receive a push message. Alternatively, in a daily diary study, students may be requested to fill out a short online questionnaire at the end of every day during a 1- or 2-week period (see Ohly et al., 2010 ).
In JD–R theory (Bakker, 2015 ; Bakker, Demerouti, & Sanz-Vergel, 2023 ), personality is positioned as a trait-level variable that moderates the loss and gain cycles displayed in Fig. 1 . Thus, the impact of daily demands and resources on well-being and study behaviors, as well as the impact of daily (proactive or reactive) behaviors on demands and resources, is proposed to be different for individuals with different personalities (see, for example, Bakker & Oerlemans, 2016 ). In an educational context, this may mean that students high (vs. low) in extraversion (i.e., likely to make contact with other students and be at the center of attention) benefit more from daily social resources in their study environment (feedback, social support). Such social interactions could result in feeling more engaged while studying. There is some preliminary evidence for the proposition that personality traits moderate daily study processes.
Longua et al. ( 2009 ) used a 30-day diary study to examine the influence of personality on how students responded to combinations of negative and positive daily events (e.g., progress on study tasks, conflicts with friends or family). They found that positive daily events buffered the effect of negative daily events on negative affect (e.g., feeling angry, jittery, nervous) for students low in neuroticism and those high in extraversion, but not for students high in neuroticism or low in extraversion. Positive daily events also buffered the impact of negative daily events on night-time stress, but only for students low in neuroticism. Bakker et al. ( 2015 ) conducted a study among psychology students and found that students’ weekly study resources (e.g., social support, feedback) and personal resources (self-esteem, optimism, self-efficacy) facilitated their student engagement (vigor, dedication, and absorption). Student engagement, in turn, was predictive of observed learning activities during the weekly educational group meetings and contributed significantly to the course grade. Moreover, as hypothesized using a SD–R theoretical perspective, the results showed that the impact of study and personal resources on student engagement was stronger for students high versus low in openness to new experiences. Future research should test other personality factors (e.g., conscientiousness, proactive personality) as cross-level moderators of the impact of daily study demands and resources on student well-being, behaviors, and outcomes.
The Higher Education Climate and Lecturer Influence
The abovementioned research recommendations focus on individual students — their perceptions of study demands and resources, their study behaviors, and their well-being. However, students are part of a system and may also be influenced by their teachers or professors, or by the overall climate of the educational institute where they study. The higher education climate refers to “factors that serve as conditions for learning and that support physical and emotional safety, connection and support, and engagement” (U.S. Department of Education, Office of Safe and Healthy Students, 2016 , p. 1). When students perceive that the climate in their institution is psychologically safe, they have a stronger sense of belonging (Allen et al., 2018 ) and their academic achievements are better (Bear et al., 2011 ). We argue that the higher education climate is predictive of study demands and resources. In institutes with a psychologically safe climate, students can be expected to be exposed to reasonable study demands and have access to sufficient study resources (cf. Dollard & Bakker, 2010 ). New research is needed to test psychosocial safety climate as a higher-level variable that influences the study environment, and indirectly contributes to student well-being and performance.
Research with the JD–R theory has shown that leaders may influence the prevalence of job demands and resources, employee well-being, and employee proactive behaviors (e.g., Thun & Bakker, 2018 ; Tummers & Bakker, 2021 ). When leaders empower their employees and show individual consideration — i.e., supporting their development and providing trust and autonomy — their employees are more likely to take initiative and proactively optimize their own job design. Consequently, employees can feel more engaged and perform better (Bakker, 2022 ). Mirroring these leader–employee effects in a work context, future research in educational contexts could test the impact of professors and lecturers on students. This is a multilevel effect in which the enthusiastic behaviors and engagement of professors are expected to influence study demands and resources, student well-being, and student behaviors. For example, it can be hypothesized that engaged professors will be best able to inspire students, influence their enthusiasm and vigor (i.e., student engagement), and influence their study performance (Bakker, 2005 ; Frenzel et al., 2018 ; Pachler et al., 2019 ). Vujčić et al. ( 2022 ) found that teacher engagement was positively related to student well-being because students were more willing to invest time and energy in study tasks and activities. In addition, teacher engagement will have a positive impact on study resources, because engaged teachers are more willing to help their students — offering support, information, and feedback (cf. Christian et al., 2011 ; Simbula & Guglielmi, 2013 ).
Expanding SD–R Theory
We have identified various opportunities for future research, but there are many more options that we will briefly mention here. First, we have indicated that more research on study demands and resources is needed, but students are also confronted with demands and resources in other life domains, including family, self, and sports, for example. Demerouti and Bakker ( 2023 ) have recently integrated demands and resources from the home and personal domains and argued that demands in one domain can be buffered or boosted by resources from another domain. In a study context, the impact of family and personal demands (e.g., high expectations, perfectionism) on student burnout may, for example, be buffered by study resources (e.g., social support and feedback from lecturers).
Second, future research is needed to explore how SD–R theory relates to or complements other established motivational theories within the educational context. One potential theory to explore is expectancy-value theory, which posits that an individual’s motivation is influenced by the perceived likelihood of success and the value they place on the potential outcome (Wigfield & Eccles, 2000 ). This theory could complement SD–R theory by providing a more nuanced understanding of how the study resource of feedback about study performance (e.g., derived from course grades and other indicators of study success) predicts student engagement. In a similar vein, future research could investigate how principles from goal-setting theory could be integrated into SD–R theory. Goal-setting theory proposes that setting specific, challenging, and attainable goals can enhance an individual’s motivation and performance (Locke & Latham, 2002 ). New research could explore how goal-setting influences students’ ability to manage study demands and proactively use study resources, and how these SD–R strategies indirectly facilitate student engagement. As a final example, it would be interesting to investigate how growth mindset (Dweck, 2006 ; Yeager & Dweck, 2020 ) may qualify the impact of daily or weekly study demands and resources on student engagement and performance. Integrating mindset and SD–R theories, it can be hypothesized that study challenges and resources will have a stronger positive impact on engagement and performance for students with a growth mindset, because they tend to view challenges as opportunities to learn and improve, rather than threats. They invest more effort, proactively try new strategies, and seek resources when needed. Exploring the potential synergies between SD–R theory and other motivational theories in the educational psychology literature could contribute to developing more comprehensive and effective interventions for supporting student well-being.
Third and finally, it would be interesting and important to investigate the role of other proactive behaviors students may use next to study crafting and playful study design, and to integrate these behaviors in the SD–R theory. For example, research has elucidated that when students proactively use their character strengths, they report more personal resources (hope, resilience), improved happiness and subjective well-being, and reduced stress and depressive complaints (e.g., Ghielen et al., 2018 ). When students use their strengths (e.g., kindness, courage, creativity), they can be authentic and are more likely to succeed, which boosts their personal resources. In a similar vein, a recent study has shown that when students use proactive vitality management — i.e., individual, goal-oriented behavior aimed at managing physical and mental energy to promote optimal functioning (Op den Kamp et al., 2020 ), they experience more meaning and improved subjective well-being (Zhang et al., 2024 ).
Practical Recommendations
SD–R theory has several implications for practice. Here, we recommend three practical approaches that can be considered. First, the SD–R theory offers a clear framework for the assessment of student well-being and its possible causes and consequences. Higher education institutions may want to include various specific study demands and resources in their underlying survey instruments to assess drivers of student burnout, engagement, and critical outcomes (e.g., information about class attendance, course grades, dropouts, and career progress). Once a higher education institution or faculty has made a clear diagnosis of their students’ levels of study demands and resources and their predictive validity for student well-being, behaviors, and study outcomes, management could detect groups of students with the most versus least favorable study demands and resources and initiate interventions at the group or department level. For example, departments in which students report very high time pressure could take measures by adjusting courses and grading, or by offering their students training in time management, goal setting, and new efficient ways of dealing with high quantities of complex information in a short time. Departments in which students report low opportunities for skill variety could try to initiate novel ways of educating their students.
A second practical implication is that student counsellors may try to reduce self-undermining behaviors and increase proactive study behaviors through training and workshops (proven effective in job crafting interventions; for a meta-analysis, see Oprea et al., 2019 ). A recent study among students has provided evidence for the effectiveness of a study crafting intervention (Körner et al., 2023 ), showing that students can learn to optimize their own study demands and resources, and increase their own well-being. Furthermore, workshops and trainings could be organized to reduce burnout and self-undermining behaviors. These trainings may first use rational emotive training therapy or mindfulness (Madigan et al., 2023 ), and then explain how self-undermining behaviors may be warning signs of burnout. Once students have regained energy in several sessions, they may learn about study crafting to optimize their study design so that the root cause of their burnout complaints is addressed as well.
Third, higher education institutions may provide interventions for lecturers and professors to facilitate positive crossover of engagement and optimization of the study design (study demands and resources). In trainings and workshops, professors may learn about SD–R theory, role-modeling, and the crossover of teacher engagement to students (cf. Bakker, 2005 , 2022 ). Through exercises, lecturers can learn about possible ways to increase study resources for students and to stimulate proactive behaviors such as study crafting and playful study design. Research in the work context has suggested that leaders who intellectually challenge their employees and inspire and empower them can indeed increase employee proactive behaviors and engagement (Bakker, Hetland, et al., 2023 ).
In this article, we introduced the Study Demands–Resources theory to explain various processes that are involved in student well-being (burnout and engagement). We used the well-established Job Demands–Resources theory, previous Study Demands–Resources models, and existing literature on student well-being to develop 10 propositions. Our review showed that students are confronted with a variety of study demands and resources, and that it is crucial to self-regulate the impact of these study characteristics. We identified several studies showing that students can engage in proactive study behaviors, including study crafting and playful study design. Students feel more engaged when they proactively optimize their own job demands and resources and playfully redesign their study tasks to be more fun or more challenging. Higher education institutions’ management, lecturers, professors, and students may use SD–R theory to optimize student well-being and outcomes. We hope that this article will inspire educational psychology scholars, fostering collective efforts to enhance the well-being and academic success of our students.
‘t Mannetje, J., Heijne-Penninga, M., Mastenbroek, N., Wolfensberger, M., & Jaarsma, D. (2021). Personal resources conducive to educational success: High achieving students’ perspectives. Journal of the European Honors Council, 5 (1), 1–20. https://doi.org/10.31378/jehc.147
Article Google Scholar
Allen, H. K., Lilly, F., Green, K. M., Zanjani, F., Vincent, K. B., & Arria, A. M. (2022). Graduate student burnout: Substance use, mental health, and the moderating role of advisor satisfaction. International Journal of Mental Health and Addiction, 20, 1130-1146.
Allen, K., Kern, M. L., Vella-Brodrick, D., Hattie, J., & Waters, L. (2018). What schools need to know about fostering school belonging: A meta-analysis. Educational Psychology Review, 30 , 1–34.
Aloia, L. S., & McTigue, M. (2019). Buffering against sources of academic stress: The influence of supportive informational and emotional communication on psychological well-being. Communication Research Reports, 36 (2), 126–135. https://doi.org/10.1080/08824096.2019.1590191
Álvarez-Pérez, P. R., López-Aguilar, D., González-Morales, M. O., & Peña-Vázquez, R. (2021). Academic engagement and dropout intention in undergraduate university students (p. 152102512110636). https://doi.org/10.1177/15210251211063611
Book Google Scholar
Bakker, A. B. (2005). Flow among music teachers and their students: The crossover of peak experiences. Journal of Vocational Behavior, 66 , 26–44.
Bakker, A. B. (2015). Towards a multilevel approach of employee well-being. European Journal of Work and Organizational Psychology, 24 (6), 839–843. https://doi.org/10.1080/1359432x.2015.1071423
Bakker, A. B. (2017). Strategic and proactive approaches to work engagement. Organizational Dynamics, 46 (2), 67–75. https://doi.org/10.1016/j.orgdyn.2017.04.002
Bakker, A. B. (2022). The social psychology of work engagement: State of the field. Career Development International, 27 , 36–53.
Bakker, A. B., & Costa, P. (2014). Chronic job burnout and daily functioning: A theoretical analysis. Burnout Research, 1 (3), 112–119. https://doi.org/10.1016/j.burn.2014.04.003
Bakker, A. B., & Demerouti, E. (2007). The Job Demands-Resources model: State of the art. Journal of Managerial Psychology, 22 (3), 309–328. https://doi.org/10.1108/02683940710733115
Bakker, A. B., & Demerouti, E. (2024). Job Demands–Resources theory: Frequently-asked questions. Journal of Occupational Health Psychology .
Bakker, A. B., Demerouti, E., & Sanz-Vergel, A. (2023). Job Demands–Resources theory: Ten years later. Annual Review of Organizational Psychology and Organizational Behavior, 10 , 25–53.
Bakker, A. B., Demerouti, E., & Sanz-Vergel, A. I. (2014). Burnout and work engagement: The JD–R approach. Annual Review of Organizational Psychology and Organizational Behavior, 1 (1), 389–411. https://doi.org/10.1146/annurev-orgpsych-031413-091235
Bakker, A. B., Hetland, J., Kjellevold-Olsen, O., & Espevik, R. (2023). Daily transformational leadership: A source of inspiration for follower performance? European Management Journal, 41 , 700–708.
Bakker, A. B., & Oerlemans, W. G. (2016). Momentary work happiness as a function of enduring burnout and work engagement. The Journal of Psychology, 150 (6), 755–778. https://doi.org/10.1080/00223980.2016.1182888
Bakker, A. B., & Sanz-Vergel, A. I. (2013). Weekly work engagement and flourishing: The role of hindrance and challenge job demands. Journal of Vocational Behavior, 83 (3), 397–409. https://doi.org/10.1016/j.jvb.2013.06.008
Bakker, A. B., Sanz-Vergel, A. I., & Kuntze, J. (2015). Student engagement and performance: A weekly diary study on the role of openness. Motivation and Emotion, 39 (1), 49–62. https://doi.org/10.1007/s11031-014-9422-5
Bakker, A. B., & Scharp, Y. S. (2024). It pays to play: Playful work design. In S. Parker, F. Klonek, C. Knight, & F. Zhang (Eds.), Transformative work design: Synthesis and new directions . SIOP Frontier Series.
Google Scholar
Bakker, A. B., & Van Wingerden, J. (2021). Do personal resources and strengths use increase work engagement? The effects of a training intervention. Journal of Occupational Health Psychology, 26 , 20–30.
Bakker, A. B., & Wang, Y. (2020). Self-undermining behavior at work: Evidence of construct and predictive validity. International Journal of Stress Management, 27 (3), 241–251. https://doi.org/10.1037/str0000150
Bakker, A. B., Xanthopoulou, D., & Demerouti, E. (2023). How does chronic burnout affect dealing with weekly job demands? A test of central propositions in JD–R and COR-theories. Applied Psychology: An International Review, 72 , 389–410.
Bear, G. G., Gaskins, C., Blank, J., & Chen, F. F. (2011). Delaware School Climate Survey—Student: Its factor structure, concurrent validity, and reliability. Journal of School Psychology, 49 (2), 157–174. https://doi.org/10.1016/j.jsp.2011.01.001
Bellini, D., Barbieri, B., Barattucci, M., Mascia, M. L., & Ramaci, T. (2022). The role of a restorative resource in the academic context in improving intrinsic and extrinsic motivation and flow within the Job Demands–Resources Model. International Journal of Environmental Research and Public Health, 19 (22), 15263. https://doi.org/10.3390/ijerph192215263
Bindl, U. K., & Parker, S. K. (2011). Proactive work behavior: Forward-thinking and change-oriented action in organizations. In S. Zedeck (Ed.), APA handbook of industrial and organizational psychology, Vol. 2. Selecting and developing members for the organization (pp. 567–598). American Psychological Association.
Chapter Google Scholar
Bolger, N., Davis, A., & Rafaeli, E. (2003). Diary methods: Capturing life as it is lived. Annual Review of Psychology, 54 (1), 579–616. https://doi.org/10.1146/annurev.psych.54.101601.145030
Brouwer, J., & Engels, M. C. (2022). The role of prosocial attitudes and academic achievement in peer networks in higher education. European Journal of Psychology of Education, 37 (2), 567–584.
Bücker, S., Nuraydin, S., Simonsmeier, B. A., Schneider, M., & Luhmann, M. (2018). Subjective well-being and academic achievement: A meta-analysis. Journal of Research in Personality, 74 , 83–94.
Bumbacco, C., & Scharfe, E. (2023). Why attachment matters: First-year post-secondary students’ experience of burnout, disengagement, and drop-out. Journal of College Student Retention: Research, Theory & Practice, 24 (4), 988–1001.
Calderwood, C., & Gabriel, A. S. (2017). Thriving at school and succeeding at work? A demands-resources view of spillover processes in working students. Journal of Vocational Behavior, 103 , 1–13.
Cao, Y., Zhou, T., & Gao, J. (2024). Heterogeneous peer effects of college roommates on academic performance. Nature Communications, 15 , 4785.
Chaudhry, S., Tandon, A., Shinde, S., & Bhattacharya, A. (2024). Student psychological well-being in higher education: The role of internal team environment, institutional, friends and family support and academic engagement. PLoS One, 19 (1), e0297508. https://doi.org/10.1371/journal.pone.0297508
Cheng, J., Zhao, Y. Y., Wang, J., & Sun, Y. H. (2020). Academic burnout and depression of Chinese medical students in the pre-clinical years: The buffering hypothesis of resilience and social support. Psychology, Health & Medicine, 25 , 1094–1105.
Cho, S., Lee, M., & Lee, S. M. (2023). Burned-out classroom climate, intrinsic motivation, and academic engagement: Exploring unresolved issues in the Job Demands-Resources Model. Psychological Reports, 126 (4), 1954–1976. https://doi.org/10.1177/00332941211054776
Christian, M. S., Garza, A. S., & Slaughter, J. E. (2011). Work engagement: A quantitative review and test of its relations with task and contextual performance. Personnel Psychology, 64 , 89–136.
Cilliers, J. R., Mostert, K., & Nel, J. A. (2018). Study demands, study resources and the role of personality characteristics in predicting the engagement of first-year university students. South African Journal of Higher Education, 32 (1). https://doi.org/10.20853/32-1-1575
Clements, A. J., & Kamau, C. (2018). Understanding students’ motivation towards proactive career behaviours through goal-setting theory and the job demands-resources model. Studies in Higher Education, 43 (12), 2279–2293. https://doi.org/10.1080/03075079.2017.1326022
Collie, R. J., Martin, A. J., Malmberg, L., Hall, J., & Ginns, P. (2015). Academic buoyancy, student’s achievement, and the linking role of control: A cross-lagged analysis of high school students. British Journal of Educational Psychology, 85 (1), 113–130. https://doi.org/10.1111/bjep.12066
Demerouti, E., & Bakker, A. B. (2023). Job demands-resources theory in times of crises: New propositions. Organizational Psychology Review, 13 (3), 209–236. https://doi.org/10.1177/20413866221135022
Demerouti, E., & Bakker, A. B. (2024). Job crafting: A powerful job redesign approach. In M. C. W. Peeters, J. de Jonge, & T. Taris (Eds.), An introduction to contemporary work psychology . Wiley.
Demerouti, E., Bakker, A. B., Nachreiner, F., & Schaufeli, W. B. (2001). The job demands-resources model of burnout. Journal of Applied Psychology, 86 (3), 499–512. https://doi.org/10.1037/0021-9010.86.3.499
Demirović Bajrami, D., Petrović, M. D., Sekulić, D., Radovanović, M. M., Blešić, I., Vuksanović, N., Cimbaljević, M., & Tretiakova, T. N. (2022). Significance of the work environment and personal resources for employees’ well-being at work in the hospitality sector. International Journal of Environmental Research and Public Health, 19 (23), 16165.
Doerksen, S. E., Elavsky, S., Rebar, A. L., & Conroy, D. E. (2014). Weekly fluctuations in college student leisure activities and well-being. Leisure Sciences, 36 , 14–34.
Dollard, M. F., & Bakker, A. B. (2010). Psychosocial safety climate as a precursor to conducive work environments, psychological health problems, and employee engagement. Journal of Occupational and Organizational Psychology, 83 , 579–599.
Dormann, C., & Griffin, M. (2015). Optimal time lags in panel studies. Psychological Methods, 20 (4), 489–505. https://doi.org/10.1037/met0000041
Dweck, C. S. (2006). Mindset: The new psychology of success . Random house.
Dyrbye, L. N., Thomas, M. R., Massie, F. S., Power, D. V., Eacker, A., Harper, W., Durning, S., Moutier, C., Szydlo, D. W., Novotny, P. J., Sloan, J. A., & Shanafelt, T. D. (2008). Burnout and suicidal ideation among U.S. medical students. Annals of Internal Medicine, 149 , 334–341.
Finn, J. D., & Zimmer, K. S. (2012). Student engagement: What is it? Why does it matter? In S. L. Christenson, A. L. Reschly, & C. Wylie (Eds.), Handbook of research on student engagement (pp. 97–131). Springer Science + Business Media. https://doi.org/10.1007/978-1-4614-2018-7_5
Frenzel, A. C., Becker-Kurz, B., Pekrun, R., Goetz, T., & Lüdtke, O. (2018). Emotion transmission in the classroom revisited: A reciprocal effects model of teacher and student enjoyment. Journal of Educational Psychology, 110 (5), 628–639.
Gadosey, C. K., Schnettler, T., Scheunemann, A., Bäulke, L., Thies, D. O., Dresel, M., Fries, S., Leutner, D., Wirth, J., & Grunschel, C. (2023). Vicious and virtuous relationships between procrastination and emotions: An investigation of the reciprocal relationship between academic procrastination and learning-related anxiety and hope. European Journal of Psychology of Education. https://doi.org/10.1007/s10212-023-00756-8
Ghielen, S. T. S., Van Woerkom, M., & Meyers, M. C. (2018). Promoting positive outcomes through strengths interventions: A literature review. The Journal of Positive Psychology, 13 , 573–585.
Gusy, B., Wörfel, F., & Lohmann, K. (2016). Erschöpfung und Engagement im Studium. Zeitschrift Für Gesundheitspsychologie, 24 (1), 41–53. https://doi.org/10.1026/0943-8149/a000153
Haverila, M. J., Haverila, K., & McLaughlin, C. (2020). Variables affecting the retention intentions of students in higher education institutions. Journal of International Students, 10 (2), 358–382. https://doi.org/10.32674/jis.v10i2.1849
Hobfoll, S. E., Halbesleben, J. R. B., Neveu, J., & Westman, M. (2018). Conservation of resources in the organizational context: The reality of resources and their consequences. Annual Review of Organizational Psychology and Organizational Behavior, 5 (1), 103–128. https://doi.org/10.1146/annurev-orgpsych-032117-104640
Hospel, V., & Galand, B. (2016). Are both classroom autonomy support and structure equally important for students’ engagement? A multilevel analysis. Learning and Instruction, 41 , 1–10.
Hu, S. (2010). Reconsidering the relationship between student engagement and persistence in college. Innovative Higher Education, 36 (2), 97–106. https://doi.org/10.1007/s10755-010-9158-4
Ilić, I., & Ilić, M. (2023). The relationship between the burnout syndrome and academic success of medical students: A cross-sectional study. Arhiv Za Higijenu Rada I Toksikologiju, 74 (2), 134–141. https://doi.org/10.2478/aiht-2023-74-3719
Jackson, E. R., Shanafelt, T. D., Hasan, O., Satele, D. V., & Dyrbye, L. N. (2016). Burnout and alcohol abuse/dependence among US medical students. Academic Medicine, 91 (9), 1251–1256. https://doi.org/10.1097/ACM.0000000000001138
Jagodics, B., & Szabó, É. (2023). Student burnout in higher education: A demand-resource model approach. Trends in Psychology, 31 (4), 757–776.
Jia, J., Wang, L., Xu, J., Lin, X., Zhang, B., & Jiang, Q. (2021). Self-handicapping in Chinese medical students during the COVID-19 pandemic: The role of academic anxiety, procrastination and hardiness. Frontiers in Psychology, 12 , 741821.
Jones, E. E., & Berglas, S. (1978). Control of attributions about the self through self-handicapping strategies: The appeal of alcohol and the role of underachievement. Personality and Social Psychology Bulletin, 4 , 200–206.
Junça Silva, A. J., Abreu, P., & Da Rocha Brito, S. M. (2022). The path from social and organizational resources to satisfaction: The mediating role of academic engagement and the moderating role of psychological capital. International Journal of Educational Management, 36 (5), 750–765. https://doi.org/10.1108/ijem-01-2022-0041
Kaggwa, M. M., Kajjimu, J., Sserunkuma, J., Najjuka, S. M., Atim, L. M., Olum, R., Tagg, A., & Bongomin, F. (2021). Prevalence of burnout among university students in low- and middle-income countries: A systematic review and meta-analysis. PLoS One, 16 (8), e0256402. https://doi.org/10.1371/journal.pone.0256402
Kaya, M., & Erdem, C. (2021). Students’ well-being and academic achievement: A meta-analysis study. Child Indicators Research, 14 (5), 1743–1767. https://doi.org/10.1007/s12187-021-09821-4
Kiuru, N., Wang, M.-T., Salmela-Aro, K., Kannas, L., Ahonen, T., & Hirvonen, R. (2020). Associations between adolescents’ interpersonal relationships, school well-being, and academic achievement during educational transitions. Journal of Youth and Adolescence, 49 , 1057–1072.
Knight, C., Patterson, M., & Dawson, J. (2017). Building work engagement: A systematic review and meta-analysis investigating the effectiveness of work engagement interventions. Journal of Organizational Behavior, 38 , 792–812.
Körner, L. S., Mülder, L. M., Bruno, L., Janneck, M., Dettmers, J., & Rigotti, T. (2023). Fostering study crafting to increase engagement and reduce exhaustion among higher education students: A randomized controlled trial of the STUDYCoach online intervention. Applied Psychology: Health and Well-Being, 15 , 776–802.
Körner, L. S., Rigotti, T., & Rieder, K. (2021). Study crafting and self-undermining in higher education students: A weekly diary study on the antecedents. International Journal of Environmental Research and Public Health, 18 , 7090.
Kulikowski, K., Potoczek, A., Antipow, E., & Król, S. (2019). How to survive in academia: Demands, resources and study satisfaction among Polish PhD students. Kuram Ve Uygulamada Egitim Bilimleri, 19 (4), 65–79. https://doi.org/10.12738/estp.2019.4.005
Laughman, C. A., Boyd, E. M., & Rusbasan, D. (2016). Burnout as a mediator between work–school conflict and work outcomes. Journal of Career Development, 43 (5), 413–425. https://doi.org/10.1177/0894845316633523
Lazazzara, A., Tims, M., & de Gennaro, D. (2020). The process of reinventing a job: A meta–synthesis of qualitative job crafting research. Journal of Vocational Behavior, 116 (Part B), 103267.
Lee, S. J., Srinivasan, S., Trail, T., Lewis, D. C., & Lopez, S. (2011). Examining the relationship among student perception of support, course satisfaction, and learning outcomes in online learning. The Internet and Higher Education, 14 (3), 158–163. https://doi.org/10.1016/j.iheduc.2011.04.001
Lee, T. R., Lee, S., Ko, H., & Lee, S. M. (2022). Self-compassion among university students as a personal resource in the Job Demand-Resources Model. Educational Psychology, 42 (9), 1160–1179. https://doi.org/10.1080/01443410.2022.2120600
Lesener, T., Gusy, B., & Wolter, C. (2019). The job demands-resources model: A meta-analytic review of longitudinal studies. Work & Stress, 33 , 76–103.
Lesener, T., Pleiss, L. S., Gusy, B., & Wolter, C. (2020). The study demands–resources framework: An empirical introduction. International Journal of Environmental Research and Public Health, 17 , 5183.
Liu, M., Gorgievski, M. J., Zwaga, J., & Paas, F. (2023). How entrepreneurship program characteristics foster students’ study engagement and entrepreneurial career intentions: A longitudinal study. Learning and Individual Differences, 101 , 102249. https://doi.org/10.1016/j.lindif.2022.102249
Liu, W., Zhang, W., van der Linden, D., & Bakker, A. B. (2023). Flow and flourishing during the pandemic: The roles of strengths use and playful design. Journal of Happiness Studies, 24 (7), 2153–2175. https://doi.org/10.1007/s10902-023-00670-2
Llorens, S., Schaufeli, W. B., Bakker, A. B., & Salanova, M. (2007). Does a positive gain spiral of resources, efficacy beliefs and engagement exist? Computers in Human Behavior, 23 (1), 825–841. https://doi.org/10.1016/j.chb.2004.11.012
Locke, E. A., & Latham, G. P. (2002). Building a practically useful theory of goal setting and task motivation: A 35-year odyssey. American Psychologist, 57 (9), 705–717.
Longua, J., DeHart, T., Tennen, H., & Armeli, S. (2009). Personality moderates the interaction between positive and negative daily events predicting negative affect and stress. Journal of Research in Personality, 43 , 547–555.
Loyens, S. M. M., Rikers, R. M. J. P., & Schmidt, H. G. (2007). The impact of students’ conceptions of constructivist assumptions on academic achievement and drop-out. Studies in Higher Education, 32 , 581–602.
Luthans, B. C., Luthans, K. W., & Avey, J. B. (2013). Building the leaders of tomorrow. Journal of Leadership & Organizational Studies, 21 (2), 191–199. https://doi.org/10.1177/1548051813517003
Luu, T. T., & Vo, T. T. (2020). Crafting study tasks among medical students: The role of authentic medical teachers. BMC Medical Education, 20 , 430.
Lyubomirsky, S., King, L. A., & Diener, E. (2005). The benefits of frequent positive affect: Does happiness lead to success? Psychological Bulletin, 131 (6), 803–855. https://doi.org/10.1037/0033-2909.131.6.803
Ma, Y., & Bennett, D. (2021). The relationship between higher education students’ perceived employability, academic engagement and stress among students in China. Education + Training, 63 , 744–762.
Madigan, D. J., & Curran, T. (2021). Does burnout affect academic achievement? A meta-analysis of over 100,000 students. Educational Psychology Review, 33 (2), 387–405. https://doi.org/10.1007/s10648-020-09533-1
Madigan, D. J., Kim, L. E., & Glandorf, H. L. (2023). Interventions to reduce burnout in students: A systematic review and meta-analysis. European Journal of Psychology of Education , 1–27.
Martin, A. J., & Collie, R. J. (2022). The Academic and Cultural Demands-Resources (ACD-R) Framework: Supporting the academic development of culturally and ethnically diverse students. In A. J. Holliman & K. Sheehy (Eds.), Overcoming adversity in education (pp. 249–261). Routledge. https://doi.org/10.4324/9781003180029-22
Martin, A. J., Collie, R. J., & Nagy, R. P. (2021). Adaptability and high school students’ online learning during COVID-19: A Job Demands-Resources Perspective. Frontiers in Psychology, 12 , 702163. https://doi.org/10.3389/fpsyg.2021.702163
Martin, A. J., Ginns, P., & Collie, R. J. (2023). University students in COVID-19 lockdown: The role of adaptability and fluid reasoning in supporting their academic motivation and engagement. Learning and Instruction, 83 , 101712. https://doi.org/10.1016/j.learninstruc.2022.101712
Martínez, I. G., Youssef-Morgan, C. M., Chambel, M. J., & Pinto, A. M. (2019). Antecedents of academic performance of university students: Academic engagement and psychological capital resources. Educational Psychology, 39 (8), 1047–1067. https://doi.org/10.1080/01443410.2019.1623382
Mokgele, K. R. F., & Rothmann, S. (2014). A structural model of student well-being. South African Journal of Psychology, 44 (4), 514–527. https://doi.org/10.1177/0081246314541589
Mostert, K., & Pienaar, J. (2020). The moderating effect of social support on the relationship between burnout, intention to drop out, and satisfaction with studies of first-year university students. Journal of Psychology in Africa, 30 (3), 197–202. https://doi.org/10.1080/14330237.2020.1767928
Mülder, L. M., Schimek, S., Werner, A. M., Reichel, J. L., Heller, S., Tibubos, A. N., Schäfer, M., Dietz, P., Letzel, S., Beutel, M. E., Stark, B., Simon, P., & Rigotti, T. (2022). Distinct patterns of university students study crafting and the relationships to exhaustion, well-being, and engagement. Frontiers in Psychology, 13 , 895930.
Munir, J., Faiza, M., Jamal, B., Daud, S., & Iqbal, K. (2023). The impact of socio-economic status on academic achievement. Journal of Social Sciences Review, 3 (2), 695–705. https://doi.org/10.54183/jssr.v3i2.308
Naylor, R. (2022). Key factors influencing psychological distress in university students: The effects of tertiary entrance scores. Studies in Higher Education, 47 (3), 630–642. https://doi.org/10.1080/03075079.2020.1776245
Ohly, S., Sonnentag, S., Niessen, C., & Zapf, D. (2010). Diary studies in organizational research: An introduction and some practical recommendations. Journal of Personnel Psychology, 9 , 79–93.
Op den Kamp, E. M., Bakker, A. B., Tims, M., & Demerouti, E. (2020). Proactive vitality management and creative work performance: The role of self-insight and social support. The Journal of Creative Behavior, 54 , 323–336.
Oprea, B. T., Barzin, L., Vîrgă, D., Iliescu, D., & Rusu, A. (2019). Effectiveness of job crafting interventions: A meta-analysis and utility analysis. European Journal of Work and Organizational Psychology, 28 , 723–741.
Öz, Y., & Boyacı, A. (2021). The role of student engagement in student outcomes in higher education: Implications from a developing country. International Journal of Educational Research, 110 , 101880. https://doi.org/10.1016/j.ijer.2021.101880
Pachler, D., Kuonath, A., & Frey, D. (2019). How transformational lecturers promote students’ engagement, creativity, and task performance: The mediating role of trust in lecturer and self-efficacy. Learning and Individual Differences, 69 , 162–172.
Pekrun, R., & Linnenbrink-Garcia, L. (2012). Academic emotions and student engagement. In S. L. Christenson, A. L. Reschly, & C. Wylie (Eds.), Handbook of research on student engagement (pp. 259–282). Springer Science + Business Media. https://doi.org/10.1007/978-1-4614-2018-7_5
Postema, A., Van Mierlo, H., Bakker, A. B., & Barendse, M. T. (2022). Study-to-sports spillover among competitive athletes: A field study. International Journal of Sport and Exercise Psychology , 1–24.
Robayo-Tamayo, M., Blanco-Donoso, L. M., Román, F. J., Carmona-Cobo, I., Moreno-Jiménez, B., & Garrosa, E. (2020). Academic engagement: A diary study on the mediating role of academic support. Learning and Individual Differences, 80 , 101887.
Robins, T. G., Roberts, R. M., & Sarris, A. (2015). Burnout and engagement in health profession students: The relationships between study demands, study resources and personal resources. Australasian Journal of Organisational Psychology, 8 (e1), 1–13. https://doi.org/10.1017/orp.2014.7
Roczniewska, M., & Bakker, A. B. (2021). Burnout and self-regulation failure: A diary study of self-undermining and job crafting among nurses. Journal of Advanced Nursing, 77 , 3424–3435.
Rudolph, C. W., Katz, I. M., Lavigne, K. N., & Zacher, H. (2017). Job crafting: A meta-analysis of relationships with individual differences, job characteristics, and work outcomes. Journal of Vocational Behavior, 102 , 112–138. https://doi.org/10.1016/j.jvb.2017.05.008
Saeed, K. M., Ahmed, A. S., Rahman, Z. F., & Sleman, N. A. (2023). How social support predicts academic achievement among secondary students with special needs: The mediating role of self-esteem. Middle East Current Psychiatry, 30 (1), 1–9. https://doi.org/10.1186/s43045-023-00316-2
Salanova, M., Schaufeli, W. B., Martínez, I. M. M., & Bresó, E. (2010). How obstacles and facilitators predict academic performance: The mediating role of study burnout and engagement. Anxiety, Stress, & Coping, 23 (1), 53–70. https://doi.org/10.1080/10615800802609965
Salmela-Aro, K., & Read, S. (2017). Study engagement and burnout profiles among Finnish higher education students. Burnout Research, 7 , 21–28.
Salmela-Aro, K., Tang, X., & Upadyaya, K. (2022). Study Demands-Resources model of student engagement and burnout. In A. L. Reschly & S. L. Christenson (Eds.), Handbook of research on student engagement (pp. 77–93). Springer. https://doi.org/10.1007/978-3-031-07853-84
Salmela-Aro, K., & Upadyaya, K. (2014). School burnout and engagement in the context of demands-resources model. British Journal of Educational Psychology, 84 (1), 137–151.
Scharp, Y., Bakker, A. B., Breevaart, K., Kruup, K., & Uusberg, A. (2023). Playful work design: Conceptualization, measurement, and validity. Human Relations, 76 , 509–550.
Schaufeli, W. B., & Bakker, A. B. (2004). Job demands, job resources, and their relationship with burnout and engagement: A multi-sample study. Journal of Organizational Behavior, 25 (3), 293–315. https://doi.org/10.1002/job.248
Schaufeli, W. B., Salanova, M., González-Romá, V., & Bakker, A. B. (2002). The measurement of engagement and burnout: A two sample confirmatory factor analytic approach. Journal of Happiness Studies, 3 (1), 71–92. https://doi.org/10.1023/A:1015630930326
Schreiber, B., & Yu, D. (2016). Exploring student engagement practices at a South African university: Student engagement as a reliable predictor of academic performance. South African Journal of Higher Education, 30 (5). https://doi.org/10.20853/30-5-593
Seibert, G. S., Bauer, K. N., May, R. W., & Fincham, F. D. (2017). Emotion regulation and academic underperformance: The role of school burnout. Learning and Individual Differences, 60 , 1–9.
Simbula, S., & Guglielmi, D. (2013). I am engaged, I feel good, and I go the extra-mile: Reciprocal relationships between work engagement and consequences. Journal of Work and Organizational Psychology, 29 (3), 117–125.
Sirois, F. M., & Kitner, R. (2015). Less adaptive or more maladaptive? A meta–analytic investigation of procrastination and coping. European Journal of Personality, 29 (4), 433–444.
Theron, B. (2022). Demands and resources experienced by first-year students in a South African higher education institute: Developing and testing an integrated framework (Unpublished Doctoral thesis) . North-West University.
Tho, N. D. (2023). Business students’ psychological capital and quality of university life: The moderating role of study crafting. Journal of Education and Training, 65 (1), 163–177. https://doi.org/10.1108/et-05-2022-0176
Thun, S., & Bakker, A. B. (2018). Empowering leadership and job crafting: The role of employee optimism. Stress and Health, 34 (4), 573–581. https://doi.org/10.1002/smi.2818
Tice, D. M., & Baumeister, R. F. (1997). Longitudinal study of procrastination, performance, stress, and health: The costs and benefits of dawdling. Psychological Science, 8 , 454–445.
Tims, M., & Bakker, A. B. (2010). Job crafting: Towards a new model of individual job redesign. South African Journal of Industrial Psychology, 36 (2), 841. https://doi.org/10.4102/sajip.v36i2.841
Travis, J., Kaszycki, A., Geden, M., & Bunde, J. (2020). Some stress is good stress: The challenge-hindrance framework, academic self-efficacy, and academic outcomes. Journal of Educational Psychology, 112 (8), 1632–1643. https://doi.org/10.1037/edu0000478
Tummers, L., & Bakker, A. B. (2021). Leadership and Job Demands-Resources theory: A systematic review. Frontiers in Psychology, 12 , 722080. https://doi.org/10.3389/fpsyg.2021.722080
Tuominen-Soini, H., & Salmela-Aro, K. (2014). Schoolwork engagement and burnout among Finnish high school students and young adults: Profiles, progressions, and educational outcomes. Developmental Psychology, 50 , 649–662.
Turhan, D., Schnettler, T., Scheunemann, A., Gadosey, C. K., Kegel, L. S., Bäulke, L., Thies, D. O., Thomas, L., Buhlmann, U., Dresel, M., Fries, S., Leutner, D., Wirth, J., & Grunschel, C. (2022). University students’ profiles of burnout symptoms amid the COVID-19 pandemic in Germany and their relation to concurrent study behavior and experiences. International Journal of Educational Research, 116 , 102081. https://doi.org/10.1016/j.ijer.2022.102081
U.S. Department of Education, & Office of Safe and Healthy Students. (2016). Quick guide on making school climate improvements . Author.
Van Zyl, A. (2016). The contours of inequality: The links between socio-economic status of students and other variables at the University of Johannesburg. Journal of Student Affairs in Africa, 4 (1), 1–13. https://doi.org/10.14426/jsaa.v4i1.141
Vansteenkiste, M., Sierens, E., Soenens, B., Luyckx, K., & Lens, W. (2009). Motivational profiles from a self-determination perspective: The quality of motivation matters. Journal of Educational Psychology, 101 (3), 671–688.
Vujčić, M. T., Garcia-Garzon, E., Gonul, B., & Gioaba, I. (2022). From teachers’ work engagement to pupils’ positive affect: A weekly diary study on the role of pupils’ autonomous motivation. Applied Research Quality Life, 17 , 613–633.
Wang, H., Ren, Y., & Liu, W. (2023). A weekly diary study on playful study design, study engagement, and goal attainment: The role of proactive personality. Journal of Happiness Studies, 24 (1), 159–184.
Widlund, A., Tuominen, H., & Korhonen, J. (2021). Development of school engagement and burnout across lower and upper secondary education: Trajectory profiles and educational outcomes. Contemporary Educational Psychology, 66 , 1–13.
Wigfield, A., & Eccles, J. S. (2000). Expectancy–value theory of achievement motivation. Contemporary Educational Psychology, 25 (1), 68–81. https://doi.org/10.1006/ceps.1999.1015
Wilson, E. V., & Sheetz, S. D. (2010). A demands-resources model of work pressure in IT student task groups. Computers & Education, 55 (1), 415–426. https://doi.org/10.1016/j.compedu.2010.02.006
Wolff, W., Brand, R., Baumgarten, F., Lösel, J., & Ziegler, M. (2014). Modeling students’ instrumental (mis-) use of substances to enhance cognitive performance: Neuroenhancement in the light of job-demands-resources theory. BioPsychoSocial Medicine, 8 (1), 2–22. https://doi.org/10.1186/1751-0759-8-12
Wrzesniewski, A., & Dutton, J. E. (2001). Crafting a job: Revisioning employees as active crafters of their work. Academy of Management Review, 26 (2), 179–201. https://doi.org/10.2307/259118
Xanthopoulou, D., Bakker, A. B., Demerouti, E., & Schaufeli, W. B. (2009). Reciprocal relationships between job resources, personal resources, and work engagement. Journal of Vocational Behavior, 74 , 235–244.
Xue, Q., Yang, J., Wang, H., & Zhang, D. (2022). How and when leisure crafting enhances college students’ well-being: A (quantitative) weekly diary study. Psychology Research and Behavior Management, 15 , 273–290.
Yeager, D. S., & Dweck, C. S. (2020). What can be learned from growth mindset controversies? American Psychologist, 75 (9), 1269.
Yeung, R., & Nguyen-Hoang, P. (2016). Endogenous peer effects: Fact or fiction? The Journal of Educational Research, 109 (1), 37–49. https://doi.org/10.1080/00220671.2014.918528
Zhang, J., Zheng, W., Li, H., Hua, W., & Fu, M. (2024). Meaning matters: Linking proactive vitality management to subjective well-being. Journal of General Psychology, 22 , 1–24.
Download references
Author information
Authors and affiliations.
Center of Excellence for Positive Organizational Psychology, Erasmus University Rotterdam, Woudestein Campus, Mandeville Building T16-35, PO Box 1738, 3000, DR, Rotterdam, The Netherlands
Arnold B. Bakker
North-West University, Potchefstroom, South Africa
Arnold B. Bakker & Karina Mostert
You can also search for this author in PubMed Google Scholar
Corresponding author
Correspondence to Arnold B. Bakker .
Ethics declarations
Conflict of interest.
The authors declare no competing interests.
Additional information
Publisher’s note.
Springer Nature remains neutral with regard to jurisdictional claims in published maps and institutional affiliations.
Rights and permissions
Open Access This article is licensed under a Creative Commons Attribution 4.0 International License, which permits use, sharing, adaptation, distribution and reproduction in any medium or format, as long as you give appropriate credit to the original author(s) and the source, provide a link to the Creative Commons licence, and indicate if changes were made. The images or other third party material in this article are included in the article's Creative Commons licence, unless indicated otherwise in a credit line to the material. If material is not included in the article's Creative Commons licence and your intended use is not permitted by statutory regulation or exceeds the permitted use, you will need to obtain permission directly from the copyright holder. To view a copy of this licence, visit http://creativecommons.org/licenses/by/4.0/ .
Reprints and permissions
About this article
Bakker, A.B., Mostert, K. Study Demands–Resources Theory: Understanding Student Well-Being in Higher Education. Educ Psychol Rev 36 , 92 (2024). https://doi.org/10.1007/s10648-024-09940-8
Download citation
Accepted : 12 August 2024
Published : 19 August 2024
DOI : https://doi.org/10.1007/s10648-024-09940-8
Share this article
Anyone you share the following link with will be able to read this content:
Sorry, a shareable link is not currently available for this article.
Provided by the Springer Nature SharedIt content-sharing initiative
- Student burnout
- Student engagement
- Study crafting
- Study demands
- Study resources
- Find a journal
- Publish with us
- Track your research
What can significantly boost the Philippine economy?

Using historical analysis and empirical evidence, DLSU School of Economics Distinguished Professor and Angelo King Institute of Economic and Business Studies Director Dr. Jesus Felipe offers a fresh outlook on the development of an innovative economy and the role of the government and private sector in shaping it.
With a 5.5% growth rate in 2023, the Philippines painted a steady recovery past the global pandemic. For this year, the government expects the economy to grow between 6% and 7% amid anticipated world economic slowdown.
While the expected growth rate is lower than the 6.5%-7.5% projection made last December, the government tries to be optimistic, with the country’s economic managers setting their goal for 2025: an upper middle-income status (which is at least USD 4,256 gross national income per capita). Government officials list the following to achieve the target: a low inflation, a labor force with access to more and better jobs, a stronger fiscal standing, and a dynamic and innovative economy.
But are these enough to allow the Philippines to be on par with its more developed Asian neighbors?
In order to answer this complex question, Dr. Jesus Felipe, Distinguished Professor of the De La Salle University School of Economics and director of the Angelo King Institute of Economic and Business Studies, traces the journey to innovation and industrialization. South Korea is a good case study of a country he considers the best example in the region.
“First, we expect at the School of Economics that growth in 2024 will be about 5.6%, significantly below the government’s expectations. Growth will pick in 2025 and 2026, when we expect it will reach 6.3 and 6.0%, respectively. We think this is the most the Philippine economy can grow today. It does not have the capacity to grow faster, much less for a long time,” Felipe shares.
On the broader question of what a successful nation like South Korea did back in the 1960s, President Park Chung Hee realized that industrialization through manufacturing was his country’s ticket out of poverty. “Manufacturing is a sector with special properties. Once you learn how to make certain products within the electronics cluster, for example, you develop some capabilities that you can redeploy to make many other products. The same thing happens when you make it into the automobile or chemical sectors. You create linkages with other sectors of the economy, and learn by doing, which increases your productivity and reduces your costs. All this is much harder in traditional agriculture and in many services—think of waiters in restaurants or sales personnel in department stores,” Felipe says.
“South Korea realized that the world is very large. It was producing manufacturing products, not just for the South Korean consumers but for the world,” he points out.
A second element of industrialization is the role of exports. South Korea’s rapid export expansion also began in the 1960s and this accelerated their process of development. Exports played two fundamental roles. First, it forced companies to compete with the world economy. This means that they had to become better as time passed by. That is, they had to compete not just in price but also, and more important in the long run, in terms of product quality and sophistication. Korean cars improved in quality very quickly. Second, a developing country needs to import all those goods that are necessary for its development, in particular capital goods (machinery) that help transform the economy. These goods have to be paid in a foreign currency, typically US dollars. These have to be earned via exports.
Felipe also notes that other countries like Japan, Taiwan, Singapore, China, and Vietnam, even with differing economies, have also applied the same model. “That’s the essence of what development is about—once you start manufacturing products, you move up the ladder. You start manufacturing and exporting very simple products, then you end up manufacturing very complex cars, machines, electronics, and chemical products.”
Unlike its neighbors, the Philippines followed a completely different economic path, relegating its manufacturing sector to a small sector, in particular from the employment side. Felipe notes: “Historically, we do not know of any nation that has attained high income per capita without developing a significant manufacturing sector. Where do you think innovation comes from? Manufacturing. You may have some type of innovation in the service sector but it is, one way or another, always linked to what you do in manufacturing.”
When asked about the chances of the Philippines to catch up in the industrialization train, Felipe says, “There are always opportunities out there in terms of niches. In the manufacturing sector, it is a big aggregate that, in reality, is thousands of products. And I’m familiar with manufacturing companies in the Philippines in most of the sectors, including chemicals. So, the challenge is how to multiply that experience in the next few years, in the next couple of decades, to be able to develop some niches where we may have opportunities.”
He adds that the key is for the Philippines to have very competitive, meaning productive, firms that will both produce for the domestic market and export and compete in the world economy.
Likewise, Felipe emphasizes the vital role of the government. “It is absolutely fundamental. Without a government directing the economy in the right direction, it is going to be very difficult for the economy to progress. The government must have a clear national vision and must work with the private sector, which is composed of thousands of firms. The government must indicate the overall direction.”
The thrust on industrialization should be at the center of the country’s policy agenda, he says, adding that the work needs strong collaboration between the private and public sectors, from academe to media to the general public.
“How do we become an industrial nation? How do we strengthen the niches that are going to help us?” He notes that for the country “to achieve higher wages and higher capita income, it needs to create a wide base of domestic industrial companies that produce the myriad of basic products across the whole manufacturing spectrum that support national development. This is something that everybody needs to be aware of.”
To make various stakeholders understand the state of the Philippine economy, Dr. Jesus Felipe and the DLSU School of Economics have initiated various seminar-lectures that feature international speakers and faculty researchers tackling topics such as short- and long-run growth, inflation, fiscal consolidation, investment, and economic structure.
Contact: Dr. Jesus Felipe | [email protected]

IMAGES
COMMENTS
Research in Higher Education is a journal that publishes empirical research on postsecondary education. Open to studies using a wide range of methods, with a special interest in advanced quantitative research methods. Covers topics such as student access, retention, success, faculty issues, institutional assessment, and higher education policy. ...
Research in Higher Education publishes empirical studies that enhance our understanding of an educational institution or allow comparison among institutions. It focuses on post-secondary education, including two-year and four-year colleges, universities, and graduate and professional schools. Papers in the journal assist faculty and ...
In education, this assumption is particularly crucial since the search for the holy grail of some universal, but of course entirely illusive, instructional design drives much of the empirical investigation within the field. Two researchers working in different contexts who employ the same experimental method ought to arrive at the same conclusion.
able to think with wisdom" (p. 29). As higher education places greater emphasis on empirical data from standardized learning, research regarding why assessment is conducted, how it is leveraged for change, and the ramifications of assessment's purposes must be elevated to a more meaningful level. To this end a new
Another hint: some scholarly journals use a specific layout, called the "IMRaD" format, to communicate empirical research findings. Such articles typically have 4 components: Introduction: sometimes called "literature review" -- what is currently known about the topic -- usually includes a theoretical framework and/or discussion of previous studies
Section 3.3 discusses PBL in higher education and empirical research findings with regard to its effectiveness. Section 3.4 refers to complex models of learning opportunities and instructional quality based on social-constructivist and situated perspectives. Research on everyday classroom instruction, mainly conducted in schools with teachers ...
The Review of Higher Education is interested in empirical research studies, empirically-based historical and theoretical articles, and scholarly reviews and essays that move the study of colleges and universities forward.The most central aspect of RHE is the saliency of the subject matter to other scholars in the field as well as its usefulness to academic leaders and public policymakers.
Considering that publishing research and participating in ongoing discussions are an important part of participating in a research field (Bourdieu and Wacquant Citation 1992), an interesting finding is the number of scientific journals involved: 190 unique journals for the 247 articles situated in higher education and 48 journals for the 66 ...
higher education. This research article studies how AI impacts higher education based on previous studies and participants' experiences, views, and predictions. 2. Literature review Currently, AI has become a vital part of the virtual world. Unquestionably, AI plays an important role in general education and higher education (Edtech, 2020).
A large and growing body of research examining sustainability in higher education has emerged in the past decade. This literature is dominated by empirical and descriptive studies of specific approaches and individual initiatives, but lacks a cohesive research agenda and is not yet supported by strong theoretical underpinnings.This paper contributes to the advancement of this emerging field by ...
The results are based on an analysis of 61 articles conducting empirical studies on pair programming in higher education published between 2010 and 2020. Findings Results of studies on the effects of pair programming in comparison with solo programming are to a great extent positive.
Recent empirical evidence indicates that mobile learning can be used to support students' learning in higher education settings (Ke & Hsu, 2015; Wu, Wu, Chen, Kao, Kin & Huang, 2012). However, research in mobile learning has been fragmented and idiosyncratic and based on the understanding of the individual researcher ( Alrasheedi, Capretz ...
Simulations are increasingly often used in higher education settings. In STEM (science, technology, engineering, and mathematics) education (e.g., D'Angelo et al., 2014; Wu & Anderson, 2015), they are used to facilitate a deeper understanding of concepts and relationships between them, advance inquiry, problem solving, and decision making.A lot of research has been done in the field of ...
This article reports on a review of empirical research published in selected higher education journals in 2008, which was focused on examining how often theories are developed through research. This review found relatively little evidence of theory development.
Multidisciplinary in perspective, this bimonthly journal offers insights into theory and research that can help guide the efforts of institutions of higher education in the pursuit of inclusive excellence. Journal of Diversity in Higher Education largely publishes empirical research focused on issues related to diversity, equity, and inclusion ...
A large and growing body of research examining sustainability in higher education has emerged in the past decade. This literature is dominated by empirical and descriptive studies of specific approaches and individual initiatives, but lacks a cohesive research agenda and is not yet supported by strong theoretical underpinnings.
This study presents a hierarchical differential game between universities and scholars in order to examine innovation and research in higher education. In this stylized setup, scholars maximize the impact of their research, and universities maximize their market value. Innovations play a key role among the incentives given by the university to boost scholars' productivity, as measured by ...
In revisiting the Society for Experiential Education's eight principles of good practice, members of the most recent cohort of the SEE fellows program explored the impact of institutional context on experiential education in higher education. Previous research outlines campus and institutional structures where experiential education takes place. Our group leveraged a scholar ...
There are several reasons to review access and equity in HE research. First, most of the research on this topic to date has mainly focused on two areas: policy and quantitative data (Jia and Ericson, 2017; Leach, 2013).Second, previous research about this topic has been scattered among countries, based on disadvantaged student criteria and policy or programs that the government had to support ...
PDF | On May 1, 2020, Diane Fittipaldi published Managing the Dynamics of Group Projects in Higher Education: Best Practices Suggested by Empirical Research | Find, read and cite all the research ...
This systematic review provides unique findings with an up-to-date examination of artificial intelligence (AI) in higher education (HE) from 2016 to 2022. Using PRISMA principles and protocol, 138 articles were identified for a full examination. Using a priori, and grounded coding, the data from the 138 articles were extracted, analyzed, and coded. The findings of this study show that in 2021 ...
As an effort to further understand AI in online higher education, this systematic review examines empirical research of AI applications in online higher education from the instructional and learning perspective and investigates the functions of AI applications, algorithms used, and the effects of AI on the instruction and learning process within online higher education.
A classic example of empirical research with correlational research is when studying if high education helps in obtaining better-paying jobs. If outcomes indicate that higher education does allow individuals to have high-salaried jobs, then it follows that people with less education tend to have lower-paying jobs. ... Empirical Research and ...
The main focus of this journal is to provide a platform for original empirical investigations in the field of professional, vocational and technical education, comparing the effectiveness, efficiency and equity of different vocational education systems at the school, company and systemic level.The journal fills a gap in the existing literature focusing on empirically-oriented academic research ...
Higher education classification arose in the United States as a result of the dramatic growth in institutions and students in the 1950s and 1960s, as well as the growing federal role as sponsor of research. This paper discusses two epistemological approaches to classification: a priori and empirical and the strengths and weaknesses of both.
This article reviews the literature on student well-being (burnout and engagement) and their relationships with study demands and resources, student behaviors (proactive and self-undermining study behaviors), and student outcomes in higher education. Building on research that used Job Demands-Resources and Study Demands-Resources models to investigate student well-being, we develop the ...
Using historical analysis and empirical evidence, DLSU School of Economics Distinguished Professor and Angelo King Institute of Economic and Business Studies Director Dr. Jesus Felipe offers a fresh outlook on the development of an innovative economy and the role of the government and private sector in shaping it. With a 5.5% growth rate in 2023, the Philippines painted a steady recovery past ...