
An official website of the United States government
The .gov means it’s official. Federal government websites often end in .gov or .mil. Before sharing sensitive information, make sure you’re on a federal government site.
The site is secure. The https:// ensures that you are connecting to the official website and that any information you provide is encrypted and transmitted securely.
- Publications
- Account settings
Preview improvements coming to the PMC website in October 2024. Learn More or Try it out now .
- Advanced Search
- Journal List
- BMC Med Res Methodol


The case study approach
Sarah crowe.
1 Division of Primary Care, The University of Nottingham, Nottingham, UK
Kathrin Cresswell
2 Centre for Population Health Sciences, The University of Edinburgh, Edinburgh, UK
Ann Robertson
3 School of Health in Social Science, The University of Edinburgh, Edinburgh, UK
Anthony Avery
Aziz sheikh.
The case study approach allows in-depth, multi-faceted explorations of complex issues in their real-life settings. The value of the case study approach is well recognised in the fields of business, law and policy, but somewhat less so in health services research. Based on our experiences of conducting several health-related case studies, we reflect on the different types of case study design, the specific research questions this approach can help answer, the data sources that tend to be used, and the particular advantages and disadvantages of employing this methodological approach. The paper concludes with key pointers to aid those designing and appraising proposals for conducting case study research, and a checklist to help readers assess the quality of case study reports.
Introduction
The case study approach is particularly useful to employ when there is a need to obtain an in-depth appreciation of an issue, event or phenomenon of interest, in its natural real-life context. Our aim in writing this piece is to provide insights into when to consider employing this approach and an overview of key methodological considerations in relation to the design, planning, analysis, interpretation and reporting of case studies.
The illustrative 'grand round', 'case report' and 'case series' have a long tradition in clinical practice and research. Presenting detailed critiques, typically of one or more patients, aims to provide insights into aspects of the clinical case and, in doing so, illustrate broader lessons that may be learnt. In research, the conceptually-related case study approach can be used, for example, to describe in detail a patient's episode of care, explore professional attitudes to and experiences of a new policy initiative or service development or more generally to 'investigate contemporary phenomena within its real-life context' [ 1 ]. Based on our experiences of conducting a range of case studies, we reflect on when to consider using this approach, discuss the key steps involved and illustrate, with examples, some of the practical challenges of attaining an in-depth understanding of a 'case' as an integrated whole. In keeping with previously published work, we acknowledge the importance of theory to underpin the design, selection, conduct and interpretation of case studies[ 2 ]. In so doing, we make passing reference to the different epistemological approaches used in case study research by key theoreticians and methodologists in this field of enquiry.
This paper is structured around the following main questions: What is a case study? What are case studies used for? How are case studies conducted? What are the potential pitfalls and how can these be avoided? We draw in particular on four of our own recently published examples of case studies (see Tables Tables1, 1 , ,2, 2 , ,3 3 and and4) 4 ) and those of others to illustrate our discussion[ 3 - 7 ].
Example of a case study investigating the reasons for differences in recruitment rates of minority ethnic people in asthma research[ 3 ]
Minority ethnic people experience considerably greater morbidity from asthma than the White majority population. Research has shown however that these minority ethnic populations are likely to be under-represented in research undertaken in the UK; there is comparatively less marginalisation in the US. |
To investigate approaches to bolster recruitment of South Asians into UK asthma studies through qualitative research with US and UK researchers, and UK community leaders. |
Single intrinsic case study |
Centred on the issue of recruitment of South Asian people with asthma. |
In-depth interviews were conducted with asthma researchers from the UK and US. A supplementary questionnaire was also provided to researchers. |
Framework approach. |
Barriers to ethnic minority recruitment were found to centre around: |
1. The attitudes of the researchers' towards inclusion: The majority of UK researchers interviewed were generally supportive of the idea of recruiting ethnically diverse participants but expressed major concerns about the practicalities of achieving this; in contrast, the US researchers appeared much more committed to the policy of inclusion. |
2. Stereotypes and prejudices: We found that some of the UK researchers' perceptions of ethnic minorities may have influenced their decisions on whether to approach individuals from particular ethnic groups. These stereotypes centred on issues to do with, amongst others, language barriers and lack of altruism. |
3. Demographic, political and socioeconomic contexts of the two countries: Researchers suggested that the demographic profile of ethnic minorities, their political engagement and the different configuration of the health services in the UK and the US may have contributed to differential rates. |
4. Above all, however, it appeared that the overriding importance of the US National Institute of Health's policy to mandate the inclusion of minority ethnic people (and women) had a major impact on shaping the attitudes and in turn the experiences of US researchers'; the absence of any similar mandate in the UK meant that UK-based researchers had not been forced to challenge their existing practices and they were hence unable to overcome any stereotypical/prejudicial attitudes through experiential learning. |
Example of a case study investigating the process of planning and implementing a service in Primary Care Organisations[ 4 ]
Health work forces globally are needing to reorganise and reconfigure in order to meet the challenges posed by the increased numbers of people living with long-term conditions in an efficient and sustainable manner. Through studying the introduction of General Practitioners with a Special Interest in respiratory disorders, this study aimed to provide insights into this important issue by focusing on community respiratory service development. |
To understand and compare the process of workforce change in respiratory services and the impact on patient experience (specifically in relation to the role of general practitioners with special interests) in a theoretically selected sample of Primary Care Organisations (PCOs), in order to derive models of good practice in planning and the implementation of a broad range of workforce issues. |
Multiple-case design of respiratory services in health regions in England and Wales. |
Four PCOs. |
Face-to-face and telephone interviews, e-mail discussions, local documents, patient diaries, news items identified from local and national websites, national workshop. |
Reading, coding and comparison progressed iteratively. |
1. In the screening phase of this study (which involved semi-structured telephone interviews with the person responsible for driving the reconfiguration of respiratory services in 30 PCOs), the barriers of financial deficit, organisational uncertainty, disengaged clinicians and contradictory policies proved insurmountable for many PCOs to developing sustainable services. A key rationale for PCO re-organisation in 2006 was to strengthen their commissioning function and those of clinicians through Practice-Based Commissioning. However, the turbulence, which surrounded reorganisation was found to have the opposite desired effect. |
2. Implementing workforce reconfiguration was strongly influenced by the negotiation and contest among local clinicians and managers about "ownership" of work and income. |
3. Despite the intention to make the commissioning system more transparent, personal relationships based on common professional interests, past work history, friendships and collegiality, remained as key drivers for sustainable innovation in service development. |
It was only possible to undertake in-depth work in a selective number of PCOs and, even within these selected PCOs, it was not possible to interview all informants of potential interest and/or obtain all relevant documents. This work was conducted in the early stages of a major NHS reorganisation in England and Wales and thus, events are likely to have continued to evolve beyond the study period; we therefore cannot claim to have seen any of the stories through to their conclusion. |
Example of a case study investigating the introduction of the electronic health records[ 5 ]
Healthcare systems globally are moving from paper-based record systems to electronic health record systems. In 2002, the NHS in England embarked on the most ambitious and expensive IT-based transformation in healthcare in history seeking to introduce electronic health records into all hospitals in England by 2010. |
To describe and evaluate the implementation and adoption of detailed electronic health records in secondary care in England and thereby provide formative feedback for local and national rollout of the NHS Care Records Service. |
A mixed methods, longitudinal, multi-site, socio-technical collective case study. |
Five NHS acute hospital and mental health Trusts that have been the focus of early implementation efforts. |
Semi-structured interviews, documentary data and field notes, observations and quantitative data. |
Qualitative data were analysed thematically using a socio-technical coding matrix, combined with additional themes that emerged from the data. |
1. Hospital electronic health record systems have developed and been implemented far more slowly than was originally envisioned. |
2. The top-down, government-led standardised approach needed to evolve to admit more variation and greater local choice for hospitals in order to support local service delivery. |
3. A range of adverse consequences were associated with the centrally negotiated contracts, which excluded the hospitals in question. |
4. The unrealistic, politically driven, timeline (implementation over 10 years) was found to be a major source of frustration for developers, implementers and healthcare managers and professionals alike. |
We were unable to access details of the contracts between government departments and the Local Service Providers responsible for delivering and implementing the software systems. This, in turn, made it difficult to develop a holistic understanding of some key issues impacting on the overall slow roll-out of the NHS Care Record Service. Early adopters may also have differed in important ways from NHS hospitals that planned to join the National Programme for Information Technology and implement the NHS Care Records Service at a later point in time. |
Example of a case study investigating the formal and informal ways students learn about patient safety[ 6 ]
There is a need to reduce the disease burden associated with iatrogenic harm and considering that healthcare education represents perhaps the most sustained patient safety initiative ever undertaken, it is important to develop a better appreciation of the ways in which undergraduate and newly qualified professionals receive and make sense of the education they receive. | |
---|---|
To investigate the formal and informal ways pre-registration students from a range of healthcare professions (medicine, nursing, physiotherapy and pharmacy) learn about patient safety in order to become safe practitioners. | |
Multi-site, mixed method collective case study. | |
: Eight case studies (two for each professional group) were carried out in educational provider sites considering different programmes, practice environments and models of teaching and learning. | |
Structured in phases relevant to the three knowledge contexts: | |
Documentary evidence (including undergraduate curricula, handbooks and module outlines), complemented with a range of views (from course leads, tutors and students) and observations in a range of academic settings. | |
Policy and management views of patient safety and influences on patient safety education and practice. NHS policies included, for example, implementation of the National Patient Safety Agency's , which encourages organisations to develop an organisational safety culture in which staff members feel comfortable identifying dangers and reporting hazards. | |
The cultures to which students are exposed i.e. patient safety in relation to day-to-day working. NHS initiatives included, for example, a hand washing initiative or introduction of infection control measures. | |
1. Practical, informal, learning opportunities were valued by students. On the whole, however, students were not exposed to nor engaged with important NHS initiatives such as risk management activities and incident reporting schemes. | |
2. NHS policy appeared to have been taken seriously by course leaders. Patient safety materials were incorporated into both formal and informal curricula, albeit largely implicit rather than explicit. | |
3. Resource issues and peer pressure were found to influence safe practice. Variations were also found to exist in students' experiences and the quality of the supervision available. | |
The curriculum and organisational documents collected differed between sites, which possibly reflected gatekeeper influences at each site. The recruitment of participants for focus group discussions proved difficult, so interviews or paired discussions were used as a substitute. |
What is a case study?
A case study is a research approach that is used to generate an in-depth, multi-faceted understanding of a complex issue in its real-life context. It is an established research design that is used extensively in a wide variety of disciplines, particularly in the social sciences. A case study can be defined in a variety of ways (Table (Table5), 5 ), the central tenet being the need to explore an event or phenomenon in depth and in its natural context. It is for this reason sometimes referred to as a "naturalistic" design; this is in contrast to an "experimental" design (such as a randomised controlled trial) in which the investigator seeks to exert control over and manipulate the variable(s) of interest.
Definitions of a case study
Author | Definition |
---|---|
Stake[ ] | (p.237) |
Yin[ , , ] | (Yin 1999 p. 1211, Yin 1994 p. 13) |
• | |
• (Yin 2009 p18) | |
Miles and Huberman[ ] | (p. 25) |
Green and Thorogood[ ] | (p. 284) |
George and Bennett[ ] | (p. 17)" |
Stake's work has been particularly influential in defining the case study approach to scientific enquiry. He has helpfully characterised three main types of case study: intrinsic , instrumental and collective [ 8 ]. An intrinsic case study is typically undertaken to learn about a unique phenomenon. The researcher should define the uniqueness of the phenomenon, which distinguishes it from all others. In contrast, the instrumental case study uses a particular case (some of which may be better than others) to gain a broader appreciation of an issue or phenomenon. The collective case study involves studying multiple cases simultaneously or sequentially in an attempt to generate a still broader appreciation of a particular issue.
These are however not necessarily mutually exclusive categories. In the first of our examples (Table (Table1), 1 ), we undertook an intrinsic case study to investigate the issue of recruitment of minority ethnic people into the specific context of asthma research studies, but it developed into a instrumental case study through seeking to understand the issue of recruitment of these marginalised populations more generally, generating a number of the findings that are potentially transferable to other disease contexts[ 3 ]. In contrast, the other three examples (see Tables Tables2, 2 , ,3 3 and and4) 4 ) employed collective case study designs to study the introduction of workforce reconfiguration in primary care, the implementation of electronic health records into hospitals, and to understand the ways in which healthcare students learn about patient safety considerations[ 4 - 6 ]. Although our study focusing on the introduction of General Practitioners with Specialist Interests (Table (Table2) 2 ) was explicitly collective in design (four contrasting primary care organisations were studied), is was also instrumental in that this particular professional group was studied as an exemplar of the more general phenomenon of workforce redesign[ 4 ].
What are case studies used for?
According to Yin, case studies can be used to explain, describe or explore events or phenomena in the everyday contexts in which they occur[ 1 ]. These can, for example, help to understand and explain causal links and pathways resulting from a new policy initiative or service development (see Tables Tables2 2 and and3, 3 , for example)[ 1 ]. In contrast to experimental designs, which seek to test a specific hypothesis through deliberately manipulating the environment (like, for example, in a randomised controlled trial giving a new drug to randomly selected individuals and then comparing outcomes with controls),[ 9 ] the case study approach lends itself well to capturing information on more explanatory ' how ', 'what' and ' why ' questions, such as ' how is the intervention being implemented and received on the ground?'. The case study approach can offer additional insights into what gaps exist in its delivery or why one implementation strategy might be chosen over another. This in turn can help develop or refine theory, as shown in our study of the teaching of patient safety in undergraduate curricula (Table (Table4 4 )[ 6 , 10 ]. Key questions to consider when selecting the most appropriate study design are whether it is desirable or indeed possible to undertake a formal experimental investigation in which individuals and/or organisations are allocated to an intervention or control arm? Or whether the wish is to obtain a more naturalistic understanding of an issue? The former is ideally studied using a controlled experimental design, whereas the latter is more appropriately studied using a case study design.
Case studies may be approached in different ways depending on the epistemological standpoint of the researcher, that is, whether they take a critical (questioning one's own and others' assumptions), interpretivist (trying to understand individual and shared social meanings) or positivist approach (orientating towards the criteria of natural sciences, such as focusing on generalisability considerations) (Table (Table6). 6 ). Whilst such a schema can be conceptually helpful, it may be appropriate to draw on more than one approach in any case study, particularly in the context of conducting health services research. Doolin has, for example, noted that in the context of undertaking interpretative case studies, researchers can usefully draw on a critical, reflective perspective which seeks to take into account the wider social and political environment that has shaped the case[ 11 ].
Example of epistemological approaches that may be used in case study research
Approach | Characteristics | Criticisms | Key references |
---|---|---|---|
Involves questioning one's own assumptions taking into account the wider political and social environment. | It can possibly neglect other factors by focussing only on power relationships and may give the researcher a position that is too privileged. | Howcroft and Trauth[ ] Blakie[ ] Doolin[ , ] | |
Interprets the limiting conditions in relation to power and control that are thought to influence behaviour. | Bloomfield and Best[ ] | ||
Involves understanding meanings/contexts and processes as perceived from different perspectives, trying to understand individual and shared social meanings. Focus is on theory building. | Often difficult to explain unintended consequences and for neglecting surrounding historical contexts | Stake[ ] Doolin[ ] | |
Involves establishing which variables one wishes to study in advance and seeing whether they fit in with the findings. Focus is often on testing and refining theory on the basis of case study findings. | It does not take into account the role of the researcher in influencing findings. | Yin[ , , ] Shanks and Parr[ ] |
How are case studies conducted?
Here, we focus on the main stages of research activity when planning and undertaking a case study; the crucial stages are: defining the case; selecting the case(s); collecting and analysing the data; interpreting data; and reporting the findings.
Defining the case
Carefully formulated research question(s), informed by the existing literature and a prior appreciation of the theoretical issues and setting(s), are all important in appropriately and succinctly defining the case[ 8 , 12 ]. Crucially, each case should have a pre-defined boundary which clarifies the nature and time period covered by the case study (i.e. its scope, beginning and end), the relevant social group, organisation or geographical area of interest to the investigator, the types of evidence to be collected, and the priorities for data collection and analysis (see Table Table7 7 )[ 1 ]. A theory driven approach to defining the case may help generate knowledge that is potentially transferable to a range of clinical contexts and behaviours; using theory is also likely to result in a more informed appreciation of, for example, how and why interventions have succeeded or failed[ 13 ].
Example of a checklist for rating a case study proposal[ 8 ]
Clarity: Does the proposal read well? | |
Integrity: Do its pieces fit together? | |
Attractiveness: Does it pique the reader's interest? | |
The case: Is the case adequately defined? | |
The issues: Are major research questions identified? | |
Data Resource: Are sufficient data sources identified? | |
Case Selection: Is the selection plan reasonable? | |
Data Gathering: Are data-gathering activities outlined? | |
Validation: Is the need and opportunity for triangulation indicated? | |
Access: Are arrangements for start-up anticipated? | |
Confidentiality: Is there sensitivity to the protection of people? | |
Cost: Are time and resource estimates reasonable? |
For example, in our evaluation of the introduction of electronic health records in English hospitals (Table (Table3), 3 ), we defined our cases as the NHS Trusts that were receiving the new technology[ 5 ]. Our focus was on how the technology was being implemented. However, if the primary research interest had been on the social and organisational dimensions of implementation, we might have defined our case differently as a grouping of healthcare professionals (e.g. doctors and/or nurses). The precise beginning and end of the case may however prove difficult to define. Pursuing this same example, when does the process of implementation and adoption of an electronic health record system really begin or end? Such judgements will inevitably be influenced by a range of factors, including the research question, theory of interest, the scope and richness of the gathered data and the resources available to the research team.
Selecting the case(s)
The decision on how to select the case(s) to study is a very important one that merits some reflection. In an intrinsic case study, the case is selected on its own merits[ 8 ]. The case is selected not because it is representative of other cases, but because of its uniqueness, which is of genuine interest to the researchers. This was, for example, the case in our study of the recruitment of minority ethnic participants into asthma research (Table (Table1) 1 ) as our earlier work had demonstrated the marginalisation of minority ethnic people with asthma, despite evidence of disproportionate asthma morbidity[ 14 , 15 ]. In another example of an intrinsic case study, Hellstrom et al.[ 16 ] studied an elderly married couple living with dementia to explore how dementia had impacted on their understanding of home, their everyday life and their relationships.
For an instrumental case study, selecting a "typical" case can work well[ 8 ]. In contrast to the intrinsic case study, the particular case which is chosen is of less importance than selecting a case that allows the researcher to investigate an issue or phenomenon. For example, in order to gain an understanding of doctors' responses to health policy initiatives, Som undertook an instrumental case study interviewing clinicians who had a range of responsibilities for clinical governance in one NHS acute hospital trust[ 17 ]. Sampling a "deviant" or "atypical" case may however prove even more informative, potentially enabling the researcher to identify causal processes, generate hypotheses and develop theory.
In collective or multiple case studies, a number of cases are carefully selected. This offers the advantage of allowing comparisons to be made across several cases and/or replication. Choosing a "typical" case may enable the findings to be generalised to theory (i.e. analytical generalisation) or to test theory by replicating the findings in a second or even a third case (i.e. replication logic)[ 1 ]. Yin suggests two or three literal replications (i.e. predicting similar results) if the theory is straightforward and five or more if the theory is more subtle. However, critics might argue that selecting 'cases' in this way is insufficiently reflexive and ill-suited to the complexities of contemporary healthcare organisations.
The selected case study site(s) should allow the research team access to the group of individuals, the organisation, the processes or whatever else constitutes the chosen unit of analysis for the study. Access is therefore a central consideration; the researcher needs to come to know the case study site(s) well and to work cooperatively with them. Selected cases need to be not only interesting but also hospitable to the inquiry [ 8 ] if they are to be informative and answer the research question(s). Case study sites may also be pre-selected for the researcher, with decisions being influenced by key stakeholders. For example, our selection of case study sites in the evaluation of the implementation and adoption of electronic health record systems (see Table Table3) 3 ) was heavily influenced by NHS Connecting for Health, the government agency that was responsible for overseeing the National Programme for Information Technology (NPfIT)[ 5 ]. This prominent stakeholder had already selected the NHS sites (through a competitive bidding process) to be early adopters of the electronic health record systems and had negotiated contracts that detailed the deployment timelines.
It is also important to consider in advance the likely burden and risks associated with participation for those who (or the site(s) which) comprise the case study. Of particular importance is the obligation for the researcher to think through the ethical implications of the study (e.g. the risk of inadvertently breaching anonymity or confidentiality) and to ensure that potential participants/participating sites are provided with sufficient information to make an informed choice about joining the study. The outcome of providing this information might be that the emotive burden associated with participation, or the organisational disruption associated with supporting the fieldwork, is considered so high that the individuals or sites decide against participation.
In our example of evaluating implementations of electronic health record systems, given the restricted number of early adopter sites available to us, we sought purposively to select a diverse range of implementation cases among those that were available[ 5 ]. We chose a mixture of teaching, non-teaching and Foundation Trust hospitals, and examples of each of the three electronic health record systems procured centrally by the NPfIT. At one recruited site, it quickly became apparent that access was problematic because of competing demands on that organisation. Recognising the importance of full access and co-operative working for generating rich data, the research team decided not to pursue work at that site and instead to focus on other recruited sites.
Collecting the data
In order to develop a thorough understanding of the case, the case study approach usually involves the collection of multiple sources of evidence, using a range of quantitative (e.g. questionnaires, audits and analysis of routinely collected healthcare data) and more commonly qualitative techniques (e.g. interviews, focus groups and observations). The use of multiple sources of data (data triangulation) has been advocated as a way of increasing the internal validity of a study (i.e. the extent to which the method is appropriate to answer the research question)[ 8 , 18 - 21 ]. An underlying assumption is that data collected in different ways should lead to similar conclusions, and approaching the same issue from different angles can help develop a holistic picture of the phenomenon (Table (Table2 2 )[ 4 ].
Brazier and colleagues used a mixed-methods case study approach to investigate the impact of a cancer care programme[ 22 ]. Here, quantitative measures were collected with questionnaires before, and five months after, the start of the intervention which did not yield any statistically significant results. Qualitative interviews with patients however helped provide an insight into potentially beneficial process-related aspects of the programme, such as greater, perceived patient involvement in care. The authors reported how this case study approach provided a number of contextual factors likely to influence the effectiveness of the intervention and which were not likely to have been obtained from quantitative methods alone.
In collective or multiple case studies, data collection needs to be flexible enough to allow a detailed description of each individual case to be developed (e.g. the nature of different cancer care programmes), before considering the emerging similarities and differences in cross-case comparisons (e.g. to explore why one programme is more effective than another). It is important that data sources from different cases are, where possible, broadly comparable for this purpose even though they may vary in nature and depth.
Analysing, interpreting and reporting case studies
Making sense and offering a coherent interpretation of the typically disparate sources of data (whether qualitative alone or together with quantitative) is far from straightforward. Repeated reviewing and sorting of the voluminous and detail-rich data are integral to the process of analysis. In collective case studies, it is helpful to analyse data relating to the individual component cases first, before making comparisons across cases. Attention needs to be paid to variations within each case and, where relevant, the relationship between different causes, effects and outcomes[ 23 ]. Data will need to be organised and coded to allow the key issues, both derived from the literature and emerging from the dataset, to be easily retrieved at a later stage. An initial coding frame can help capture these issues and can be applied systematically to the whole dataset with the aid of a qualitative data analysis software package.
The Framework approach is a practical approach, comprising of five stages (familiarisation; identifying a thematic framework; indexing; charting; mapping and interpretation) , to managing and analysing large datasets particularly if time is limited, as was the case in our study of recruitment of South Asians into asthma research (Table (Table1 1 )[ 3 , 24 ]. Theoretical frameworks may also play an important role in integrating different sources of data and examining emerging themes. For example, we drew on a socio-technical framework to help explain the connections between different elements - technology; people; and the organisational settings within which they worked - in our study of the introduction of electronic health record systems (Table (Table3 3 )[ 5 ]. Our study of patient safety in undergraduate curricula drew on an evaluation-based approach to design and analysis, which emphasised the importance of the academic, organisational and practice contexts through which students learn (Table (Table4 4 )[ 6 ].
Case study findings can have implications both for theory development and theory testing. They may establish, strengthen or weaken historical explanations of a case and, in certain circumstances, allow theoretical (as opposed to statistical) generalisation beyond the particular cases studied[ 12 ]. These theoretical lenses should not, however, constitute a strait-jacket and the cases should not be "forced to fit" the particular theoretical framework that is being employed.
When reporting findings, it is important to provide the reader with enough contextual information to understand the processes that were followed and how the conclusions were reached. In a collective case study, researchers may choose to present the findings from individual cases separately before amalgamating across cases. Care must be taken to ensure the anonymity of both case sites and individual participants (if agreed in advance) by allocating appropriate codes or withholding descriptors. In the example given in Table Table3, 3 , we decided against providing detailed information on the NHS sites and individual participants in order to avoid the risk of inadvertent disclosure of identities[ 5 , 25 ].
What are the potential pitfalls and how can these be avoided?
The case study approach is, as with all research, not without its limitations. When investigating the formal and informal ways undergraduate students learn about patient safety (Table (Table4), 4 ), for example, we rapidly accumulated a large quantity of data. The volume of data, together with the time restrictions in place, impacted on the depth of analysis that was possible within the available resources. This highlights a more general point of the importance of avoiding the temptation to collect as much data as possible; adequate time also needs to be set aside for data analysis and interpretation of what are often highly complex datasets.
Case study research has sometimes been criticised for lacking scientific rigour and providing little basis for generalisation (i.e. producing findings that may be transferable to other settings)[ 1 ]. There are several ways to address these concerns, including: the use of theoretical sampling (i.e. drawing on a particular conceptual framework); respondent validation (i.e. participants checking emerging findings and the researcher's interpretation, and providing an opinion as to whether they feel these are accurate); and transparency throughout the research process (see Table Table8 8 )[ 8 , 18 - 21 , 23 , 26 ]. Transparency can be achieved by describing in detail the steps involved in case selection, data collection, the reasons for the particular methods chosen, and the researcher's background and level of involvement (i.e. being explicit about how the researcher has influenced data collection and interpretation). Seeking potential, alternative explanations, and being explicit about how interpretations and conclusions were reached, help readers to judge the trustworthiness of the case study report. Stake provides a critique checklist for a case study report (Table (Table9 9 )[ 8 ].
Potential pitfalls and mitigating actions when undertaking case study research
Potential pitfall | Mitigating action |
---|---|
Selecting/conceptualising the wrong case(s) resulting in lack of theoretical generalisations | Developing in-depth knowledge of theoretical and empirical literature, justifying choices made |
Collecting large volumes of data that are not relevant to the case or too little to be of any value | Focus data collection in line with research questions, whilst being flexible and allowing different paths to be explored |
Defining/bounding the case | Focus on related components (either by time and/or space), be clear what is outside the scope of the case |
Lack of rigour | Triangulation, respondent validation, the use of theoretical sampling, transparency throughout the research process |
Ethical issues | Anonymise appropriately as cases are often easily identifiable to insiders, informed consent of participants |
Integration with theoretical framework | Allow for unexpected issues to emerge and do not force fit, test out preliminary explanations, be clear about epistemological positions in advance |
Stake's checklist for assessing the quality of a case study report[ 8 ]
1. Is this report easy to read? |
2. Does it fit together, each sentence contributing to the whole? |
3. Does this report have a conceptual structure (i.e. themes or issues)? |
4. Are its issues developed in a series and scholarly way? |
5. Is the case adequately defined? |
6. Is there a sense of story to the presentation? |
7. Is the reader provided some vicarious experience? |
8. Have quotations been used effectively? |
9. Are headings, figures, artefacts, appendices, indexes effectively used? |
10. Was it edited well, then again with a last minute polish? |
11. Has the writer made sound assertions, neither over- or under-interpreting? |
12. Has adequate attention been paid to various contexts? |
13. Were sufficient raw data presented? |
14. Were data sources well chosen and in sufficient number? |
15. Do observations and interpretations appear to have been triangulated? |
16. Is the role and point of view of the researcher nicely apparent? |
17. Is the nature of the intended audience apparent? |
18. Is empathy shown for all sides? |
19. Are personal intentions examined? |
20. Does it appear individuals were put at risk? |
Conclusions
The case study approach allows, amongst other things, critical events, interventions, policy developments and programme-based service reforms to be studied in detail in a real-life context. It should therefore be considered when an experimental design is either inappropriate to answer the research questions posed or impossible to undertake. Considering the frequency with which implementations of innovations are now taking place in healthcare settings and how well the case study approach lends itself to in-depth, complex health service research, we believe this approach should be more widely considered by researchers. Though inherently challenging, the research case study can, if carefully conceptualised and thoughtfully undertaken and reported, yield powerful insights into many important aspects of health and healthcare delivery.
Competing interests
The authors declare that they have no competing interests.
Authors' contributions
AS conceived this article. SC, KC and AR wrote this paper with GH, AA and AS all commenting on various drafts. SC and AS are guarantors.
Pre-publication history
The pre-publication history for this paper can be accessed here:
http://www.biomedcentral.com/1471-2288/11/100/prepub
Acknowledgements
We are grateful to the participants and colleagues who contributed to the individual case studies that we have drawn on. This work received no direct funding, but it has been informed by projects funded by Asthma UK, the NHS Service Delivery Organisation, NHS Connecting for Health Evaluation Programme, and Patient Safety Research Portfolio. We would also like to thank the expert reviewers for their insightful and constructive feedback. Our thanks are also due to Dr. Allison Worth who commented on an earlier draft of this manuscript.
- Yin RK. Case study research, design and method. 4. London: Sage Publications Ltd.; 2009. [ Google Scholar ]
- Keen J, Packwood T. Qualitative research; case study evaluation. BMJ. 1995; 311 :444–446. [ PMC free article ] [ PubMed ] [ Google Scholar ]
- Sheikh A, Halani L, Bhopal R, Netuveli G, Partridge M, Car J. et al. Facilitating the Recruitment of Minority Ethnic People into Research: Qualitative Case Study of South Asians and Asthma. PLoS Med. 2009; 6 (10):1–11. [ PMC free article ] [ PubMed ] [ Google Scholar ]
- Pinnock H, Huby G, Powell A, Kielmann T, Price D, Williams S, The process of planning, development and implementation of a General Practitioner with a Special Interest service in Primary Care Organisations in England and Wales: a comparative prospective case study. Report for the National Co-ordinating Centre for NHS Service Delivery and Organisation R&D (NCCSDO) 2008. http://www.sdo.nihr.ac.uk/files/project/99-final-report.pdf
- Robertson A, Cresswell K, Takian A, Petrakaki D, Crowe S, Cornford T. et al. Prospective evaluation of the implementation and adoption of NHS Connecting for Health's national electronic health record in secondary care in England: interim findings. BMJ. 2010; 41 :c4564. [ PMC free article ] [ PubMed ] [ Google Scholar ]
- Pearson P, Steven A, Howe A, Sheikh A, Ashcroft D, Smith P. the Patient Safety Education Study Group. Learning about patient safety: organisational context and culture in the education of healthcare professionals. J Health Serv Res Policy. 2010; 15 :4–10. doi: 10.1258/jhsrp.2009.009052. [ PubMed ] [ CrossRef ] [ Google Scholar ]
- van Harten WH, Casparie TF, Fisscher OA. The evaluation of the introduction of a quality management system: a process-oriented case study in a large rehabilitation hospital. Health Policy. 2002; 60 (1):17–37. doi: 10.1016/S0168-8510(01)00187-7. [ PubMed ] [ CrossRef ] [ Google Scholar ]
- Stake RE. The art of case study research. London: Sage Publications Ltd.; 1995. [ Google Scholar ]
- Sheikh A, Smeeth L, Ashcroft R. Randomised controlled trials in primary care: scope and application. Br J Gen Pract. 2002; 52 (482):746–51. [ PMC free article ] [ PubMed ] [ Google Scholar ]
- King G, Keohane R, Verba S. Designing Social Inquiry. Princeton: Princeton University Press; 1996. [ Google Scholar ]
- Doolin B. Information technology as disciplinary technology: being critical in interpretative research on information systems. Journal of Information Technology. 1998; 13 :301–311. doi: 10.1057/jit.1998.8. [ CrossRef ] [ Google Scholar ]
- George AL, Bennett A. Case studies and theory development in the social sciences. Cambridge, MA: MIT Press; 2005. [ Google Scholar ]
- Eccles M. the Improved Clinical Effectiveness through Behavioural Research Group (ICEBeRG) Designing theoretically-informed implementation interventions. Implementation Science. 2006; 1 :1–8. doi: 10.1186/1748-5908-1-1. [ PMC free article ] [ PubMed ] [ CrossRef ] [ Google Scholar ]
- Netuveli G, Hurwitz B, Levy M, Fletcher M, Barnes G, Durham SR, Sheikh A. Ethnic variations in UK asthma frequency, morbidity, and health-service use: a systematic review and meta-analysis. Lancet. 2005; 365 (9456):312–7. [ PubMed ] [ Google Scholar ]
- Sheikh A, Panesar SS, Lasserson T, Netuveli G. Recruitment of ethnic minorities to asthma studies. Thorax. 2004; 59 (7):634. [ PMC free article ] [ PubMed ] [ Google Scholar ]
- Hellström I, Nolan M, Lundh U. 'We do things together': A case study of 'couplehood' in dementia. Dementia. 2005; 4 :7–22. doi: 10.1177/1471301205049188. [ CrossRef ] [ Google Scholar ]
- Som CV. Nothing seems to have changed, nothing seems to be changing and perhaps nothing will change in the NHS: doctors' response to clinical governance. International Journal of Public Sector Management. 2005; 18 :463–477. doi: 10.1108/09513550510608903. [ CrossRef ] [ Google Scholar ]
- Lincoln Y, Guba E. Naturalistic inquiry. Newbury Park: Sage Publications; 1985. [ Google Scholar ]
- Barbour RS. Checklists for improving rigour in qualitative research: a case of the tail wagging the dog? BMJ. 2001; 322 :1115–1117. doi: 10.1136/bmj.322.7294.1115. [ PMC free article ] [ PubMed ] [ CrossRef ] [ Google Scholar ]
- Mays N, Pope C. Qualitative research in health care: Assessing quality in qualitative research. BMJ. 2000; 320 :50–52. doi: 10.1136/bmj.320.7226.50. [ PMC free article ] [ PubMed ] [ CrossRef ] [ Google Scholar ]
- Mason J. Qualitative researching. London: Sage; 2002. [ Google Scholar ]
- Brazier A, Cooke K, Moravan V. Using Mixed Methods for Evaluating an Integrative Approach to Cancer Care: A Case Study. Integr Cancer Ther. 2008; 7 :5–17. doi: 10.1177/1534735407313395. [ PubMed ] [ CrossRef ] [ Google Scholar ]
- Miles MB, Huberman M. Qualitative data analysis: an expanded sourcebook. 2. CA: Sage Publications Inc.; 1994. [ Google Scholar ]
- Pope C, Ziebland S, Mays N. Analysing qualitative data. Qualitative research in health care. BMJ. 2000; 320 :114–116. doi: 10.1136/bmj.320.7227.114. [ PMC free article ] [ PubMed ] [ CrossRef ] [ Google Scholar ]
- Cresswell KM, Worth A, Sheikh A. Actor-Network Theory and its role in understanding the implementation of information technology developments in healthcare. BMC Med Inform Decis Mak. 2010; 10 (1):67. doi: 10.1186/1472-6947-10-67. [ PMC free article ] [ PubMed ] [ CrossRef ] [ Google Scholar ]
- Malterud K. Qualitative research: standards, challenges, and guidelines. Lancet. 2001; 358 :483–488. doi: 10.1016/S0140-6736(01)05627-6. [ PubMed ] [ CrossRef ] [ Google Scholar ]
- Yin R. Case study research: design and methods. 2. Thousand Oaks, CA: Sage Publishing; 1994. [ Google Scholar ]
- Yin R. Enhancing the quality of case studies in health services research. Health Serv Res. 1999; 34 :1209–1224. [ PMC free article ] [ PubMed ] [ Google Scholar ]
- Green J, Thorogood N. Qualitative methods for health research. 2. Los Angeles: Sage; 2009. [ Google Scholar ]
- Howcroft D, Trauth E. Handbook of Critical Information Systems Research, Theory and Application. Cheltenham, UK: Northampton, MA, USA: Edward Elgar; 2005. [ Google Scholar ]
- Blakie N. Approaches to Social Enquiry. Cambridge: Polity Press; 1993. [ Google Scholar ]
- Doolin B. Power and resistance in the implementation of a medical management information system. Info Systems J. 2004; 14 :343–362. doi: 10.1111/j.1365-2575.2004.00176.x. [ CrossRef ] [ Google Scholar ]
- Bloomfield BP, Best A. Management consultants: systems development, power and the translation of problems. Sociological Review. 1992; 40 :533–560. [ Google Scholar ]
- Shanks G, Parr A. Proceedings of the European Conference on Information Systems. Naples; 2003. Positivist, single case study research in information systems: A critical analysis. [ Google Scholar ]

- SUGGESTED TOPICS
- The Magazine
- Newsletters
- Managing Yourself
- Managing Teams
- Work-life Balance
- The Big Idea
- Data & Visuals
- Reading Lists
- Case Selections
- HBR Learning
- Topic Feeds
- Account Settings
- Email Preferences
What the Case Study Method Really Teaches
- Nitin Nohria

Seven meta-skills that stick even if the cases fade from memory.
It’s been 100 years since Harvard Business School began using the case study method. Beyond teaching specific subject matter, the case study method excels in instilling meta-skills in students. This article explains the importance of seven such skills: preparation, discernment, bias recognition, judgement, collaboration, curiosity, and self-confidence.
During my decade as dean of Harvard Business School, I spent hundreds of hours talking with our alumni. To enliven these conversations, I relied on a favorite question: “What was the most important thing you learned from your time in our MBA program?”
- Nitin Nohria is the George F. Baker Jr. and Distinguished Service University Professor. He served as the 10th dean of Harvard Business School, from 2010 to 2020.
Partner Center
Hypothesis-Driven Approach: Crack Your Case Like a Consultant
- Last Updated June, 2023
A hypothesis-driven approach in consulting is a structured method of problem-solving. Consultants formulate a hypothesis for the solution to a business problem, then gather data to support or disprove it.
Cracking a case interview can be a daunting task, with a wide range of potential solutions and approaches to consider. However, using a hypothesis-driven approach is a systematic and effective problem-solving method. It will impress your interviewer and demonstrate your readiness for a career in consulting.
In this article, we will talk about:
- The definitions of a hypothesis and a hypothesis-driven approach
- The differences between a hypothesis-driven approach and a non-hypothesis-driven approach
- An example of how to solve a case using both approaches
- Our 5-step process for using a hypothesis-driven approach to solve consulting cases
Let’s get started!
What Is a Hypothesis & a Hypothesis-Driven Approach?
Differences between a hypothesis-driven approach vs. non-hypothesis-driven approach, our 5-step process for using the hypothesis-driven mindset to solve cases, other consulting tools that will strengthen your problem-solving.
In the realm of science, the term “hypothesis” is used to describe a proposed explanation for a question or phenomenon, based on limited evidence, as a starting point for further investigation. Similarly, consultants act as scientists or as doctors solving their clients’ business problems, constantly forming and testing hypotheses to identify the best solutions.
The key phrase here is “starting point,” as a hypothesis is an educated guess at the solution, formed from currently available information. As more data is gathered, the hypothesis may be adjusted or even discarded entirely.
Nail the case & fit interview with strategies from former MBB Interviewers that have helped 89.6% of our clients pass the case interview.
Hypothesis-Driven Approach
Consultants are engaged to efficiently and effectively solve their clients’ problems and assist in making critical business decisions. With the vast amount of data available and an array of options to consider, it can be overwhelming to examine everything. Time constraints on projects make it imperative that consultants avoid getting bogged down in excessive analysis and questioning, without making meaningful progress toward a recommendation.
Instead, consultants begin by forming a hypothesis after gaining an understanding of the client’s problem and high-level range of possibilities. Then, they gather data to test the initial hypothesis. If the data disproves the hypothesis, the consultants repeat the process with the next best hypothesis. This method of problem-solving is commonly used by top consulting firms, such as McKinsey.
A non-hypothesis-driven approach is the opposite of a hypothesis-driven approach. Instead of forming a hypothesis, the individual makes a recommendation only after thoroughly evaluating all data and possibilities. This approach may rely on intuition, trial and error, or exhaustively exploring all options to solve the problem. This is not an efficient method for a case interview, where time is limited.
An analogy that illustrates the distinction between the two methods is to look at problem-solving as trying to find a needle in a haystack. A non-hypothesis-driven approach would involve randomly searching through the entire stack without any clear strategy.
On the other hand, a hypothesis-driven approach would involve dividing the haystack into smaller piles, and systematically searching through one section at a time. The searcher would gather information from the person who lost the needle, such as their location when it was lost, to identify the most likely pile to search first. This not only saves time but also increases the likelihood of finding the needle. If the needle is not found in the initial pile, the search can then move on to the next most probable pile.
Solving a Case Interview Using the Hypothesis-Driven Approach vs. the Non-Hypothesis-Driven Approach
To further illustrate the advantages of a hypothesis-driven approach, let’s examine two different approaches to the same case interview example. We’ll compare and contrast these approaches, highlighting the key distinctions between them. By the end, you’ll have a clear understanding of the benefits of using a hypothesis-driven approach in problem-solving.
The client is SnackCo, a consumer goods company that manufactures and sells trail mixes in the United States. Over the past decade, SnackCo has seen significant growth following the launch of premium trail mix products, capitalizing on the trend toward healthier snacking options. Despite this success, the company’s operations have remained unchanged for the past decade. SnackCo is asking for your help to improve its bottom line.
Let’s look at how two candidates, Alex and Julie, solve the same case.
The Non-Hypothesis-Driven Approach
After hearing this prompt, Alex jumps right into listing possible questions related to how to improve the bottom line.
Alex: I understand SnackCo wants to improve profitability. Here are some questions I want to look into. Has SnackCo’s retail prices remained the same in recent years?
Interviewer: No, SnackCo has adjusted prices quite closely to what competitive products are selling at.
Alex: Oh interesting. Are consumers willing to pay more for premium trail mix? Do we know if we are underpricing?
Interviewer: SnackCo’s Director of Sales strongly believes that they should not change product prices. He believes the consumers love the product and it is priced fairly.
Alex: Got it. Has the client’s market share decreased?
Interviewer: No, the market share has increased over the years.
Alex: In that case, it seems like our growth is fine. Have the costs increased?
Interviewer: SnackCo has not made many changes to its costs and operations in the last decade. What are some ways we can help them look at their cost savings opportunities?
Although Alex is making progress and may eventually solve the case, his communication style gives the impression that he is randomly guessing at the sources of the problem, rather than using logical reasoning and structure to pinpoint the solution.
The Hypothesis-Driven Approach
Julie has prepared for her case interviews with My Consulting Offer’s coaches so she is well-versed in the hypothesis-driven approach.
After hearing the same prompt, she takes a moment to write down the key issues she wants to dig into to solve this case and organizes her thoughts.
Julie: For the goal of improving profitability, we could look at how to improve revenue or decrease costs. For revenue, we could look at if prices or volumes have changed. Since the client said they haven’t made any changes to the business operations in the last decade, I would like to start with a better understanding of their costs. However, before we begin, I want to confirm if there have been any changes to prices or volumes recently.
Interviewer: SnackCo’s Director of Sales strongly believes that they should not change product prices. They also believe the volumes have grown well as SnackCo is one of the market leaders now.
Julie: Great. That confirms what I was thinking. It’s likely a cost problem. We could look at their variable costs, such as ingredients, or fixed costs, such as manufacturing facilities. Given that this is an established business, I would assume their fixed costs are likely consistent. Therefore, let’s start with their variable costs.
Interviewer: How should we think about variable costs?
Julie: Variable costs for SnackCo likely include ingredients, packaging, and freight. The levers they could pull to reduce these costs would be through supplier relationships or changing the product composition.
Julie quickly identifies that variable costs are likely the problem and has a structured approach to understanding which opportunities to explore.
Key Differences
The interviewer is looking for candidates with strong problem-solving and communication skills, which are the qualities of a good consultant. Let’s look at how the two candidates performed.
Problem-Solving
Alex’s approach to solving the client’s problem was haphazard, as he posed a series of seemingly unrelated questions in no particular order. This method felt more like a rapid-fire Q&A session rather than a structured problem-solving approach.
On the other hand, Julie takes a structured and analytical approach to address profitability concerns. She quickly realizes that while revenue is one factor of profitability, it is likely costs that are the main concern, as they haven’t changed much in the last decade. She then breaks down the major cost categories and concludes that variable costs are the most likely opportunity for cost reduction. Julie is laser-focused on the client’s goal and efficiently gets to a solution.
Communication
Alex is not making a positive initial impression. If this were an actual client interaction, his questioning would appear disorganized and unprofessional.
On the other hand, Julie appears more organized through her clear communication style. She only considers the most pertinent issues at hand (i.e., the client’s business operations and costs) and avoids going down irrelevant rabbit holes.
- Understand the client’s problem; ask clarifying questions if needed.
- Formulate an issue tree to break down the problem into smaller parts.
- State the initial hypothesis and key assumptions to be tested.
- Gather and analyze information to prove or disprove the hypothesis; do not panic if the hypothesis is disproven.
- Pivot the hypothesis if necessary and repeat step 4. Otherwise, make your recommendation on what the client can do to solve their problem.
Other helpful tips to remember when using the hypothesis-driven approach:
- Stay focused on the client’s problem and remember what the end goal is.
- Think outside the box and consider new perspectives beyond traditional frameworks. The basic case interview frameworks are useful to understand but interviewers expect candidates to tailor to the specific client situation.
- Clearly communicate assumptions and implications throughout the interview; don’t assume the interviewer can read your mind.
A hypothesis-driven approach is closely tied to other key consulting concepts, such as issue trees, MECE, and 80/20. Let’s take a closer look at these topics and how they relate.
- Issue Trees
Issue trees, also known as decision trees, are visual tools that break down complex business problems into smaller, more manageable parts. In a consulting interview, candidates use the issue tree to outline key issues and potential factors in the client’s problem, demonstrating their understanding of the situation. This structure is then used to guide the case discussion, starting with the candidate’s best hypothesis, represented as one branch of the issue tree. For more information and examples of issue trees, check out our i ssue tree post.
During the interview process, consulting firms look for candidates who can demonstrate a MECE (mutually exclusive and collectively exhaustive) approach to problem-solving, which involves breaking down complex issues into distinct, non-overlapping components.
A MECE approach in case interviews involves identifying all potential paths to solving a client’s problem at a high level. This allows the candidate to form an initial hypothesis with confidence that no potential solutions have been overlooked. To gain a deeper understanding, read our comprehensive guide on the MECE case structure .
Consultants use the 80/20 rule, also known as the Pareto principle, to prioritize their efforts and focus on the most important things. This principle states that 80% of effects come from 20% of causes, which means a small number of issues often drive a large portion of the problem. By identifying and focusing on the key issues, consultants can achieve significant results with relatively minimal resources.
By following these tips and developing a solid understanding of the hypothesis-driven approach to case-solving, you will have the necessary tools to excel in your case interview. For more interview resources, check out Our Ultimate Guide to Case Interview Prep .
– – – – –
In this article, we’ve covered:
- Explanations of a hypothesis and hypothesis-driven approach
- Comparison between a hypothesis-driven approach and a non-hypothesis-driven approach
- Examples of the same case using both approaches and the key differences
- Practical tips on how to develop a hypothesis-driven mindset to ace the case
Still have questions?
If you have more questions about the best degrees for a career in consulting, leave them in the comments below. One of My Consulting Offer’s recruiters will answer them.
Other people preparing to apply to consulting firms found the following pages helpful:
- Our Ultimate Guide to Case Interview Prep
- Types of Case Interviews
- Case Frameworks
- Hypothesis Trees
Help with Your Consulting Application
Thanks for turning to My Consulting Offer for advice on the best majors for consulting. My Consulting Offer has helped almost 89.6% of the people we’ve worked with to get a job in management consulting. We want you to be successful in your consulting interviews too. For example, here is how Misha was able to get his offer from BCG.
Leave a Comment Cancel reply
Save my name, email, and website in this browser for the next time I comment.
© My CONSULTING Offer
3 Top Strategies to Master the Case Interview in Under a Week
We are sharing our powerful strategies to pass the case interview even if you have no business background, zero casing experience, or only have a week to prepare.
No thanks, I don't want free strategies to get into consulting.
We are excited to invite you to the online event., where should we send you the calendar invite and login information.

- 14 min read
Data-Driven Decision-Making Case Studies: Insights from Real-World Examples
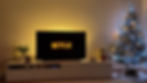
Data has become crucial for making informed decisions in today's fast-paced and ever-changing business environment. Companies use data to gain valuable insights, improve processes, and foster innovation. By studying successful examples of data-driven decision-making, we can gain valuable insights and comprehend the impact of data-driven strategies on business outcomes.
Define Data-Driven Decision Making (DDDM)
Are you tired of making business decisions based on gut instincts and guesswork? It's time to adopt Data-Driven Decision Making (DDDM). DDDM is a strategic approach that leverages collected data to inform and guide your business decisions. You can gain insights by identifying patterns and making informed choices using relevant and accurate data. "This can enhance the precision and efficiency of your decision-making procedure." allowing you to optimize outcomes, mitigate risks, and adapt more dynamically to changing circumstances in today's data-rich environment. Switch to DDDM and give your business the competitive edge it needs!
Importance of DDDM in Modern Businesses
In today's fast-paced and competitive business world, making informed and accurate decisions is more critical than ever. Data-Driven Decision Making (DDDM) is a powerful tool to help modern businesses achieve this goal. By using data and insights to inform business decisions rather than relying on guesswork, companies "Businesses that strategically position themselves to gain a competitive advantage are more likely to achieve success." With DDDM, businesses can make data-backed decisions, leading to better outcomes and tremendous success. So, if you want to stay ahead of your competition and make helpful decisions that drive success, embracing DDDM is the way to go!
Brief Overview of the Success Stories to be Discussed
Discover the success stories showcasing how businesses leverage advanced technologies to drive growth and profitability. Join me for an engaging and thought-provoking session where we will delve into the intricacies of these fascinating case studies. Your active participation will help us uncover valuable insights and unlock new perspectives that can benefit your work. "Make the most of this valuable opportunity to enhance your knowledge and skills!"
1. Netflix's Personalized Recommendations
2. Amazon's Supply Chain Optimization
3. Starbucks Location Analytics
4. American Express Fraud Detection
5. Zara's Fast Fashion Foresight.
You can benefit greatly from this unique opportunity to learn from some of the most innovative companies in the industry. Ensure you take advantage of this chance to expand your knowledge and skills!
Case Study 1: Netflix's Personalized Recommendation
Overview of netflix's challenges in content delivery.
Netflix faced challenges delivering content due to the diverse viewer preferences and vast content library. However, the company has been working hard to address these challenges and ensure users can discover content that aligns with their tastes. By doing so, Netflix aims to improve user satisfaction and retention rates.
How Netflix Used Viewer Data to Tailor Recommendations
By leveraging extensive viewer data, Netflix confidently tackled the challenge of recommending relevant content to its users. The platform thoroughly analyzed user behavior, viewing history, and preferences to create highly sophisticated algorithms. These algorithms were based on machine learning and could personalize content recommendations for each user. This approach significantly increased the likelihood of viewers engaging with content that resonated with their interests.
The Impact on Customer Retention and Satisfaction
The personalized content recommendations profoundly affected customer retention and satisfaction rates. Netflix enhanced the value of its service by providing users with content that closely matched their preferences. This created a stronger bond between users and the platform, leading to longer subscription durations and increased satisfaction.
Lessons Learned and Key Takeaways
Data is a Strategic Asset: Netflix's strategic use of data has wholly revolutionized content delivery. By utilizing excellent viewer data, they have successfully met the needs and preferences of each viewer in an incredibly effective manner.
Personalization Enhances Customer Experience: Personalized recommendations are essential to enhancing the overall customer experience. They can increase engagement, satisfaction, loyalty, and retention. Make no mistake - if you want to take your business to new heights, personalized recommendations are a must!
Continuous Adaptation is Crucial: It is crucial to adapt to achieve success, as Netflix continuously demonstrates. With the ever-evolving preferences of viewers, it is imperative to perform ongoing analysis and make necessary adjustments to algorithms to ensure that recommendations stay consistently relevant.
Balancing Privacy and Personalization: When utilizing viewer data, it is crucial to hit the right balance between personalization and privacy. Netflix has accomplished this by delivering highly personalized recommendations without compromising user privacy.
Netflix's approach to content delivery serves as an inspiration for the transformative power of data-driven decision-making. The personalized recommendations derived from customer data have proven to be a game changer regarding customer retention and satisfaction. The significance of adaptability, strategic use of data, and the balance between personalization and privacy, as highlighted by Netflix's success, can serve as a guide for other businesses looking to impact their customers positively.
Case study 2: amazon's supply chain optimization.
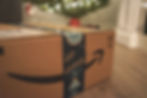
Understanding Amazon's Complex Supply Chain
Amazon has a complex supply chain involves various stages, from sourcing the products to delivering them to customers. The company manages a vast network of fulfillment centers, distribution hubs, and transportation systems. The complexity arises due to the need to manage different types of products, fluctuating demand, and the commitment to fast and efficient delivery.
Implementation of Predictive Analytics for Inventory Management
Using predictive analytics, Amazon has optimized inventory management by accurately forecasting future demand by analyzing historical data, current market trends, and seasonality. This has helped them prevent stockouts and overstock situations, improving their overall business efficiency and customer satisfaction.
Results Achieved in Cost Savings and Delivery Times
Anticipating the future, the implementation of predictive analytics is expected to yield significant results. Amazon will likely achieve cost savings by minimizing excess inventory and improving warehouse efficiency. Additionally, streamlined inventory management contributes to faster order fulfillment, which reduces delivery times and enhances the customer experience. We can expect a boost in efficiency and a better customer experience shortly.
Insights Gained and How Businesses Can Apply Similar Strategies
Data-Driven Decision-Making: In today's business landscape, data-driven decision-making has become the cornerstone of success. If you want your business to thrive, you must leverage advanced analytics to gain actionable insights into your supply chains. This will enable you to make proactive and strategic decisions to enhance your projects. Don't fall behind the competition - take charge and start leveraging the power of data-driven decision-making now.
Dynamic Inventory Optimization: Incorporating a dynamic approach to inventory management that relies on predictive analytics is "Businesses must prioritize their competitive edge and remain ahead of the industry. This is crucial to ensure success and longevity.". It helps them to quickly adjust to changing market conditions and meet the ever-evolving demands of consumers. This not only optimizes the utilization of resources but also reduces wastage, making it a sound strategy crucial for any business that wishes to survive in today's competitive market landscape.
Focus on Customer-Centric Logistics: To improve customer satisfaction, businesses can focus on optimizing logistics and reducing delivery times. Amazon's customer-centric approach demonstrates the importance of fast and reliable delivery. Companies can boost customer loyalty and drive growth by enhancing the customer experience.
Investment in Technology: To stay ahead in supply chain optimization, businesses must adopt cutting-edge technologies like AI and machine learning. Amazon's supply chain success is a testament to the power of continuous investment in technology. So, if you want to thrive in today's competitive market, it's high time you leverage these technologies to your advantage.
Amazon's journey toward optimizing its supply chain through predictive analytics has tremendously impacted cost savings and delivery times. Other businesses can achieve similar results by utilizing data-driven decision-making, implementing dynamic inventory management, prioritizing customer-centric logistics, and investing in advanced technologies.
Case study 3: starbucks location analytics.
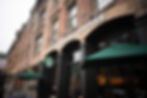
The Problem with Traditional Site Selection
The traditional approach to retail site selection, which relied on broad demographic data and market trends, must be improved in identifying optimal locations. Adopting a more precise approach that considers specific local factors influencing consumer behavior and store performance is imperative to ensure success.
How Starbucks Leveraged Geographic Information Systems (GIS)
Starbucks has transformed its approach to selecting store locations by leveraging Geographic Information Systems (GIS). This innovative technology has enabled Starbucks to systematically evaluate and visualize location-specific data, such as foot traffic patterns, nearby businesses, demographics, and local economic factors. By conducting this comprehensive analysis, Starbucks can gain a more nuanced understanding of potential store locations and make informed decisions.
Outcomes in Terms of New Store Performance and Sales
Starbucks, the renowned coffeehouse chain, has achieved notable success in its site selection strategy by implementing a Geographic Information System (GIS). GIS technology has enabled Starbucks to strategically place its new stores in locations that cater to the preferences and traffic patterns of the local population. As a result, the company has witnessed a significant improvement in the performance of its new stores, surpassing the sales of those selected through traditional methods. The successful implementation of GIS in site selection has contributed to optimizing location decisions, leading to a more efficient and effective expansion strategy for Starbucks.
Broader Implications for Retail Location Decision-Making
Precision in Site Selection: Geographic Information System (GIS) technology has opened new doors for retailers to make informed decisions. By leveraging GIS, businesses can analyze and interpret specific geographic data to optimize their site selection process. This helps them understand local nuances and customer behavior more precisely, allowing them to make data-driven decisions that lead to better business outcomes.
Adaptability to Local Factors: To establish a closer relationship with their customers, retailers must consider various local factors such as competition, demographics, and cultural preferences. By doing so, they can customize their offerings and marketing strategies to fit the local communities' specific needs and preferences. This approach can lead to better customer engagement and loyalty and, ultimately, higher sales for the retailer.
Cost Efficiency: Regarding retail businesses, selecting the right location for a store is crucial for success. Optimal site selection can significantly reduce the risk of underperforming stores and minimize the financial impact of poor location decisions. Retail businesses can enhance their overall cost efficiency and profitability by doing so. This is why it is essential for companies to carefully analyze and consider factors before making any site selection decisions.
Strategic Expansion: Geographic Information System (GIS) provides retailers with a powerful tool to make informed decisions about expanding their business. By leveraging location-based data, retailers can discover new markets and potential locations for growth. This data-driven approach helps retailers create a more sustainable and prosperous expansion plan, resulting in long-term prosperity for the business.
Enhanced Customer Experience: Retailers can improve the shopping experience for their customers by strategically selecting store locations that cater to their preferences and habits. Retailers can attract more foot traffic and enhance customer satisfaction by offering conveniently located stores. In this case, it can increase sales and customer loyalty.
To make it more understandable, Starbucks has been using fantastic GIS technology to help them pick the best locations for their stores. It's like a digital map that allows them to look at much different information, like how many people live nearby, how much traffic there is, and what other businesses are in the area. By using this technology, Starbucks can make better choices about where to put their stores and how they can be successful. Other businesses can also use GIS to make better decisions about where to open new stores and how to compete in the changing world of retail.
Case study 4: american express fraud detection.
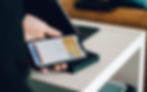
Rise in Credit Card Fraud and the Challenge for Card Issuers
As more and more people turn to digital transactions and online commerce, it's essential to be aware of the increased incidence of credit card fraud. Protect yourself and others from financial crimes by staying informed and proactively safeguarding your financial information. Thus, posing a significant challenge for card issuers. Fraudsters are constantly devising new and innovative tactics to steal sensitive information and exploit vulnerabilities in payment systems. This makes it imperative for financial institutions to stay ahead of the game in detecting and preventing fraudulent activities. Today, with advanced technologies like machine learning and artificial intelligence, card issuers can analyze big data that can identify patterns and anomalies and indicate fraudulent behavior. By adopting a proactive approach to fraud detection and prevention, financial institutions can safeguard their customers' personal information and financial assets, thus building trust and loyalty among their clients.
American Express's Use of Machine Learning for Early Detection
American Express always prioritizes the security of its customers' financial transactions. The company has employed advanced machine-learning algorithms to analyze vast amounts of real-time transaction data to achieve this. These algorithms can identify patterns, anomalies, and behavioral indicators typically associated with fraudulent activities. By continuously learning from new data, the system adapts to evolving fraud tactics and enhances its ability to detect irregularities early on. This advanced technology is a critical component of American Express's fraud prevention strategy, and it helps the company safeguard its customers against potential financial losses.
Effectiveness in Preventing Fraud and Protecting Customers
American Express utilizes an advanced fraud detection system powered by machine learning that has demonstrated exceptional efficacy in preventing fraudulent activities and safeguarding customers. By detecting fraudulent transactions early, the company can promptly take necessary measures, such as notifying customers of suspicious activities or blocking them altogether, thus reinforcing trust in the company's commitment to security and ensuring customer satisfaction.
What Companies Can Learn About Proactive Data Monitoring
Invest in Advanced Analytics: Advanced analytics, such as machine learning, helps companies proactively monitor data for potential fraud indicators and unusual patterns. It identifies issues before they become significant problems, saving the company millions. It also identifies new business opportunities, market trends, and operational inefficiencies, enhancing customer satisfaction and the bottom line.
Real-Time Analysis: Real-time data analysis is a powerful tool for detecting and responding to suspicious activities. By monitoring data in real-time, organizations can quickly "Identify possible threats and take prompt action to reduce their impact." we can overcome any challenges that come our way and pave the path to success. This approach reduces the window of vulnerability and enhances the effectiveness of fraud prevention measures. Therefore, real-time data analysis can help organizations prevent fraudsters from exploiting them. It's important to stay vigilant and proactive in protecting yourself and your finances from potential threats. Please take action now and don't give them the chance to cause any harm. Remember, it's better to be safe than sorry. their interests.
Continuous Learning Systems: Adopting systems that can learn and adapt to new fraud patterns is highly recommended. This approach ensures that the monitoring mechanisms remain up-to-date and effective despite the constantly evolving threats. Embracing such systems can protect businesses. The objective is to safeguard individuals and organizations against financial losses and reputational harm resulting from fraudulent activities.
Customer Communication: Implementing solid and effective communication methods is essential to inform customers of any potential fraud promptly. Through transparent communication, customers can be informed of the situation and take immediate action, building trust between them and the organization.
Collaboration with Industry Partners: Collaborating with industry partners and sharing insights on emerging fraud trends is essential. By working together, we can enhance our ability to combat fraud and protect the entire ecosystem. We can stay informed and better equipped to prevent fraudulent activities through a collective effort.
Balancing Security and User Experience: It's crucial to balance strong security measures with a seamless user experience for online platform security. While taking all necessary steps to prevent fraud and unauthorized access to your system is critical, ensuring that your legitimate customers don't face any inconvenience or dissatisfaction due to stringent security protocols is equally essential. Therefore, adopting a multi-layered approach to security is recommended to shield your system from potential threats without making the user experience cumbersome or frustrating. This may involve utilizing two-factor and risk-based authentication and real-time fraud detection. Furthermore, educating users on secure online practices and equipping them with the necessary tools and resources to protect their personal information and transactions is essential.
American Express has implemented machine learning techniques to detect and prevent fraud at an early stage. This is an excellent example for businesses seeking to improve their proactive data monitoring capabilities. By adopting advanced analytical tools, real-time analysis, continuous learning systems, and effective communication, companies can establish a solid and proactive strategy to combat emerging threats in the digital realm.
Case study 5: zara's fast fashion foresight.
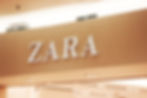
Fast Fashion Industry Challenges in Demand Forecasting
The fast fashion industry, known for producing trendy and affordable clothing rapidly, faces significant challenges in accurately predicting consumer demand. One of the main reasons for this is the constantly changing nature of fashion trends. What is popular today may be out of fashion tomorrow, making it difficult for companies to plan their production processes effectively.
Additionally, fast fashion products have short life cycles, meaning they are only in style for a limited time. As a result, companies need to be able to respond to market shifts and adjust their production accordingly quickly. This can be challenging, as traditional forecasting methods rely on historical data, which may need to be more relevant in a fast-changing market.
To overcome these challenges, the fast fashion industry needs innovative and agile forecasting methods to keep up with the dynamic nature of consumer preferences. This may involve leveraging data analytics and machine learning algorithms to identify emerging trends and predict future demand. Companies can enhance efficiency, reduce waste, and provide excellent customer value.
Zara's Integration of Real-Time Sales Data into Production Decisions
Zara, one of the world's leading fashion retailers, has redefined the fashion industry by leveraging real-time sales data. Zara has integrated real-time sales data into its production decisions, allowing the company to stay ahead of the competition. Zara's vertically integrated supply chain and responsive production model enable it to capture up-to-the-minute sales data from its stores worldwide. This data is then fed back to the design and production teams, who use it to rapidly adjust inventory levels and introduce new designs based on current demand trends. Using real-time sales data, Zara can create a customer-centric approach, ensuring its customers always have access to the latest and most stylish designs.
Benefits Seen in Reduced Waste and Increased Sales
Zara has adopted a real-time analytics approach that has proven to be highly beneficial. The company's production is now closely aligned with actual customer demand, which results in a significant reduction in overstock and markdowns. This approach has minimized the environmental impact of excessive inventory. In addition, the quick response to emerging trends and consumer preferences has led to an increase in full-price sales, boosting revenue and profitability for Zara.
Strategies for Incorporating Real-Time Analytics into Product Development
Connected Supply Chain: Establishing a connected and transparent supply chain that enables seamless real-time data flow from sales channels to production and design teams is imperative. This will ensure that all the teams are on the same page and can make quick, informed decisions based on accurate, up-to-date information. Failure to do so can result in costly delays, inefficiencies, and missed opportunities. So, let's prioritize this and set up a robust supply chain that works for us!
Agile Production Processes: To maintain a competitive edge, it is crucial to adopt constructive and flexible production processes that can quickly respond to changes in demand. This involves embracing shorter production cycles and smaller batch sizes, which makes us more efficient and proactive in meeting customer needs.
Advanced Data Analytics: To optimize your sales strategies, you can use advanced data analytics tools to process and analyze real-time sales data efficiently. You can accurately forecast demand and make data-driven decisions by implementing predictive modeling and machine learning algorithms.
Cross-Functional Collaboration: Promoting collaboration among different organizational departments is crucial to ensure the sales, marketing, design, and production teams have a unified interpretation of real-time data. This way, they can collectively make informed decisions that are in the company's best interest. The organization can improve its efficiency, productivity, and profitability by promoting open communication and collaboration between departments.
Customer Feedback Integration: One way to enhance the accuracy of real-time analytics is to consider customer feedback and preferences. Social media listening and direct customer interactions can provide valuable insights into emerging trends and demands.
Technology Integration: As we look towards the future, it's becoming increasingly clear that investing in technologies that facilitate real-time data collection and processing will be crucial. With the rise of automation and the growing need for instant information, businesses that have point-of-sale systems, inventory management software, and communication tools that streamline information flow will be better equipped to thrive in the fast-paced and ever-changing world of tomorrow. So, it's never too soon to start thinking about how you can integrate these technologies into your business strategy.
Zara's outstanding achievement in the fast fashion industry is a remarkable example of how incorporating real-time sales data into production decisions can lead to immense success. By reducing waste, swiftly responding to market trends, and utilizing advanced analytics, Zara has set a benchmark for other companies in integrating real-time insights into their product development strategies. This approach enhances efficiency and competitiveness in the highly dynamic and ever-evolving fashion industry.
In the constantly shifting business realm, the adage' knowledge is power' has never been more accurate, particularly about tangible, data-derived knowledge. data-driven decision making (dddm) presents an approach where critical business decisions are made not on intuition or experience alone, but on deep dives into data analysis. empirical evidence garnered through this method provides actionable insights leading to strategic, evidence-based decisions..
The stories of Netflix, Amazon, Starbucks, American Express, and Zara demonstrate the immense potential of Data-Driven Decision Making (DDDM). By analyzing vast data points and leveraging advanced analytics, these companies transformed their businesses and achieved unparalleled success.
For instance, Netflix utilized DDDM to create tailor-made recommendations, engaging existing users and attracting new ones. Amazon used data analytics to optimize its supply chain, lowering costs and accelerating shipping times. Starbucks leveraged location analytics to predict the profitability of new store locations with impressive accuracy. American Express used machine learning algorithms to identify frauds faster than ever, saving millions in potential losses. Lastly, Zara demonstrated agility in the competitive fast fashion market by adapting its production and supply chain to meet real-time demand.
As seen in these success stories, data-driven decision-making can powerfully impact business, from customer engagement to trend forecasting. They underscore the importance of meticulous data analysis in navigating the present and forecasting an ever-changing future, paving the way for unparalleled business success. Companies can draw inspiration from these cases and embark on their DDDM journey to achieve similar outcomes.
Recent Posts
Maximizing the potential of WFM in the Gig Economy: Achieving the perfect balance between Flexibility and Stability through Case Studies.
WFM in the Gig Economy: Balancing Flexibility and Stability
Data-Driven Decision Making: Utilizing Analytics in Workforce Management
- Business Essentials
- Leadership & Management
- Credential of Leadership, Impact, and Management in Business (CLIMB)
- Entrepreneurship & Innovation
- Digital Transformation
- Finance & Accounting
- Business in Society
- For Organizations
- Support Portal
- Media Coverage
- Founding Donors
- Leadership Team
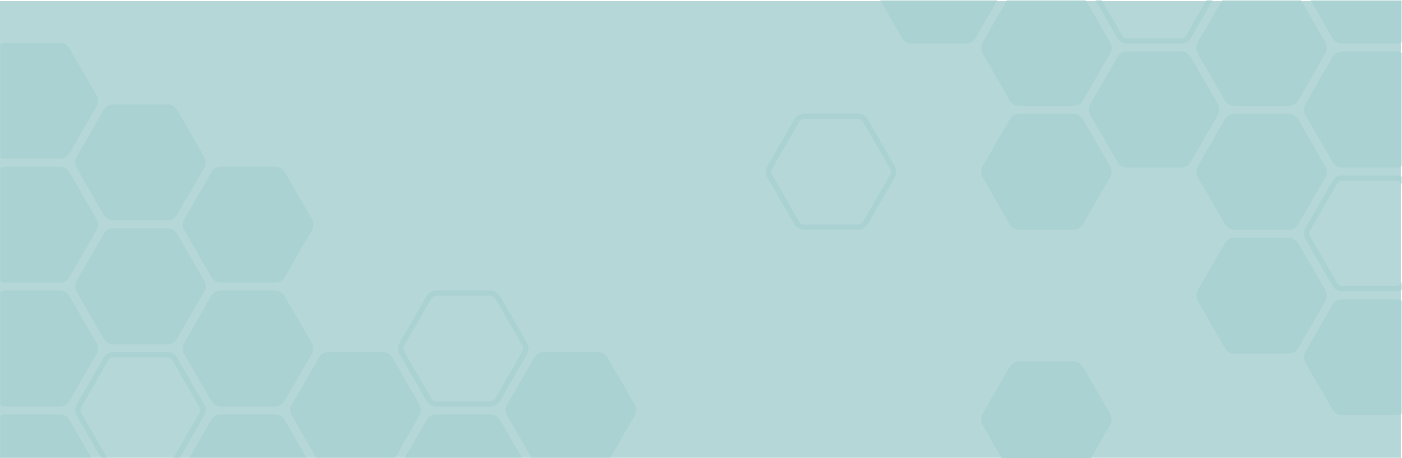
- Harvard Business School →
- HBS Online →
- Business Insights →
Business Insights
Harvard Business School Online's Business Insights Blog provides the career insights you need to achieve your goals and gain confidence in your business skills.
- Career Development
- Communication
- Decision-Making
- Earning Your MBA
- Negotiation
- News & Events
- Productivity
- Staff Spotlight
- Student Profiles
- Work-Life Balance
- AI Essentials for Business
- Alternative Investments
- Business Analytics
- Business Strategy
- Business and Climate Change
- Creating Brand Value
- Design Thinking and Innovation
- Digital Marketing Strategy
- Disruptive Strategy
- Economics for Managers
- Entrepreneurship Essentials
- Financial Accounting
- Global Business
- Launching Tech Ventures
- Leadership Principles
- Leadership, Ethics, and Corporate Accountability
- Leading Change and Organizational Renewal
- Leading with Finance
- Management Essentials
- Negotiation Mastery
- Organizational Leadership
- Power and Influence for Positive Impact
- Strategy Execution
- Sustainable Business Strategy
- Sustainable Investing
- Winning with Digital Platforms
The Advantages of Data-Driven Decision-Making
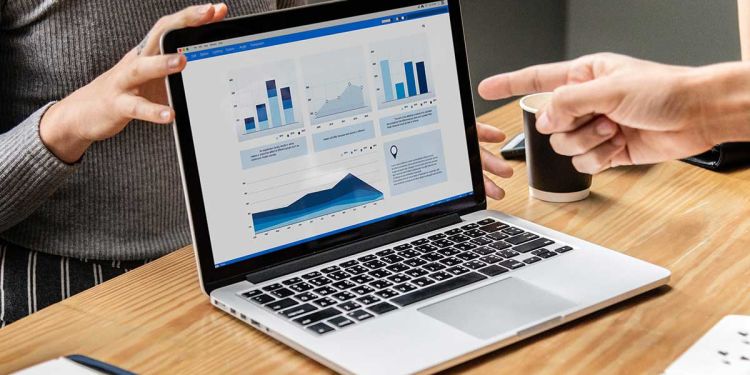
- 26 Aug 2019
Society has imbued the concept of “intuition”—of simply knowing when something is right or wrong—with a tremendous amount of prestige, importance, and influence.
In fact, according to some studies, more than half of Americans rely on their “gut” in order to decide what to believe, even when they are confronted with evidence that speaks to the contrary.
The concept of intuition has become so romanticized in modern life that it's now a part of how many people talk about and understand the “geniuses” of our generation. In science, for example, Albert Einstein is often quoted as saying, “The intuitive mind is a sacred gift," and in business, Steve Jobs is quoted as saying, “Have the courage to follow your heart and intuition; they somehow already know what you want to become.”
Though intuition can be a helpful tool , it would be a mistake to base all decisions around a mere gut feeling.
While intuition can provide a hunch or spark that starts you down a particular path, it's through data that you verify, understand, and quantify. According to a survey of more than 1,000 senior executives conducted by PwC, highly data-driven organizations are three times more likely to report significant improvements in decision-making compared to those who rely less on data.
Are you interested in learning how data-driven decision-making can enable you to be a more effective entrepreneur or member of your organization? Below is information about the benefits of becoming more data-driven, as well as a number of steps you can take to become more analytical in your processes.
Access your free e-book today.
What Is Data-Driven Decision-Making?
Data-driven decision-making (sometimes abbreviated as DDDM) is the process of using data to inform your decision-making process and validate a course of action before committing to it.
In business, this is seen in many forms. For example, a company might:
- Collect survey responses to identify products, services, and features their customers would like
- Conduct user testing to observe how customers are inclined to use their product or services and to identify potential issues that should be resolved prior to a full release
- Launch a new product or service in a test market in order to test the waters and understand how a product might perform in the market
- Analyze shifts in demographic data to determine business opportunities or threats
How exactly data can be incorporated into the decision-making process will depend on a number of factors, such as your business goals and the types and quality of data you have access to.
The collection and analysis of data have long played an important role in enterprise-level corporations and organizations. But as humanity generates more than 2.5 quintillion bytes of data each day, it's never been easier for businesses of all sizes to collect, analyze, and interpret data into real, actionable insights. Though data-driven decision-making has existed in business in one form or another for centuries, it’s a truly modern phenomenon.
Examples of Data-Driven Decision-Making
Today’s largest and most successful organizations use data to their advantage when making high-impact business decisions. To better understand how your organization can incorporate data analytics into its decision-making process, consider the success stories of these well-known businesses.
1. Leadership Development at Google
Google maintains a heavy focus on what it refers to as “people analytics.” As part of one of its well-known people analytics initiatives, Project Oxygen, Google mined data from more than 10,000 performance reviews and compared the data with employee retention rates. Google used the information to identify common behaviors of high-performing managers and created training programs to develop these competencies. These efforts boosted median favorability scores for managers from 83 percent to 88 percent .
2. Real Estate Decisions at Starbucks
After hundreds of Starbucks locations were closed in 2008, then-CEO Howard Schultz promised that the company would take a more analytical approach to identifying future store locations.
Starbucks now partners with a location-analytics company to pinpoint ideal store locations using data like demographics and traffic patterns. The organization also considers input from its regional teams before making decisions. Starbucks uses this data to determine the likelihood of success for a particular location before taking on a new investment.
3. Driving Sales at Amazon
Amazon uses data to decide which products they should recommend to customers based on their prior purchases and patterns in search behavior. Rather than blindly suggesting a product, Amazon uses data analytics and machine learning to drive its recommendation engine. McKinsey estimated that, in 2017, 35 percent of Amazon’s consumer purchases could be tied back to the company’s recommendation system.
Benefits of Data-Driven Decision-Making
1. you’ll make more confident decisions.
Once you begin collecting and analyzing data, you’re likely to find that it’s easier to reach a confident decision about virtually any business challenge, whether you’re deciding to launch or discontinue a product, adjust your marketing message , branch into a new market, or something else entirely.
Data performs multiple roles. On the one hand, it serves to benchmark what currently exists, which allows you to better understand the impact that any decision you make will have on your business.
Beyond this, data is logical and concrete in a way that gut instinct and intuition simply aren’t. By removing the subjective elements from your business decisions, you can instill confidence in yourself and your company as a whole. This confidence allows your organization to commit fully to a particular vision or strategy without being overly concerned that the wrong decision has been made.
Just because a decision is based on data doesn’t mean it will always be correct. While the data might show a particular pattern or suggest a certain outcome, if the data collection process or interpretation is flawed, then any decision based on the data would be inaccurate. This is why the impact of every business decision should be regularly measured and monitored.
Related: 3 Examples of Business Analytics in Action
2. You’ll Become More Proactive
When you first implement a data-driven decision-making process, it’s likely to be reactionary in nature. The data tells a story, which you and your organization must then react to.
While this is valuable in its own right, it’s not the only role that data and analysis can play within your business. Given enough practice and the right types and quantities of data, it’s possible to leverage it in a more proactive way—for example, by identifying business opportunities before your competition does, or by detecting threats before they grow too serious.
3. You Can Realize Cost Savings
There are many reasons a business might choose to invest in a big data initiative and aim to become more data-driven in its processes. According to a recent survey of Fortune 1,000 executives conducted by NewVantage Partners for the Harvard Business Review , these initiatives vary in their rates of success.
One of the most impactful initiatives, according to the survey, is using data to decrease expenses. Of the organizations which began projects designed to decrease expenses, more than 49 percent have seen value from their projects. Other initiatives have shown more mixed results.
“Big data is already being used to improve operational efficiency,” said Randy Bean, CEO and managing partner of consultancy firm NewVantage Partners, when announcing the results of the survey. “And the ability to make informed decisions based on the very latest up-to-the-moment information is rapidly becoming the mainstream norm.”
How to Become More Data-Driven
If you have a goal of becoming more data-driven in your approach to business, there are many steps you can take to reach that goal. Here's a look at some of the ways you can approach your daily tasks with an analytical mindset.
1. Look for Patterns Everywhere
Data analysis is, at its heart, an attempt to find a pattern within, or correlation between, different data points. It’s from these patterns and correlations that insights and conclusions can be drawn.
The first step in becoming more data-driven is making a conscious decision to be more analytical —both in business as well as in your personal life. While this might seem simple, it’s something that takes practice.
Whether you’re in the office pouring over financial statements , standing in line at the grocery store, or commuting on the train, look for patterns in the data around you. Once you have noticed those patterns, practice extrapolating insights and try to draw conclusions as to why they exist. This simple exercise can help you train yourself to become more data-driven in other areas of your life.
2. Tie Every Decision Back to the Data
Whenever you’re presented with a decision, whether business-related or personal in nature, do your best to avoid relying on gut instinct or past behavior when determining a course of action. Instead, make a conscious effort to apply an analytical mindset.
Identify what data you have available that can be used to inform your decision. If no data exists, consider ways in which you could collect it on your own. Once you have the data, analyze it, and use any insights to help you make your decision. As with the pattern-spotting exercise, the idea is to give yourself enough practice that analysis becomes a natural part of your decision-making process .
3. Visualize the Meaning Behind the Data
Data visualization is a huge part of the data analysis process. It’s nearly impossible to derive meaning from a table of numbers. By creating engaging visuals in the form of charts and graphs, you’ll be able to quickly identify trends and make conclusions about the data.
Familiarize yourself with popular data visualization techniques and tools , and practice creating visualizations with any form of data you have readily available. This can be as simple as creating a graph to visualize your monthly spending habits and drawing conclusions from the visualization. You can then use these insights to make a personal budget for the next month. After completing that exercise, you’ll have successfully made a data-driven decision.
4. Consider Furthering Your Education
If you’re uncomfortable with the idea of learning how to incorporate data into your decision-making process on your own, there are a number of educational options you can pursue to develop the data science skills needed to succeed.
Which option makes the most sense will depend on your personal and professional goals. For example, individuals considering a serious career change might decide to pursue a master’s degree with an emphasis on data analytics or data science. But for everyone else, simply taking an online business analytics or data science course could be enough to lay the foundation necessary for success.

Using Data to Answer Critical Questions
While there are many benefits to data-driven decision-making, it’s important to note that you don’t need to take an all-or-nothing approach to get there. By starting small, benchmarking your performance, documenting everything, and adjusting as you go, you can become more data-driven and thrive at your organization.
Do you want to learn more about how to use data to inform business decisions at your organization? Download our Beginner’s Guide to Data & Analytics to learn how you can leverage the power of data for professional and organizational success. This post was updated on February 19, 2021. It was originally published on August 26, 2019.

About the Author
Thank you for visiting nature.com. You are using a browser version with limited support for CSS. To obtain the best experience, we recommend you use a more up to date browser (or turn off compatibility mode in Internet Explorer). In the meantime, to ensure continued support, we are displaying the site without styles and JavaScript.
- View all journals
- Explore content
- About the journal
- Publish with us
- Sign up for alerts
- Open access
- Published: 09 September 2022
Machine learning in project analytics: a data-driven framework and case study
- Shahadat Uddin 1 ,
- Stephen Ong 1 &
- Haohui Lu 1
Scientific Reports volume 12 , Article number: 15252 ( 2022 ) Cite this article
11k Accesses
21 Citations
18 Altmetric
Metrics details
- Applied mathematics
- Computational science
The analytic procedures incorporated to facilitate the delivery of projects are often referred to as project analytics. Existing techniques focus on retrospective reporting and understanding the underlying relationships to make informed decisions. Although machine learning algorithms have been widely used in addressing problems within various contexts (e.g., streamlining the design of construction projects), limited studies have evaluated pre-existing machine learning methods within the delivery of construction projects. Due to this, the current research aims to contribute further to this convergence between artificial intelligence and the execution construction project through the evaluation of a specific set of machine learning algorithms. This study proposes a machine learning-based data-driven research framework for addressing problems related to project analytics. It then illustrates an example of the application of this framework. In this illustration, existing data from an open-source data repository on construction projects and cost overrun frequencies was studied in which several machine learning models (Python’s Scikit-learn package) were tested and evaluated. The data consisted of 44 independent variables (from materials to labour and contracting) and one dependent variable (project cost overrun frequency), which has been categorised for processing under several machine learning models. These models include support vector machine, logistic regression, k -nearest neighbour, random forest, stacking (ensemble) model and artificial neural network. Feature selection and evaluation methods, including the Univariate feature selection, Recursive feature elimination, SelectFromModel and confusion matrix, were applied to determine the most accurate prediction model. This study also discusses the generalisability of using the proposed research framework in other research contexts within the field of project management. The proposed framework, its illustration in the context of construction projects and its potential to be adopted in different contexts will significantly contribute to project practitioners, stakeholders and academics in addressing many project-related issues.
Similar content being viewed by others
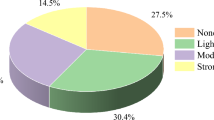
Long-term prediction modeling of shallow rockburst with small dataset based on machine learning
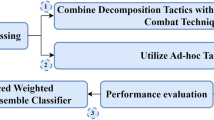
An ensemble-based machine learning solution for imbalanced multiclass dataset during lithology log generation
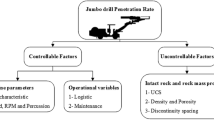
Prediction of jumbo drill penetration rate in underground mines using various machine learning approaches and traditional models
Introduction.
Successful projects require the presence of appropriate information and technology 1 . Project analytics provides an avenue for informed decisions to be made through the lifecycle of a project. Project analytics applies various statistics (e.g., earned value analysis or Monte Carlo simulation) among other models to make evidence-based decisions. They are used to manage risks as well as project execution 2 . There is a tendency for project analytics to be employed due to other additional benefits, including an ability to forecast and make predictions, benchmark with other projects, and determine trends such as those that are time-dependent 3 , 4 , 5 . There has been increasing interest in project analytics and how current technology applications can be incorporated and utilised 6 . Broadly, project analytics can be understood on five levels 4 . The first is descriptive analytics which incorporates retrospective reporting. The second is known as diagnostic analytics , which aims to understand the interrelationships and underlying causes and effects. The third is predictive analytics which seeks to make predictions. Subsequent to this is prescriptive analytics , which prescribes steps following predictions. Finally, cognitive analytics aims to predict future problems. The first three levels can be applied with ease with the help of technology. The fourth and fifth steps require data that is generally more difficult to obtain as they may be less accessible or unstructured. Further, although project key performance indicators can be challenging to define 2 , identifying common measurable features facilitates this 7 . It is anticipated that project analytics will continue to experience development due to its direct benefits to the major baseline measures focused on productivity, profitability, cost, and time 8 . The nature of project management itself is fluid and flexible, and project analytics allows an avenue for which machine learning algorithms can be applied 9 .
Machine learning within the field of project analytics falls into the category of cognitive analytics, which deals with problem prediction. Generally, machine learning explores the possibilities of computers to improve processes through training or experience 10 . It can also build on the pre-existing capabilities and techniques prevalent within management to accomplish complex tasks 11 . Due to its practical use and broad applicability, recent developments have led to the invention and introduction of newer and more innovative machine learning algorithms and techniques. Artificial intelligence, for instance, allows for software to develop computer vision, speech recognition, natural language processing, robot control, and other applications 10 . Specific to the construction industry, it is now used to monitor construction environments through a virtual reality and building information modelling replication 12 or risk prediction 13 . Within other industries, such as consumer services and transport, machine learning is being applied to improve consumer experiences and satisfaction 10 , 14 and reduce the human errors of traffic controllers 15 . Recent applications and development of machine learning broadly fall into the categories of classification, regression, ranking, clustering, dimensionality reduction and manifold learning 16 . Current learning models include linear predictors, boosting, stochastic gradient descent, kernel methods, and nearest neighbour, among others 11 . Newer and more applications and learning models are continuously being introduced to improve accessibility and effectiveness.
Specific to the management of construction projects, other studies have also been made to understand how copious amounts of project data can be used 17 , the importance of ontology and semantics throughout the nexus between artificial intelligence and construction projects 18 , 19 as well as novel approaches to the challenges within this integration of fields 20 , 21 , 22 . There have been limited applications of pre-existing machine learning models on construction cost overruns. They have predominantly focussed on applications to streamline the design processes within construction 23 , 24 , 25 , 26 , and those which have investigated project profitability have not incorporated the types and combinations of algorithms used within this study 6 , 27 . Furthermore, existing applications have largely been skewed towards one type or another 28 , 29 .
In addition to the frequently used earned value method (EVM), researchers have been applying many other powerful quantitative methods to address a diverse range of project analytics research problems over time. Examples of those methods include time series analysis, fuzzy logic, simulation, network analytics, and network correlation and regression. Time series analysis uses longitudinal data to forecast an underlying project's future needs, such as the time and cost 30 , 31 , 32 . Few other methods are combined with EVM to find a better solution for the underlying research problems. For example, Narbaev and De Marco 33 integrated growth models and EVM for forecasting project cost at completion using data from construction projects. For analysing the ongoing progress of projects having ambiguous or linguistic outcomes, fuzzy logic is often combined with EVM 34 , 35 , 36 . Yu et al. 36 applied fuzzy theory and EVM for schedule management. Ponz-Tienda et al. 35 found that using fuzzy arithmetic on EVM provided more objective results in uncertain environments than the traditional methodology. Bonato et al. 37 integrated EVM with Monte Carlo simulation to predict the final cost of three engineering projects. Batselier and Vanhoucke 38 compared the accuracy of the project time and cost forecasting using EVM and simulation. They found that the simulation results supported findings from the EVM. Network methods are primarily used to analyse project stakeholder networks. Yang and Zou 39 developed a social network theory-based model to explore stakeholder-associated risks and their interactions in complex green building projects. Uddin 40 proposed a social network analytics-based framework for analysing stakeholder networks. Ong and Uddin 41 further applied network correlation and regression to examine the co-evolution of stakeholder networks in collaborative healthcare projects. Although many other methods have already been used, as evident in the current literature, machine learning methods or models are yet to be adopted for addressing research problems related to project analytics. The current investigation is derived from the cognitive analytics component of project analytics. It proposes an approach for determining hidden information and patterns to assist with project delivery. Figure 1 illustrates a tree diagram showing different levels of project analytics and their associated methods from the literature. It also illustrates existing methods within the cognitive component of project analytics to where the application of machine learning is situated contextually.
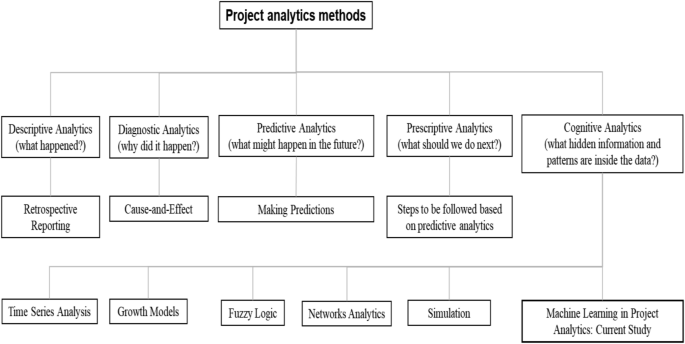
A tree diagram of different project analytics methods. It also shows where the current study belongs to. Although earned value analysis is commonly used in project analytics, we do not include it in this figure since it is used in the first three levels of project analytics.
Machine learning models have several notable advantages over traditional statistical methods that play a significant role in project analytics 42 . First, machine learning algorithms can quickly identify trends and patterns by simultaneously analysing a large volume of data. Second, they are more capable of continuous improvement. Machine learning algorithms can improve their accuracy and efficiency for decision-making through subsequent training from potential new data. Third, machine learning algorithms efficiently handle multi-dimensional and multi-variety data in dynamic or uncertain environments. Fourth, they are compelling to automate various decision-making tasks. For example, machine learning-based sentiment analysis can easily a negative tweet and can automatically take further necessary steps. Last but not least, machine learning has been helpful across various industries, for example, defence to education 43 . Current research has seen the development of several different branches of artificial intelligence (including robotics, automated planning and scheduling and optimisation) within safety monitoring, risk prediction, cost estimation and so on 44 . This has progressed from the applications of regression on project cost overruns 45 to the current deep-learning implementations within the construction industry 46 . Despite this, the uses remain largely limited and are still in a developmental state. The benefits of applications are noted, such as optimising and streamlining existing processes; however, high initial costs form a barrier to accessibility 44 .
The primary goal of this study is to demonstrate the applicability of different machine learning algorithms in addressing problems related to project analytics. Limitations in applying machine learning algorithms within the context of construction projects have been explored previously. However, preceding research has mainly been conducted to improve the design processes specific to construction 23 , 24 , and those investigating project profitabilities have not incorporated the types and combinations of algorithms used within this study 6 , 27 . For instance, preceding research has incorporated a different combination of machine-learning algorithms in research of predicting construction delays 47 . This study first proposed a machine learning-based data-driven research framework for project analytics to contribute to the proposed study direction. It then applied this framework to a case study of construction projects. Although there are three different machine learning algorithms (supervised, unsupervised and semi-supervised), the supervised machine learning models are most commonly used due to their efficiency and effectiveness in addressing many real-world problems 48 . Therefore, we will use machine learning to represent supervised machine learning throughout the rest of this article. The contribution of this study is significant in that it considers the applications of machine learning within project management. Project management is often thought of as being very fluid in nature, and because of this, applications of machine learning are often more difficult 9 , 49 . Further to this, existing implementations have largely been limited to safety monitoring, risk prediction, cost estimation and so on 44 . Through the evaluation of machine-learning applications, this study further demonstrates a case study for which algorithms can be used to consider and model the relationship between project attributes and a project performance measure (i.e., cost overrun frequency).
Machine learning-based framework for project analytics
When and why machine learning for project analytics.
Machine learning models are typically used for research problems that involve predicting the classification outcome of a categorical dependent variable. Therefore, they can be applied in the context of project analytics if the underlying objective variable is a categorical one. If that objective variable is non-categorical, it must first be converted into a categorical variable. For example, if the objective or target variable is the project cost, we can convert this variable into a categorical variable by taking only two possible values. The first value would be 0 to indicate a low-cost project, and the second could be 1 for showing a high-cost project. The average or median cost value for all projects under consideration can be considered for splitting project costs into low-cost and high-cost categories.
For data-driven decision-making, machine learning models are advantageous. This is because traditional statistical methods (e.g., ordinary least square (OLS) regression) make assumptions about the underlying research data to produce explicit formulae for the objective target measures. Unlike these statistical methods, machine learning algorithms figure out patterns on their own directly from the data. For instance, for a non-linear but separable dataset, an OLS regression model will not be the right choice due to its assumption that the underlying data must be linear. However, a machine learning model can easily separate the dataset into the underlying classes. Figure 2 (a) presents a situation where machine learning models perform better than traditional statistical methods.
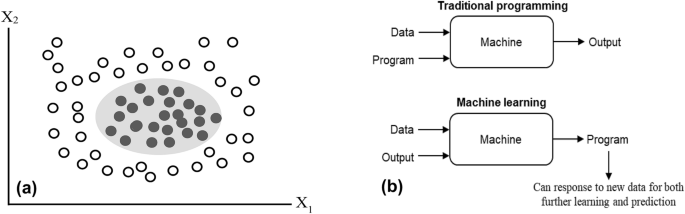
( a ) An illustration showing the superior performance of machine learning models compared with the traditional statistical models using an abstract dataset with two attributes (X 1 and X 2 ). The data points within this abstract dataset consist of two classes: one represented with a transparent circle and the second class illustrated with a black-filled circle. These data points are non-linear but separable. Traditional statistical models (e.g., ordinary least square regression) will not accurately separate these data points. However, any machine learning model can easily separate them without making errors; and ( b ) Traditional programming versus machine learning.
Similarly, machine learning models are compelling if the underlying research dataset has many attributes or independent measures. Such models can identify features that significantly contribute to the corresponding classification performance regardless of their distributions or collinearity. Traditional statistical methods have become prone to biased results when there exists a correlation between independent variables. Machine learning-based current studies specific to project analytics have been largely limited. Despite this, there have been tangential studies on the use of artificial intelligence to improve cost estimations as well as risk prediction 44 . Additionally, models have been implemented in the optimisation of existing processes 50 .
Machine learning versus traditional programming
Machine learning can be thought of as a process of teaching a machine (i.e., computers) to learn from data and adjust or apply its present knowledge when exposed to new data 42 . It is a type of artificial intelligence that enables computers to learn from examples or experiences. Traditional programming requires some input data and some logic in the form of code (program) to generate the output. Unlike traditional programming, the input data and their corresponding output are fed to an algorithm to create a program in machine learning. This resultant program can capture powerful insights into the data pattern and can be used to predict future outcomes. Figure 2 (b) shows the difference between machine learning and traditional programming.
Proposed machine learning-based framework
Figure 3 illustrates the proposed machine learning-based research framework of this study. The framework starts with breaking the project research dataset into the training and test components. As mentioned in the previous section, the research dataset may have many categorical and/or nominal independent variables, but its single dependent variable must be categorical. Although there is no strict rule for this split, the training data size is generally more than or equal to 50% of the original dataset 48 .
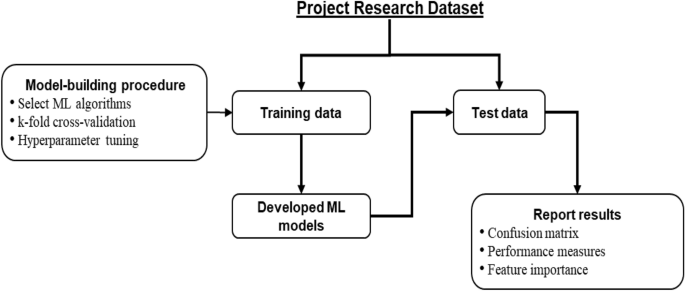
The proposed machine learning-based data-driven framework.
Machine learning algorithms can handle variables that have only numerical outcomes. So, when one or more of the underlying categorical variables have a textual or string outcome, we must first convert them into the corresponding numerical values. Suppose a variable can take only three textual outcomes (low, medium and high). In that case, we could consider, for example, 1 to represent low , 2 to represent medium , and 3 to represent high . Other statistical techniques, such as the RIDIT (relative to an identified distribution) scoring 51 , can also be used to convert ordered categorical measurements into quantitative ones. RIDIT is a parametric approach that uses probabilistic comparison to determine the statistical differences between ordered categorical groups. The remaining components of the proposed framework have been briefly described in the following subsections.
Model-building procedure
The next step of the framework is to follow the model-building procedure to develop the desired machine learning models using the training data. The first step of this procedure is to select suitable machine learning algorithms or models. Among the available machine learning algorithms, the commonly used ones are support vector machine, logistic regression, k -nearest neighbours, artificial neural network, decision tree and random forest 52 . One can also select an ensemble machine learning model as the desired algorithm. An ensemble machine learning method uses multiple algorithms or the same algorithm multiple times to achieve better predictive performance than could be obtained from any of the constituent learning models alone 52 . Three widely used ensemble approaches are bagging, boosting and stacking. In bagging, the research dataset is divided into different equal-sized subsets. The underlying machine learning algorithm is then applied to these subsets for classification. In boosting, a random sample of the dataset is selected and then fitted and trained sequentially with different models to compensate for the weakness observed in the immediately used model. Stacking combined different weak machine learning models in a heterogeneous way to improve the predictive performance. For example, the random forest algorithm is an ensemble of different decision tree models 42 .
Second, each selected machine learning model will be processed through the k -fold cross-validation approach to improve predictive efficiency. In k -fold cross-validation, the training data is divided into k folds. In an iteration, the (k-1) folds are used to train the selected machine models, and the remaining last fold isF used for validation purposes. This iteration process continues until each k folds will get a turn to be used for validation purposes. The final predictive efficiency of the trained models is based on the average values from the outcomes of these iterations. In addition to this average value, researchers use the standard deviation of the results from different iterations as the predictive training efficiency. Supplementary Fig 1 shows an illustration of the k -fold cross-validation.
Third, most machine learning algorithms require a pre-defined value for their different parameters, known as hyperparameter tuning. The settings of these parameters play a vital role in the achieved performance of the underlying algorithm. For a given machine learning algorithm, the optimal value for these parameters can be different from one dataset to another. The same algorithm needs to run multiple times with different parameter values to find its optimal parameter value for a given dataset. Many algorithms are available in the literature, such as the Grid search 53 , to find the optimal parameter value. In the Grid search, hyperparameters are divided into discrete grids. Each grid point represents a specific combination of the underlying model parameters. The parameter values of the point that results in the best performance are the optimal parameter values 53 .
Testing of the developed models and reporting results
Once the desired machine learning models have been developed using the training data, they need to be tested using the test data. The underlying trained model is then applied to predict its dependent variable for each data instance. Therefore, for each data instance, two categorical outcomes will be available for its dependent variable: one predicted using the underlying trained model, and the other is the actual category. These predicted and actual categorical outcome values are used to report the results of the underlying machine learning model.
The fundamental tool to report results from machine learning models is the confusion matrix, which consists of four integer values 48 . The first value represents the number of positive cases correctly identified as positive by the underlying trained model (true-positive). The second value indicates the number of positive instances incorrectly identified as negative (false-negative). The third value represents the number of negative cases incorrectly identified as positive (false-positive). Finally, the fourth value indicates the number of negative instances correctly identified as negative (true-negative). Researchers also use a few performance measures based on the four values of the confusion matrix to report machine learning results. The most used measure is accuracy which is the ratio of the number of correct predictions (true-positive + true-negative) and the total number of data instances (sum of all four values of the confusion matrix). Other measures commonly used to report machine learning results are precision, recall and F1-score. Precision refers to the ratio between true-positives and the total number of positive predictions (i.e., true-positive + false-positive), often used to indicate the quality of a positive prediction made by a model 48 . Recall, also known as the true-positive rate, is calculated by dividing true-positive by the number of data instances that should have been predicted as positive (i.e., true-positive + false-negative). F1-score is the harmonic mean of the last two measures, i.e., [(2 × Precision × Recall)/(Precision + Recall)] and the error-rate equals to (1-Accuracy).
Another essential tool for reporting machine learning results is variable or feature importance, which identifies a list of independent variables (features) contributing most to the classification performance. The importance of a variable refers to how much a given machine learning algorithm uses that variable in making accurate predictions 54 . The widely used technique for identifying variable importance is the principal component analysis. It reduces the dimensionality of the data while minimising information loss, which eventually increases the interpretability of the underlying machine learning outcome. It further helps in finding the important features in a dataset as well as plotting them in 2D and 3D 54 .
Ethical approval
Ethical approval is not required for this study since this study used publicly available data for research investigation purposes. All research was performed in accordance with relevant guidelines/regulations.
Informed consent
Due to the nature of the data sources, informed consent was not required for this study.
Case study: an application of the proposed framework
This section illustrates an application of this study’s proposed framework (Fig. 2 ) in a construction project context. We will apply this framework in classifying projects into two classes based on their cost overrun experience. Projects rarely experience a delay belonging to the first class (Rare class). The second class indicates those projects that often experience a delay (Often class). In doing so, we consider a list of independent variables or features.
Data source
The research dataset is taken from an open-source data repository, Kaggle 55 . This survey-based research dataset was collected to explore the causes of the project cost overrun in Indian construction projects 45 , consisting of 44 independent variables or features and one dependent variable. The independent variables cover a wide range of cost overrun factors, from materials and labour to contractual issues and the scope of the work. The dependent variable is the frequency of experiencing project cost overrun (rare or often). The dataset size is 139; 65 belong to the rare class, and the remaining 74 are from the often class. We converted each categorical variable with a textual or string outcome into an appropriate numerical value range to prepare the dataset for machine learning analysis. For example, we used 1 and 2 to represent rare and often class, respectively. The correlation matrix among the 44 features is presented in Supplementary Fig 2 .
Machine learning algorithms
This study considered four machine learning algorithms to explore the causes of project cost overrun using the research dataset mentioned above. They are support vector machine, logistic regression, k- nearest neighbours and random forest.
Support vector machine (SVM) is a process applied to understand data. For instance, if one wants to determine and interpret which projects are classified as programmatically successful through the processing of precedent data information, SVM would provide a practical approach for prediction. SVM functions by assigning labels to objects 56 . The comparison attributes are used to cluster these objects into different groups or classes by maximising their marginal distances and minimising the classification errors. The attributes are plotted multi-dimensionally, allowing a separation line, known as a hyperplane , see supplementary Fig 3 (a), to distinguish between underlying classes or groups 52 . Support vectors are the data points that lie closest to the decision boundary on both sides. In Supplementary Fig 3 (a), they are the circles (both transparent and shaded ones) close to the hyperplane. Support vectors play an essential role in deciding the position and orientation of the hyperplane. Various computational methods, including a kernel function to create more derived attributes, are applied to accommodate this process 56 . Support vector machines are not only limited to binary classes but can also be generalised to a larger variety of classifications. This is accomplished through the training of separate SVMs 56 .
Logistic regression (LR) builds on the linear regression model and predicts the outcome of a dichotomous variable 57 ; for example, the presence or absence of an event. It uses a scatterplot to understand the connection between an independent variable and one or more dependent variables (see Supplementary Fig 3 (b)). LR model fits the data to a sigmoidal curve instead of fitting it to a straight line. The natural logarithm is considered when developing the model. It provides a value between 0 and 1 that is interpreted as the probability of class membership. Best estimates are determined by developing from approximate estimates until a level of stability is reached 58 . Generally, LR offers a straightforward approach for determining and observing interrelationships. It is more efficient compared to ordinary regressions 59 .
k -nearest neighbours (KNN) algorithm uses a process that plots prior information and applies a specific sample size ( k ) to the plot to determine the most likely scenario 52 . This method finds the nearest training examples using a distance measure. The final classification is made by counting the most common scenario or votes present within the specified sample. As illustrated in Supplementary Fig 3 (c), the closest four nearest neighbours in the small circle are three grey squares and one white square. The majority class is grey. Hence, KNN will predict the instance (i.e., Χ ) as grey. On the other hand, if we look at the larger circle of the same figure, the nearest neighbours consist of ten white squares and four grey squares. The majority class is white. Thus, KNN will classify the instance as white. KNN’s advantage lies in its ability to produce a simplified result and handle missing data 60 . In summary, KNN utilises similarities (as well as differences) and distances in the process of developing models.
Random forest (RF) is a machine learning process that consists of many decision trees. A decision tree is a tree-like structure where each internal node represents a test on the input attribute. It may have multiple internal nodes at different levels, and the leaf or terminal nodes represent the decision outcomes. It produces a classification outcome for a distinctive and separate part to the input vector. For non-numerical processes, it considers the average value, and for discrete processes, it considers the number of votes 52 . Supplementary Fig 3 (d) shows three decision trees to illustrate the function of a random forest. The outcomes from trees 1, 2 and 3 are class B, class A and class A, respectively. According to the majority vote, the final prediction will be class A. Because it considers specific attributes, it can have a tendency to emphasise specific attributes over others, which may result in some attributes being unevenly weighted 52 . Advantages of the random forest include its ability to handle multidimensionality and multicollinearity in data despite its sensitivity to sampling design.
Artificial neural network (ANN) simulates the way in which human brains work. This is accomplished by modelling logical propositions and incorporating weighted inputs, a transfer and one output 61 (Supplementary Fig 3 (e)). It is advantageous because it can be used to model non-linear relationships and handle multivariate data 62 . ANN learns through three major avenues. These include error-back propagation (supervised), the Kohonen (unsupervised) and the counter-propagation ANN (supervised) 62 . There are two types of ANN—supervised and unsupervised. ANN has been used in a myriad of applications ranging from pharmaceuticals 61 to electronic devices 63 . It also possesses great levels of fault tolerance 64 and learns by example and through self-organisation 65 .
Ensemble techniques are a type of machine learning methodology in which numerous basic classifiers are combined to generate an optimal model 66 . An ensemble technique considers many models and combines them to form a single model, and the final model will eliminate the weaknesses of each individual learner, resulting in a powerful model that will improve model performance. The stacking model is a general architecture comprised of two classifier levels: base classifier and meta-learner 67 . The base classifiers are trained with the training dataset, and a new dataset is constructed for the meta-learner. Afterwards, this new dataset is used to train the meta-classifier. This study uses four models (SVM, LR, KNN and RF) as base classifiers and LR as a meta learner, as illustrated in Supplementary Fig 3 (f).
Feature selection
The process of selecting the optimal feature subset that significantly influences the predicted outcomes, which may be efficient to increase model performance and save running time, is known as feature selection. This study considers three different feature selection approaches. They are the Univariate feature selection (UFS), Recursive feature elimination (RFE) and SelectFromModel (SFM) approach. UFS examines each feature separately to determine the strength of its relationship with the response variable 68 . This method is straightforward to use and comprehend and helps acquire a deeper understanding of data. In this study, we calculate the chi-square values between features. RFE is a type of backwards feature elimination in which the model is fit first using all features in the given dataset and then removing the least important features one by one 69 . After that, the model is refit until the desired number of features is left over, which is determined by the parameter. SFM is used to choose effective features based on the feature importance of the best-performing model 70 . This approach selects features by establishing a threshold based on feature significance as indicated by the model on the training set. Those characteristics whose feature importance is more than the threshold are chosen, while those whose feature importance is less than the threshold are deleted. In this study, we apply SFM after we compare the performance of four machine learning methods. Afterwards, we train the best-performing model again using the features from the SFM approach.
Findings from the case study
We split the dataset into 70:30 for training and test purposes of the four selected machine learning algorithms. We used Python’s Scikit-learn package for implementing these algorithms 70 . Using the training data, we first developed six models based on these six algorithms. We used fivefold validation and target to improve the accuracy value. Then, we applied these models to the test data. We also executed all required hyperparameter tunings for each algorithm for the possible best classification outcome. Table 1 shows the performance outcomes for each algorithm during the training and test phase. The hyperparameter settings for each algorithm have been listed in Supplementary Table 1 .
As revealed in Table 1 , random forest outperformed the other three algorithms in terms of accuracy for both the training and test phases. It showed an accuracy of 78.14% and 77.50% for the training and test phases, respectively. The second-best performer in the training phase is k- nearest neighbours (76.98%), and for the test phase, it is the support vector machine, k- nearest neighbours and artificial neural network (72.50%).
Since random forest showed the best performance, we explored further based on this algorithm. We applied the three approaches (UFS, RFE and SFM) for feature optimisation on the random forest. The result is presented in Table 2 . SFM shows the best outcome among these three approaches. Its accuracy is 85.00%, whereas the accuracies of USF and RFE are 77.50% and 72.50%, respectively. As can be seen in Table 2 , the accuracy for the testing phase increases from 77.50% in Table 1 (b) to 85.00% with the SFM feature optimisation. Table 3 shows the 19 selected features from the SFM output. Out of 44 features, SFM found that 19 of them play a significant role in predicting the outcomes.
Further, Fig. 4 illustrates the confusion matrix when the random forest model with the SFM feature optimiser was applied to the test data. There are 18 true-positive, five false-negative, one false-positive and 16 true-negative cases. Therefore, the accuracy for the test phase is (18 + 16)/(18 + 5 + 1 + 16) = 85.00%.
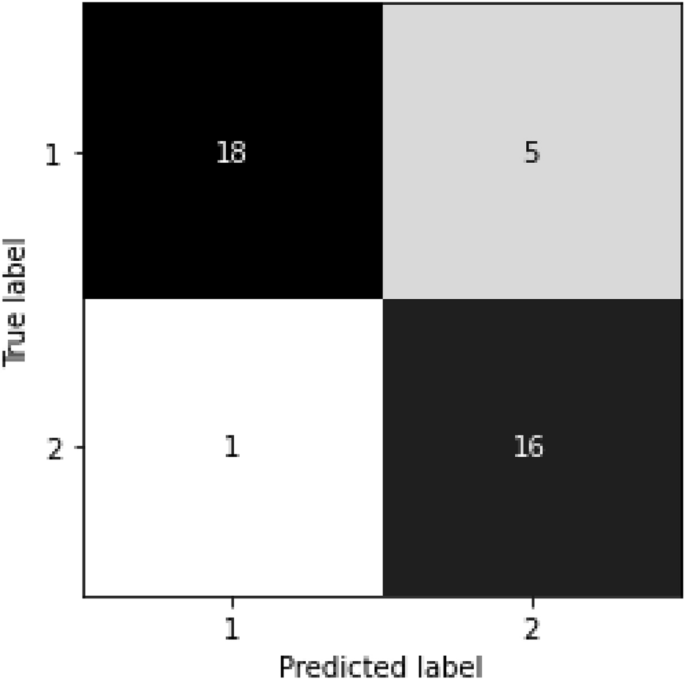
Confusion matrix results based on the random forest model with the SFM feature optimiser (1 for the rare class and 2 for the often class).
Figure 5 illustrates the top-10 most important features or variables based on the random forest algorithm with the SFM optimiser. We used feature importance based on the mean decrease in impurity in identifying this list of important variables. Mean decrease in impurity computes each feature’s importance as the sum over the number of splits that include the feature in proportion to the number of samples it splits 71 . According to this figure, the delays in decision marking attribute contributed most to the classification performance of the random forest algorithm, followed by cash flow problem and construction cost underestimation attributes. The current construction project literature also highlighted these top-10 factors as significant contributors to project cost overrun. For example, using construction project data from Jordan, Al-Hazim et al. 72 ranked 20 causes for cost overrun, including causes similar to these causes.
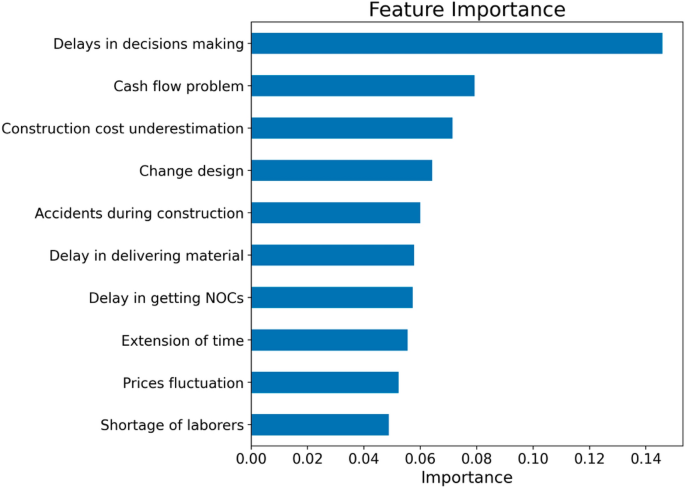
Feature importance (top-10 out of 19) based on the random forest model with the SFM feature optimiser.
Further, we conduct a sensitivity analysis of the model’s ten most important features (from Fig. 5 ) to explore how a change in each feature affects the cost overrun. We utilise the partial dependence plot (PDP), which is a typical visualisation tool for non-parametric models 73 , to display this analysis’s outcomes. A PDP can demonstrate whether the relation between the target and a feature is linear, monotonic, or more complicated. The result of the sensitivity analysis is presented in Fig. 6 . For the ‘delays in decisions making’ attribute, the PDP shows that the probability is below 0.4 until the rating value is three and increases after. A higher value for this attribute indicates a higher risk of cost overrun. On the other hand, there are no significant differences can be seen in the remaining nine features if the value changes.
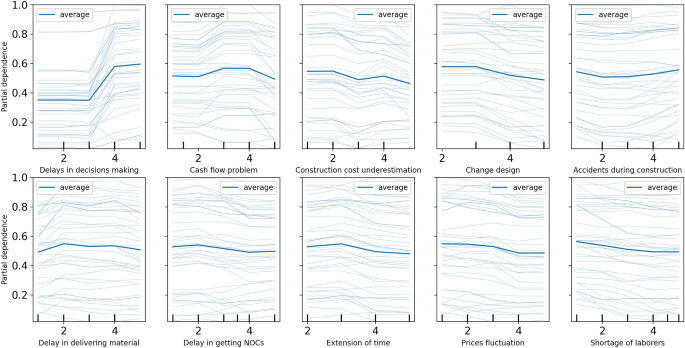
The result of the sensitivity analysis from the partial dependency plot tool for the ten most important features.
Summary of the case study
We illustrated an application of the proposed machine learning-based research framework in classifying construction projects. RF showed the highest accuracy in predicting the test dataset. For a new data instance with information for its 19 features but has not had any information on its classification, RF can identify its class ( rare or often ) correctly with a probability of 85.00%. If more data is provided, in addition to the 139 instances of the case study, to the machine learning algorithms, then their accuracy and efficiency in making project classification will improve with subsequent training. For example, if we provide 100 more data instances, these algorithms will have an additional 50 instances for training with a 70:30 split. This continuous improvement facility put the machine learning algorithms in a superior position over other traditional methods. In the current literature, some studies explore the factors contributing to project delay or cost overrun. In most cases, they applied factor analysis or other related statistical methods for research data analysis 72 , 74 , 75 . In addition to identifying important attributes, the proposed machine learning-based framework identified the ranking of factors and how eliminating less important factors affects the prediction accuracy when applied to this case study.
We shared the Python software developed to implement the four machine learning algorithms considered in this case study using GitHub 76 , a software hosting internet site. user-friendly version of this software can be accessed at https://share.streamlit.io/haohuilu/pa/main/app.py . The accuracy findings from this link could be slightly different from one run to another due to the hyperparameter settings of the corresponding machine learning algorithms.
Due to their robust prediction ability, machine learning methods have already gained wide acceptability across a wide range of research domains. On the other side, EVM is the most commonly used method in project analytics due to its simplicity and ease of interpretability 77 . Essential research efforts have been made to improve its generalisability over time. For example, Naeni et al. 34 developed a fuzzy approach for earned value analysis to make it suitable to analyse project scenarios with ambiguous or linguistic outcomes. Acebes 78 integrated Monte Carlo simulation with EVM for project monitoring and control for a similar purpose. Another prominent method frequently used in project analytics is the time series analysis, which is compelling for the longitudinal prediction of project time and cost 30 . Apparently, as evident in the present current literature, not much effort has been made to bring machine learning into project analytics for addressing project management research problems. This research made a significant attempt to contribute to filling up this gap.
Our proposed data-driven framework only includes the fundamental model development and application process components for machine learning algorithms. It does not have a few advanced-level machine learning methods. This study intentionally did not consider them for the proposed model since they are required only in particular designs of machine learning analysis. For example, the framework does not contain any methods or tools to handle the data imbalance issue. Data imbalance refers to a situation when the research dataset has an uneven distribution of the target class 79 . For example, a binary target variable will cause a data imbalance issue if one of its class labels has a very high number of observations compared with the other class. Commonly used techniques to address this issue are undersampling and oversampling. The undersampling technique decreases the size of the majority class. On the other hand, the oversampling technique randomly duplicates the minority class until the class distribution becomes balanced 79 . The class distribution of the case study did not produce any data imbalance issues.
This study considered only six fundamental machine learning algorithms for the case study, although many other such algorithms are available in the literature. For example, it did not consider the extreme gradient boosting (XGBoost) algorithm. XGBoost is based on the decision tree algorithm, similar to the random forest algorithm 80 . It has become dominant in applied machine learning due to its performance and speed. Naïve Bayes and convolutional neural networks are other popular machine learning algorithms that were not considered when applying the proposed framework to the case study. In addition to the three feature selection methods, multi-view can be adopted when applying the proposed framework to the case study. Multi-view learning is another direction in machine learning that considers learning with multiple views of the existing data with the aim to improve predictive performance 81 , 82 . Similarly, although we considered five performance measures, there are other potential candidates. One such example is the area under the receiver operating curve, which is the ability of the underlying classifier to distinguish between classes 48 . We leave them as a potential application scope while applying our proposed framework in any other project contexts in future studies.
Although this study only used one case study for illustration, our proposed research framework can be used in other project analytics contexts. In such an application context, the underlying research goal should be to predict the outcome classes and find attributes playing a significant role in making correct predictions. For example, by considering two types of projects based on the time required to accomplish (e.g., on-time and delayed ), the proposed framework can develop machine learning models that can predict the class of a new data instance and find out attributes contributing mainly to this prediction performance. This framework can also be used at any stage of the project. For example, the framework’s results allow project stakeholders to screen projects for excessive cost overruns and forecast budget loss at bidding and before contracts are signed. In addition, various factors that contribute to project cost overruns can be figured out at an earlier stage. These elements emerge at each stage of a project’s life cycle. The framework’s feature importance helps project managers locate the critical contributor to cost overrun.
This study has made an important contribution to the current project analytics literature by considering the applications of machine learning within project management. Project management is often thought of as being very fluid in nature, and because of this, applications of machine learning are often more difficult. Further, existing implementations have largely been limited to safety monitoring, risk prediction and cost estimation. Through the evaluation of machine learning applications, this study further demonstrates the uses for which algorithms can be used to consider and model the relationship between project attributes and cost overrun frequency.
The applications of machine learning in project analytics are still undergoing constant development. Within construction projects, its applications have been largely limited and focused on profitability or the design of structures themselves. In this regard, our study made a substantial effort by proposing a machine learning-based framework to address research problems related to project analytics. We also illustrated an example of this framework’s application in the context of construction project management.
Like any other research, this study also has a few limitations that could provide scopes for future research. First, the framework does not include a few advanced machine learning techniques, such as data imbalance issues and kernel density estimation. Second, we considered only one case study to illustrate the application of the proposed framework. Illustrations of this framework using case studies from different project contexts would confirm its robust application. Finally, this study did not consider all machine learning models and performance measures available in the literature for the case study. For example, we did not consider the Naïve Bayes model and precision measure in applying the proposed research framework for the case study.
Data availability
This study obtained research data from publicly available online repositories. We mentioned their sources using proper citations. Here is the link to the data https://www.kaggle.com/datasets/amansaxena/survey-on-road-construction-delay .
Venkrbec, V. & Klanšek, U. In: Advances and Trends in Engineering Sciences and Technologies II 685–690 (CRC Press, 2016).
Google Scholar
Damnjanovic, I. & Reinschmidt, K. Data Analytics for Engineering and Construction Project Risk Management (Springer, 2020).
Book Google Scholar
Singh, H. Project Management Analytics: A Data-driven Approach to Making Rational and Effective Project Decisions (FT Press, 2015).
Frame, J. D. & Chen, Y. Why Data Analytics in Project Management? (Auerbach Publications, 2018).
Ong, S. & Uddin, S. Data Science and Artificial Intelligence in Project Management: The Past, Present and Future. J. Mod. Proj. Manag. 7 , 26–33 (2020).
Bilal, M. et al. Investigating profitability performance of construction projects using big data: A project analytics approach. J. Build. Eng. 26 , 100850 (2019).
Article Google Scholar
Radziszewska-Zielina, E. & Sroka, B. Planning repetitive construction projects considering technological constraints. Open Eng. 8 , 500–505 (2018).
Neely, A. D., Adams, C. & Kennerley, M. The Performance Prism: The Scorecard for Measuring and Managing Business Success (Prentice Hall Financial Times, 2002).
Kanakaris, N., Karacapilidis, N., Kournetas, G. & Lazanas, A. In: International Conference on Operations Research and Enterprise Systems. 135–155 Springer.
Jordan, M. I. & Mitchell, T. M. Machine learning: Trends, perspectives, and prospects. Science 349 , 255–260 (2015).
Article ADS MathSciNet CAS PubMed MATH Google Scholar
Shalev-Shwartz, S. & Ben-David, S. Understanding Machine Learning: From Theory to Algorithms (Cambridge University Press, 2014).
Book MATH Google Scholar
Rahimian, F. P., Seyedzadeh, S., Oliver, S., Rodriguez, S. & Dawood, N. On-demand monitoring of construction projects through a game-like hybrid application of BIM and machine learning. Autom. Constr. 110 , 103012 (2020).
Sanni-Anibire, M. O., Zin, R. M. & Olatunji, S. O. Machine learning model for delay risk assessment in tall building projects. Int. J. Constr. Manag. 22 , 1–10 (2020).
Cong, J. et al. A machine learning-based iterative design approach to automate user satisfaction degree prediction in smart product-service system. Comput. Ind. Eng. 165 , 107939 (2022).
Li, F., Chen, C.-H., Lee, C.-H. & Feng, S. Artificial intelligence-enabled non-intrusive vigilance assessment approach to reducing traffic controller’s human errors. Knowl. Based Syst. 239 , 108047 (2021).
Mohri, M., Rostamizadeh, A. & Talwalkar, A. Foundations of Machine Learning (MIT press, 2018).
MATH Google Scholar
Whyte, J., Stasis, A. & Lindkvist, C. Managing change in the delivery of complex projects: Configuration management, asset information and ‘big data’. Int. J. Proj. Manag. 34 , 339–351 (2016).
Zangeneh, P. & McCabe, B. Ontology-based knowledge representation for industrial megaprojects analytics using linked data and the semantic web. Adv. Eng. Inform. 46 , 101164 (2020).
Akinosho, T. D. et al. Deep learning in the construction industry: A review of present status and future innovations. J. Build. Eng. 32 , 101827 (2020).
Soman, R. K., Molina-Solana, M. & Whyte, J. K. Linked-Data based constraint-checking (LDCC) to support look-ahead planning in construction. Autom. Constr. 120 , 103369 (2020).
Soman, R. K. & Whyte, J. K. Codification challenges for data science in construction. J. Constr. Eng. Manag. 146 , 04020072 (2020).
Soman, R. K. & Molina-Solana, M. Automating look-ahead schedule generation for construction using linked-data based constraint checking and reinforcement learning. Autom. Constr. 134 , 104069 (2022).
Shi, F., Soman, R. K., Han, J. & Whyte, J. K. Addressing adjacency constraints in rectangular floor plans using Monte-Carlo tree search. Autom. Constr. 115 , 103187 (2020).
Chen, L. & Whyte, J. Understanding design change propagation in complex engineering systems using a digital twin and design structure matrix. Eng. Constr. Archit. Manag. (2021).
Allison, J. T. et al. Artificial intelligence and engineering design. J. Mech. Des. 144 , 020301 (2022).
Dutta, D. & Bose, I. Managing a big data project: The case of ramco cements limited. Int. J. Prod. Econ. 165 , 293–306 (2015).
Bilal, M. & Oyedele, L. O. Guidelines for applied machine learning in construction industry—A case of profit margins estimation. Adv. Eng. Inform. 43 , 101013 (2020).
Tayefeh Hashemi, S., Ebadati, O. M. & Kaur, H. Cost estimation and prediction in construction projects: A systematic review on machine learning techniques. SN Appl. Sci. 2 , 1–27 (2020).
Arage, S. S. & Dharwadkar, N. V. In: International Conference on I-SMAC (IoT in Social, Mobile, Analytics and Cloud)(I-SMAC). 594–599 (IEEE, 2017).
Cheng, C.-H., Chang, J.-R. & Yeh, C.-A. Entropy-based and trapezoid fuzzification-based fuzzy time series approaches for forecasting IT project cost. Technol. Forecast. Soc. Chang. 73 , 524–542 (2006).
Joukar, A. & Nahmens, I. Volatility forecast of construction cost index using general autoregressive conditional heteroskedastic method. J. Constr. Eng. Manag. 142 , 04015051 (2016).
Xu, J.-W. & Moon, S. Stochastic forecast of construction cost index using a cointegrated vector autoregression model. J. Manag. Eng. 29 , 10–18 (2013).
Narbaev, T. & De Marco, A. Combination of growth model and earned schedule to forecast project cost at completion. J. Constr. Eng. Manag. 140 , 04013038 (2014).
Naeni, L. M., Shadrokh, S. & Salehipour, A. A fuzzy approach for the earned value management. Int. J. Proj. Manag. 29 , 764–772 (2011).
Ponz-Tienda, J. L., Pellicer, E. & Yepes, V. Complete fuzzy scheduling and fuzzy earned value management in construction projects. J. Zhejiang Univ. Sci. A 13 , 56–68 (2012).
Yu, F., Chen, X., Cory, C. A., Yang, Z. & Hu, Y. An active construction dynamic schedule management model: Using the fuzzy earned value management and BP neural network. KSCE J. Civ. Eng. 25 , 2335–2349 (2021).
Bonato, F. K., Albuquerque, A. A. & Paixão, M. A. S. An application of earned value management (EVM) with Monte Carlo simulation in engineering project management. Gest. Produção 26 , e4641 (2019).
Batselier, J. & Vanhoucke, M. Empirical evaluation of earned value management forecasting accuracy for time and cost. J. Constr. Eng. Manag. 141 , 05015010 (2015).
Yang, R. J. & Zou, P. X. Stakeholder-associated risks and their interactions in complex green building projects: A social network model. Build. Environ. 73 , 208–222 (2014).
Uddin, S. Social network analysis in project management–A case study of analysing stakeholder networks. J. Mod. Proj. Manag. 5 , 106–113 (2017).
Ong, S. & Uddin, S. Co-evolution of project stakeholder networks. J. Mod. Proj. Manag. 8 , 96–115 (2020).
Khanzode, K. C. A. & Sarode, R. D. Advantages and disadvantages of artificial intelligence and machine learning: A literature review. Int. J. Libr. Inf. Sci. (IJLIS) 9 , 30–36 (2020).
Loyola-Gonzalez, O. Black-box vs. white-box: Understanding their advantages and weaknesses from a practical point of view. IEEE Access 7 , 154096–154113 (2019).
Abioye, S. O. et al. Artificial intelligence in the construction industry: A review of present status, opportunities and future challenges. J. Build. Eng. 44 , 103299 (2021).
Doloi, H., Sawhney, A., Iyer, K. & Rentala, S. Analysing factors affecting delays in Indian construction projects. Int. J. Proj. Manag. 30 , 479–489 (2012).
Alkhaddar, R., Wooder, T., Sertyesilisik, B. & Tunstall, A. Deep learning approach’s effectiveness on sustainability improvement in the UK construction industry. Manag. Environ. Qual. Int. J. 23 , 126–139 (2012).
Gondia, A., Siam, A., El-Dakhakhni, W. & Nassar, A. H. Machine learning algorithms for construction projects delay risk prediction. J. Constr. Eng. Manag. 146 , 04019085 (2020).
Witten, I. H. & Frank, E. Data Mining: Practical Machine Learning Tools and Techniques (Morgan Kaufmann, 2005).
Kanakaris, N., Karacapilidis, N. I. & Lazanas, A. In: ICORES. 362–369.
Heo, S., Han, S., Shin, Y. & Na, S. Challenges of data refining process during the artificial intelligence development projects in the architecture engineering and construction industry. Appl. Sci. 11 , 10919 (2021).
Article CAS Google Scholar
Bross, I. D. How to use ridit analysis. Biometrics 14 , 18–38 (1958).
Uddin, S., Khan, A., Hossain, M. E. & Moni, M. A. Comparing different supervised machine learning algorithms for disease prediction. BMC Med. Inform. Decis. Mak. 19 , 1–16 (2019).
LaValle, S. M., Branicky, M. S. & Lindemann, S. R. On the relationship between classical grid search and probabilistic roadmaps. Int. J. Robot. Res. 23 , 673–692 (2004).
Abdi, H. & Williams, L. J. Principal component analysis. Wiley Interdiscip. Rev. Comput. Stat. 2 , 433–459 (2010).
Saxena, A. Survey on Road Construction Delay , https://www.kaggle.com/amansaxena/survey-on-road-construction-delay (2021).
Noble, W. S. What is a support vector machine?. Nat. Biotechnol. 24 , 1565–1567 (2006).
Article CAS PubMed Google Scholar
Hosmer, D. W. Jr., Lemeshow, S. & Sturdivant, R. X. Applied Logistic Regression Vol. 398 (John Wiley & Sons, 2013).
LaValley, M. P. Logistic regression. Circulation 117 , 2395–2399 (2008).
Article PubMed Google Scholar
Menard, S. Applied Logistic Regression Analysis Vol. 106 (Sage, 2002).
Batista, G. E. & Monard, M. C. A study of K-nearest neighbour as an imputation method. His 87 , 48 (2002).
Agatonovic-Kustrin, S. & Beresford, R. Basic concepts of artificial neural network (ANN) modeling and its application in pharmaceutical research. J. Pharm. Biomed. Anal. 22 , 717–727 (2000).
Zupan, J. Introduction to artificial neural network (ANN) methods: What they are and how to use them. Acta Chim. Slov. 41 , 327–327 (1994).
CAS Google Scholar
Hopfield, J. J. Artificial neural networks. IEEE Circuits Devices Mag. 4 , 3–10 (1988).
Zou, J., Han, Y. & So, S.-S. Overview of artificial neural networks. Artificial Neural Networks . 14–22 (2008).
Maind, S. B. & Wankar, P. Research paper on basic of artificial neural network. Int. J. Recent Innov. Trends Comput. Commun. 2 , 96–100 (2014).
Wolpert, D. H. Stacked generalization. Neural Netw. 5 , 241–259 (1992).
Pavlyshenko, B. In: IEEE Second International Conference on Data Stream Mining & Processing (DSMP). 255–258 (IEEE).
Jović, A., Brkić, K. & Bogunović, N. In: 38th International Convention on Information and Communication Technology, Electronics and Microelectronics (MIPRO). 1200–1205 (Ieee, 2015).
Guyon, I., Weston, J., Barnhill, S. & Vapnik, V. Gene selection for cancer classification using support vector machines. Mach. Learn. 46 , 389–422 (2002).
Article MATH Google Scholar
Pedregosa, F. et al. Scikit-learn: Machine learning in python. J. Mach. Learn. Res. 12 , 2825–2830 (2011).
MathSciNet MATH Google Scholar
Louppe, G., Wehenkel, L., Sutera, A. & Geurts, P. Understanding variable importances in forests of randomized trees. Adv. Neural. Inf. Process. Syst. 26 , 431–439 (2013).
Al-Hazim, N., Salem, Z. A. & Ahmad, H. Delay and cost overrun in infrastructure projects in Jordan. Procedia Eng. 182 , 18–24 (2017).
Breiman, L. Random forests. Mach. Learn. 45 , 5–32. https://doi.org/10.1023/A:1010933404324 (2001).
Shehu, Z., Endut, I. R. & Akintoye, A. Factors contributing to project time and hence cost overrun in the Malaysian construction industry. J. Financ. Manag. Prop. Constr. 19 , 55–75 (2014).
Akomah, B. B. & Jackson, E. N. Contractors’ perception of factors contributing to road project delay. Int. J. Constr. Eng. Manag. 5 , 79–85 (2016).
GitHub: Where the world builds software , https://github.com/ .
Anbari, F. T. Earned value project management method and extensions. Proj. Manag. J. 34 , 12–23 (2003).
Acebes, F., Pereda, M., Poza, D., Pajares, J. & Galán, J. M. Stochastic earned value analysis using Monte Carlo simulation and statistical learning techniques. Int. J. Proj. Manag. 33 , 1597–1609 (2015).
Japkowicz, N. & Stephen, S. The class imbalance problem: A systematic study. Intell. data anal. 6 , 429–449 (2002).
Chen, T. et al. Xgboost: extreme gradient boosting. R Packag. Version 0.4–2.1 1 , 1–4 (2015).
Guarino, A., Lettieri, N., Malandrino, D., Zaccagnino, R. & Capo, C. Adam or Eve? Automatic users’ gender classification via gestures analysis on touch devices. Neural Comput. Appl. 1–23 (2022).
Zaccagnino, R., Capo, C., Guarino, A., Lettieri, N. & Malandrino, D. Techno-regulation and intelligent safeguards. Multimed. Tools Appl. 80 , 15803–15824 (2021).
Download references
Acknowledgements
The authors acknowledge the insightful comments from Prof Jennifer Whyte on an earlier version of this article.
Author information
Authors and affiliations.
School of Project Management, The University of Sydney, Level 2, 21 Ross St, Forest Lodge, NSW, 2037, Australia
Shahadat Uddin, Stephen Ong & Haohui Lu
You can also search for this author in PubMed Google Scholar

Contributions
S.U.: Conceptualisation; Data curation; Formal analysis; Methodology; Supervision; and Writing (original draft, review and editing) S.O.: Data curation; and Writing (original draft, review and editing) H.L.: Methodology; and Writing (original draft, review and editing) All authors reviewed the manuscript).
Corresponding author
Correspondence to Shahadat Uddin .
Ethics declarations
Competing interests.
The authors declare no competing interests.
Additional information
Publisher's note.
Springer Nature remains neutral with regard to jurisdictional claims in published maps and institutional affiliations.
Supplementary Information
Supplementary information., rights and permissions.
Open Access This article is licensed under a Creative Commons Attribution 4.0 International License, which permits use, sharing, adaptation, distribution and reproduction in any medium or format, as long as you give appropriate credit to the original author(s) and the source, provide a link to the Creative Commons licence, and indicate if changes were made. The images or other third party material in this article are included in the article's Creative Commons licence, unless indicated otherwise in a credit line to the material. If material is not included in the article's Creative Commons licence and your intended use is not permitted by statutory regulation or exceeds the permitted use, you will need to obtain permission directly from the copyright holder. To view a copy of this licence, visit http://creativecommons.org/licenses/by/4.0/ .
Reprints and permissions
About this article
Cite this article.
Uddin, S., Ong, S. & Lu, H. Machine learning in project analytics: a data-driven framework and case study. Sci Rep 12 , 15252 (2022). https://doi.org/10.1038/s41598-022-19728-x
Download citation
Received : 13 April 2022
Accepted : 02 September 2022
Published : 09 September 2022
DOI : https://doi.org/10.1038/s41598-022-19728-x
Share this article
Anyone you share the following link with will be able to read this content:
Sorry, a shareable link is not currently available for this article.
Provided by the Springer Nature SharedIt content-sharing initiative
By submitting a comment you agree to abide by our Terms and Community Guidelines . If you find something abusive or that does not comply with our terms or guidelines please flag it as inappropriate.
Quick links
- Explore articles by subject
- Guide to authors
- Editorial policies
Sign up for the Nature Briefing: AI and Robotics newsletter — what matters in AI and robotics research, free to your inbox weekly.

The Forecasting and Case Study Modeling of COVID-19 in Chicago: A Data-driven Approach
- Oluwaseun T. Ajayi Illinois Institute of Technology
Probabilistic graphical models and machine learning are powerful data-driven tools for extracting useful knowledge from historical data; this knowledge can facilitate improved decision-making. With the prevailing efforts to combat the coronavirus disease 2019 (COVID-19) pandemic, there are still uncertainties that are yet to be discovered about its spread, future impact, and resurgence. In this paper, a data-driven approach has been adopted in distilling the hidden information about COVID-19 and its symptoms. This paper proposes: a Bayesian network which encodes the causal relationships among COVID-19 symptoms, an unsupervised machine learning algorithm that learns symptoms pattern in COVID-19 dataset, a deep neural network which predicts the symptoms class of patients based on clustering experience with a 99.47% testing accuracy, and a time-series forecasting model that predicts the trend of COVID-19. The results from the experiments show the capability of data-driven methods in addressing the concerns of the society and government in understanding the uncertainties about the virus, providing insights on developing policies, and reducing the spread of the virus.
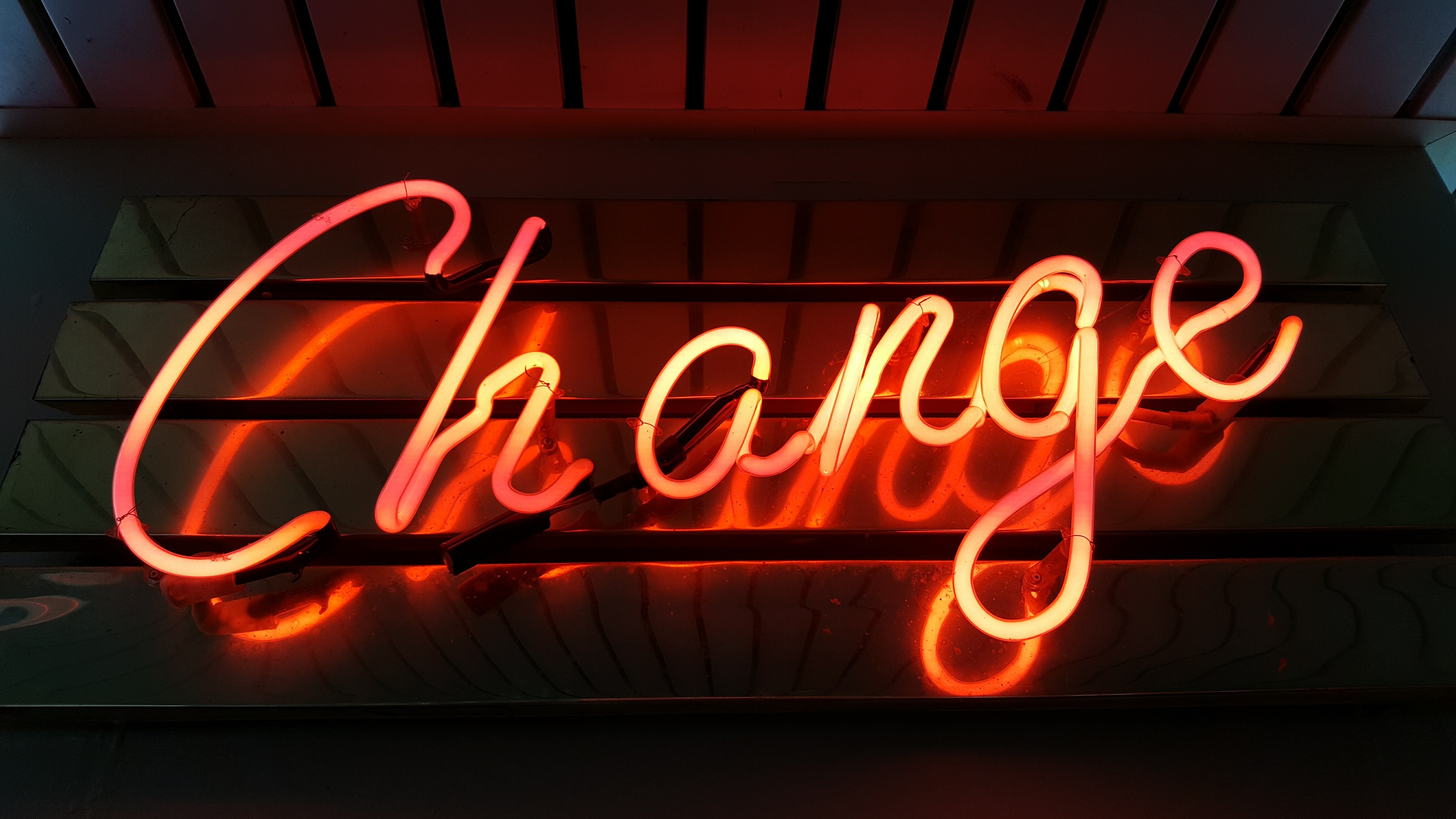
- Read online
Copyright (c) 2023 Oluwaseun Ajayi

This work is licensed under a Creative Commons Attribution-NonCommercial-NoDerivatives 4.0 International License .
How to Cite
- Endnote/Zotero/Mendeley (RIS)
Information
- For Readers
- For Authors
- For Librarians

- Open access
- Published: 27 June 2011
The case study approach
- Sarah Crowe 1 ,
- Kathrin Cresswell 2 ,
- Ann Robertson 2 ,
- Guro Huby 3 ,
- Anthony Avery 1 &
- Aziz Sheikh 2
BMC Medical Research Methodology volume 11 , Article number: 100 ( 2011 ) Cite this article
792k Accesses
1115 Citations
42 Altmetric
Metrics details
The case study approach allows in-depth, multi-faceted explorations of complex issues in their real-life settings. The value of the case study approach is well recognised in the fields of business, law and policy, but somewhat less so in health services research. Based on our experiences of conducting several health-related case studies, we reflect on the different types of case study design, the specific research questions this approach can help answer, the data sources that tend to be used, and the particular advantages and disadvantages of employing this methodological approach. The paper concludes with key pointers to aid those designing and appraising proposals for conducting case study research, and a checklist to help readers assess the quality of case study reports.
Peer Review reports
Introduction
The case study approach is particularly useful to employ when there is a need to obtain an in-depth appreciation of an issue, event or phenomenon of interest, in its natural real-life context. Our aim in writing this piece is to provide insights into when to consider employing this approach and an overview of key methodological considerations in relation to the design, planning, analysis, interpretation and reporting of case studies.
The illustrative 'grand round', 'case report' and 'case series' have a long tradition in clinical practice and research. Presenting detailed critiques, typically of one or more patients, aims to provide insights into aspects of the clinical case and, in doing so, illustrate broader lessons that may be learnt. In research, the conceptually-related case study approach can be used, for example, to describe in detail a patient's episode of care, explore professional attitudes to and experiences of a new policy initiative or service development or more generally to 'investigate contemporary phenomena within its real-life context' [ 1 ]. Based on our experiences of conducting a range of case studies, we reflect on when to consider using this approach, discuss the key steps involved and illustrate, with examples, some of the practical challenges of attaining an in-depth understanding of a 'case' as an integrated whole. In keeping with previously published work, we acknowledge the importance of theory to underpin the design, selection, conduct and interpretation of case studies[ 2 ]. In so doing, we make passing reference to the different epistemological approaches used in case study research by key theoreticians and methodologists in this field of enquiry.
This paper is structured around the following main questions: What is a case study? What are case studies used for? How are case studies conducted? What are the potential pitfalls and how can these be avoided? We draw in particular on four of our own recently published examples of case studies (see Tables 1 , 2 , 3 and 4 ) and those of others to illustrate our discussion[ 3 – 7 ].
What is a case study?
A case study is a research approach that is used to generate an in-depth, multi-faceted understanding of a complex issue in its real-life context. It is an established research design that is used extensively in a wide variety of disciplines, particularly in the social sciences. A case study can be defined in a variety of ways (Table 5 ), the central tenet being the need to explore an event or phenomenon in depth and in its natural context. It is for this reason sometimes referred to as a "naturalistic" design; this is in contrast to an "experimental" design (such as a randomised controlled trial) in which the investigator seeks to exert control over and manipulate the variable(s) of interest.
Stake's work has been particularly influential in defining the case study approach to scientific enquiry. He has helpfully characterised three main types of case study: intrinsic , instrumental and collective [ 8 ]. An intrinsic case study is typically undertaken to learn about a unique phenomenon. The researcher should define the uniqueness of the phenomenon, which distinguishes it from all others. In contrast, the instrumental case study uses a particular case (some of which may be better than others) to gain a broader appreciation of an issue or phenomenon. The collective case study involves studying multiple cases simultaneously or sequentially in an attempt to generate a still broader appreciation of a particular issue.
These are however not necessarily mutually exclusive categories. In the first of our examples (Table 1 ), we undertook an intrinsic case study to investigate the issue of recruitment of minority ethnic people into the specific context of asthma research studies, but it developed into a instrumental case study through seeking to understand the issue of recruitment of these marginalised populations more generally, generating a number of the findings that are potentially transferable to other disease contexts[ 3 ]. In contrast, the other three examples (see Tables 2 , 3 and 4 ) employed collective case study designs to study the introduction of workforce reconfiguration in primary care, the implementation of electronic health records into hospitals, and to understand the ways in which healthcare students learn about patient safety considerations[ 4 – 6 ]. Although our study focusing on the introduction of General Practitioners with Specialist Interests (Table 2 ) was explicitly collective in design (four contrasting primary care organisations were studied), is was also instrumental in that this particular professional group was studied as an exemplar of the more general phenomenon of workforce redesign[ 4 ].
What are case studies used for?
According to Yin, case studies can be used to explain, describe or explore events or phenomena in the everyday contexts in which they occur[ 1 ]. These can, for example, help to understand and explain causal links and pathways resulting from a new policy initiative or service development (see Tables 2 and 3 , for example)[ 1 ]. In contrast to experimental designs, which seek to test a specific hypothesis through deliberately manipulating the environment (like, for example, in a randomised controlled trial giving a new drug to randomly selected individuals and then comparing outcomes with controls),[ 9 ] the case study approach lends itself well to capturing information on more explanatory ' how ', 'what' and ' why ' questions, such as ' how is the intervention being implemented and received on the ground?'. The case study approach can offer additional insights into what gaps exist in its delivery or why one implementation strategy might be chosen over another. This in turn can help develop or refine theory, as shown in our study of the teaching of patient safety in undergraduate curricula (Table 4 )[ 6 , 10 ]. Key questions to consider when selecting the most appropriate study design are whether it is desirable or indeed possible to undertake a formal experimental investigation in which individuals and/or organisations are allocated to an intervention or control arm? Or whether the wish is to obtain a more naturalistic understanding of an issue? The former is ideally studied using a controlled experimental design, whereas the latter is more appropriately studied using a case study design.
Case studies may be approached in different ways depending on the epistemological standpoint of the researcher, that is, whether they take a critical (questioning one's own and others' assumptions), interpretivist (trying to understand individual and shared social meanings) or positivist approach (orientating towards the criteria of natural sciences, such as focusing on generalisability considerations) (Table 6 ). Whilst such a schema can be conceptually helpful, it may be appropriate to draw on more than one approach in any case study, particularly in the context of conducting health services research. Doolin has, for example, noted that in the context of undertaking interpretative case studies, researchers can usefully draw on a critical, reflective perspective which seeks to take into account the wider social and political environment that has shaped the case[ 11 ].
How are case studies conducted?
Here, we focus on the main stages of research activity when planning and undertaking a case study; the crucial stages are: defining the case; selecting the case(s); collecting and analysing the data; interpreting data; and reporting the findings.
Defining the case
Carefully formulated research question(s), informed by the existing literature and a prior appreciation of the theoretical issues and setting(s), are all important in appropriately and succinctly defining the case[ 8 , 12 ]. Crucially, each case should have a pre-defined boundary which clarifies the nature and time period covered by the case study (i.e. its scope, beginning and end), the relevant social group, organisation or geographical area of interest to the investigator, the types of evidence to be collected, and the priorities for data collection and analysis (see Table 7 )[ 1 ]. A theory driven approach to defining the case may help generate knowledge that is potentially transferable to a range of clinical contexts and behaviours; using theory is also likely to result in a more informed appreciation of, for example, how and why interventions have succeeded or failed[ 13 ].
For example, in our evaluation of the introduction of electronic health records in English hospitals (Table 3 ), we defined our cases as the NHS Trusts that were receiving the new technology[ 5 ]. Our focus was on how the technology was being implemented. However, if the primary research interest had been on the social and organisational dimensions of implementation, we might have defined our case differently as a grouping of healthcare professionals (e.g. doctors and/or nurses). The precise beginning and end of the case may however prove difficult to define. Pursuing this same example, when does the process of implementation and adoption of an electronic health record system really begin or end? Such judgements will inevitably be influenced by a range of factors, including the research question, theory of interest, the scope and richness of the gathered data and the resources available to the research team.
Selecting the case(s)
The decision on how to select the case(s) to study is a very important one that merits some reflection. In an intrinsic case study, the case is selected on its own merits[ 8 ]. The case is selected not because it is representative of other cases, but because of its uniqueness, which is of genuine interest to the researchers. This was, for example, the case in our study of the recruitment of minority ethnic participants into asthma research (Table 1 ) as our earlier work had demonstrated the marginalisation of minority ethnic people with asthma, despite evidence of disproportionate asthma morbidity[ 14 , 15 ]. In another example of an intrinsic case study, Hellstrom et al.[ 16 ] studied an elderly married couple living with dementia to explore how dementia had impacted on their understanding of home, their everyday life and their relationships.
For an instrumental case study, selecting a "typical" case can work well[ 8 ]. In contrast to the intrinsic case study, the particular case which is chosen is of less importance than selecting a case that allows the researcher to investigate an issue or phenomenon. For example, in order to gain an understanding of doctors' responses to health policy initiatives, Som undertook an instrumental case study interviewing clinicians who had a range of responsibilities for clinical governance in one NHS acute hospital trust[ 17 ]. Sampling a "deviant" or "atypical" case may however prove even more informative, potentially enabling the researcher to identify causal processes, generate hypotheses and develop theory.
In collective or multiple case studies, a number of cases are carefully selected. This offers the advantage of allowing comparisons to be made across several cases and/or replication. Choosing a "typical" case may enable the findings to be generalised to theory (i.e. analytical generalisation) or to test theory by replicating the findings in a second or even a third case (i.e. replication logic)[ 1 ]. Yin suggests two or three literal replications (i.e. predicting similar results) if the theory is straightforward and five or more if the theory is more subtle. However, critics might argue that selecting 'cases' in this way is insufficiently reflexive and ill-suited to the complexities of contemporary healthcare organisations.
The selected case study site(s) should allow the research team access to the group of individuals, the organisation, the processes or whatever else constitutes the chosen unit of analysis for the study. Access is therefore a central consideration; the researcher needs to come to know the case study site(s) well and to work cooperatively with them. Selected cases need to be not only interesting but also hospitable to the inquiry [ 8 ] if they are to be informative and answer the research question(s). Case study sites may also be pre-selected for the researcher, with decisions being influenced by key stakeholders. For example, our selection of case study sites in the evaluation of the implementation and adoption of electronic health record systems (see Table 3 ) was heavily influenced by NHS Connecting for Health, the government agency that was responsible for overseeing the National Programme for Information Technology (NPfIT)[ 5 ]. This prominent stakeholder had already selected the NHS sites (through a competitive bidding process) to be early adopters of the electronic health record systems and had negotiated contracts that detailed the deployment timelines.
It is also important to consider in advance the likely burden and risks associated with participation for those who (or the site(s) which) comprise the case study. Of particular importance is the obligation for the researcher to think through the ethical implications of the study (e.g. the risk of inadvertently breaching anonymity or confidentiality) and to ensure that potential participants/participating sites are provided with sufficient information to make an informed choice about joining the study. The outcome of providing this information might be that the emotive burden associated with participation, or the organisational disruption associated with supporting the fieldwork, is considered so high that the individuals or sites decide against participation.
In our example of evaluating implementations of electronic health record systems, given the restricted number of early adopter sites available to us, we sought purposively to select a diverse range of implementation cases among those that were available[ 5 ]. We chose a mixture of teaching, non-teaching and Foundation Trust hospitals, and examples of each of the three electronic health record systems procured centrally by the NPfIT. At one recruited site, it quickly became apparent that access was problematic because of competing demands on that organisation. Recognising the importance of full access and co-operative working for generating rich data, the research team decided not to pursue work at that site and instead to focus on other recruited sites.
Collecting the data
In order to develop a thorough understanding of the case, the case study approach usually involves the collection of multiple sources of evidence, using a range of quantitative (e.g. questionnaires, audits and analysis of routinely collected healthcare data) and more commonly qualitative techniques (e.g. interviews, focus groups and observations). The use of multiple sources of data (data triangulation) has been advocated as a way of increasing the internal validity of a study (i.e. the extent to which the method is appropriate to answer the research question)[ 8 , 18 – 21 ]. An underlying assumption is that data collected in different ways should lead to similar conclusions, and approaching the same issue from different angles can help develop a holistic picture of the phenomenon (Table 2 )[ 4 ].
Brazier and colleagues used a mixed-methods case study approach to investigate the impact of a cancer care programme[ 22 ]. Here, quantitative measures were collected with questionnaires before, and five months after, the start of the intervention which did not yield any statistically significant results. Qualitative interviews with patients however helped provide an insight into potentially beneficial process-related aspects of the programme, such as greater, perceived patient involvement in care. The authors reported how this case study approach provided a number of contextual factors likely to influence the effectiveness of the intervention and which were not likely to have been obtained from quantitative methods alone.
In collective or multiple case studies, data collection needs to be flexible enough to allow a detailed description of each individual case to be developed (e.g. the nature of different cancer care programmes), before considering the emerging similarities and differences in cross-case comparisons (e.g. to explore why one programme is more effective than another). It is important that data sources from different cases are, where possible, broadly comparable for this purpose even though they may vary in nature and depth.
Analysing, interpreting and reporting case studies
Making sense and offering a coherent interpretation of the typically disparate sources of data (whether qualitative alone or together with quantitative) is far from straightforward. Repeated reviewing and sorting of the voluminous and detail-rich data are integral to the process of analysis. In collective case studies, it is helpful to analyse data relating to the individual component cases first, before making comparisons across cases. Attention needs to be paid to variations within each case and, where relevant, the relationship between different causes, effects and outcomes[ 23 ]. Data will need to be organised and coded to allow the key issues, both derived from the literature and emerging from the dataset, to be easily retrieved at a later stage. An initial coding frame can help capture these issues and can be applied systematically to the whole dataset with the aid of a qualitative data analysis software package.
The Framework approach is a practical approach, comprising of five stages (familiarisation; identifying a thematic framework; indexing; charting; mapping and interpretation) , to managing and analysing large datasets particularly if time is limited, as was the case in our study of recruitment of South Asians into asthma research (Table 1 )[ 3 , 24 ]. Theoretical frameworks may also play an important role in integrating different sources of data and examining emerging themes. For example, we drew on a socio-technical framework to help explain the connections between different elements - technology; people; and the organisational settings within which they worked - in our study of the introduction of electronic health record systems (Table 3 )[ 5 ]. Our study of patient safety in undergraduate curricula drew on an evaluation-based approach to design and analysis, which emphasised the importance of the academic, organisational and practice contexts through which students learn (Table 4 )[ 6 ].
Case study findings can have implications both for theory development and theory testing. They may establish, strengthen or weaken historical explanations of a case and, in certain circumstances, allow theoretical (as opposed to statistical) generalisation beyond the particular cases studied[ 12 ]. These theoretical lenses should not, however, constitute a strait-jacket and the cases should not be "forced to fit" the particular theoretical framework that is being employed.
When reporting findings, it is important to provide the reader with enough contextual information to understand the processes that were followed and how the conclusions were reached. In a collective case study, researchers may choose to present the findings from individual cases separately before amalgamating across cases. Care must be taken to ensure the anonymity of both case sites and individual participants (if agreed in advance) by allocating appropriate codes or withholding descriptors. In the example given in Table 3 , we decided against providing detailed information on the NHS sites and individual participants in order to avoid the risk of inadvertent disclosure of identities[ 5 , 25 ].
What are the potential pitfalls and how can these be avoided?
The case study approach is, as with all research, not without its limitations. When investigating the formal and informal ways undergraduate students learn about patient safety (Table 4 ), for example, we rapidly accumulated a large quantity of data. The volume of data, together with the time restrictions in place, impacted on the depth of analysis that was possible within the available resources. This highlights a more general point of the importance of avoiding the temptation to collect as much data as possible; adequate time also needs to be set aside for data analysis and interpretation of what are often highly complex datasets.
Case study research has sometimes been criticised for lacking scientific rigour and providing little basis for generalisation (i.e. producing findings that may be transferable to other settings)[ 1 ]. There are several ways to address these concerns, including: the use of theoretical sampling (i.e. drawing on a particular conceptual framework); respondent validation (i.e. participants checking emerging findings and the researcher's interpretation, and providing an opinion as to whether they feel these are accurate); and transparency throughout the research process (see Table 8 )[ 8 , 18 – 21 , 23 , 26 ]. Transparency can be achieved by describing in detail the steps involved in case selection, data collection, the reasons for the particular methods chosen, and the researcher's background and level of involvement (i.e. being explicit about how the researcher has influenced data collection and interpretation). Seeking potential, alternative explanations, and being explicit about how interpretations and conclusions were reached, help readers to judge the trustworthiness of the case study report. Stake provides a critique checklist for a case study report (Table 9 )[ 8 ].
Conclusions
The case study approach allows, amongst other things, critical events, interventions, policy developments and programme-based service reforms to be studied in detail in a real-life context. It should therefore be considered when an experimental design is either inappropriate to answer the research questions posed or impossible to undertake. Considering the frequency with which implementations of innovations are now taking place in healthcare settings and how well the case study approach lends itself to in-depth, complex health service research, we believe this approach should be more widely considered by researchers. Though inherently challenging, the research case study can, if carefully conceptualised and thoughtfully undertaken and reported, yield powerful insights into many important aspects of health and healthcare delivery.
Yin RK: Case study research, design and method. 2009, London: Sage Publications Ltd., 4
Google Scholar
Keen J, Packwood T: Qualitative research; case study evaluation. BMJ. 1995, 311: 444-446.
Article CAS PubMed PubMed Central Google Scholar
Sheikh A, Halani L, Bhopal R, Netuveli G, Partridge M, Car J, et al: Facilitating the Recruitment of Minority Ethnic People into Research: Qualitative Case Study of South Asians and Asthma. PLoS Med. 2009, 6 (10): 1-11.
Article Google Scholar
Pinnock H, Huby G, Powell A, Kielmann T, Price D, Williams S, et al: The process of planning, development and implementation of a General Practitioner with a Special Interest service in Primary Care Organisations in England and Wales: a comparative prospective case study. Report for the National Co-ordinating Centre for NHS Service Delivery and Organisation R&D (NCCSDO). 2008, [ http://www.sdo.nihr.ac.uk/files/project/99-final-report.pdf ]
Robertson A, Cresswell K, Takian A, Petrakaki D, Crowe S, Cornford T, et al: Prospective evaluation of the implementation and adoption of NHS Connecting for Health's national electronic health record in secondary care in England: interim findings. BMJ. 2010, 41: c4564-
Pearson P, Steven A, Howe A, Sheikh A, Ashcroft D, Smith P, the Patient Safety Education Study Group: Learning about patient safety: organisational context and culture in the education of healthcare professionals. J Health Serv Res Policy. 2010, 15: 4-10. 10.1258/jhsrp.2009.009052.
Article PubMed Google Scholar
van Harten WH, Casparie TF, Fisscher OA: The evaluation of the introduction of a quality management system: a process-oriented case study in a large rehabilitation hospital. Health Policy. 2002, 60 (1): 17-37. 10.1016/S0168-8510(01)00187-7.
Stake RE: The art of case study research. 1995, London: Sage Publications Ltd.
Sheikh A, Smeeth L, Ashcroft R: Randomised controlled trials in primary care: scope and application. Br J Gen Pract. 2002, 52 (482): 746-51.
PubMed PubMed Central Google Scholar
King G, Keohane R, Verba S: Designing Social Inquiry. 1996, Princeton: Princeton University Press
Doolin B: Information technology as disciplinary technology: being critical in interpretative research on information systems. Journal of Information Technology. 1998, 13: 301-311. 10.1057/jit.1998.8.
George AL, Bennett A: Case studies and theory development in the social sciences. 2005, Cambridge, MA: MIT Press
Eccles M, the Improved Clinical Effectiveness through Behavioural Research Group (ICEBeRG): Designing theoretically-informed implementation interventions. Implementation Science. 2006, 1: 1-8. 10.1186/1748-5908-1-1.
Article PubMed Central Google Scholar
Netuveli G, Hurwitz B, Levy M, Fletcher M, Barnes G, Durham SR, Sheikh A: Ethnic variations in UK asthma frequency, morbidity, and health-service use: a systematic review and meta-analysis. Lancet. 2005, 365 (9456): 312-7.
Sheikh A, Panesar SS, Lasserson T, Netuveli G: Recruitment of ethnic minorities to asthma studies. Thorax. 2004, 59 (7): 634-
CAS PubMed PubMed Central Google Scholar
Hellström I, Nolan M, Lundh U: 'We do things together': A case study of 'couplehood' in dementia. Dementia. 2005, 4: 7-22. 10.1177/1471301205049188.
Som CV: Nothing seems to have changed, nothing seems to be changing and perhaps nothing will change in the NHS: doctors' response to clinical governance. International Journal of Public Sector Management. 2005, 18: 463-477. 10.1108/09513550510608903.
Lincoln Y, Guba E: Naturalistic inquiry. 1985, Newbury Park: Sage Publications
Barbour RS: Checklists for improving rigour in qualitative research: a case of the tail wagging the dog?. BMJ. 2001, 322: 1115-1117. 10.1136/bmj.322.7294.1115.
Mays N, Pope C: Qualitative research in health care: Assessing quality in qualitative research. BMJ. 2000, 320: 50-52. 10.1136/bmj.320.7226.50.
Mason J: Qualitative researching. 2002, London: Sage
Brazier A, Cooke K, Moravan V: Using Mixed Methods for Evaluating an Integrative Approach to Cancer Care: A Case Study. Integr Cancer Ther. 2008, 7: 5-17. 10.1177/1534735407313395.
Miles MB, Huberman M: Qualitative data analysis: an expanded sourcebook. 1994, CA: Sage Publications Inc., 2
Pope C, Ziebland S, Mays N: Analysing qualitative data. Qualitative research in health care. BMJ. 2000, 320: 114-116. 10.1136/bmj.320.7227.114.
Cresswell KM, Worth A, Sheikh A: Actor-Network Theory and its role in understanding the implementation of information technology developments in healthcare. BMC Med Inform Decis Mak. 2010, 10 (1): 67-10.1186/1472-6947-10-67.
Article PubMed PubMed Central Google Scholar
Malterud K: Qualitative research: standards, challenges, and guidelines. Lancet. 2001, 358: 483-488. 10.1016/S0140-6736(01)05627-6.
Article CAS PubMed Google Scholar
Yin R: Case study research: design and methods. 1994, Thousand Oaks, CA: Sage Publishing, 2
Yin R: Enhancing the quality of case studies in health services research. Health Serv Res. 1999, 34: 1209-1224.
Green J, Thorogood N: Qualitative methods for health research. 2009, Los Angeles: Sage, 2
Howcroft D, Trauth E: Handbook of Critical Information Systems Research, Theory and Application. 2005, Cheltenham, UK: Northampton, MA, USA: Edward Elgar
Book Google Scholar
Blakie N: Approaches to Social Enquiry. 1993, Cambridge: Polity Press
Doolin B: Power and resistance in the implementation of a medical management information system. Info Systems J. 2004, 14: 343-362. 10.1111/j.1365-2575.2004.00176.x.
Bloomfield BP, Best A: Management consultants: systems development, power and the translation of problems. Sociological Review. 1992, 40: 533-560.
Shanks G, Parr A: Positivist, single case study research in information systems: A critical analysis. Proceedings of the European Conference on Information Systems. 2003, Naples
Pre-publication history
The pre-publication history for this paper can be accessed here: http://www.biomedcentral.com/1471-2288/11/100/prepub
Download references
Acknowledgements
We are grateful to the participants and colleagues who contributed to the individual case studies that we have drawn on. This work received no direct funding, but it has been informed by projects funded by Asthma UK, the NHS Service Delivery Organisation, NHS Connecting for Health Evaluation Programme, and Patient Safety Research Portfolio. We would also like to thank the expert reviewers for their insightful and constructive feedback. Our thanks are also due to Dr. Allison Worth who commented on an earlier draft of this manuscript.
Author information
Authors and affiliations.
Division of Primary Care, The University of Nottingham, Nottingham, UK
Sarah Crowe & Anthony Avery
Centre for Population Health Sciences, The University of Edinburgh, Edinburgh, UK
Kathrin Cresswell, Ann Robertson & Aziz Sheikh
School of Health in Social Science, The University of Edinburgh, Edinburgh, UK
You can also search for this author in PubMed Google Scholar
Corresponding author
Correspondence to Sarah Crowe .
Additional information
Competing interests.
The authors declare that they have no competing interests.
Authors' contributions
AS conceived this article. SC, KC and AR wrote this paper with GH, AA and AS all commenting on various drafts. SC and AS are guarantors.
Rights and permissions
This article is published under license to BioMed Central Ltd. This is an Open Access article distributed under the terms of the Creative Commons Attribution License ( http://creativecommons.org/licenses/by/2.0 ), which permits unrestricted use, distribution, and reproduction in any medium, provided the original work is properly cited.
Reprints and permissions
About this article
Cite this article.
Crowe, S., Cresswell, K., Robertson, A. et al. The case study approach. BMC Med Res Methodol 11 , 100 (2011). https://doi.org/10.1186/1471-2288-11-100
Download citation
Received : 29 November 2010
Accepted : 27 June 2011
Published : 27 June 2011
DOI : https://doi.org/10.1186/1471-2288-11-100
Share this article
Anyone you share the following link with will be able to read this content:
Sorry, a shareable link is not currently available for this article.
Provided by the Springer Nature SharedIt content-sharing initiative
- Case Study Approach
- Electronic Health Record System
- Case Study Design
- Case Study Site
- Case Study Report
BMC Medical Research Methodology
ISSN: 1471-2288
- General enquiries: [email protected]
Data and Analytics Case Study
Made possible by ey, exclusive global insights case study sponsor.
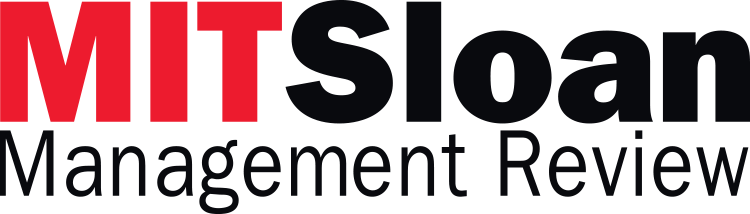
Data-Driven City Management
A close look at amsterdam’s smart city initiative, may 19, 2016, by: michael fitzgerald.
Despite the raw, rainy November afternoon in 2015, Ger Baron, Amsterdam’s chief technology officer, was radiating good feeling. Earlier in the day, city officials had finalized an agreement to share their traffic data with a major technology company. In exchange, the company would provide algorithms that Baron’s team could use to alleviate congestion on Amsterdam’s crowded streets.
Baron hoped this arrangement would trigger a cascade of civic-boosting partnerships between the city and outside companies, aimed at making Amsterdam a truly smart city. Data and analytics were crucial features of Amsterdam’s eight-year-old Smart City initiative, a public-private partnership that had produced more than 80 pilot projects across the city touching many areas of urban life.
Baron himself had led the Smart City initiative prior to his position as the city’s CTO. One activity his team had helped organize was an effort called Apps for Amsterdam, which challenged app developers to take publicly available data and build apps to improve the lives of residents and visitors. The initial projects weren’t quite what Amsterdam had envisioned, however. The first app developed had been a public toilet finder, which drew chuckles. Another early app had pointed out the best places to burgle houses, by triangulating data about public street lighting, the most expensive houses, and distances from police stations.
The burglar app was a sign that the city needed to refine its approach to using its troves of data to address city management issues. Partnering with companies to find data that met shared interests seemed like a fruitful path, especially for addressing traffic congestion on Amsterdam’s Reformation-era core city streets. Many of these are barely wider than a truck and are shared by bicycles, cars, pedestrians, trains, and buses. Amsterdam is a world leader in encouraging alternatives to car travel and in its electric vehicle infrastructure. The city also draws tens of millions of tourists a year to its vibrant fairs and floating flower markets, red-light district, cannabis coffee shops, and world-famous museums. Improving traffic and parking would support commerce, safety, and tourism.
About the Author
Michael Fitzgerald is a contributing editor at MIT Sloan Management Review .
1. Community Analysis Bureau, quoted in M. Vallianatos, “Uncovering the Early History of ‘Big Data’ and the ‘Smart City’ in Los Angeles,” Boom, note 12, June 2015, www.boomcalifornia.com.
2. P. Swabey, “IBM, Cisco, and the Business of Smart Cities,” February 23, 2012, www.information-age.com.
3. A. Townsend, “Smart Cities: Big Data, Civic Hackers, and the Quest for A New Utopia” (New York: W.W. Norton, 2013).
4. S. Mathis, “The Rise and Fall and Eventual Rise Again of the ‘Smart City’,” January 13, 2014, www.citylab.com. In September 2015, the U.S. government announced its commitment to spend $160 million to research and develop smart city technologies; see B. Miller, “Obama Places $160 Million Bet on Smart Cities, Internet of Things,” September 16, 2015, www.govtech.com.
5. All of Alliander’s shares are owned by Dutch provincial and municipal authorities. See “Legal Structure,” 2016, https://www.alliander.com/en.
6. “Amsterdam Smart City,” March 1, 2012, http://wwf.panda.org.
7. “Aanpak Top600,” n.d., https://www.amsterdam.nl. Source in Dutch; translated here: https://translate.google.com/translate?hl=en&sl=nl&u=https://www.amsterdam.nl/wonen-leefomgeving/veiligheid/openbare-orde/aanpak-top600/.
8. "Amsterdam Smart City: Waste and Energy Company. (n.d.)" http://amsterdamsmartcity.com/projects/detail/id/134/slug/waste-and-energy-company.
9. M. Halper, “Philips Teams Up With Cisco and Dutch Energy Utility Alliander on Smart Lighting Project,” February 12, 2016, www.ledsmagazine.com.
i. S. Quinton, “What Is a Smart City?,” April 26, 2016, www.pewtrusts.org.
ii. “Smart City,” May 2, 2016, https://en.wikipedia.org.
iii. T. Wolpe, "Big data deluge: How Dutch water is trying to turn the tide", October 1, 2013, zdnet.com.
v. P. Horner, "Dutch Delta team earns Edelman," ORMS Today 40 no. 3 (June 2013), www.informs.org.
vi. L. Winig, “GE’s Big Bet on Data and Analytics,” February 18, 2016, https://sloanreview.mit.edu.
vii. S. Ransbotham, D. Kiron, and P.K. Prentice, “Beyond the Hype: The Hard Work Behind Analytics Success,” March 8, 2016, https://sloanreview.mit.edu.
viii. S. Ransbotham, “Deodorizing Your Data,” August 24, 2015, https://sloanreview.mit.edu.
More Like This
Add a comment cancel reply.
You must sign in to post a comment. First time here? Sign up for a free account : Comment on articles and get access to many more articles.
Case study: Using insights to drive (and change) strategy
by Jeff Harris
September 30, 2020
Case Studies
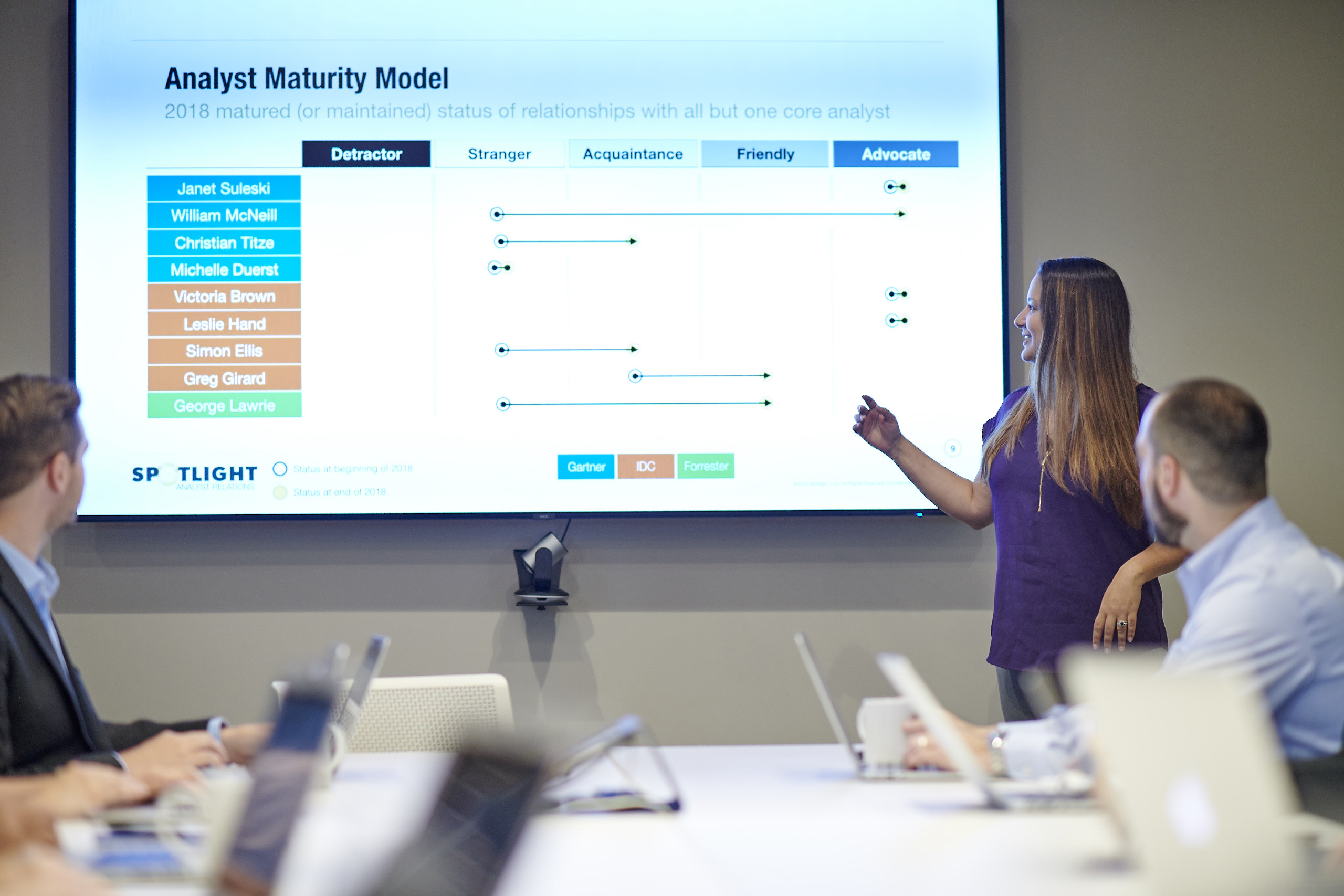
The insights-driven approach to AR has many benefits like providing more realistic AR metrics , offering competitive intel back to the business , and making analysts feel heard and valued . But sometimes other benefits of this approach aren’t realized until you’re in the moment capturing insights and surfacing issues you may have otherwise easily overlooked.
Recently, we worked with a client to establish insights-driven initiatives – AR plans centered around specific goals or themes – to help them win in their market with analysts. This client is a platform company looking to prove their place in the market and promote capabilities they feel they should be known for. We established a few key initiatives and took action on them, but soon found insights that changed our strategy.
First, we looked at our corporate goals.
Our client wanted to be known for their customer facing functionality, so our first initiative was to promote this capability. Secondly, they disagreed about how analysts define their platform so our second initiative became to change the analysts’ definition.
Initiative 1: Promoting our capabilities uncovered an unforeseen roadblock
We had nine interactions related to the capability we wanted to promote, and from those interactions we captured 5 insights. The insights were generally somewhat positive, but we were unclear why one analyst’s feedback wasn’t as positive as others’.
What we found was this analyst wasn’t quite understanding our message. We needed to spend more time with him to ask him questions about his point of view and to hopefully explain ours in more detail. This finding was surprising as we had no prior inclination that this analyst would struggle with how we were promoting our capability.
The insights-driven framework helped us catch when an analyst didn’t “get it.” If we hadn’t clearly outlined our mission and gathered feedback insights, we might have missed his perception gap of our business. Because the insights-driven approach shined a light on this issue, we were able to adjust our strategy with him.
Initiative 2: Combating a market definition – but ultimately learning from it
With our second initiative, we wanted to show analysts our point of view around our platform’s market definition. We planned a series of interactions to discuss the market and in doing so we uncovered significant insights – the analysts lack clarity themselves about what the market definition is.
This discovery allowed us to pivot our strategy to be more efficient and effective in our conversations with these analysts. Instead of touting our definition of the market against theirs, we realized we needed to learn more about the different viewpoints they have of the market. Because there isn’t one common definition, we want to know, how does each analyst define it? How do they think we should be talking about ourselves?
While this initiative started as one thing, the insights we learned from it gave us valuable information about what our strategy should be with these analysts. If we hadn’t been paying attention or capturing insights, we may not have seen the ambiguity among the analysts in this space.
Our takeaways from taking an insights-driven approach
Taking an insights-driven approach has certainly helped us create better strategies and better interactions with analysts. By focusing on capturing and analyzing insights, we were able to see potential roadblocks or challenges that may have gone unnoticed if we weren’t using this approach.
It’s also helped us prioritize our AR program goals. When we look at all the things we want to do and say to analysts against the realities of our working world, we can see exactly what’s feasible to accomplish and what is most important. Using insights to plan initiatives gives us this prioritization power.
If you’re curious to hear more about the insights-driven approach to AR, check out some of our webinars . We share how using insights can not only help you plan and strategize but also give you the power to make a significant impact on other aspects of your business.
Case Studies | Insights Driven AR
Related Posts
Get our latest ar blogs in your inbox.
RICHARD CV

Harnessing People-Driven-Change to accelerate transformation and the implementation of strategic priorities.
As another milestone on the roadmap out of lockdown is passed, now is the time for leaders to ensure that business and digital transformation activity underway is implemented successfully, at pace and delivering ROI. Whether the priority is to respond to new customer needs, generate efficiencies or to consolidate gains in the face of competitors who are intent on raising their game. The successful implementation of strategic initiatives to deliver growth, profit or resilience is imperative.
However, according to McKinsey 70% of transformations fail and just 16% of executives say that their digital transformation efforts are succeeding. So how do business leaders accelerate transformation and ensure they are successful?
As an experienced leader of both global and in-country transformation, I have experienced first-hand what really makes a difference when driving successful transformation and strategy implementation. I have also felt the pain and learnt from what doesn’t work.
Regardless of the change and programme management methodologies applied, it is people that drive success. Change happens at the individual level – whether it’s the implementation of a new operating model or technology, changes to process or an improved customer delivery approach; business benefits are delivered because individuals embrace working in a different way. The focus of transformation activity needs to be on driving employee behaviour change, ensuring individuals are capable of working in a new way and that the culture encourages them to do so - business benefits follow. The speed at which benefits are delivered are, to a great extent, determined by how committed and capable individuals and teams are to adopting the changes.
People-Driven Change builds on best practice change and team management, it involves the participation and engagement of individuals from all levels of the business in the design and implementation ensuring 1) the development of ‘fit for purpose’ organisational solutions and 2) leader and employee belief, advocacy and ownership of the transformation which drives momentum. Individuals are motivated to want to change and to want to work for change. Change Accelerators are critical activities which support successful delivery of the transformation.
Research by McKinsey supports this view, it shows that companies that have successfully delivered transformation are more likely to have roles across the organisation including line managers and front-line employees, visibly engaged in the transformation.
People-Driven-Change: an example
Pre Covid-19, a transformation team that I led in a UK market research agency ran a working session on ‘systems and automation’ as a part of a launch week for a new strategy. During the session those who had volunteered to attend discussed ideas around the ‘leveraging technology’ strategic priority. The session was surprisingly energetic and engaging given the attendees were mostly market researchers not typically interested in systems and process optimisation. It resulted in the resolution to investigate whether manual components of the research delivery process could be automated to free up time for researchers to focus on their clients.
Following agreement from the Board, I initiated a People-Driven Change approach by forming a cross functional team of enthusiastic or appropriately skilled IT, operations and client service people to spend a proportion of their time identifying automation solutions. A systems implementation specialist supported the team, providing guidance and challenge. The involvement of cross functional, multi-level teams of this nature were not the norm at the time, so it was important to reinforce the significance of what they were undertaking. From day one, the team, their mission and their progress was made visible to the whole business. This not only motivated the project team to succeed, it showed people that the system was being designed by people close to the work and close to the clients which built trust and belief.
Working in conjunction with a people-led approach to change are Change Accelerators, critical activities which support successful delivery of the transformation. Leadership buy-in and involvement for example is an accelerator. In this case the CEO, not someone close to the detail of the delivery process, spent time understanding both the issues being addressed and the technical solutions being developed. He became a visible advocate of the work and continually reinforced how it delivered not only the business strategy but also the many benefits for individuals and teams.
The task the team was undertaking cannot be underestimated- introducing new system functionality and process to replace inefficient, inconsistent, error prone, manual processes. However, the biggest barrier to the success of the digital transformation was the aversion of the users to move away from embedded ways of working. A prerequisite for the automation to work was both selling and delivering in a more standardised way, something client service teams had concerns about. Involving people from all levels of the business at the design phase not only meant that solutions were fit for purpose, but also meant that they were owned within the business and the benefits understood, this helped to overcome concerns.
To further drive participation, I gave the team the specific task of involving as many people as possible in the design and build process. Content development and expertise were crowdsourced, automation champions (change agents) were identified and positioned in delivery teams. All in all, over 110 people were involved out of a user population (the client service team) of 400, plus a further 15 people from the IT and Data Operations teams. Wide scale participation ensured not only that the best possible solution was built, but that from a very early stage there was an excitement within the business about what was coming, and individual commitment to support the implementation.
Engagement and communication is also key, a huge amount of focus was given to engaging business leaders, delivery teams and end users through the design, build and piloting phases. Progress and issues were shared openly, employee and client feedback was listened to and changes made in response. People not only knew what was going on, understood the importance but they felt a part of it.
Automation was launched to the business using a compelling employee benefits driven story, crafted and told by junior members of the client service team during a strategy activation week. They produced a short movie called ‘A day in the life of a researcher’ that emphasised that the digital transformation would mean less issues to deal with, the ability to spend more of their time on higher value tasks and less late nights in the office. In short, automation made the work more interesting and valuable. People also appreciated the value automation delivered for clients – quicker results, less errors and for our business improved efficiencies meant projects could be priced lower or be deliver more profit.
A sure sign that People-Driven Change was working was when, employees took the lead sharing their success stories at team meetings and in posts and videos on internal social media. In-team focus on progress and ROI became competitive across teams – with each wanting to be seen to be doing the best job embracing the automation. Another indication that the transformation was owned and being driven from within the business was the proactivity of the change agents. We put them in place to motivate change, answer questions and to support those struggling with the personal transition into the new ways of working. Very early on in the implementation process, the change agents started working together across teams and creating their own materials to support the roll out.
The energy, ownership and advocacy created by the approach drove high levels of adoption of the new technology, with 65% of applicable projects harnessing the new capability within 8 months of launch. The system improvements and new ways of working delivered benefits for clients, for teams as well as the business with up to 15% of project hours being saved on every project that was automated.
People-Driven Change delivers benefits beyond the transformation.
Perhaps one of the biggest benefits a participative approach to transformation delivers is the level of engagement, excitement and satisfaction that people feel being part of a major change initiative. You can feel an energy within the business.
As we emerge from the pandemic, experience the challenges of home/office hybrid working and recognise the experiences people have faced living through covid, People-Driven Change will provide the focus, energy and engagement to help businesses drive positive team experiences and build genuine engagement and commitment into the future.
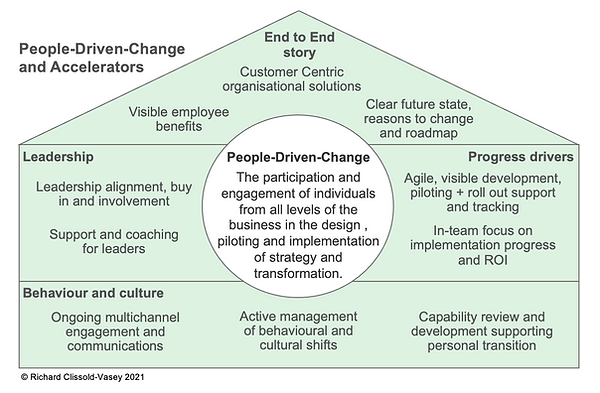
Information
- Author Services
Initiatives
You are accessing a machine-readable page. In order to be human-readable, please install an RSS reader.
All articles published by MDPI are made immediately available worldwide under an open access license. No special permission is required to reuse all or part of the article published by MDPI, including figures and tables. For articles published under an open access Creative Common CC BY license, any part of the article may be reused without permission provided that the original article is clearly cited. For more information, please refer to https://www.mdpi.com/openaccess .
Feature papers represent the most advanced research with significant potential for high impact in the field. A Feature Paper should be a substantial original Article that involves several techniques or approaches, provides an outlook for future research directions and describes possible research applications.
Feature papers are submitted upon individual invitation or recommendation by the scientific editors and must receive positive feedback from the reviewers.
Editor’s Choice articles are based on recommendations by the scientific editors of MDPI journals from around the world. Editors select a small number of articles recently published in the journal that they believe will be particularly interesting to readers, or important in the respective research area. The aim is to provide a snapshot of some of the most exciting work published in the various research areas of the journal.
Original Submission Date Received: .
- Active Journals
- Find a Journal
- Proceedings Series
- For Authors
- For Reviewers
- For Editors
- For Librarians
- For Publishers
- For Societies
- For Conference Organizers
- Open Access Policy
- Institutional Open Access Program
- Special Issues Guidelines
- Editorial Process
- Research and Publication Ethics
- Article Processing Charges
- Testimonials
- Preprints.org
- SciProfiles
- Encyclopedia
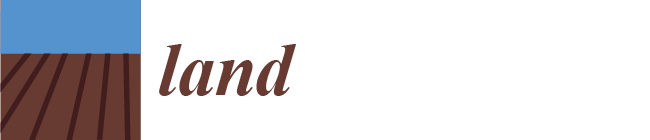
Article Menu
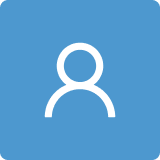
- Subscribe SciFeed
- Recommended Articles
- Google Scholar
- on Google Scholar
- Table of Contents
Find support for a specific problem in the support section of our website.
Please let us know what you think of our products and services.
Visit our dedicated information section to learn more about MDPI.
JSmol Viewer
Comprehensive ecological functional zoning: a data-driven approach for sustainable land use and environmental management—a case study in shenzhen, china, 1. introduction, 2. methodology, 2.1. zoning methods, 2.2. sub-watershed unit division techniques, 2.3. construction of indicator system for three-level zoning, 2.4. techniques for identification of main functions, 3. case study, 3.1. overview of the study area and data, 3.2. identification of candidate indicators, 3.2.1. sensitivity analysis of indicators, 3.2.2. factor analysis, 3.2.3. aquatic biological correlations analysis, 3.2.4. correlation analysis, 3.3. shenzhen city ecological function three-level zoning, 3.3.1. zoning index spatialization, 3.3.2. indicator weight, 3.3.3. comprehensive indicators analysis, 4. discussion, 5. conclusions.
- Definition and framework of watershed ecological function zoning: The concept and system of ecological zoning are elucidated. This method not only reflects the impact of natural factors on ecological systems, but also quantitatively incorporates the influence of human activities within a certain range. It considers the dual function of aquatic ecosystems in self-sustaining and providing water resources for human needs. Considering the spatial scales of different watershed levels, the hierarchical structural characteristics of aquatic ecosystems, and other factors, a comprehensive framework for the three-level zoning of watershed aquatic ecological functions is proposed. Specific zoning methods for different levels within the system are suggested, ultimately establishing a complete technical roadmap and research methodology for the three-level zoning of watershed aquatic ecology.
- Theoretical basis and technical methods for three-level zoning of river basin aquatic ecological functions: Based on the integrity of aquatic ecosystems, a structural characteristic index is proposed as the three-level zoning indicator for watershed aquatic ecological functions. This index can distinguish habitat characteristics and functional differences in different regions, thus enabling more effective management. In pursuit of finer river basin management, a three-level zoning theory based on the structural characteristics of aquatic ecosystems is presented, complementing the existing two-level zoning. This expanded framework better captures the spatial variability of aquatic ecosystem functions within river basins. Additionally, corresponding zoning objectives and unique principles are introduced. Guided by the three-level zoning theory, this paper proposes a method for dividing zoning units (sub-basin units), covering the division, indicator system construction, zoning technology, technical pathways, and main function identification.
- Completion of three-level zoning for terrestrial aquatic ecological functions in Shenzhen: In line with ecological zoning goals, 148 small basin units were identified. Through factor and correlation analyses, six key three-level zoning indicators for aquatic ecosystems were established, with weights determined by the entropy weight method. A spatial cluster analysis was used to integrate these results, resulting in 24 zones with distinct aquatic ecological functions. Standards for ecological function assessment, indicator weights, and evaluation principles were defined, with zoning results validated through a spatial functional analysis, finalizing the three-level zoning plan for Shenzhen.
Author Contributions
Data availability statement, conflicts of interest.
- Fang, Q.; Zhang, L.; Hong, H.; Zhang, L.; Bristow, F. Ecological function zoning for environmental planning at different levels. Environ. Dev. Sustain. 2008 , 10 , 41–49. [ Google Scholar ] [ CrossRef ]
- Xu, F.; Shao, Y.; Xu, B.; Li, H.; Xie, X.; Xu, Y.; Pu, L. Evaluation and zoning of cultivated land quality based on a space–function–environment. Land 2023 , 12 , 174. [ Google Scholar ] [ CrossRef ]
- Talukdar, G.; Bhattacharjya, R.K.; Sarma, A.K. Understanding the effect of long term and short term hydrological components on landscape ecosystem. Ecol. Inform. 2023 , 77 , 102267. [ Google Scholar ] [ CrossRef ]
- Deng, Y.; Ming, L.; Hai, Y.; Chen, H.; Jize, D.; Luo, J.; Yan, X.; Zhang, X.; Yao, S.; Hou, M. Has the Establishment of National Key Ecological Function Zones Improved Eco-Environmental Quality?—Evidence from a Quasi-Natural Experiment in 130 Counties in Sichuan Province, China. Land 2024 , 13 , 677. [ Google Scholar ] [ CrossRef ]
- Merriam, C.H. Life Zones and Crop Zones of the United States (No. 10) ; US Government Printing Office: Washington, DC, USA, 1898; pp. 7–8.
- Dong, J.; Metternicht, G.; Hostert, P.; Fensholt, R.; Chowdhury, R.R. Remote sensing and geospatial technologies in support of a normative land system science: Status and prospects. Curr. Opin. Environ. Sustain. 2019 , 38 , 44–52. [ Google Scholar ] [ CrossRef ]
- Liu, C.; Li, W.; Zhu, G.; Zhou, H.; Yan, H.; Xue, P. Land use/land cover changes and their driving factors in the Northeastern Tibetan Plateau based on Geographical Detectors and Google Earth Engine: A case study in Gannan Prefecture. Remote Sens. 2020 , 12 , 3139. [ Google Scholar ] [ CrossRef ]
- Aryal, B.; Escarzaga, S.M.; Vargas Zesati, S.A.; Velez-Reyes, M.; Fuentes, O.; Tweedie, C. Semi-automated semantic segmentation of arctic shorelines using very high-resolution airborne imagery, spectral indices and weakly supervised machine learning approaches. Remote Sens. 2021 , 13 , 4572. [ Google Scholar ] [ CrossRef ]
- Abdi, A.M. Land cover and land use classification performance of machine learning algorithms in a boreal landscape using Sentinel-2 data. GIScience Remote Sens. 2020 , 57 , 1–20. [ Google Scholar ] [ CrossRef ]
- Martínez Prentice, R.; Villoslada Peciña, M.; Ward, R.D.; Bergamo, T.F.; Joyce, C.B.; Sepp, K. Machine learning classification and accuracy assessment from high-resolution images of coastal wetlands. Remote Sens. 2021 , 13 , 3669. [ Google Scholar ] [ CrossRef ]
- Herridge, D.F.; Win, M.M.; Nwe, K.M.M.; Kyu, K.L.; Win, S.S.; Shwe, T.; Min, Y.Y.; Denton, M.D.; Cornish, P.S. The cropping systems of the Central Dry Zone of Myanmar: Productivity constraints and possible solutions. Agric. Syst. 2019 , 169 , 31–40. [ Google Scholar ] [ CrossRef ]
- Wickware, G.M. Classification for Stratification, Mapping, and Data Display for Range Inventories: Comment and Discussion. In Developing Strategies For Rangeland Management ; CRC Press: Boca Raton, FL, USA, 2021; pp. 631–646. [ Google Scholar ]
- Dufour, S.; Rodríguez-González, P.M.; Laslier, M. Tracing the scientific trajectory of riparian vegetation studies: Main topics, approaches and needs in a globally changing world. Sci. Total Environ. 2019 , 653 , 1168–1185. [ Google Scholar ] [ CrossRef ] [ PubMed ]
- Zheng, Z.; Wu, Z.; Chen, Y.; Yang, Z.; Marinello, F. Exploration of eco-environment and urbanization changes in coastal zones: A case study in China over the past 20 years. Ecol. Indic. 2020 , 119 , 106847. [ Google Scholar ] [ CrossRef ]
- Li, Z.T.; Li, M.; Xia, B.C. Spatio-temporal dynamics of ecological security pattern of the Pearl River Delta urban agglomeration based on LUCC simulation. Ecol. Indic. 2020 , 114 , 106319. [ Google Scholar ] [ CrossRef ]
- Gao, J.; Zou, C.; Zhang, K.; Xu, M.; Wang, Y. The establishment of Chinese ecological conservation redline and insights into improving international protected areas. J. Environ. Manag. 2020 , 264 , 110505. [ Google Scholar ] [ CrossRef ]
- Li, X.; Yu, X.; Wu, K.; Feng, Z.; Liu, Y.; Li, X. Land-use zoning management to protecting the Regional Key Ecosystem Services: A case study in the city belt along the Chaobai River, China. Sci. Total Environ. 2021 , 762 , 143167. [ Google Scholar ] [ CrossRef ]
- Zong, S.; Hu, Y.; Zhang, Y.; Wang, W. Identification of land use conflicts in China’s coastal zones: From the perspective of ecological security. Ocean Coast. Manag. 2021 , 213 , 105841. [ Google Scholar ] [ CrossRef ]
- Jin, X.; Wei, L.; Wang, Y.; Lu, Y. Construction of ecological security pattern based on the importance of ecosystem service functions and ecological sensitivity assessment: A case study in Fengxian County of Jiangsu Province, China. Environ. Dev. Sustain. 2021 , 23 , 563–590. [ Google Scholar ] [ CrossRef ]
- Li, R.; Zheng, H.; Polasky, S.; Hawthorne, P.L.; O’Connor, P.; Wang, L.; Li, R.; Xiao, Y.; Wu, T.; Ouyang, Z. Ecosystem restoration on Hainan Island: Can we optimize for enhancing regulating services and poverty alleviation? Environ. Res. Lett. 2020 , 15 , 084039. [ Google Scholar ] [ CrossRef ]
- Yang, L.; Li, X.; Yang, Q.; Zhang, L.; Zhang, S.; Wu, S.; Zhou, C. Extracting knowledge from legacy maps to delineate eco-geographical regions. Int. J. Geogr. Inf. Sci. 2021 , 35 , 250–272. [ Google Scholar ] [ CrossRef ]
- Fu, B. Several key points in territorial ecological restoration. Bull. Chin. Acad. Sci. 2021 , 36 , 64–69. [ Google Scholar ]
- Hong, W.; Jiang, R.; Yang, C.; Zhang, F.; Su, M.; Liao, Q. Establishing an ecological vulnerability assessment indicator system for spatial recognition and management of ecologically vulnerable areas in highly urbanized regions: A case study of Shenzhen, China. Ecol. Indic. 2016 , 69 , 540–547. [ Google Scholar ] [ CrossRef ]
- Liu, J.; Jiang, T.; Ye, Z. Information efficiency research of China’s carbon markets. Financ. Res. Lett. 2021 , 38 , 101444. [ Google Scholar ] [ CrossRef ]
- Yu, X.; Wan, K.; Du, Q. Can carbon market policies achieve a “point-to-surface” effect?—Quasi-experimental evidence from China. Energy Policy 2023 , 183 , 113803. [ Google Scholar ] [ CrossRef ]
- Lin, J.; Li, X. Large-scale ecological red line planning in urban agglomerations using a semi-automatic intelligent zoning method. Sustain. Cities Soc. 2019 , 46 , 101410. [ Google Scholar ] [ CrossRef ]
- Domingo, D.; Palka, G.; Hersperger, A.M. Effect of zoning plans on urban land-use change: A multi-scenario simulation for supporting sustainable urban growth. Sustain. Cities Soc. 2021 , 69 , 102833. [ Google Scholar ] [ CrossRef ]
- Xu, Z.; Peng, J.; Dong, J.; Liu, Y.; Liu, Q.; Lyu, D.; Qiao, R.; Zhang, Z. Spatial correlation between the changes of ecosystem service supply and demand: An ecological zoning approach. Landsc. Urban Plan. 2022 , 217 , 104258. [ Google Scholar ] [ CrossRef ]
- Zhou, Y.; Wu, T.; Wang, Y. Urban expansion simulation and development-oriented zoning of rapidly urbanising areas: A case study of Hangzhou. Sci. Total Environ. 2022 , 807 , 150813. [ Google Scholar ] [ CrossRef ]
- Liu, X.; Heilig, G.K.; Chen, J.; Heino, M. Interactions between economic growth and environmental quality in Shenzhen, China’s first special economic zone. Ecol. Econ. 2007 , 62 , 559–570. [ Google Scholar ] [ CrossRef ]
- Liu, X.; Su, Y.; Li, Z.; Zhang, S. Constructing ecological security patterns based on ecosystem services trade-offs and ecological sensitivity: A case study of Shenzhen metropolitan area, China. Ecol. Indic. 2023 , 154 , 110626. [ Google Scholar ] [ CrossRef ]
- Wang, G.; Yang, D.; Xia, F.; Zhong, R.; Xiong, C. Three types of spatial function zoning in key ecological function areas based on ecological and economic coordinated development: A case study of Tacheng Basin, China. Chin. Geogr. Sci. 2019 , 29 , 689–699. [ Google Scholar ] [ CrossRef ]
- Sun, M.; Yang, R.; Li, X.; Zhang, L.; Liu, Q. Designing a path for the sustainable development of key ecological function zones: A case study of southwest China. Glob. Ecol. Conserv. 2021 , 31 , e01840. [ Google Scholar ] [ CrossRef ]
- Ning, J.; Liu, J.; Kuang, W.; Xu, X.; Zhang, S.; Yan, C.; Li, R.; Wu, S.; Hu, Y.; Du, G.; et al. Spatiotemporal patterns and characteristics of land-use change in China during 2010–2015. J. Geogr. Sci. 2018 , 28 , 547–562. [ Google Scholar ] [ CrossRef ]
- Wang, W.; Jiao, L.; Jia, Q.; Liu, J.; Mao, W.; Xu, Z.; Li, W. Land use optimization modelling with ecological priority perspective for large-scale spatial planning. Sustain. Cities Soc. 2021 , 65 , 102575. [ Google Scholar ] [ CrossRef ]
- Zhang, L.; Liu, J.; Wan, D.; Yao, H.; Zheng, J.; Jiang, Y. Analysis and calculation of ecological flow in Shenzhen River based on zoning and classification. In Advances in Civil Engineering and Environmental Engineering ; CRC Press: Boca Raton, FL, USA, 2023; Volume 2, pp. 494–503. [ Google Scholar ]
- Tölgyesi, C.; Bátori, Z.; Erdős, L. Using statistical tests on relative ecological indicator values to compare vegetation units–Different approaches and weighting methods. Ecol. Indic. 2014 , 36 , 441–446. [ Google Scholar ] [ CrossRef ]
- Chi, G.; Yu, S.; Zhou, Y. A novel credit evaluation model based on the maximum discrimination of evaluation results. Emerg. Mark. Financ. Trade 2020 , 56 , 2543–2562. [ Google Scholar ] [ CrossRef ]
- Li, Y.; Wang, X.K.; Zhang, H.Y.; Wang, J.Q.; Li, L. An integrated regional water quality assessment method considering interrelationships among monitoring indicators. Environ. Monit. Assess. 2021 , 193 , 1–20. [ Google Scholar ] [ CrossRef ]
- Xian, C.; Gong, C.; Lu, F.; Wu, H.; Ouyang, Z. The evaluation of greenhouse gas emissions from sewage treatment with urbanization: Understanding the opportunities and challenges for climate change mitigation in China’s low-carbon pilot city, Shenzhen. Sci. Total Environ. 2023 , 855 , 158629. [ Google Scholar ] [ CrossRef ] [ PubMed ]
- Bin, Y.; Jingjing, J.; Lixin, M.; Peng, Y. Sustainable energy options for a low carbon demonstration city project in Shenzhen, China. J. Renew. Sustain. Energy 2015 , 7 , 023122. [ Google Scholar ] [ CrossRef ]
- Czúcz, B.; Keith, H.; Maes, J.; Driver, A.; Jackson, B.; Nicholson, E.; Kiss, M.; Obst, C. Selection criteria for ecosystem condition indicators. Ecol. Indic. 2021 , 133 , 108376. [ Google Scholar ] [ CrossRef ]
- Talke, S.A.; Jay, D.A. Changing tides: The role of natural and anthropogenic factors. Annu. Rev. Mar. Sci. 2020 , 12 , 121–151. [ Google Scholar ] [ CrossRef ]
- Akhtar, N.; Syakir Ishak, M.I.; Bhawani, S.A.; Umar, K. Various natural and anthropogenic factors responsible for water quality degradation: A review. Water 2021 , 13 , 2660. [ Google Scholar ] [ CrossRef ]
- Kaiser, H.F. The varimax criterion for analytic rotation in factor analysis. Psychometrika 1958 , 23 , 187–200. [ Google Scholar ] [ CrossRef ]
- Braeken, J.; Van Assen, M.A. An empirical Kaiser criterion. Psychol. Methods 2017 , 22 , 450. [ Google Scholar ] [ CrossRef ]
- Liu, C.W.; Lin, K.H.; Kuo, Y.M. Application of factor analysis in the assessment of groundwater quality in a blackfoot disease area in Taiwan. Sci. Total Environ. 2003 , 313 , 77–89. [ Google Scholar ] [ CrossRef ]
- Singh, K.P.; Malik, A.; Singh, V.K.; Mohan, D.; Sinha, S. Chemometric analysis of groundwater quality data of alluvial aquifer of Gangetic plain, North India. Anal. Chim. Acta 2005 , 550 , 82–91. [ Google Scholar ] [ CrossRef ]
- Qian, Y.; Migliaccio, K.W.; Wan, Y.; Li, Y. Surface water quality evaluation using multivariate methods and a new water quality index in the Indian River Lagoon, Florida. Water Resour. Res. 2007 , 43 , 7–8. [ Google Scholar ] [ CrossRef ]
- Hill, M.O.; Gauch, H.G., Jr. Detrended correspondence analysis: An improved ordination technique. Vegetatio 1980 , 42 , 47–58. [ Google Scholar ] [ CrossRef ]
- Ter Braak, C.J.; Prentice, I.C. A theory of gradient analysis. In Advances in Ecological Research ; Academic Press: Cambridge, MA, USA, 1988; Volume 18, pp. 271–317. [ Google Scholar ]
- Asuero, A.G.; Sayago, A.; González, A.G. The correlation coefficient: An overview. Crit. Rev. Anal. Chem. 2006 , 36 , 41–59. [ Google Scholar ] [ CrossRef ]
- Charnes, A.; Cooper, W.W.; Rhodes, E. Measuring the efficiency of decision making units. Eur. J. Oper. Res. 1978 , 2 , 429–444. [ Google Scholar ] [ CrossRef ]
- Hong, W.; Guo, R.; Su, M.; Tang, H.; Chen, L.; Hu, W. Sensitivity evaluation and land-use control of urban ecological corridors: A case study of Shenzhen, China. Land Use Policy 2017 , 62 , 316–325. [ Google Scholar ] [ CrossRef ]
- Peng, J.; Zhao, M.; Guo, X.; Pan, Y.; Liu, Y. Spatial-temporal dynamics and associated driving forces of urban ecological land: A case study in Shenzhen City, China. Habitat Int. 2017 , 60 , 81–90. [ Google Scholar ] [ CrossRef ]
- Yi, L.; Chen, J.; Jin, Z.; Quan, Y.; Han, P.; Guan, S.; Jiang, X. Impacts of human activities on coastal ecological environment during the rapid urbanization process in Shenzhen, China. Ocean Coast. Manag. 2018 , 154 , 121–132. [ Google Scholar ] [ CrossRef ]
- Garland, G.; Banerjee, S.; Edlinger, A.; Miranda Oliveira, E.; Herzog, C.; Wittwer, R.; Philippot, L.; Maestre, F.T.; van Der Heijden, M.G. A closer look at the functions behind ecosystem multifunctionality: A review. J. Ecol. 2021 , 109 , 600–613. [ Google Scholar ] [ CrossRef ]
- Carlucci, M.B.; Brancalion, P.H.; Rodrigues, R.R.; Loyola, R.; Cianciaruso, M.V. Functional traits and ecosystem services in ecological restoration. Restor. Ecol. 2020 , 28 , 1372–1383. [ Google Scholar ] [ CrossRef ]
- Jalkanen, J.; Toivonen, T.; Moilanen, A. Identification of ecological networks for land-use planning with spatial conservation prioritization. Landsc. Ecol. 2020 , 35 , 353–371. [ Google Scholar ] [ CrossRef ]
- Bibri, S.E.; Krogstie, J.; Kärrholm, M. Compact city planning and development: Emerging practices and strategies for achieving the goals of sustainability. Dev. Built Environ. 2020 , 4 , 100021. [ Google Scholar ] [ CrossRef ]
- Almenar, J.B.; Elliot, T.; Rugani, B.; Philippe, B.; Gutierrez, T.N.; Sonnemann, G.; Geneletti, D. Nexus between nature-based solutions, ecosystem services and urban challenges. Land Use Policy 2021 , 100 , 104898. [ Google Scholar ] [ CrossRef ]
- Soininen, J.; Bartels, P.I.A.; Heino, J.; Luoto, M.; Hillebrand, H. Toward more integrated ecosystem research in aquatic and terrestrial environments. BioScience 2015 , 65 , 174–182. [ Google Scholar ] [ CrossRef ]
- d’Alcalà, M.R. Similarities, differences and mechanisms of climate impact on terrestrial vs. marine ecosystems. Nat. Conserv. 2019 , 34 , 505–523. [ Google Scholar ] [ CrossRef ]
- Prampolini, M.; Savini, A.; Foglini, F.; Soldati, M. Seven good reasons for integrating terrestrial and marine spatial datasets in changing environments. Water 2020 , 12 , 2221. [ Google Scholar ] [ CrossRef ]
Click here to enlarge figure
Index | Unit | Minimum | Maximum | Range | Mean | Standard Deviation | Coefficient of Variation (%) | |
---|---|---|---|---|---|---|---|---|
F1 | Forest Area Ratio | % | 0.00 | 100.00 | 100.00 | 36.00 | 34.50 | 95.92 |
F2 | GDP Per Unit Area | 10 CNY/km | 0.00 | 29,550.30 | 29,550.30 | 362.67 | 1343.41 | 370.42 |
F3 | Drainage Density | km | 0.00 | 6.60 | 6.60 | 0.46 | 0.44 | 96.64 |
F4 | Farmland Area Ratio | % | 0.00 | 100.00 | 100.00 | 37.00 | 31.50 | 85.13 |
F5 | Urban Area Ratio | % | 0.00 | 95.00 | 95.00 | 3.00 | 6.12 | 204.20 |
F6 | Watershed Slope | Degree | 0.00 | 17.56 | 17.56 | 4.00 | 3.61 | 90.14 |
F7 | Watershed Slope Direction | - | 0.00 | 286.24 | 286.24 | 170.43 | 21.31 | 12.50 |
F8 | Water Area Ratio | % | 0.00 | 100.00 | 100.00 | 3.00 | 8.90 | 296.67 |
F9 | Volume of Water | mm | 0.00 | 13,667.88 | 13,667.88 | 5419.74 | 1085.70 | 20.03 |
F10 | Population Density | p/km | 0.00 | 10,841.00 | 10,841.00 | 116.91 | 446.76 | 382.13 |
F11 | Grassland Area Ratio | % | 0.00 | 100.00 | 100.00 | 11.00 | 15.90 | 144.30 |
Principal Component | Initial Eigenvalue | Selection Sums of Squared Loadings | Rotation Sums of Squared Loadings | ||||||
---|---|---|---|---|---|---|---|---|---|
Sum | Variance % | Accumulate % | Sum | Variance % | Accumulate % | Sum | Variance % | Accumulate % | |
1 | 2.890 | 32.110 | 32.110 | 2.890 | 32.110 | 32.110 | 2.461 | 27.341 | 27.341 |
2 | 1.717 | 19.076 | 51.186 | 1.717 | 19.076 | 51.186 | 2.063 | 22.924 | 50.265 |
3 | 1.372 | 15.241 | 66.426 | 1.372 | 15.241 | 66.426 | 1.411 | 15.680 | 65.945 |
4 | 1.095 | 12.171 | 78.597 | 1.095 | 12.171 | 78.597 | 1.139 | 12.652 | 78.597 |
Principal Component 1 | Principal Component 2 | Principal Component 3 | Principal Component 4 | |
---|---|---|---|---|
Zscore (F1) | 0.932 | −0.218 | −0.118 | −0.142 |
Zscore (F2) | −0.013 | −0.086 | −0.064 | 0.977 |
Zscore (F3) | −0.082 | 0.030 | 0.821 | 0.062 |
Zscore (F4) | 0.854 | 0.061 | −0.174 | −0.361 |
Zscore (F5) | −0.222 | 0.841 | −0.058 | −0.091 |
Zscore (F6) | 0.884 | −0.107 | −0.172 | −0.097 |
Zscore (F8) | −0.031 | 0.047 | 0.765 | −0.105 |
Zscore (F10) | −0.057 | 0.885 | −0.062 | −0.036 |
Zscore (F11) | −0.013 | 0.727 | 0.252 | 0.001 |
Axis | Eigenvalue | Correlation Coefficient | Cumulative Percentage of Variance | |
---|---|---|---|---|
Species | Species-Environment | |||
AX1 | 0.464 | 0.720 | 46.438 | 96.109 |
AX2 | 0.008 | 0.483 | 47.323 | 97.920 |
AX3 | 0.006 | 0.547 | 47.935 | 99.243 |
AX4 | 0.004 | 0.292 | 48.321 | 100.000 |
F1 | F2 | F4 | F5 | F6 | F8 | ||
---|---|---|---|---|---|---|---|
F1 | Pearson correlation | 1 | |||||
Significance (bilateral) | |||||||
F2 | Pearson correlation | −0.129 ** | 1 | ||||
Significance (bilateral) | 0.000 | ||||||
F4 | Pearson correlation | −0.444 ** | −0.290 ** | 1 | |||
Significance (bilateral) | 0.000 | 0.000 | |||||
F5 | Pearson correlation | −0.303 ** | −0.143 ** | 0.242 ** | 1 | ||
Significance (bilateral) | 0.000 | 0.000 | 0.000 | ||||
F6 | Pearson correlation | 0.422 ** | −0.043 ** | −0.399 ** | −0.254 ** | 1 | |
Significance (bilateral) | 0.000 | 0.000 | 0.000 | 0.000 | |||
F8 | Pearson correlation | −0.117 ** | −0.078 ** | −0.050 ** | 0.068 ** | −0.141 ** | 1 |
Significance (bilateral) | 0.000 | 0.000 | 0.000 | 0.000 | 0.000 |
Index | Weight |
---|---|
Forest area ratio | 0.170 |
farmland area ratio | 0.140 |
Water area ratio | 0.246 |
Urban area ratio | 0.256 |
GDP per unit area | 0.068 |
Watershed slope | 0.120 |
The statements, opinions and data contained in all publications are solely those of the individual author(s) and contributor(s) and not of MDPI and/or the editor(s). MDPI and/or the editor(s) disclaim responsibility for any injury to people or property resulting from any ideas, methods, instructions or products referred to in the content. |
Share and Cite
Li, Y.; Zhang, F.; Li, R.; Yu, H.; Chen, Y.; Yu, H. Comprehensive Ecological Functional Zoning: A Data-Driven Approach for Sustainable Land Use and Environmental Management—A Case Study in Shenzhen, China. Land 2024 , 13 , 1413. https://doi.org/10.3390/land13091413
Li Y, Zhang F, Li R, Yu H, Chen Y, Yu H. Comprehensive Ecological Functional Zoning: A Data-Driven Approach for Sustainable Land Use and Environmental Management—A Case Study in Shenzhen, China. Land . 2024; 13(9):1413. https://doi.org/10.3390/land13091413
Li, Yu, Fenghao Zhang, Ruifan Li, Hongbing Yu, Yao Chen, and Han Yu. 2024. "Comprehensive Ecological Functional Zoning: A Data-Driven Approach for Sustainable Land Use and Environmental Management—A Case Study in Shenzhen, China" Land 13, no. 9: 1413. https://doi.org/10.3390/land13091413
Article Metrics
Article access statistics, further information, mdpi initiatives, follow mdpi.
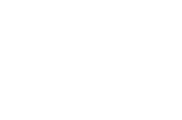
Subscribe to receive issue release notifications and newsletters from MDPI journals
Introducing The CLM Landscape 2024: From Use Case-Driven Solutions To Enterprise Contract Management Platforms
Alla Valente , Senior Analyst
As global interconnectedness exponentially increases the number of commercial and business relationships between organizations, the speed of innovation is also reshaping how they operate. In other words, for an organization to execute on its generative AI (genAI) strategy (and for the record, the absence of a genAI strategy is a genAI strategy), they’ll need to change the process and language of how they contract.
In my new report, The Contract Lifecycle Management Platforms Landscape, Q3 2024 , I looked at the 27 notable vendors that a wide range of corporate stakeholders, such as procurement, legal, tech execs, and risk professionals, use to support corporate strategy, commercial activity, business operations, technology investment, and other business processes that require contractual arrangements. We define contract lifecycle management (CLM) platforms as:
Technology that automates contract digitization, creation, negotiation, execution, and governance. CLM platforms include analysis and reporting for insights into the terms, risks, obligations, and entitlements at the contract and portfolio level; metrics capture for contract(ing) optimization; and integration with adjacent technologies to ensure accuracy, efficiency, and compliance across multiple types of contracts. CLM platforms support the entire contract lifecycle, including pre-signature, post-signature, contract governance, and obligations management.
Enterprise Benefit Gain Requires An Enterprise Approach To Contract Management
In the 2023 Forrester landscape report covering CLM, it was noted that the platforms had matured from acting as digital contract repositories with authoring capabilities to complete contract lifecycle management technologies. In the 20 months since then, CLM has evolved further from use case-driven solutions for buy-side and sell-side contracts toward more comprehensive support for enterprisewide contracting. This is due to the following factors:
- Contracts are the bridge between genAI strategy and execution. How your organization goes from genAI as a strategy to genAI as a reality will largely depend on the products, services, and contracts involved. In many organizations, genAI will come by way of third parties in the form of foundation models, pretrained data, open-source large language models, and new genAI capabilities of existing third parties. The CLM becomes a critical tool to align contract language, clauses, terms, and obligations that reflect guidelines and requirements of how third parties can and should use genAI to support your organization.
- New risk/reward trade-offs require a deeper dive. Contracts are legally binding and enforceable, which means failing to align your genAI strategy and updated policies with your contracts, SLAs, and terms of use/service is a risk to your organization. To avoid this, organizations turn to CLM to identify outdated language and to flag active contracts that are not aligned with current policies.
- The speed of new regulatory change demands better alignment. Regulators are working overtime to issue new requirements to keep up with business reality. With quickly approaching effective dates for new regulations such as the Digital Operational Resilience Act ( DORA ), the SEC’s climate-related disclosure rules, AI regulations in the EU , and state-level AI requirements in the US, organizations must ensure their own compliance as well as assure the compliance of counterparties. As these regulations cover many risk domains and span many areas of the business, organizations require a CLM that can provide an enterprisewide perspective.
- The litigation juggernaut proves that compliance is the floor, not the ceiling. Like it or not, corporate class-action lawsuits are on the rise and compounded by the velocity and complexity of claims. As the speed and scale of AI adoption accelerates and as updates to existing regulation lag, the courtroom becomes the arena for AI concerns. To avoid that fate, the CLM has become a crucial tool for enterprise risk, governance, and accountability.
Read the full report for more details on CLM market dynamics, notable vendors, and top use cases. Also, schedule an inquiry or guidance session with me for further insights or to discuss your CLM program.
- Age of the Customer
- security risk management
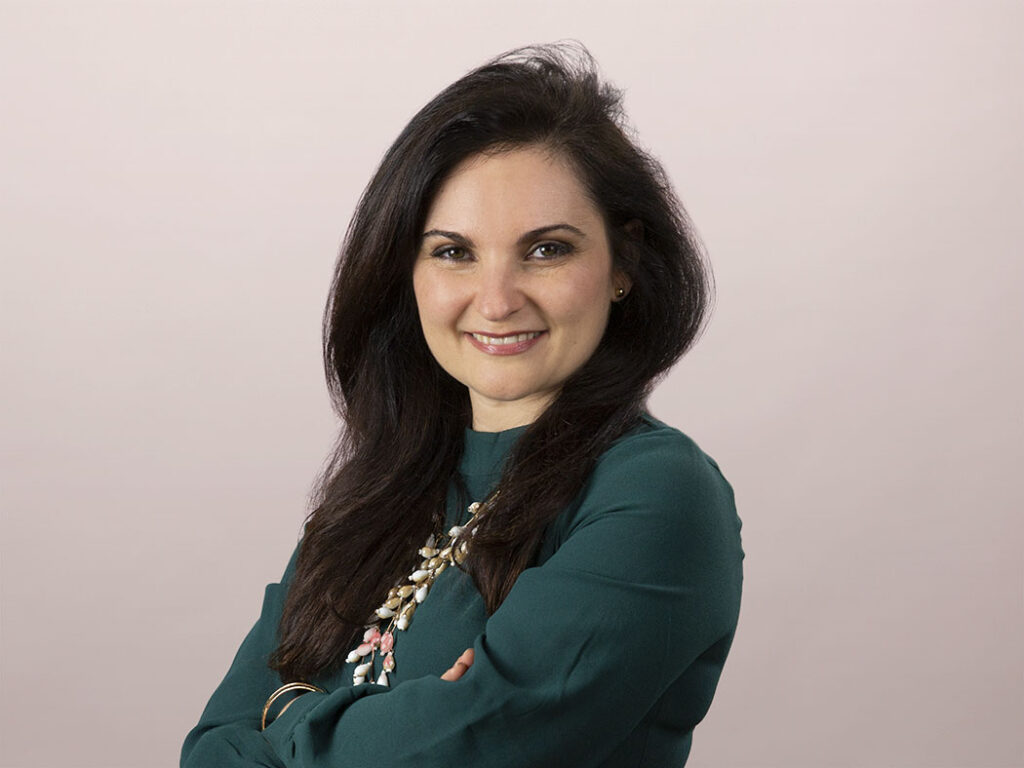
Thanks for signing up.
Stay tuned for updates from the Forrester blogs.
What’s Your 2025 Budget Plan For AI, Software, & Tech Debt?
Attend our live webinar on sept. 24 hear analyst insights on where to invest, divest, and experiment with 2025 tech and security budgets for maximum business impact., build an alliance with your cio, fraud management in apac: what’s new in 2024, get the insights at work newsletter, help us improve.
- Open access
- Published: 06 September 2024
Motivations of family advisors in engaging in research to improve a palliative approach to care for persons living with dementia: an interpretive descriptive study
- Stephanie Lucchese 1 , 2 ,
- Marie-Lee Yous 1 ,
- Julia Kruizinga 1 ,
- Shirin Vellani 2 , 3 ,
- Vanessa Maradiaga Rivas 1 ,
- Bianca Tétrault 4 ,
- Pam Holliday 1 ,
- Carmel Geoghegan 5 ,
- Danielle Just 2 ,
- Tamara Sussman 4 ,
- Rebecca Ganann 1 &
- Sharon Kaasalainen 1
Research Involvement and Engagement volume 10 , Article number: 94 ( 2024 ) Cite this article
6 Altmetric
Metrics details
A Strategic Guiding Council (SGC) was created within a Family Carer Decisions Support study, to engage family carers of persons with advanced dementia as advisors to inform the design and implementation of the study. The SGC consists of an international group of family advisors from Canada, the Republic of Ireland, the United Kingdom, the Netherlands, and the Czech Republic. There are limited studies that have explored the integration of Patient and Public Involvement (PPI) in dementia research, end-of-life care and long-term care. Therefore, this study explores PPI engagement in health research with family carers to understand further their interest in being involved in the SCG within the FCDS intervention which is focused on supporting caregivers to make decisions about end-of-life care for residents with advanced dementia.
This study utilized an interpretive descriptive design and explored the motivations of ten family advisors from Canada, the Republic of Ireland, the United Kingdom, and the Netherlands in being part of the SGC. Semi-structured interviews were conducted by phone or videoconferencing and were recorded, transcribed, and analyzed using thematic analysis.
Three themes generated from the findings of the study were (1) engaging in reciprocal learning; (2) using lived experience to support other family carers; and (3) creating a collective momentum for advocacy and change.
Conclusions
Family carers motivations to being part of the SCG was driven by their intent to help carers navigate the health system and to create a psychosocial support system for other carers experiencing end-of-life with their loved ones. Being part of the SCG provided a benefit to family carers which provided a venue for them to contribute meaningful information from their experience, learn from other health professionals, research and other advisors and an avenue for advocacy work to improve access to end-of-life care supports through education. To our knowledge, this is the first study that explores the motivations of an international group of family advisors’ engagement in health research to promote integration of a palliative approach to dementia care in long-term care homes. This study further contributes to the literature from an international perspective the importance of PPI in research. Further research is warranted that explores PPI in research to improve access to end-of-life supports.
Plain English summary
Patient and public involvement (PPI) in research has been growing worldwide and ensures that research conducted is relevant to the needs of patients. Within the Family Carer Decisions Support study, we created a Strategic Guiding Council (SGC) to engage family carers of persons with advanced dementia as advisors to improve access to a palliative approach to care. The SGC includes family advisors from Canada, the Republic of Ireland, the United Kingdom, the Netherlands, Italy and the Czech Republic. The goal of the strategy was to engage with family advisors in research activities to improve access to a palliative approach and quality of care provided to people with dementia receiving end-of-life care. Including patients and family in research is extremely important, therefore our aim in this study was to explore family advisors’ motivations to engaging in the SGC. Family advisors reported encountering challenges with navigating the health system with end-of-life care and they chose to participate in the SGC to support other carers who are experiencing a similar situation. Being part of the SGC also provided the family advisors with an avenue to advocate on the importance of improving access to end-of-lifesupports and the opportunity to learn from other advisors, health professionals and researchers.
Peer Review reports
Introduction
Patient and Public Involvement (PPI) in research has been growing internationally due to efforts to ensure that health research is relevant, meaningful and impactful for patients and the public [ 1 ]. PPI ensures studies are focused on patient-identified priorities and encourages patients and their family/friends to be proactive partners in all stages of the research process: study design, intervention implementation, interpretation and developing of findings and knowledge translation [ 2 , 3 ]. PPI values a person’s lived experience and reported benefits include improvement in patient experiences, high quality health care and ensuring research funding is allocated to studies that are relevant and deemed a priority to patients and the public [ 4 , 5 ]. Most importantly, PPI ensures that work is being carried out with or by members of the public, rather than for them or about them.
The inclusion of PPI in health research has been deemed a priority in many countries [ 6 ]. The United Kingdom has made PPI participation a requirement to increase community involvement in health service planning and decision making in the delivery of health initiatives and research [ 6 ]. Similarly, in Canada, the Canadian Institute for Health Research (CIHR) developed partnerships with funding agencies to develop a Strategy for Patient-Oriented Research to improve health outcomes by promoting the engagement of patients and focusing on priorities identified by patients [ 7 ]. PPI has been studied in stroke care [ 8 ], dialysis [ 9 ], mental health research [ 10 ] and has been growing within dementia research [ 11 ]. Findings from the Bethell et al. (2018) scoping review discussed the importance of collective learning from people with lived experience with dementia and reinforced the importance of challenging societal views that portray people with dementia as not fully capable of participating in research [ 11 ]. Although there is broad consensus on the importance of integrating PPI in health research, further research is warranted to explore the integration of PPI in dementia research, long term care (LTC) and end-of-life care to improve health outcomes for older adults living with dementia and their family carers through meaningful engagement in health research.
In order to improve the quality, relevance and uptake of research amongst family carers, PPI engagement was integrated within the Family Carer Decision Support (FCDS) mySupport study [ 12 , 13 , 14 ]. The FCDS intervention focused on supporting caregivers to make decisions about end-of-life care for residents with advanced dementia. Introducing a palliative approach to care promotes a comprehensive person-centered approach to care for residents during end-of-life through advance care planning, management of disease symptoms, psychosocial and spiritual care and bereavement support [ 15 ]. The FCDS study was implemented in Canada, the Republic of Ireland, the United Kingdom, the Netherlands, Czech Republic, and Italy during the COVID-19 pandemic. An international PPI panel, called The Strategic Guiding Council, was formed early on to help inform the design, implementation and evaluation of the FCDS intervention by advising the research team and sharing their experiences in caring for individuals living with advanced dementia in LTC. There were 15 people involved in the Strategic Guiding Council. These individuals met via Zoom at quarterly Strategic Guiding Council meetings. They were involved in reviewing draft intervention components such as the comfort care booklet, providing assistance in interpreting study findings, and offering recommendations for knowledge translation and dissemination activities [ 16 , 17 ].
To our knowledge, there are currently no research studies that have explored PPI engagement in health research within an international Strategic Guiding Council. In addition, there is little information in the literature that discusses how to engage and involve families in LTC research [ 18 , 19 ]. Meaningful engagement with family carers is an important area to focus on in research as it provides researchers with a unique perspective of their lived experience which can also lead to an improvement in research questions, facilitate knowledge transfer and promote trust [ 18 , 20 ]. Therefore, this qualitative study aimed to contribute to the literature on PPI engagement with family carers by exploring the motivations of family carers to become family advisors on the Strategic Guiding Council. It is imperative to explore the motivations of family carers in research for recruitment and retention purposes as they are important members of the research team who can provide feedback on the practicality and relevance of interventions. The research question was: What are family advisors’ motivations in working with a Strategic Guiding Council and research team to promote integration of a palliative approach to dementia care in LTC homes?
This study utilized Sally Thorne’s (2016) interpretive description methodology to address the research question. Interpretive description is grounded in a naturalistic inquiry and objective knowledge is unattainable through empirical analysis but rather, the participants and researcher construct meaning together [ 21 ]. Interpretive descriptive is known as a useful methodology to generate knowledge for health service research due to its focus on experiential knowledge and exploring human commonalities and differences [ 21 ]. This approach was suitable for this study to reflect on the diverse experiences of family carers in supporting persons living with dementia which shaped their motivations to engage in research as family advisors.
Convenience sampling [ 22 ] was used to locate participants who were interested in being a member of the Strategic Guiding Council to advise the FCDS study and share their viewpoint as family carers for individuals living with advanced dementia. Specifically, they met the following inclusion criteria: (a) family carer of a person with advanced dementia (living or passed away in the last five years); (b) 16 years of age or older; and (d) comfortable communicating in English.
Recruitment
Recruitment for the Strategic Guiding Council consisted of both passive and active recruitment strategies. We attempted to recruit participants from all six countries involved in the FCDS intervention, however we were unable to recruit family carers from Italy and the Czech Republic due to language barriers. Hence, we ended up with family carers from four of the six participating countries: Canada, United Kingdom, the Republic of Ireland and the Netherlands. The recruitment of family advisors to join the Strategic Guiding Council and research study was conducted through the completion of an Expression of Interest Form and country-specific recruitment flyer, which were posted at local organizations (i.e., LTC homes) for a period of two months between June to August 2020. The Expression of Interest Form and the Country-Specific Recruitment Flyers were also posted on the mySupport website. Family carers who were interested in participating in the Strategic Guiding Council completed the Expression of Interest Forms and once completed, the PPI study coordinator, along with the country-specific research representative, contacted eligible individuals who met the inclusion criteria.
Active recruitment strategies for the study consisted of staff working in LTC homes or nursing homes to share information to family carers regarding the Strategic Guiding Council and mySupport study following a recruitment script guideline developed by the research team. Verbal permission was sought from the family carers to have their contact information shared with the research team to discuss the Strategic Guiding Council and mySupport study further. A telephone or email recruitment script was used by research staff to inform interested family carers about the study, answer any questions and affirm their continued interest to participate. Family carers that provided consent to be part of the Strategic Guiding Council as family advisors were provided with an orientation, which was conducted by the PPI coordinator or the country-specific research representative either in-person or virtually. Two family advisors were included in this manuscript as co-authors as they contributed to writing and reviewing the manuscript.
Data collection
Demographic data such as country of residence, sex, age, and relationship to person living with dementia were collected electronically using LimeSurvey. Semi-structured interviews were conducted by the PPI coordinator or by the country specific research representative either on the telephone or Zoom videoconference platform based on participants’ preference from September 2020–2021. The interviewers were master’s prepared or graduate students in the field of nursing and health studies. Interviews were scheduled based on their preference for time in their respective time zones and within the first three months of joining the Strategic Guiding Council. Interviews were conducted in English and the country specific research coordinator was available to assist participants with translation support as a ‘buddy’. Participants took part in an interview in a quiet, private location of their choosing such as in their home. An interview guide was used to explore family carers’ motivation in working with a Strategic Guiding Council and research team and developed through a review of the literature for PPI concepts. Examples of interview questions were: What is your experience with palliative care? What is your experience working with research and what about your experience working on a panel or a committee? What is your understanding of a Strategic Guiding Council? The interviews lasted 30 to 40 min and were audio-recorded and transcribed verbatim. Identifying information was removed and transcripts were reviewed against the recording for accuracy [ 23 ]. Participants’ identity was protected throughout the study and all study information that was recorded, transcribed and analyzed were kept in a locked cabinet or stored in a password protected, encrypted file.
Data analysis
Demographic data were analyzed using counts for categorical data and means and standard deviations (SD) for continuous data. The first stage of data analysis consisted of “sorting and organizing” [21 pp 156] the data by reading the transcripts. In the second stage, “making sense of pattern” [21 pp 163], the authors (SL and JK) analyzed two transcripts independently and generated a code list and themes. The authors (SL and JK) met together and discussed their preliminary findings. Descriptive codes were merged to develop themes or groupings [ 21 ]. The findings were then discussed with additional research team members (SV, VM and SK) and themes were defined based on consensus. Once consensus was gained within the research team, SL proceeded with analyzing the remaining transcripts using Dedoose qualitative software. For the final stage, “transforming pattern into findings” [21 pp 173] the author SL met frequently with the research team during data analysis to review the findings and themes generated. The final written conceptualization of the findings was reviewed by the research team and presented in this study [ 21 ].
Ethical considerations
This study was approved by local research ethics board (#5837). Research was conducted following the standards outlined by the Tri- Council Policy Statement for Ethical Conduct for Research Involving Humans [ 24 ]. Written and verbal informed consent was sought from all participants with the understanding that their participation in the study would not influence them or their relatives care at the LTC home. Each participant had experience as a family carer for a relative with dementia and due to this, were sensitive to emotional reactions. Therefore, the sensitive interviewing techniques were employed, which included validation of emotions and experiences of the interviewer, frequent breaks and if needed, discontinuation of the interview.
Rigour and trustworthiness
Several strategies were employed by the research team to enhance trustworthiness and rigour in the study. The research team participated in co-constructing knowledge by interpreting participants experiences from the semi-structured interviews. Researcher triangulation was achieved by meeting frequently with the research team to discuss data analysis and themes developed. Engaging in triangulation allowed the researcher to confirm constructed perception of an event or process [ 21 ]. An audit trial was maintained by the primary author to establish confirmability of the research findings and ensure the findings are based on participants responses instead of the researchers’ own preconceptions and biases.
Characteristics of sample
A total of 10 family advisors shared their perspectives in being part of the Strategic Guiding Council for the mySupport Family Carer Decision Support Study. There was representation from four countries, Canada ( n = 4), United Kingdom ( n = 1), the Netherlands ( n = 1), and the Republic of Ireland ( n = 4). Demographics were missing for some advisors ( n = 5). Half of the advisors were female ( n = 5). The mean age of advisors was 65.6 years (SD = 5.0) and most were caring for a spouse or parent. Most of the advisors reported having education at the post-secondary level (i.e., college, bachelor’s degree, masters).
Overview of findings
Three themes represented the family advisors’ motivation in engaging with the Strategic Guiding Council: (1) engaging in reciprocal learning; (2) using lived experience to support other family carers ; and (3) creating a collective momentum for advocacy and change.
Theme 1. Engaging in reciprocal learning
This first theme explains how family advisors were motivated to be engaged with the Strategic Guiding Council and research team to help and also learn about health and social services from other care partner experiences. This was identified by one advisor who shared: “I have experiences that might be valuable, but other people on the council may have experiences that could help me as well” (P2, Canada). A family advisor expressed that engaging with health professionals on the research team (e.g., nurses, physicians, social workers) allowed for reciprocal learning, where the care partner learned from the health professional and the health professional had the chance to learn from caregivers:
I also very much enjoy the interaction of working with professionals [health]. I love the learning experience that both of us can get and ultimately again as we discussed before, what I learn I can then take back to the people who can gain the most from what I learn. Which are the people suffering the condition. (P5, United Kingdom)
By engaging with the Strategic Guiding Council, participants mentioned that it provided them with the opportunity to learn about interventions and programs that are happening in other countries. Family advisors further endorsed that being involved with the Strategic Guiding Council, not only did it promote reciprocal learning between the caregiver and healthcare team, it also allowed family advisors to be active participants in their loved one’s care and not “only visitors” (P6, The Netherlands).
Theme 2. Using lived experience to support other family carers
This theme describes the participants’ lived experience as a family carer of a person with dementia and how their experience was a driver for engaging with the Strategic Guiding Council. Based on their own experiences, family advisors expressed a strong desire to create a circle of moral and psychosocial support system for other carers. Advisors endorsed that their personal experience with a loved one gave them “the drive to do something” (P10, the Republic of Ireland). This revealed how they reflected on their previous experiences in supporting persons with dementia to inform necessary changes for other family carers. The advisor further elaborated: “I thought for anybody that’s going through it, you know I’m going to try and do something to help improve this”. This sentiment was further expressed by another participant:
I think just giving my personal experience that I’ve had in Long Term Care. I watched how my father lived and how his end of life was and just want to help in any way that I can for other families going through such a difficult time. (P1, Canada)
Family advisors expressed experiencing challenges with obtaining appropriate end-of-life care for their loved one due to clinicians working in care homes limited knowledge regarding a palliative approach to care, which contributed to negative experiences. One participant mentioned, “My mom’s GP [general practitioner] denied her Palliative Care because he said she didn’t have Cancer” (P9, The Republic of Ireland). The advisor further attributed that the lack of information from the physician led to feelings of frustration but then ‘sparked something within’, which led the participant to determine that: “rather than fighting the system, I educated myself. I just decided I would speak out and tell mom’s story to change those mistakes and hopefully make a difference” (P9, The Republic of Ireland)
Another advisor expressed a similar situation where she experienced challenges with her husband receiving adequate pain management despite advocating on his behalf to stakeholders in his long-term care home and physician. The advisor shared:
With my husband, the home didn’t know anything about Palliative Care and we became increasingly desperate because he was having such terrible pain. Awful pain, and it was just awful to see his body twisting. I was so desperate. I tried to get him into a hospice and they had no beds and I turned to the Palliative Care nursing team that cover residents around the country side and towns. They said they can’t come in and help unless they are asked to by the GP that covers the residential home and I asked him [GP] and he wouldn’t speak to me. (P5, United Kingdom)
After advocating on behalf of her husband for adequate end-of-life care, the advisor further shared:
It was only the day before he died that he was completely pain free. The last few days of his life he had some pain control. That shouldn’t have happened, he should have had pain control months before, or weeks before he became in such a terrible state. That’s why I’m desperate that it doesn’t happen to other people. (P5, United Kingdom)
Although many family advisors shared negative experiences with end-of-life care, an advisor described end-of-life care as a “beautiful, worthwhile thing to be a part of” (P2, Canada). She further describes how end-of-life care has been a large part of her life since she was a caregiver to family members who passed away in LTC. Therefore, she hopes to be able to help others going through a difficult moment by sharing her experience:
It’s worthwhile to be able to share the things you know and share your experience with families to have when they are facing this end-of-life care phase. It’s a really difficult circumstance to be in and it’s very complex it’s nothing that you can rehearse for… I think that my experience could be valuable to other people. (P2, Canada)
A strong motivating factor that led advisors to engage with the Strategic Guiding Council and research team was to help others navigate the health system. Advisors expressed encountering challenges navigating the health system to ensure that their family member with dementia was provided with the treatment that they needed. Many endorsed a motivation to be engaged with the Strategic Guiding Council was to help other caregivers navigate the health system. An advisor shared: "I think it is in helping people find their way through different systems … as they begin to navigate it and know the important things to look for and what things to just let go" (P4, Canada).
In addition, an advisor also learned from her experience that it is important to seek help early on and engage in discussions about dying early on in a person’s care until they are at the end-of-life. She shared:
My mission is that what happened to my husband never happens to other people. They get to that stage and understand and get help quickly … definitely get help early on and leave it sort of until its Palliative and it can’t be controlled. They need to know earlier on. (P5, United Kingdom)
A family advisor further described supporting a family member with dementia as the power of attorney and described the process as not black or white. The advisor disclosed the challenges and complexities of being power of attorney, especially in relation with capacity of understanding concerns. Supporting families navigate these concerns was a motivating factor for the advisor to join the Strategic Guiding Council. One advisor expressed: “To think that I could help family members understand what might be ahead of them, I thought was interesting and useful…so that was the motivation” (P8, The Republic of Ireland). Overall, participants identified the importance of sharing their knowledge and experience as a means of enlightening others and helping other carers in their caregiving journey.
Theme 3. Creating a collective momentum for advocacy and change
This theme describes the importance of advocacy to improve access to palliative care supports and education to reduce stigma in end-of-life care. Family advisors are motivated to be engaged with the Strategic Guiding Council to be able to use their voice to improve the quality of care provided to individuals with dementia and use their voices to “support or influence the direction of the program (P7, The Republic of Ireland). An advisor shared:
Advocate for elderly people to have Comfort Care, even if it is not in the last part of their lives. They have to have Comfort Care even when the disease of Dementia comes, to have all the people around you and try to make the life of the person as comfortable as can be. (P6, The Netherlands)
An advisor shared how being involved in their loved ones care brought fulfillment in their life through the advocacy work they are engaged in:
I look back now and I think it’s probably the best thing I have achieved in my life. Plus, it’s opened up my world to meeting amazing people. Like even now talking to you today [researcher], like if mom hadn’t come into my life, I wouldn’t be doing any of this [advocacy]. I don’t’ know what I’d be doing but I certainly wouldn’t’ be worrying about Dementia and end of life or having anything to do with it. It’s completely the opposite to what my life was. (P9, The Republic of Ireland)
Despite programs developed to bring more awareness regarding dementia care, advisors endorsed that there is still stigma that exists regarding dementia.
I don’t know about other countries but in the Republic of Ireland there’s not as much of a stigma as there was but there’s definitely still a stigma around Dementia or Alzheimer’s. There’s definitely huge education needed for the general public as well. It’s getting better. People are more aware, as well as there are more supports. But there’s still … you know, it’s one of those things that some people don’t want to talk about. (P10, The Republic of Ireland)
The Strategic Guiding Council was identified as an opportunity for advisors to be engaged in research initiatives and cross-country collaborations to improve integration of a palliative approach to care for individuals with dementia. An advisor who also had a long career in research shared:
I like the idea that it’s an international project and it will be interesting to see what the results are and also to make a difference for families that have family members or friends who have Dementia and reach end of life. It would be nice to make a difference if we can somehow. I like fairly efficient things, but I want to see some real impact you know collectively from this research project. I’ve been doing research for forty-five years so I know that lots of things take a long time to make a difference but I’d like to see some real outcomes from the research. (P3, Canada)
Through engagement in the Strategic Guiding Council and research team, a collective momentum for change can be made to improve the care being provided to people with dementia and provide person-centered care. An advisor emphasized the importance for health professionals to explore the wishes of the person with dementia and their family members to improve quality of life:
The nurses and the staff must be aware of the wishes of patients and family of the patients so they can be part of the last few times [moments] of the patient’s life and try to make it more about the person and family want to have and not the wishes of the organization to be efficien.t (P6, The Netherlands)
This study sought to explore the perspectives of family advisors’ motivations in working with a Strategic Guiding Council and research team to promote integration of a palliative approach to improve dementia care in LTC homes. To our knowledge, this is the first study which explores the perspectives of family carers motivations to participate in PPI research in an international Strategic Guiding Council, from four participating countries (Canada, United Kingdom, Republic of Ireland, and the Netherlands). The Strategic Guiding Council international study provided the advisors with an opportunity to be engaged in cross-country collaborations to improve end-of-life care for individuals with dementia and support the research team in determining opportunities to improve patient and family engagement.
The study findings are relevant to healthcare as it provides the perspectives of family carers motivation in being partners in care and research, which is an area of research that requires further exploration in LTC settings [ 18 ]. Involving family and patients in the development and implementation of research, enriches the relevance of the study by generating interventions that are sustainable and translatable to the community of interest [ 25 ]. PPI involvement is a growing area in health research and ensures that research is conducted in a manner that is relevant and meaningful to patients and the public [ 26 , 27 ]. Warner et al. [ 28 ] emphasizes the importance of involving patients and families in their care to ensure care reflects their needs and values. Through patient and family integration in the planning process, it can lead to positive patient end-of-life and family bereavement outcomes [ 28 ].
Family advisors in the study discussed how their lived experience providing care for a person with dementia was a motivating factor to wanting to be engaged with a Strategic Guiding Council and research team. Participants wanted to use their experience to help others who are going through difficult situations. Similar to the findings in our study, a study conducted by Cashin et al. [ 29 ] explored family’s motivation to being engaged in research and education development with health professionals, which was driven by their desire to improve their knowledge and promote awareness of dementia. Findings from the study are also consistent with family centered research that has been conducted in different disciplines. O’Keeffe et al. [ 30 ] explored family members motivation to being engaged in research in the field of adolescent mental health. A commonly cited motivation for being engaged with a research team was a desire to help others going through similar difficulties [ 30 ].
Advisors in the study discussed challenges with obtaining appropriate referral for end-of-life care or palliative treatment (i.e., pain management) for their loved one which was a driver for them to want to be engaged in a Strategic Guiding Council. Advisors reported stakeholders in care homes and health professionals limited knowledge regarding a palliative approach to care as a reason for experiencing challenges with obtaining a referral or treatment. This finding is consistent in the literature which discusses the need to expand education on end-of-life care amongst health professionals of all disciplines, patients and family [ 31 , 32 , 33 ]. In a study from Bolt et al. [ 34 ], the author emphasizes that nurses who provide care to people with dementia have a crucial role in identifying end-of-life needs and observing changes in clinical health status due to their frequent interactions with residents. However, it is imperative to explore effective education opportunities to promote capacity building within health professionals working in long-term care homes to provide a palliative approach to care to improve the health service delivery of older adults receiving end-of-life care.
PPI research is critical and it is imperative that researchers aim to achieve actionable outcomes with the participation of patients and public participation in research [ 11 ]. Advisors in the study disclosed how they want to be involved with the research team to be able to ‘see some real impact’ from the research projects they are engaged with. In a systematic review conducted by Domecq et al. [ 35 ], patient engagement in the planning and execution of research may improve its translation into clinical practice and may yield meaningful reports that’s written in a language that is understandable for participants and the community, thus supporting knowledge translation. Increasing participatory action research amongst patients and family to improve end-of-life careand dementia care may improve a person’s quality of life through the inclusion of individuals with lived experience to make program improvement recommendations [ 11 ]. Thus, reinforcing the importance of an interdisciplinary and unified approach to research.
Results from this study generated implications for research, policy, and practice. Research and policy implications generated from the study further emphasizes the importance of ensuring PPI is integrated in LTC settings and international research projects to further improve the implementation of a palliative approach to care within nursing homes and improve the quality of life of residents in the late phase of their illness. Advisors in the Strategic Guiding Council supported the FCDS study and were actively involved in creating guides for families and education materials along with the research team. Practice implications from the study reinforces the importance of involving family members in the decision-making process of their loved one’s goals of care during end of life. Families who are not provided the opportunity to discuss their relative’s illness, prognosis, and treatment experience difficulties with decision-making processes, feel uncertainty about the illness and may also feel unprepared for their relative’s death [ 31 ]. Exploring the motivations of family carers allowed the research team to engage family carers meaningfully by ensuring that their perspectives and lived experiences were incorporated in the FCDS research study. In addition, this study further contributes to the limited body of literature that explores PPI research amongst family caregivers in LTC [ 18 , 19 ].
Strengths and limitations
A major strength of this study is that the Strategic Guiding Council includes advisors from four countries with unique contexts with regards to healthcare systems and available services and presents findings PPI research within an international team. Additional study strengths include strategies to enhance trustworthiness of the study findings which consisted of meeting with the research team to engage in debriefing to ensure reflexivity and conformability with the research findings. The authors in the study also utilized triangulation to ensure rigour, which allowed for the confirmation of the findings and different perspective with the phenomena of interest [ 36 ]. Despite the research study utilizing a small sample of individuals from participating countries, this study offered an international perspective that explored the motivation of family advisors’ in working with a Strategic Guiding Council and research team which has not be explored before. Future qualitative research could include a large sample size and more countries beyond Canada and Europe. Advisors had to be comfortable in speaking in English, however a ‘buddy’ such as a research coordinator was assigned to those who required some translation. Despite the use of a ‘buddy’, due to limited time and resources we were unable to recruit family advisors in Italy and Czech Republic. Therefore, we were unable to include advisors in the study due to their limited proficiency in English. Future research should have measures in place to allow participants to take part in interviews in the language of their choosing. This study was also conducted during the COVID-19 pandemic and therefore future research should explore the impact of the pandemic with family caregivers’ participation in research. Additionally, the findings reinforce that family caregivers are motivated to be active participants in their loved ones care and want to be involved in meaningful work through research engagement. Therefore, researchers should aim to explore further family caregivers role in research to support in recruitment and to conduct meaningful research to improve health service delivery for patients.
This study contributed to the enrichment of literature by exploring PPI in research from an international perspective. Motivations that led to engagement with the Strategic Guiding Council and research team consisted of family advisors’ using lived experience to support other family carers and the motivation to engage in reciprocal learning with other care partners and research professionals. While many Family Advisors had strong motivations to be engaged with the Strategic Guiding Council and research team, many endorsed aspirations to support care partners navigate through the complexity of the health system and create a collective momentum for advocacy and change to improve end of life dementia care through their involvement with the Strategic Guiding Council.
Data availability
No datasets were generated or analysed during the current study.
Abbreviations
Patient and Public Involvement
Strategic Guiding Council
L’Esperance A, O’Brien N, Gregoire A, Abelson J, Canfield C, Del Grande C, Dogba MJ, Fancott C, Levasseur MA, Loignon C, Majnemer A, Pomey MP, Rasiah J, Salsberg J, Santana M, Tremblay MC, Urquhart R, Boivin A. Developing a Canadian evaluation framework for patient and public engagement in research: a study protocol. Res Involv Engagem. 2021;7(1):10. https://doi.org/10.1186/s40900-021-00255-4 .
Article PubMed PubMed Central Google Scholar
Burgers JS, van der Weijden T, Bischoff EA. Challenges of research on person-centered care in general practice: a scoping review. Front Med. 2021;8(669491). https://doi.org/10.3389/fmed.2021.669491 .
Burns KK, Bellows M, Eigenseher C, Gallivan J. Practical’ resources to support patient and family engagement in healthcare decisions: a scoping review. BMC Health Serv Res. 2014;14(175):1–15. https://doi.org/10.1186/1472-6963-14-175 .
Article Google Scholar
Holroyd-Leduc J, Resin J, Barwich D, Elliot J, Huras P, Legare F, Mahoney M, Maybee A, McNeil H, Pullman D, Sawatzky R, Stolee P. Giving voice to older adults living with frailty and their family caregivers: engagement of older adults living with frailty in research, health care decision making and in health policy. Res Involv Engagem. 2016;2(23):1–19. https://doi.org/10.1186/s40900-016-0038-7 .
Patrick K, Kebbe M, Aubin D. A home for patient-oriented research. CMAJ. 2018;190(20):E607. https://doi.org/10.1503/cmaj.180587 .
South J, Fairfax P, Green E. Developing an assessment tool for evaluating community involvement. Health Expect. 2005;8(1):64–73. https://doi.org/10.1111/j.1369-7625.2004.00313.x .
Canadian Institute of Health Research. Strategy for patient-oriented research. [internet]. https://cihr-irsc.gc.ca/e/41204.html (2022). Accessed 4 Jan 2022.
Loud F, Jain N, Thomas N. How to develop a patient and carer advisory group in a quality improvement study. J Ren Care. 2013;39(2):1–9. https://doi.org/10.1111/j.1755-6686.2013.12032 .
Jun M, Manns B, Laupacis A, Manns L, Rehal B, Crowe S, Hemmelgarn BR. Assessing the extent to which current clinical research is consistent with patient priorities: a scoping review using a case study in patients on or nearing dialysis. Can J Kidney Health Dis. 2015;2(35). https://doi.org/10.1186/s40697-1015-0070-9 .
Fox S, FitzGerald C, Dening KH, Irving K, Kernohan WG, Treloar A, Oliver D, Guerin S, Timmons S. Better palliative care for people with a dementia: summary of interdisciplinary workshop highlighting current gaps and recommendations for future research. BMC Palliat Care. 2018;17(1):1–11. https://doi.org/10.1186/s12904-017-0221-0 .
Bethell J, Commisso E, Rostad HM, Puts M, Babineau J, Grinbergs-Saull A, Wighton MB, Hammell J, Doyle E, Nadeau S, McGilton KS. Patient engagement in research related to dementia: a scoping review. Dementia. 2018;17(8):944–74. https://doi.org/10.1177/1471301218789292 .
Article PubMed Google Scholar
Bavelaar L, Visser M, Walshe C, Preston N, Kaasalainen S, Sussman T, Cornally N, Hartigan I, Loucka M, di Giulio P, Brazil K, Achterberg WP, van der Steen JT. The impact of the mySupport advance care planning intervention on family caregivers’ perceptions of decision-making and care for nursing home residents with dementia: pretest–post-test study in six countries. Age Ageing. 2023;52(3):1–9. https://doi.org/10.1093/ageing/afad027 .
Harding AJ, Doherty J, Bavelaar L, Walshe C, Preston N, Kaasalainen S, Sussman T, van der Steen JT, Cornally N, Hartigan I, Louka M, Vlckova K, Di Giulio P, Gonella S, Brazil K. A family carer decision support intervention for people with advanced dementia residing in a nursing home: a study protocol for an international advance care planning intervention (mySupport study). BMC Geriatr. 2022;22(1):1–10. https://doi.org/10.1186/s12877-022-03533-2 .
Brazil K, Walshe C, Doherty J, Harding AE, Preston N, Bavelaar L, Cornally N, Di Giulio P, Gonella S, Hartigan I. Implementation of an advance care planning intervention in nursing homes: an international multiple case study. Gerontologist. 2024;gnae007. https://doi.org/10.1093/geront/gnae007 .
Vellani S, Maradiaga VR, Nicula M, Lucchese S, Kruizinga J, Sussman T, Kaasalainen S. Palliative approach to care education for multidisciplinary staff of long-term care homes: a pretest post-test study. Gerontol Geriatr Med. 2023;9:1–9. https://doi.org/10.1177/23337214231158470 .
Bavelaar L, McCann A, Cornally N, Hartigan I, Kaasalainen S, Vankova H, Di Giulio P, Volicer L, Arcand M, van der Steen JT, Brazil K. mySupport study group. Guidance for family about comfort care in dementia: a comparison of an educational booklet adopted in six jurisdictions over a 15 year timespan. BMC Palliat Care. 2022;21(1):76. https://doi.org/10.1186/s12904-022-00962-z .
Bavelaar L, Nicula M, Morris S, Kaasalainen S, Achterberg WP, Loucka M, Vlckova K, Thompson G, Cornally N, Hartigan I, Harding A, Preston NJ, Walshe C, Cousins E, Harrison-Dening K, Devries K, Brazil K, van der Steen JT. Developing Country-specific questions about end-of-life care for nursing home residents with Advanced Dementia using the nominal group technique with Family caregivers. Patient Educ Couns. 2022;105(4):965–73. https://doi.org/10.1016/j.pec.2021.07.031 .
Yous ML, Connelly DM, Zhuang R, Hay ME, Garnett A, Hung L, Snobelen N, Gao H, Criferg K, Furlan-Craievich C, Snelgrove S. Perceptive responses and familiar staff facilitate meaningful engagement of older adults and family/care partners in long-term care home implementation science research during COVID-19. Patient Experience J. 2023;10(2):138.
Hung L, Berndt A, Wallsworth C, Horne N, Gregorio M. Involving patients and families in a social robot study. Patient Experience J. 2019;6(2):66–74. https://doi.org/10.35680/2372-0247.1362 .
Allard J, Ballesteros F, Anthony SJ, Dumez V, Hartell D, Knoll G, Wright L, Fortin MC. What does patient engagement mean for Canadian national transplant research program researchers? Res Involv Engagem. 2018;4(13). https://doi.org/10.1186/s40900-018-0096-0 .
Thorne S. Interpretive description: qualitative research for applied practice. 2nd ed. Routledge. Crossref;; 2016.
Robinson OC. Sampling in interview-based qualitative research: a theoretical and practical guide. Qual Res Psychol. 2014;11(1). https://doi.org/10.1080/14780887.2013.801543 .
Patton MQ. Qualitative research and evaluation methods. 4th ed. Washington, DC: Sage; 2015.
Google Scholar
Canadian Institute of Health Research. Tri-council policy statement: Ethical conduct for research involving humans. [internet]. https://ethics.gc.ca/eng/documents/tcps2-2018-en-interactive-final.pdf (2018). Accessed 4 Jan 2022.
Kwon SC, Tandon SD, Islam N, Riley L, Trinh-Shevrin C. Applying a community-based participatory research framework to patient and family engagement in the development of patient-centered outcomes research and practice. Transl Behav Med. 2018;8(5):683–91. https://doi.org/10.1093/tbm/ibx026 .
Miah J, Parsons S, Lovell K, Starling B, Leroi I, Dawes P. Impact of involving people with dementia and their care partners in research: a qualitative study. BMJ Open. 2020;10(e039321). https://doi.org/10.1136/bmjopen-2020-039321 .
Shippee ND, Garces JP, Lopez GJ, Wang Z, Elraiyah TA, Nabhan M, Brito JP, Boehmer K, Hasan R, Firwana B, Erwin PJ, Montori VM, Murad MH. Patient and service user engagement in research: a systematic review and synthesized framework. Health Expect. 2013;18(5):1151–66. https://doi.org/10.1111/hex.12090 .
Warner G, Baird LG, McCormack B, Urquhart R, Lawson B, Tschupruk C, Christian E, Weeks L, Kumanan K, Sampalli T. Engaging family caregivers and health system partners in exploring how multi-level contexts in primary care practices affect case management functions and outcomes of patients and family caregivers at end of life: a realist synthesis. BMC Palliat Care. 2021;20(114):1–30. https://doi.org/10.1186/s12904-021-00871-8 .
Cashin Z, Daley S, Hebditch M, Hughes L, Banerjee S. Involving people with dementia and their carers in dementia education for undergraduate healthcare professionals: a qualitative study of motivation to participate and experience. Int Psychogeriatr. 2019;31(6):869–76. https://doi.org/10.1017/S1041610218001357 .
O’Keeffe S, Weitkamp K, Issacs D, Target M, Eatough V, Midgley N. Parents’ understanding and motivation to take part in a randomized controlled trial in the field of adolescent mental health: a qualitative study. Trials. 2020;21(952):1–13. https://doi.org/10.1186/s13063-020-04857-3 .
Brazil K, Kaasalainen S, McAiney C, Brink P, Kelly ML. Knowledge and perceived competence among nurses caring for the dying in long-term care homes. Int J Palliat Nurs. 2012;18(2):77–83. https://doi.org/10.12968/ijpn.2012.18.2.77 .
Chen IH, Lin KY, Hu SH, Chuang YH, Long CO, Chang CC, Liu MF. Palliative care for advanced dementia: knowledge and attitudes of long-term care staff. J Clinc Nurs. 2018;3–4:848–58. https://doi.org/10.1111/jocn.14132 .
Luckett T, Luscombe G, Phillips J, Beattie E, Chenoweth L, Davidson PM, Goodall S, Pond D, Mitchell G, Agar M. (2021). Australian long-term care personnel’s knowledge and attitudes regarding palliative care for people with advanced dementia. Dementia. 2021; 20(2):427–443. https://doi.org/10.1177/1471301219886768
Bolt SR, van der Steen JT, Schols JM, Zwakhalen SM, Pieters S, Meijers JM. Nursing staffing needs in providing palliative care for people with dementia at home or in long-term care facilities: a scoping review. Int J Nurs Stud. 2019;96:143–52. https://doi.org/10.1016/j.ijnurstu.2018.12.011 .
Domecq JP, Prutsky G, Elraiyah T, Wang Z, Nabhan M, Shippee N, Brito JP, Boehmer K, Hasan R, Firwana B, Erwin P, Eton D, Sloan J, Montori V, Asi N, Dabrh AM, Murad MH. Patient engagement in research: a systematic review. BMC Health Serv Res. 2014;14(89):1–9. https://doi.org/10.1186/1472-6963-14-89 .
Carter N, Bryant-Lukosius D, DiCenso A, Blythe J, Neville AJ. The use of triangulation in qualitative research. Oncol Nurs Forum. 2014;41(5):545–7. https://doi.org/10.1188/14.ONF.545-54 .
Download references
Acknowledgements
The authors wish to thank all the members of the Strategic Guiding Council and all the international researchers in the mySupport study. This study was supported by EU Joint Programme—Neurodegenerative Disease (JPND) project. The project is supported through the following funding organisations under the aegis of JPND— (Canada, Canadian Institutes of Health Research; Czech Republic, Ministry of Education, Youth and Sport; Ireland, Health Research Board; The Netherlands, ZonMW; United Kingdom, Alzheimer’s Society)— www.jpnd.eu .
This is an EU Joint Programme -Neurodegenerative Disease Research (JPND) project. The project is supported through the following funding organisations under the aegis of JPND - www.jpnd.eu : Canada, Canadian Institutes of Health Research (grant number 161462); the Czech Republic, Ministry of Education, Youth and Sport (grant number 8F19005); Netherlands, Netherlands Organisation for Health Research and Development (grant number 733051084); Republic of Ireland, Health Research Board (grant number JPND- HSC-2018–002); the UK, Alzheimer’s Society (grant number AS-IGF-17–001). The funders had no role in the design of the study, and will have no role in its execution, analysis, interpretation of the data or write up.
Author information
Authors and affiliations.
Faculty of Health Sciences, School of Nursing, McMaster University, 1280 Main Street West, Hamilton, ON, L8S 3Z1, Canada
Stephanie Lucchese, Marie-Lee Yous, Julia Kruizinga, Vanessa Maradiaga Rivas, Pam Holliday, Rebecca Ganann & Sharon Kaasalainen
Lawrence S. Bloomberg, Faculty of Nursing, University of Toronto, 155 College Street, Toronto, ON, M5T 1P8, Canada
Stephanie Lucchese, Shirin Vellani & Danielle Just
Virtual Behavioral Medicine Program, Toronto Rehabilitation Institute – University Health Network, 550 University Avenue, Toronto, ON, M5G 2A2, Canada
Shirin Vellani
Faculty of Arts, School of Social Work, McGill University, 550 Sherbrooke Ouest H3A B9, Montreal, QC, H3A 1B9, Canada
Bianca Tétrault & Tamara Sussman
Dementia Ireland Keeping the Spotlight on Dementia and End of Life - Oughterard, Co. Galway, Ireland
Carmel Geoghegan
You can also search for this author in PubMed Google Scholar
Contributions
SK is the principal investigator of the study. SL, JK, SV, VM and SK were involved in data analysis. SL and ML drafted the initial version of the manuscript. All authors (SK, SL, ML, JK, SV, VM, BT, PH, CG, DJ, TS, RG) have read, made critical revisions, and approved the final manuscript.
Authors' information
Faculty of Health Sciences, School of Nursing, McMaster University, 1280 Main Street West, Hamilton, Ontario, L8S 3Z1, CanadaStephanie Lucchese RN, MN, Marie-Lee Yous RN, PhD Julia Kruizinga RN, MScN Vanessa Maradiaga Rivas RN, MScN, Pam Holliday BSc(PT), MSc, Rebecca Ganann RN, PhD, Sharon Kaasalainen RN, PhD.
Lawrence S. Bloomberg, Faculty of Nursing, University of Toronto, 155 College Street, Toronto, Ontario, M5T 1P8, CanadaStephanie Lucchese RN, MN, Shirin Vellani NP, PhD, Danielle Just PhD.
Virtual Behavioral Medicine Program, Toronto Rehabilitation Institute – University Health Network, 550 University Avenue, Toronto, Ontario, M5G 2A2, Canada Shirin Vellani NP, PhD.
Faculty of Arts, School of Social Work, McGill University, 550 Sherbrooke Ouest H3A B9, Montreal, Quebec, H3A 1B9, CanadaBianca Tétrault BA, MSW, Tamara Sussman, MSW, PhD.
Dementia Ireland, Keeping the Spotlight on Dementia and End of Life - Oughterard, Co. Galway, Ireland Carmel Geoghegan
Corresponding author
Correspondence to Sharon Kaasalainen .
Ethics declarations
Ethics approval and consent to participate.
This study was approved by local research ethics board (#5837). Written and verbal informed consent was sought from all participants prior to engaging in the study.
Consent for publication
Not applicable.
Competing interests
The authors declare no competing interests.
Additional information
Publisher’s note.
Springer Nature remains neutral with regard to jurisdictional claims in published maps and institutional affiliations.
Electronic supplementary material
Below is the link to the electronic supplementary material.
Supplementary Material 1
Rights and permissions.
Open Access This article is licensed under a Creative Commons Attribution-NonCommercial-NoDerivatives 4.0 International License, which permits any non-commercial use, sharing, distribution and reproduction in any medium or format, as long as you give appropriate credit to the original author(s) and the source, provide a link to the Creative Commons licence, and indicate if you modified the licensed material. You do not have permission under this licence to share adapted material derived from this article or parts of it. The images or other third party material in this article are included in the article’s Creative Commons licence, unless indicated otherwise in a credit line to the material. If material is not included in the article’s Creative Commons licence and your intended use is not permitted by statutory regulation or exceeds the permitted use, you will need to obtain permission directly from the copyright holder. To view a copy of this licence, visit http://creativecommons.org/licenses/by-nc-nd/4.0/ .
Reprints and permissions
About this article
Cite this article.
Lucchese, S., Yous, ML., Kruizinga, J. et al. Motivations of family advisors in engaging in research to improve a palliative approach to care for persons living with dementia: an interpretive descriptive study. Res Involv Engagem 10 , 94 (2024). https://doi.org/10.1186/s40900-024-00623-w
Download citation
Received : 15 March 2024
Accepted : 10 August 2024
Published : 06 September 2024
DOI : https://doi.org/10.1186/s40900-024-00623-w
Share this article
Anyone you share the following link with will be able to read this content:
Sorry, a shareable link is not currently available for this article.
Provided by the Springer Nature SharedIt content-sharing initiative
- Patient oriented research
- Palliative care
- End-of-life care
- Long-term care
Research Involvement and Engagement
ISSN: 2056-7529
- General enquiries: [email protected]
- Share full article
For more audio journalism and storytelling, download New York Times Audio , a new iOS app available for news subscribers.

- Apple Podcasts
- Google Podcasts
The First Post-Affirmative Action Class Enters College
A supreme court ruling upended more than four decades of admissions practices. the extent of the change is now beginning to become clear..

Hosted by Katrin Bennhold
Featuring David Leonhardt
Produced by Stella Tan Alex Stern and Asthaa Chaturvedi
Edited by Liz O. Baylen Michael Benoist and Chris Haxel
Original music by Dan Powell Marion Lozano and Rowan Niemisto
Engineered by Chris Wood
Listen and follow ‘The Daily’ Apple Podcasts | Spotify | Amazon Music | YouTube | iHeartRadio
The Supreme Court’s decision to ban affirmative action last summer was expected to drastically change the demographics of college campuses around the country.
David Leonhardt, who has written about affirmative action for The Times, explains the extent and nature of that change as the new academic year gets underway.
On today’s episode

David Leonhardt , a senior writer who runs The Morning , The Times’s flagship daily newsletter.

Background reading
Two elite colleges have seen shifts in racial makeup after the affirmative action ban.
The Supreme Court decision last year rejected affirmative action programs at Harvard and North Carolina.
There are a lot of ways to listen to The Daily. Here’s how.
We aim to make transcripts available the next workday after an episode’s publication. You can find them at the top of the page.
Research help by Susan Lee.
The Daily is made by Rachel Quester, Lynsea Garrison, Clare Toeniskoetter, Paige Cowett, Michael Simon Johnson, Brad Fisher, Chris Wood, Jessica Cheung, Stella Tan, Alexandra Leigh Young, Lisa Chow, Eric Krupke, Marc Georges, Luke Vander Ploeg, M.J. Davis Lin, Dan Powell, Sydney Harper, Michael Benoist, Liz O. Baylen, Asthaa Chaturvedi, Rachelle Bonja, Diana Nguyen, Marion Lozano, Corey Schreppel, Rob Szypko, Elisheba Ittoop, Mooj Zadie, Patricia Willens, Rowan Niemisto, Jody Becker, Rikki Novetsky, Nina Feldman, Will Reid, Carlos Prieto, Ben Calhoun, Susan Lee, Lexie Diao, Mary Wilson, Alex Stern, Sophia Lanman, Shannon Lin, Diane Wong, Devon Taylor, Alyssa Moxley, Olivia Natt, Daniel Ramirez and Brendan Klinkenberg.
Our theme music is by Jim Brunberg and Ben Landsverk of Wonderly. Special thanks to Sam Dolnick, Paula Szuchman, Lisa Tobin, Larissa Anderson, Julia Simon, Sofia Milan, Mahima Chablani, Elizabeth Davis-Moorer, Jeffrey Miranda, Maddy Masiello, Isabella Anderson, Nina Lassam and Nick Pitman.
Katrin Bennhold is the Berlin bureau chief. A former Nieman fellow at Harvard University, she previously reported from London and Paris, covering a range of topics from the rise of populism to gender. More about Katrin Bennhold
David Leonhardt runs The Morning , The Times’s flagship daily newsletter. Since joining The Times in 1999, he has been an economics columnist, opinion columnist, head of the Washington bureau and founding editor of the Upshot section. He is the author of “Ours Was the Shining Future: The Story of the American Dream." More about David Leonhardt
Advertisement
Advertisement
Thermal deformation analysis of motorized spindle base on thermo-solid structure coupling theory
- Published: 05 September 2024
Cite this article
- Yan Li 1 , 2 ,
- Kejun Wu 1 , 2 ,
- Nan Wang 1 , 2 ,
- Zhuo Wang 3 ,
- Wenqiang Li 1 , 2 &
- Mohan Lei 1 , 2
Machining different types of complex parts requires changing speed of the motorized spindle to accommodate the various processes, resulting in temperature rise or decline and then causing thermal deformation of machine tool, which plays a significant impact on the machining accuracy of the parts. According to the Law of Conservation of Energy and Fourier's Law of Heat Conduction, the heat transfer mechanism of the motorized spindle is investigated. Based on the thermo-solid structure coupling theory, the analytic models of the temperature field and thermal deformation for the motorized spindle are established, and the thermal hysteresis phenomenon is explored by means of the case studies. The characteristic experiments were carried out under variable working conditions and the model parameters were identified using Genetic Algorithm (GA). The accuracy and effectiveness of the proposed models for predicting temperature and thermal deformation of the spindle are validated which is applicable to compensate the thermal errors. Moreover, compared with data-driven modeling approach, our study needs lesser data for parameter identification, greatly saving the computation time in modeling.
This is a preview of subscription content, log in via an institution to check access.
Access this article
Subscribe and save.
- Get 10 units per month
- Download Article/Chapter or eBook
- 1 Unit = 1 Article or 1 Chapter
- Cancel anytime
Price includes VAT (Russian Federation)
Instant access to the full article PDF.
Rent this article via DeepDyve
Institutional subscriptions
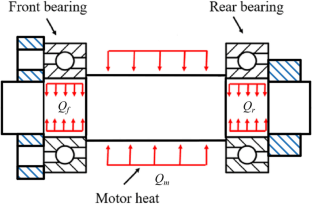
Similar content being viewed by others
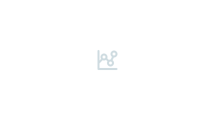
Thermal characteristic analysis of high-speed motorized spindle system based on thermal contact resistance and thermal-conduction resistance

Thermal error prediction model of a motorized spindle considering variable preload
Prediction model and experimental validation for the thermal deformation of motorized spindle, data availability.
No datasets were generated or analysed during the current study.
Abbreviations
Data-driven modeling
Physical modeling
Analytical model
Heat generation, J
Forced convection heat transfer coefficient, W/(m 2 ·K)
Heat dissipation area, m 2
Temperature, °C
Density of the motorized spindle, kg/m 3
Specific heat capacity of the motorized spindle, J/(kg·°C)
Volume of the motorized spindle, m 3
Running time of the motorized spindle, s
Integration constant
Position correction factor
Model parameters of temperature field
Coefficients of temperature field in the form of exponential function
Axial deformation, mm
The temperature variation corresponding to \(\Delta x_{i}\)
The length of the spindle, m
Model parameters of the deformation field
Thermal elongation of the motorized spindle, mm
Thermal expansion coefficient of material, K − 1
Heat conductivity coefficient, W/(m 2 ·K)
Shaft diameter, m
The axial position of the spindle, m
Front bearing
Rear bearing
Heat source
Li Y, Zhao W, Lan S et al (2015) A review on spindle thermal error compensation in machine Tools. Int J Mach Tool Manu 95:20–38. https://doi.org/10.1016/j.ijmachtools.2015.04.008
Article Google Scholar
Cao H, Zhang X, Chen X (2017) The concept and progress of intelligent spindles: A review Int J Mach Tool Manu 112:21–52. https://doi.org/10.1016/j.ijmachtools.2016.10.005
Mayr J, Jedrzejewski J, Uhlmann E et al (2012) Thermal issues in machine tools. CIRP Ann 61:771–791. https://doi.org/10.1016/J.CIRP.2012.05.008
Liu J, Ma C, Wang S et al (2019) Thermal-structure interaction characteristics of a high-speed spindle-bearing system. Int J Mach Tool Manu 137:42–57. https://doi.org/10.1016/j.ijmachtools.2018.10.004
Li Z, Zhu W, Wang B et al (2023) Optimization of cooling water jacket structure of high-speed electric spindle based on response surface method. Case Stud Therm Eng 48:103158. https://doi.org/10.1016/j.csite.2023.103158
Shi H, Qu Q, Mei X et al (2023) Robust modeling for thermal error of spindle of slant bed lathe based on error decomposition. Case Stud Therm Eng 51:103564. https://doi.org/10.1016/j.csite.2023.103564
Peng J, Yin M, Cao L et al (2022) Study on the spindle axial thermal error of a five-axis machining center considering the thermal bending effect. Precis Eng 75:210–226. https://doi.org/10.1016/j.precisioneng.2022.02.009
Li Y, Zhang Y, Zhao Y et al (2021) Thermal-mechanical coupling calculation method for deformation error of electric spindle of machine tool. Eng Fail Anal 128:105597. https://doi.org/10.1016/j.engfailanal.2021.105597
Uhlmann E, Saoji M, Peukert B (2016) Utilization of thermal energy to compensate quasistatic deformations in modular machine tool frames. Procedia CIRP 40:1–6. https://doi.org/10.1016/j.procir.01.037
Luo F, Ma C, Liu J et al (2023) Theoretical and experimental study on rotating heat pipe towards thermal error control of electric spindle. Int J Therm Sci 185:108095. https://doi.org/10.1016/j.ijthermalsci.2022.108095
Weng L, Gao W, Zhang D et al (2021) Analytical modelling method for thermal balancing design of machine tool structural components. Int J Mach Tool Manu 164:103715. https://doi.org/10.1016/j.ijmachtools.2021.103715
Bae W, Kim J, Cho S et al (2022) Suppression of thermal deformation of machine tool spindle using TiC-Fe composite. J Mech Sci Technol 36:2511–2520. https://doi.org/10.1007/s12206-022-0433-y
Kono D, Mizuno S, Muraki T et al (2019) A machine tool electric spindle with hybrid structure of steel and carbon fiber composite. CIRP Ann 68:389–392. https://doi.org/10.1016/j.cirp.2019.04.022
Wang Z, Zhang K, Wang Z et al (2021) Research on vibration of ceramic electric spindle influenced by interference and thermal displacement. J Mech Sci Technol 35:2325–2335. https://doi.org/10.1007/s12206-021-0505-4
Chen B, Guan X, Cai D et al (2022) Simulation on thermal characteristics of high-speed electric spindle. Case Stud Therm Eng 35:102144. https://doi.org/10.1016/j.csite.2022.102144
Li M, Zeng S, Hu J et al (2023) Free-mounted cooling plate multi-objective topology optimization method towards precision machine tool heat dissipation: An experimental and numerical study. Int J Heat Mass Tran 214:124394. https://doi.org/10.1016/j.ijheatmasstransfer.2023.124394
Shi X, Yang X, Chen G et al (2020) Thermal structure design optimization and temperature control for worm gear grinding machine using flood cooling technology. Int J Adv Manuf Tech 108:2419–2431. https://doi.org/10.1007/s00170-020-05600-7
Shi H, He B, Yue Y et al (2019) Cooling effect and temperature regulation of oil cooling system for ball screw feed drive system of precision machine tool. Appl Therm Eng 161:114150. https://doi.org/10.1016/j.applthermaleng.2019.114150
Morishima T, van Ostayen R, van Eijk J et al (2015) Thermal displacement error compensation in temperature domain. Precis Eng 42:66–72. https://doi.org/10.1016/j.precisioneng.2015.03.012
Zhou Z, Dai Y, Wang G et al (2022) Thermal displacement prediction model of SVR high-speed electric spindle based on SA-PSO optimization[J]. Case Stud Therm Eng 40:102551. https://doi.org/10.1016/j.csite.2022.102551
Dai Y, Pang J, Rui XK et al (2023) Thermal error prediction model of high-speed electric spindle based on DELM network optimized by weighted mean of vectors algorithm. Case Stud Therm Eng 47:103054. https://doi.org/10.1016/j.csite.2023.103054
Yin Q, Tan F, Chen H et al (2019) Spindle thermal error modeling based on selective ensemble BP neural networks. Int J Adv Manuf Tech 101:1699–1713. https://doi.org/10.1007/s00170-018-2994-6
Li B, Tian X, Zhang M (2019) Thermal error modeling of machine tool spindle based on the improved algorithm optimized BP neural network. Int J Adv Manuf Tech 105:1497–1505. https://doi.org/10.1007/s00170-019-04375-w
Yao X, Hu T, Yin G et al (2020) Thermal error modeling and prediction analysis based on OM algorithm for machine tool’s spindle. Int J Adv Manuf Tech 106:3345–3356. https://doi.org/10.1007/s00170-019-04767-y
Liu H, Miao E, Zhuang X et al (2018) Thermal error robust modeling method for CNC machine tools based on a split unbiased estimation algorithm. Precis Eng 51:169–175. https://doi.org/10.1016/j.precisioneng.2017.08.007
Li Z, Wang Q, Zhu B et al (2022) Thermal error modeling of high-speed electric spindle based on Aquila Optimizer optimized least squares support vector machine. Case Stud Therm Eng 39:102432. https://doi.org/10.1016/j.csite.2022.102432
Tan F, Yin M, Wang L et al (2018) Spindle thermal error robust modeling using LASSO and LS-SVM. Int J Adv Manuf Tech 94:2861–2874. https://doi.org/10.1007/s00170-017-1096-1
Dai Y, Pang J, Li Z et al (2022) Modeling of thermal error electric spindle based on KELM ameliorated by snake optimization. Case Stud Therm Eng 40:102504. https://doi.org/10.1016/j.csite.2022.102504
Ouerhani N, Loehr B, Rizzotti-Kaddouri A et al (2022) Data-driven thermal deviation prediction in turning machine-tool-a comparative analysis of machine learning algorithms. Procedia Comp Sci 200:185–193. https://doi.org/10.1016/j.procs.2022.01.217
Gui H, Liu J, Ma C et al (2023) New machine learning application platform for spatial–temporal thermal error prediction and control with STFGCN for ball screw system. Mech Syst Signal Process 192:110240. https://doi.org/10.1016/j.ymssp.2023.110240
Ngoc HV, Mayer JRR, Bitar-Nehme E (2022) Deep learning LSTM for predicting thermally induced geometric errors using rotary axes’ powers as input parameters. CIRP J Manuf Sci Technol 37:70–80. https://doi.org/10.1016/j.cirpj.2021.12.009
Li M, Ma C, Liu J et al (2024) Thermal error prediction of precision boring machine tools based on extreme gradient boosting algorithm-improved sailed fish optimizer-bi-directional ordered neurons-long short-term memory neural network model and physical-edge-cloud system. Eng Appl Artif Intell 127:107278. https://doi.org/10.1016/j.engappai.2023.107278
Wei X, Gao F, Li Y et al (2018) Study on optimal independent variables for the thermal error model of CNC machine tools. Int J Adv Manuf Tech 98:657–669. https://doi.org/10.1007/s00170-018-2299-9
Yang AS, Yu XH, Zhuang JR et al (2018) DOE-FEM based design improvement to minimize thermal errors of a high speed spindle system. Therm Sci Eng Prog 8:525–536. https://doi.org/10.1016/j.tsep.2018.10.011
Oyanguren A, Larranaga J, Ulacia I (2018) Thermo-mechanical modelling of ball screw preload force variation in different working conditions. Int J Adv Manuf Tech 97:723–739. https://doi.org/10.1007/s00170-018-2299-9
Zhang L, Xuan J, Shi T et al (2020) Robust, fractal theory, and FEM-based temperature field analysis for machine tool spindle. Int J Adv Manuf Tech 111:1571–1586. https://doi.org/10.1007/s00170-020-05926-2
Weng L, Gao W, Zhang D et al (2023) Analytical modelling of transient thermal characteristics of precision machine tools and real-time active thermal control method. Int J Mach Tool Manu 186:104003. https://doi.org/10.1016/j.ijmachtools.2023.104003
Li Z, Zhu W, Zhu B et al (2023) Simulation analysis model of high-speed electric spindle structure based on thermal load optimization. Case Stud Therm Eng 44:102871. https://doi.org/10.1016/j.csite.2023.102871
Gao W, Ibaraki S, Donmez MA et al (2023) Machine tool calibration: Measurement, modeling, and compensation of machine tool errors. Int J Mach Tool Manu. https://doi.org/10.1016/j.ijmachtools.2023.104017
Feng W, Li Z, Gu Q et al (2015) Thermally induced positioning error modelling and compensation based on thermal characteristic analysis. Int J Mach Tool Manu 9:26–36. https://doi.org/10.1016/j.ijmachtools.2015.03.006
Dai Y, Wang J, Li Z et al (2021) Thermal performance analysis and experimental study of high-speed electric spindle based on the gradient descent method. Case Stud Therm Eng 26:101056. https://doi.org/10.1016/j.csite.2021.101056
Shi X, Yang X, Yingjie Mu et al (2019) Thermal error compensation model for a electric spindle with shaft core cooling based on exponential function. Int J Adv Manuf Tech 103:4805–4813. https://doi.org/10.1007/s00170-019-04038-w
Creighton E, Honegger A, Tulsian A et al (2010) Analysis of thermal errors in a high-speed micro-milling spindle. Int J Mach Tool Manu 50:86–393. https://doi.org/10.1016/j.ijmachtools.2009.11.002
Shi Hu, Ma C, Yang J et al (2015) Investigation into effect of thermal expansion on thermally induced error of ball screw feed drive system of precision machine tools. Int J Mach Tool Manu 97:60–71. https://doi.org/10.1016/j.ijmachtools.2015.07.003
Jialan L, Chi Ma, Hongquan G et al (2021) Thermally-induced error compensation of spindle system based on long short term memory neural networks. Appl Soft Comput 102:107094. https://doi.org/10.1016/j.asoc.2021.107094
Liu J, Chi M, Wang S (2020) Data-driven thermal error compensation of linear x-axis of worm gear machines with error mechanism modeling. Mech Mach Theory 153:104009. https://doi.org/10.1016/j.mechmachtheory.2020.104009
Download references
This work was supported by the Shaanxi Province Modern Equipment Green Manufacturing Collaborative Innovation Center Project (No. 23JY054) and 'Leader Unveiling' projects for the industrialization of key core technologies in Shaanxi Province (No. 2205-610361-04-02-940891).
Author information
Authors and affiliations.
Key Lab of Mechanical Manufacturing Equipment of Shaanxi Province, Xi’an, 710048, China
Yan Li, Kejun Wu, Nan Wang, Wenqiang Li & Mohan Lei
School of Mechanical and Precision Instrument Engineering, Xi’an University of Technology, Xi’an, 710048, China
Xi’an Jingdiao Precision Mechanical Engineering Co., Ltd, Xi’an, 710048, China
You can also search for this author in PubMed Google Scholar
Contributions
All authors contributed to the study conception and design. Material preparation, data collection and analysis were performed by. Yan Li. The first draft of the manuscript was written by Ke-jun Wu. all authors commented on previous versions of the manuscript. All authors read and approved the final manuscript.
Corresponding author
Correspondence to Yan Li .
Ethics declarations
Competing interests.
The authors declare no competing interests.
Additional information
Publisher's note.
Springer Nature remains neutral with regard to jurisdictional claims in published maps and institutional affiliations.
Rights and permissions
Springer Nature or its licensor (e.g. a society or other partner) holds exclusive rights to this article under a publishing agreement with the author(s) or other rightsholder(s); author self-archiving of the accepted manuscript version of this article is solely governed by the terms of such publishing agreement and applicable law.
Reprints and permissions
About this article
Li, Y., Wu, K., Wang, N. et al. Thermal deformation analysis of motorized spindle base on thermo-solid structure coupling theory. Heat Mass Transfer (2024). https://doi.org/10.1007/s00231-024-03519-3
Download citation
Received : 30 May 2024
Accepted : 26 August 2024
Published : 05 September 2024
DOI : https://doi.org/10.1007/s00231-024-03519-3
Share this article
Anyone you share the following link with will be able to read this content:
Sorry, a shareable link is not currently available for this article.
Provided by the Springer Nature SharedIt content-sharing initiative
- Find a journal
- Publish with us
- Track your research

IMAGES
VIDEO
COMMENTS
The case study approach - PMC
What the Case Study Method Really Teaches
Case Study Methodology of Qualitative Research
Hypothesis Definition. In the context of a consulting interview, a hypothesis definition is "a testable statement that needs further data for verification". In other words, the meaning of a hypothesis is that it's an educated guess that you think could be the answer to your client's problem. A hypothesis is therefore not always true.
A hypothesis-driven approach in consulting is a structured method of problem-solving. Consultants formulate a hypothesis for the solution to a business problem, then gather data to support or disprove it. Cracking a case interview can be a daunting task, with a wide range of potential solutions and approaches to consider.
Discover real-world case studies of Data-Driven Decision-Making (DDDM) and how companies like Netflix, Amazon, and Starbucks leveraged data for success. ... Case Study 2: Amazon's Supply Chain Optimization. ... This data-driven approach helps retailers create a more sustainable and prosperous expansion plan, resulting in long-term prosperity ...
The Advantages of Data-Driven Decision-Making - HBS Online
Machine learning in project analytics: a data-driven ...
Voorhees, E. M., Harman, D. K. & NIST (2005) TREC: Experiment and Evaluation in Information Retrieval, USA, MIT Press. rEvaluating Unstructured Information Management: A case study driven approach ...
Six steps to approaching a case study
This book explores a new realm in data-based modeling with applications to hydrology. Pursuing a case study approach, it presents a rigorous evaluation of state-of-the-art input selection methods on the basis of detailed and comprehensive experimentation and comparative studies that employ emerging hybrid techniques for modeling and analysis.
With the prevailing efforts to combat the coronavirus disease 2019 (COVID-19) pandemic, there are still uncertainties that are yet to be discovered about its spread, future impact, and resurgence. In this paper, a data-driven approach has been adopted in distilling the hidden information about COVID-19 and its symptoms.
Optimizing Inventory Management (A Real-Life Case Study): A Data-Driven Approach Objectives and Relevance. The primary focus of the project is on deriving insights into inventory trends and ...
About the Case Study. This case study is part of a series of MIT SMR-produced stories exploring the analytically driven organization. This story is based, in part, on interviews with Nedbank executives. Both the featured company and the case study sponsor reviewed this case study prior to publication.
The case study approach | BMC Medical Research Methodology
The case studies gathered and presented here tell, in a sense, a single story. It's the story of a "management revolution," brought about by the widespread adoption of big data and analytics in both the public and private sectors.1 In these dispatches from the front lines of that revolution, we see four strikingly dissimilar organizations — a health care system, a bank, a major ...
The classification goal is to predict if the client will subscribe a term deposit (target variable y). This case study is inspired by this research paper where the researchers have used a very similar dataset as the one we will be using throughout this case study for determining the success of Bank Telemarketing. The researchers in their paper ...
This case study is part of a series of MIT SMR-produced stories exploring the analytically driven organization. This story is based, in part, on interviews with public- and private-sector executives. ... The distinction led to a less top-down, engineering-driven approach to working with different groups, including the creation of an Energy ...
The insights-driven approach to AR has many benefits like providing more realistic AR metrics, offering competitive intel back to the business, and making analysts feel heard and valued.But sometimes other benefits of this approach aren't realized until you're in the moment capturing insights and surfacing issues you may have otherwise easily overlooked.
People-Driven-Change: an example. Pre Covid-19, a transformation team that I led in a UK market research agency ran a working session on 'systems and automation' as a part of a launch week for a new strategy. During the session those who had volunteered to attend discussed ideas around the 'leveraging technology' strategic priority.
The data used in this case study is sourced from Kaggle, a popular platform for data science and machine learning enthusiasts. The dataset, titled " Netflix Movies and TV Shows," is publicly available on Kaggle and provides valuable information about the movies and TV shows on the Netflix streaming platform.
Half an hour into the session and he moved on to explaining the data driven approach via four case studies. A section that most of us were waiting for given the majority of digital natives in the ...
A comprehensive approach to ecological functional zoning in the Shenzhen region of China is presented in this study. Through the integration of advanced geospatial analysis tools, multiple data sources, and sophisticated statistical techniques, different ecological functions have been identified and categorized based on a comprehensive set of indicators and spatial analysis techniques.
Enterprise Benefit Gain Requires An Enterprise Approach To Contract Management . ... In the 20 months since then, CLM has evolved further from use case-driven solutions for buy-side and sell-side contracts toward more comprehensive support for enterprisewide contracting. This is due to the following factors:
The second case study aims to perform a multi-objective operational reliability assessment on a braking system. In this study, the proposed ADSCA (which stands for decomposition-synchronization-coordination strategy and adaptive surrogate modeling theory) is applied to derive the CSORA. 5.1. System working principle and key influence parameters
A Strategic Guiding Council (SGC) was created within a Family Carer Decisions Support study, to engage family carers of persons with advanced dementia as advisors to inform the design and implementation of the study. The SGC consists of an international group of family advisors from Canada, the Republic of Ireland, the United Kingdom, the Netherlands, and the Czech Republic.
The family office landscape is evolving rapidly, driven by new wealth dynamics and global expansion. This edition of the Family Office Insight Series delves into the world of family offices—what they are, how they are increasing in number, their future, approaches to hiring and board membership, and much more. It offers fresh insights into their growing presence, asset base, and impact, as ...
France's Far Right at the Gates of Power - The New York ...
The accuracy and effectiveness of the proposed models for predicting temperature and thermal deformation of the spindle are validated which is applicable to compensate the thermal errors. Moreover, compared with data-driven modeling approach, our study needs lesser data for parameter identification, greatly saving the computation time in modeling.