Information
- Author Services

Initiatives
You are accessing a machine-readable page. In order to be human-readable, please install an RSS reader.
All articles published by MDPI are made immediately available worldwide under an open access license. No special permission is required to reuse all or part of the article published by MDPI, including figures and tables. For articles published under an open access Creative Common CC BY license, any part of the article may be reused without permission provided that the original article is clearly cited. For more information, please refer to https://www.mdpi.com/openaccess .
Feature papers represent the most advanced research with significant potential for high impact in the field. A Feature Paper should be a substantial original Article that involves several techniques or approaches, provides an outlook for future research directions and describes possible research applications.
Feature papers are submitted upon individual invitation or recommendation by the scientific editors and must receive positive feedback from the reviewers.
Editor’s Choice articles are based on recommendations by the scientific editors of MDPI journals from around the world. Editors select a small number of articles recently published in the journal that they believe will be particularly interesting to readers, or important in the respective research area. The aim is to provide a snapshot of some of the most exciting work published in the various research areas of the journal.
Original Submission Date Received: .
- Active Journals
- Find a Journal
- Proceedings Series
- For Authors
- For Reviewers
- For Editors
- For Librarians
- For Publishers
- For Societies
- For Conference Organizers
- Open Access Policy
- Institutional Open Access Program
- Special Issues Guidelines
- Editorial Process
- Research and Publication Ethics
- Article Processing Charges
- Testimonials
- Preprints.org
- SciProfiles
- Encyclopedia
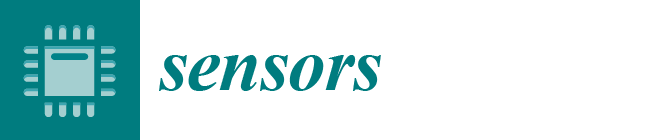
Article Menu
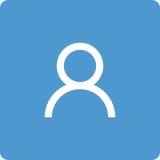
- Subscribe SciFeed
- Recommended Articles
- PubMed/Medline
- Google Scholar
- on Google Scholar
- Table of Contents
Find support for a specific problem in the support section of our website.
Please let us know what you think of our products and services.
Visit our dedicated information section to learn more about MDPI.
JSmol Viewer
Dynamic traffic assignment model based on gps data and point of interest (poi) in shanghai.
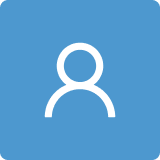
1. Introduction
2. materials and methods.
- Determine the initial value. Initial point x 0 ∈ S , given an error ε > 0 , k = 0 ;
- Solve the approximate linear programming: min ∇ f ( x k ) T x , s . t x ∈ S , to obtain the optimal solution y k ;
- Construct feasible descent directions, let d k = y k − x k , if | | ∇ f ( x k ) T x | | ≤ ε , stop the computation and output x k ; otherwise go to the next step.
- One-dimensional search: min 0 ≤ λ ≤ 1 f ( x k + λ d k ) to get step λ k . Let x k + 1 = x k + λ k d k , k updated to k + 1 , go to the second step.
3. Experiments
3.1. data description, 3.2. performance indexes, 4. interpretation of results, 4.1. the results of poi impact, 4.1.1. qualitative analysis, 4.1.2. qualitative analysis, 4.2. the results of user equilibrium model, 5. conclusions, author contributions, institutional review board statement, informed consent statement, data availability statement, conflicts of interest.
- Shanghai Research Institute. 2020 Shanghai Comprehensive Transportation Annual Report ; Shanghai Research Institute of Urban-Rural Construction and Transportation Development: Shanghai, China, 2020. [ Google Scholar ]
- 2019 Annual Traffic Analysis Report for Major Cities in China ; Amap.com, State Information Center, Joint Laboratory for Future Transport and Urban Computing et al.: Beijing, China, 2019.
- Ashfaq, M.; Gu, Z.; Waller, S.T.; Saberi, M. Comparing Dynamic User Equilibrium and Noniterative Stochastic Route Choice in A Simulation-Based Dynamic Traffic Assignment Model: Practical Considerations for Large-Scale Networks. J. Adv. Transp. 2021 , 2021 , 6667335. [ Google Scholar ] [ CrossRef ]
- Fujita, M.; Yamada, S.; Murakami, S. Time Coefficient Estimation for Hourly Origin-Destination Demand from Observed Link Flow Based on Semidynamic Traffic Assignment. J. Adv. Transp. 2017 , 2017 , 6495861. [ Google Scholar ] [ CrossRef ]
- De Grange, L.; Marechal, M.; Gonzalez, F. A Traffic Assignment Model Based on Link Densities. J. Adv. Transp. 2019 , 2019 , 5282879. [ Google Scholar ] [ CrossRef ]
- Marshall, N.L. Forecasting the Impossible: The Status Quo Of Estimating Traffic Flows with Static Traffic Assignment and the Future of Dynamic Traffic Assignment. Res. Transp. Bus. Manag. 2018 , 29 , 85–92. [ Google Scholar ] [ CrossRef ]
- Kerner, B.S. Breakdown Minimization Principle Versus Wardrop’s Equilibria for Dynamic Traffic Assignment and Control in Traffic and Transportation Networks: A Critical Mini-Review. Phys. A Stat. Mech. its Appl. 2017 , 466 , 626–662. [ Google Scholar ] [ CrossRef ]
- Wang, Y.; Szeto, W.Y.; Han, K.; Friesz, T.L. Dynamic Traffic Assignment: A Review of The Methodological Advances for Environmentally Sustainable Road Transportation Applications. Transp. Res. Part B Methodol. 2018 , 111 , 370–394. [ Google Scholar ] [ CrossRef ]
- Raadsen, M.P.H.; Bliemer, M.C.J.; Bell, M.G.H. Aggregation, Disaggregation and Decomposition Methods in Traffic Assignment: Historical Perspectives and New Trends. Transp. Res. Part B Methodol. 2020 , 139 , 199–223. [ Google Scholar ] [ CrossRef ]
- Papamichail, I.; Bekiaris-Liberis, N.; Delis, A.I.; Manolis, D.; Mountakis, K.-S.; Nikolos, I.K.; Roncoli, C.; Papageorgiou, M. Motorway Traffic Flow Modelling, Estimation and Control With Vehicle Automation and Communication Systems. Annu. Rev. Control. 2019 , 48 , 325–346. [ Google Scholar ] [ CrossRef ]
- Chiu, Y.-C.; Bottom, J.; Mahut, M. Dynamic Traffic Assignment: A Primer ; Transportation Research Board of the national academies: Birsbane, Australia, 2011. [ Google Scholar ]
- Merchant, D.K.; Nemhauser, G.L. A Model and An Algorithm for the Dynamic Traffic Assignment Problems. Transp. Sci. 1978 , 12 , 183–199. [ Google Scholar ] [ CrossRef ]
- Friesz, T.L.; Luque, J.; Tobin, R.L.; Wie, B.-W. Dynamic Network Traffic Assignment Considered as A Continuous Time Optimal Control Problem. Oper. Res. 1989 , 37 , 893–901. [ Google Scholar ] [ CrossRef ]
- Meng, M.; Shao, C.; Wong, Y.D.; Zhang, J. A Multiclass, Multimodal Dynamic Traffic Assignment Model with Departure Time. Math. Probl. Eng. 2014 , 2014 , 812614. [ Google Scholar ] [ CrossRef ]
- Zhao, C.-L.; Leclercq, L. Graphical Solution for System Optimum Dynamic Traffic Assignment with Day-Based Incentive Routing Strategies. Transp. Res. Part B Methodol. 2018 , 117 , 87–100. [ Google Scholar ] [ CrossRef ] [ Green Version ]
- Elimadi, M.; Abbas-Turki, A.; Koukam, A. A Novel Approach for Dynamic Traffic Assignment Based on Multi-Agent Node Reservation: Comparative Study on Two Competing Roads. Procedia Comput. Sci. 2021 , 191 , 320–327. [ Google Scholar ] [ CrossRef ]
- Zhang, H.; Seshadri, R.; Prakash, A.A.; Antoniou, C.; Pereira, F.C.; Ben-Akiva, M. Improving the Accuracy and Efficiency of Online Calibration for Simulation-Based Dynamic Traffic Assignment. Transp. Res. Part C Emerg. Technol. 2021 , 128 , 103195. [ Google Scholar ] [ CrossRef ]
- Banadaki, H.D.; Saffarzadeh, M.; Zoghi, H. Developing a Dynamic Traffic Assignment Model for Large-Scale Networks: A Case Study in the City of Yazd, Iran. KSCE J. Civ. Eng. 2021 , 25 , 3492–3501. [ Google Scholar ] [ CrossRef ]
- Lu, Q.; Tettamanti, T. Traffic Control Scheme for Social Optimum Traffic Assignment with Dynamic Route Pricing for Automated Vehicles. Period. Polytech. Transp. Eng. 2021 , 49 , 301–307. [ Google Scholar ] [ CrossRef ]
- Aghamohammadi, R.; Laval, J.A. Dynamic Traffic Assignment Using The Macroscopic Fundamental Diagram: A Review of Vehicular and Pedestrian Flow Models. Transp. Res. Part B Methodol. 2020 , 137 , 99–118. [ Google Scholar ] [ CrossRef ] [ Green Version ]
- Kamel, I.; Shalaby, A.; Abdulhai, B. Integrated Simulation-Based Dynamic Traffic and Transit Assignment Model for Large-Scale Network. Can. J. Civ. Eng. 2020 , 47 , 898–907. [ Google Scholar ] [ CrossRef ]
- Hu, T.Y.; Tong, C.C.; Liao, T.Y.; Chen, L.W. Dynamic Route Choice Behaviour and Simulation-Based Dynamic Traffic Assignment Model for Mixed Traffic Flows. J. Civ. Eng. 2017 , 22 , 1–10. [ Google Scholar ] [ CrossRef ]
- Zhang, H.; Zhang, Q.; Yang, L. A User Equilibrium Assignment Flow Model for Multiairport Open Network System. Math. Probl. Eng. 2015 , 2015 , 631428. [ Google Scholar ] [ CrossRef ] [ Green Version ]
- Zhang, Y.; Atasoy, B.; Akkinepally, A.; Ben-Akiva, M. Dynamic Toll Pricing Using Dynamic Traffic Assignment System with Online Calibration. Transp. Res. Rec. 2018 , 2673 , 532–546. [ Google Scholar ] [ CrossRef ]
- Mou, N.; Li, J.; Zhang, L.; Liu, W.; Xu, Y. Spatio-Temporal Characteristics of Resident Trip Based on POI and OD Data of Float Car in Beijing. ISPRS-Int. Arch. Photogramm. Remote. Sens. Spat. Inf. Sci. 2017 , XLII-2/W7 , 99–105. [ Google Scholar ] [ CrossRef ] [ Green Version ]
- Barlacchi, G.; Lepri, B.; Moschitti, A. Land Use Classification with Point of Interests and Structural Patterns. IEEE Trans. Knowl. Data Eng. 2021 , 33 , 3258–3269. [ Google Scholar ] [ CrossRef ]
- Niu, H.; Silva, E.A. Delineating Urban Functional Use from Points of Interest Data With Neural Network Embedding: A Case Study in Greater London. Comput. Environ. Urban Syst. 2021 , 88 , 101651. [ Google Scholar ] [ CrossRef ]
- Wang, Z.; Ma, D.; Sun, D.; Zhang, J. Identification and Analysis of Urban Functional Area in Hangzhou Based on OSM and POI Data. PLoS ONE 2021 , 16 , e0251988. [ Google Scholar ] [ CrossRef ]
- Zhang, T.; Sun, L.; Yao, L.; Rong, J. Impact Analysis of Land Use on Traffic Congestion Using Real-Time Traffic and POI. J. Adv. Transp. 2017 , 2017 , 7164790. [ Google Scholar ] [ CrossRef ] [ Green Version ]
- Gauglitz, P.; Geiger, D.; Ulffers, J.; Zauner, E. Modeling Public Charging Infrastructure Considering Points of Interest and Parking Potentials. Adv. Geosci. 2021 , 56 , 1–12. [ Google Scholar ] [ CrossRef ]
- Chen, Q.; Ye, T.; Zhao, N.; Ding, M.; Ouyang, Z.; Jia, P.; Yue, W.; Yang, X. Mapping China’s Regional Economic Activity by Integrating Points-of-Interest and Remote Sensing Data with Random Forest. Environ. Plan. B Urban Anal. City Sci. 2021 , 48 , 1876–1894. [ Google Scholar ] [ CrossRef ]
- Wei, Z.; Fu, C.W.; Arisona, S.M.; Schubiger, S.; Burkhard, R.; Ma, K.L. Visualizing the Relationship Between Human Mobility and Points of Interest. IEEE Trans. Intell. Transp. Syst. 2017 , 18 , 2271–2284. [ Google Scholar ]
- Xu, Z.; Cui, G.; Zhong, M.; Wang, X. Anomalous Urban Mobility Pattern Detection Based on GPS Trajectories and POI Data. ISPRS Int. J. Geo-Inf. 2019 , 8 , 308. [ Google Scholar ] [ CrossRef ] [ Green Version ]
- Yiyong, P.; Ting, Y.; Jianxiao, M. Improvement of Urban Road Impedance Function Based on Section Impedance and Node Impedance. J. Chongqing Jiaotong Univ. (Nat. Sci.) 2017 , 36 , 80–85. [ Google Scholar ]
Click here to enlarge figure
Flow | Congestion Level | Description | |
---|---|---|---|
(a) | 33 | Light | The red area of the link is small, and the road congestion is light. |
(b) | 65 | Heavy | The red area of the link is increasing, which means that parent pick-up vehicles are gathering. |
(c) | 52 | Heavy | The red area of the link decreases, because the parents’ pick-up vehicles are parked in front of the kindergarten waiting for the children to be released. The speed is null and is not counted in the track data, and this type of data points is not shown on the heat map. |
(d) | 71 | Heavy | The red area of the link increases as most of the parents have received their children. There is a short period of congestion caused by them leaving the link. |
(e) | 46 | Light | The red area of the link decreases, and the road is reopened. |
No. of Link | Link | Length (km) | Free Time (s) | Capacity | Flow | V/C |
---|---|---|---|---|---|---|
1 | [‘1’, ‘5’] | 0.41 | 0.0068 | 2000 | 213 | 0.106 |
2 | [‘1’, ‘6’] | 0.62 | 0.0103 | 2000 | 159 | 0.08 |
3 | [‘2’, ‘5’] | 0.84 | 0.014 | 2000 | 125 | 0.062 |
4 | [‘2’, ‘3’] | 0.64 | 0.0107 | 1000 | 104 | 0.104 |
5 | [‘3’, ‘2’] | 0.64 | 0.0107 | 1000 | 48 | 0.048 |
6 | [‘3’, ‘6’] | 0.93 | 0.0155 | 2000 | 75 | 0.038 |
7 | [‘3’, ‘4’] | 0.67 | 0.0112 | 1000 | 47 | 0.047 |
8 | [‘5’, ‘2’] | 0.84 | 0.014 | 2000 | 244 | 0.122 |
9 | [‘5’, ‘1’] | 0.41 | 0.0068 | 2000 | 89 | 0.044 |
10 | [‘5’, ‘6’] | 0.32 | 0.0053 | 1000 | 50 | 0.05 |
11 | [‘6’, ‘4’] | 0.55 | 0.0092 | 2000 | 95 | 0.048 |
12 | [‘6’, ‘1’] | 0.62 | 0.0103 | 2000 | 75 | 0.037 |
13 | [‘6’, ‘5’] | 0.32 | 0.0053 | 1000 | 0 | 0 |
14 | [‘6’, ‘3’] | 0.93 | 0.0155 | 2000 | 114 | 0.057 |
No. OD Pair | OD Pairs | Demand | No. of Path | Time [s] | Paths |
---|---|---|---|---|---|
0 | [‘1’, ‘1’] | 0 | 0 | 0 | [‘1’] |
1 | [‘1’, ‘2’] | 103 | |||
2 | 0.062 | [‘1’, ‘5’, ‘6’, ‘3’, ‘2’] | |||
3 | 0.0465 | [‘1’, ‘6’, ‘5’, ‘2’] | |||
4 | 0.0465 | [‘1’, ‘6’, ‘3’, ‘2’] | |||
2 | [‘1’, ‘3’] | 114 | 5 | 0.0465 | [‘1’, ‘5’, ‘2’, ‘3’] |
6 | 0.0465 | [‘1’, ‘5’, ‘6’, ‘3’] | |||
7 | 0.062 | [‘1’, ‘6’, ‘5’, ‘2’, ‘3’] | |||
3 | [‘1’, ‘4’] | 45 | 9 | 0.0775 | [‘1’, ‘5’, ‘2’, ‘3’, ‘6’, ‘4’] |
10 | 0.062 | [‘1’, ‘5’, ‘2’, ‘3’, ‘4’] | |||
11 | 0.0465 | [‘1’, ‘5’, ‘6’, ‘4’] | |||
12 | 0.062 | [‘1’, ‘5’, ‘6’, ‘3’, ‘4’] | |||
14 | 0.0775 | [‘1’, ‘6’, ‘5’, ‘2’, ‘3’, ‘4’] | |||
15 | 0.0465 | [‘1’, ‘6’, ‘3’, ‘4’] | |||
4 | [‘1’, ‘5’] | 110 | |||
17 | 0.031 | [‘1’, ‘6’, ‘5’] | |||
18 | 0.062 | [‘1’, ‘6’, ‘3’, ‘2’, ‘5’] | |||
5 | [‘2’, ‘1’] | 44 | |||
20 | 0.0465 | [‘2’, ‘5’, ‘6’, ‘1’] | |||
21 | 0.0465 | [‘2’, ‘3’, ‘6’, ‘1’] | |||
22 | 0.062 | [‘2’, ‘3’, ‘6’, ‘5’, ‘1’] | |||
6 | [‘2’, ‘2’] | 0 | 23 | 0 | [‘2’] |
7 | [‘2’, ‘3’] | 60 | 24 | 0.062 | [‘2’, ‘5’, ‘1’, ‘6’, ‘3’] |
25 | 0.0465 | [‘2’, ‘5’, ‘6’, ‘3’] | |||
8 | [‘2’, ‘4’] | 2 | 27 | 0.062 | [‘2’, ‘5’, ‘1’, ‘6’, ‘4’] |
28 | 0.0775 | [‘2’, ‘5’, ‘1’, ‘6’, ‘3’, ‘4’] | |||
29 | 0.0465 | [‘2’, ‘5’, ‘6’, ‘4’] | |||
30 | 0.062 | [‘2’, ‘5’, ‘6’, ‘3’, ‘4’] | |||
31 | 0.0465 | [‘2’, ‘3’, ‘6’, ‘4’] | |||
9 | [‘2’, ‘5’] | 51 | |||
34 | 0.062 | [‘2’, ‘3’, ‘6’, ‘1’, ‘5’] | |||
35 | 0.0465 | [‘2’, ‘3’, ‘6’, ‘5’] | |||
10 | [‘3’, ‘1’] | 75 | 36 | 0.0465 | [‘3’, ‘2’, ‘5’, ‘1’] |
37 | 0.062 | [‘3’, ‘2’, ‘5’, ‘6’, ‘1’] | |||
39 | 0.0465 | [‘3’, ‘6’, ‘5’, ‘1’] | |||
11 | [‘3’, ‘2’] | 18 | |||
41 | 0.062 | [‘3’, ‘6’, ‘1’, ‘5’, ‘2’] | |||
42 | 0.0465 | [‘3’, ‘6’, ‘5’, ‘2’] | |||
12 | [‘3’, ‘3’] | 0 | 43 | 0 | [‘3’] |
13 | [‘3’, ‘4’] | 45 | 44 | 0.0775 | [‘3’, ‘2’, ‘5’, ‘1’, ‘6’, ‘4’] |
45 | 0.062 | [‘3’, ‘2’, ‘5’, ‘6’, ‘4’] | |||
46 | 0.031 | [‘3’, ‘6’, ‘4’] | |||
14 | [‘3’, ‘5’] | 30 | |||
49 | 0.0465 | [‘3’, ‘6’, ‘1’, ‘5’] | |||
15 | [‘5’, ‘1’] | 45 | 51 | 0.062 | [‘5’, ‘2’, ‘3’, ‘6’, ‘1’] |
53 | 0.031 | [‘5’, ‘6’, ‘1’] | |||
16 | [‘5’, ‘2’] | 99 | |||
55 | 0.062 | [‘5’, ‘1’, ‘6’, ‘3’, ‘2’] | |||
56 | 0.0465 | [‘5’, ‘6’, ‘3’, ‘2’] | |||
17 | [‘5’, ‘3’] | 42 | |||
58 | 0.0465 | [‘5’, ‘1’, ‘6’, ‘3’] | |||
18 | [‘5’, ‘4’] | 50 | 60 | 0.062 | [‘5’, ‘2’, ‘3’, ‘6’, ‘4’] |
61 | 0.0465 | [‘5’, ‘2’, ‘3’, ‘4’] | |||
62 | 0.0465 | [‘5’, ‘1’, ‘6’, ‘4’] | |||
63 | 0.062 | [‘5’, ‘1’, ‘6’, ‘3’, ‘4’] | |||
65 | 0.0465 | [‘5’, ‘6’, ‘3’, ‘4’] | |||
19 | [‘5’, ‘5’] | 0 | 66 | 0 | [‘5’] |
Link | [‘1’, ‘5’] | [‘1’, ‘6’] | [‘2’, ‘5’] | [‘2’, ‘3’] | [‘3’, ‘2’] | [‘3’, ‘6’] | [‘3’, ‘4’] | [‘5’, ‘2’] | [‘5’, ‘1’] | [‘5’, ‘6’] | [‘6’, ‘4’] | [‘6’, ‘1’] | [‘6’, ‘5’] | [‘6’, ‘3’] |
---|---|---|---|---|---|---|---|---|---|---|---|---|---|---|
213 | 159 | 125 | 104 | 48 | 75 | 47 | 244 | 89 | 50 | 95 | 75 | 0 | 114 | |
149 | 124 | 92 | 104 | 52 | 91 | 81 | 205 | 87 | 127 | 142 | 122 | 86 | 165 |
Share and Cite
Song, X.; Yang, Z.; Wang, T.; Li, C.; Zhang, Y.; Chen, G. Dynamic Traffic Assignment Model Based on GPS Data and Point of Interest (POI) in Shanghai. Sensors 2021 , 21 , 7341. https://doi.org/10.3390/s21217341
Song X, Yang Z, Wang T, Li C, Zhang Y, Chen G. Dynamic Traffic Assignment Model Based on GPS Data and Point of Interest (POI) in Shanghai. Sensors . 2021; 21(21):7341. https://doi.org/10.3390/s21217341
Song, Xueying, Zheng Yang, Tao Wang, Chaoyang Li, Yi Zhang, and Ganyu Chen. 2021. "Dynamic Traffic Assignment Model Based on GPS Data and Point of Interest (POI) in Shanghai" Sensors 21, no. 21: 7341. https://doi.org/10.3390/s21217341
Article Metrics
Article access statistics, further information, mdpi initiatives, follow mdpi.
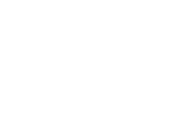
Subscribe to receive issue release notifications and newsletters from MDPI journals
- Architecture and Design
- Asian and Pacific Studies
- Business and Economics
- Classical and Ancient Near Eastern Studies
- Computer Sciences
- Cultural Studies
- Engineering
- General Interest
- Geosciences
- Industrial Chemistry
- Islamic and Middle Eastern Studies
- Jewish Studies
- Library and Information Science, Book Studies
- Life Sciences
- Linguistics and Semiotics
- Literary Studies
- Materials Sciences
- Mathematics
- Social Sciences
- Sports and Recreation
- Theology and Religion
- Publish your article
- The role of authors
- Promoting your article
- Abstracting & indexing
- Publishing Ethics
- Why publish with De Gruyter
- How to publish with De Gruyter
- Our book series
- Our subject areas
- Your digital product at De Gruyter
- Contribute to our reference works
- Product information
- Tools & resources
- Product Information
- Promotional Materials
- Orders and Inquiries
- FAQ for Library Suppliers and Book Sellers
- Repository Policy
- Free access policy
- Open Access agreements
- Database portals
- For Authors
- Customer service
- People + Culture
- Journal Management
- How to join us
- Working at De Gruyter
- Mission & Vision
- De Gruyter Foundation
- De Gruyter Ebound
- Our Responsibility
- Partner publishers

Your purchase has been completed. Your documents are now available to view.
Dynamic traffic assignment: model classifications and recent advances in travel choice principles
Dynamic Traffic Assignment (DTA) has been studied for more than four decades and numerous reviews of this research area have been conducted. This review focuses on the travel choice principle and the classification of DTA models, and is supplementary to the existing reviews. The implications of the travel choice principle for the existence and uniqueness of DTA solutions are discussed, and the interrelation between the travel choice principle and the traffic flow component is explained using the nonlinear complementarity problem, the variational inequality problem, the mathematical programming problem, and the fixed point problem formulations. This paper also points out that all of the reviewed travel choice principles are extended from those used in static traffic assignment. There are also many classifications of DTA models, in which each classification addresses one aspect of DTA modeling. Finally, some future research directions are identified.
[1] Gomes G., Horowitz R., Optimal freeway ramp metering using the asymmetric cell transmission model, Transport. Res. C-Emer., 2006, 14, 244–262 http://dx.doi.org/10.1016/j.trc.2006.08.001 10.1016/j.trc.2006.08.001 Search in Google Scholar
[2] Meng Q., Khoo H.L., A Pareto-optimization approach for a fair ramp metering, Transport. Res. C-Emer., 2010, 18, 489–506 http://dx.doi.org/10.1016/j.trc.2009.10.001 10.1016/j.trc.2009.10.001 Search in Google Scholar
[3] Park B.B., Yun I., Ahn K., Stochastic optimization for sustainable traffic signal control, Int. J. Sustain. Transp., 2009, 3, 263–284 http://dx.doi.org/10.1080/15568310802091053 10.1080/15568310802091053 Search in Google Scholar
[4] Park B.B., Kamarajugadda A., Development and evaluation of a stochastic traffic signal optimization method, Int. J. Sustain. Transp., 2007, 1, 193–207 http://dx.doi.org/10.1080/15568310600737568 10.1080/15568310600737568 Search in Google Scholar
[5] Lo H.K., Szeto W.Y., Modeling advanced traveler information services: static versus dynamic paradigms, Transport. Res. B-Meth., 2004, 38, 495–515 http://dx.doi.org/10.1016/j.trb.2003.06.001 10.1016/j.trb.2003.06.001 Search in Google Scholar
[6] Szeto W.Y., Lo H.K., The impact of advanced traveler information services on travel time and schedule delay costs, J. Intell. Transport. S., 2005, 9, 47–55 http://dx.doi.org/10.1080/15472450590916840 10.1080/15472450590916840 Search in Google Scholar
[7] Lo H.K., Szeto W.Y., Road pricing modeling for hypercongestion, Transport. Res. A-Pol., 2005, 39, 705–722 10.1016/j.tra.2005.02.019 Search in Google Scholar
[8] Zhong R.X., Sumalee A., Maruyama T., Dynamic marginal cost, access control, and pollution charge: a comparison of bottleneck and whole link models, J. Adv. Transport., 2011, Accepted 10.1002/atr.195 Search in Google Scholar
[9] Ukkusuri S., Waller S., Linear programming models for the user and system optimal dynamic network design problem: formulations, comparisons and extensions, Netw. Spat. Econ., 2008, 8, 383–406 http://dx.doi.org/10.1007/s11067-007-9019-6 10.1007/s11067-007-9019-6 Search in Google Scholar
[10] Szeto W.Y., Ghosh, B., Basu, B., O’Mahony M., Cell-based short-term traffic flow forecasting using time series modelling, Transport. Eng.-J. Asce., 2009, 135, 658–667 http://dx.doi.org/10.1061/(ASCE)0733-947X(2009)135:9(658) 10.1061/(ASCE)0733-947X(2009)135:9(658) Search in Google Scholar
[11] Abdelghany A.F., Adbelghany K.F., Mahmassani H.S., Murray P.M., Dynamic traffic assignment in design and evaluation of high-occupancy toll lanes, Transport. Res. Rec., 2000, 1733, 39–48 http://dx.doi.org/10.3141/1733-06 10.3141/1733-06 Search in Google Scholar
[12] Yagar S., Dynamic traffic assignment by individual path minimization and queuing, Transport. Res., 1971, 5, 179–196 http://dx.doi.org/10.1016/0041-1647(71)90020-7 10.1016/0041-1647(71)90020-7 Search in Google Scholar
[13] Cascetta E., Cantarella G.E., Modelling dynamics in transportation networks: state of the art and future developments, Simulat. Pract. Theory., 1993, 15, 65–91 http://dx.doi.org/10.1016/0928-4869(93)90017-K 10.1016/0928-4869(93)90017-K Search in Google Scholar
[14] Peeta S., Ziliaskopoulos A.K., Foundations of dynamic traffic assignment: the past, the present and the future, Netw. Spat. Econ., 2001, 1, 233–265 http://dx.doi.org/10.1023/A:1012827724856 10.1023/A:1012827724856 Search in Google Scholar
[15] Boyce D., Lee D.H., Ran B., Analytical models of the dynamic traffic assignment problem, Netw. Spat. Econ., 2001, 1, 377–390 http://dx.doi.org/10.1023/A:1012852413469 10.1023/A:1012852413469 Search in Google Scholar
[16] Szeto W.Y., Lo H.K., Dynamic traffic assignment: review and future, Information Technology, 2005, 5, 85–100 Search in Google Scholar
[17] Szeto W.Y., Lo H.K., Properties of dynamic traffic assignment with physical queues, J. East Asia Soc. Transport. Stud., 2005, 6, 2108–2123 Search in Google Scholar
[18] Mun J.S., Traffic performance models for dynamic traffic assignment: an assessment of existing models, Transport. Rev., 2007, 27, 231–249 http://dx.doi.org/10.1080/01441640600979403 10.1080/01441640600979403 Search in Google Scholar
[19] Jeihani M., A review of dynamic traffic assignment computer packages, J. Transport. Res. Forum, 2007, 46, 35–46 Search in Google Scholar
[20] Szeto W.Y., Cell-based dynamic equilibrium models, dynamic traffic assignment and signal control, in: Dynamic route guidance and traffic control, complex social, Economic and Engineered Networks Series, Ukkusuri S., Ozbay K. (Eds.), Springer, 2011, submitted Search in Google Scholar
[21] Wardrop J., Some theoretical aspects of road traffic research, ICE Proceedings: Part II, Engineering Divisions, 1952, 1, 325–362 http://dx.doi.org/10.1680/ipeds.1952.11259 10.1680/ipeds.1952.11259 Search in Google Scholar
[22] Oppenheim N., Urban travel demand modelling: from individual choice to general equilibrium, John Wiley & Sons, USA, 1995 Search in Google Scholar
[23] Daganzo C.F., Sheffi Y., On stochastic models of traffic assignment, Transport. Sci., 1977, 11, 253–274 http://dx.doi.org/10.1287/trsc.11.3.253 10.1287/trsc.11.3.253 Search in Google Scholar
[24] Hall R.W., Travel outcome and performance: the effect of uncertainty on accessibility, Transport. Res. BMeth., 1993, 17, 275–290 http://dx.doi.org/10.1016/0191-2615(83)90046-2 10.1016/0191-2615(83)90046-2 Search in Google Scholar
[25] Lo H.K., Luo X.W., Siu B.W.Y., Degradable transport network: travel time budget of travellers with heterogeneous risk aversion, Transport. Res. B-Meth., 2006, 40, 792–806 http://dx.doi.org/10.1016/j.trb.2005.10.003 10.1016/j.trb.2005.10.003 Search in Google Scholar
[26] Uchida T., Iida Y., Risk assignment: a new traffic assignment model considering the risk travel time variation, In: Daganzo C.F. (Ed.), Proceedings of the 12th International Symposium on Transportation and Traffic Theory (July 21–23, 1993, Berkeley, CA, USA), Elsevier, Amsterdam, 1993, 89–105 Search in Google Scholar
[27] Jackson W.B., Jucker J.V., An empirical study of travel time variability and travel choice behavior, Transport. Sci., 1982, 16, 460–475 http://dx.doi.org/10.1287/trsc.16.4.460 10.1287/trsc.16.4.460 Search in Google Scholar
[28] Brastow W.C., Jucker J.V., Use of a mean variance criterion for traffic assignment, unpublished manuscript, Department of Industrial Engineering, Stanford University, 1977 Search in Google Scholar
[29] Szeto W.Y., Solayappan M., Jiang Y., Reliabilitybased transit assignment for congested stochastic transit networks, Comput.-Aided Civ. Inf., 2011, 26, 311–326 http://dx.doi.org/10.1111/j.1467-8667.2010.00680.x 10.1111/j.1467-8667.2010.00680.x Search in Google Scholar
[30] Chen A., Zhou Z., The α-reliable mean-excess traffic equilibrium model with stochastic travel times, Transport. Res. B-Meth., 2010, 44, 493–513 http://dx.doi.org/10.1016/j.trb.2009.11.003 10.1016/j.trb.2009.11.003 Search in Google Scholar
[31] Franklin J.P., Karlstrom A., Travel time reliability for Stockholm roadways: modeling the mean lateness factor, Transport. Res. Rec., 2009, 2134, 106–113 http://dx.doi.org/10.3141/2134-13 10.3141/2134-13 Search in Google Scholar
[32] Chan K.S., Lam W.H.K., Impact of road pricing on the road network reliability, J. East Asia Soc. Transport. Stud., 2005, 6, 2060–2075 Search in Google Scholar
[33] Ordóñez F., Stier-Moses N.E., Wardrop equilibria with risk-averse users, Transport. Sci., 2010, 44, 63–86 http://dx.doi.org/10.1287/trsc.1090.0292 10.1287/trsc.1090.0292 Search in Google Scholar
[34] Lam T., Small K., The value of time and reliability: measurement from a value pricing experiment, Transport. Res. E-Log., 2001, 37, 231–251 http://dx.doi.org/10.1016/S1366-5545(00)00016-8 10.1016/S1366-5545(00)00016-8 Search in Google Scholar
[35] Bell M.G.H., Cassir C., Risk-averse user equilibrium traffic assignment: an application of game theory, Transport. Res. B-Meth., 2002, 36, 671–681 http://dx.doi.org/10.1016/S0191-2615(01)00022-4 10.1016/S0191-2615(01)00022-4 Search in Google Scholar
[36] Szeto W.Y., O’Brien L., O’Mahony M., Generalisation of the risk-averse traffic assignment, In: Bell M.G.H., Heydecker B.G., Allsop R.E. (Eds.), Proceedings of 17th International Symposium on Transportation and Traffic Theory (July 23–25, 2007, London, UK), Emerald Group Publishing Limited, 2007, 127–153 Search in Google Scholar
[37] Szeto W.Y., O’Brien L., O’Mahony M., Measuring network reliability by considering paradoxes: the multiple network demon approach, Transport. Res. Rec., 2009, 2090, 42–50 http://dx.doi.org/10.3141/2090-05 10.3141/2090-05 Search in Google Scholar
[38] Szeto W.Y., Cooperative game approaches to measuring network reliability considering paradoxes, Transport. Res. C-Emer., 2011, 11, 229–241 http://dx.doi.org/10.1016/j.trc.2010.05.010 10.1016/j.trc.2010.05.010 Search in Google Scholar
[39] Szeto W.Y., O’Brien L., O’Mahony M., Risk-averse traffic assignment with elastic demand: NCP formulation and solution method for assessing performance reliability, Netw. Spat. Econ., 2006, 6, 313–332 http://dx.doi.org/10.1007/s11067-006-9286-7 10.1007/s11067-006-9286-7 Search in Google Scholar
[40] Ordóñez F., Stier-Moses N.E., Robust Wardrop equilibrium, Netw. Control. Optim., 2007, 4465, 247–256 http://dx.doi.org/10.1007/978-3-540-72709-5_26 10.1007/978-3-540-72709-5_26 Search in Google Scholar
[41] Zhang C., Chen X., Sumalee A., Robust Wardrop’s user equilibrium assignment under stochastic demand and supply: expected residual minimization approach, Transport. Res. B-Meth., 2011, 45, 534–552 http://dx.doi.org/10.1016/j.trb.2010.09.008 10.1016/j.trb.2010.09.008 Search in Google Scholar
[42] Tversky A., Kahneman D., Advances in prospect theory: cumulative representation of uncertainty, J. Risk Uncertainty, 1992, 5, 297–323 http://dx.doi.org/10.1007/BF00122574 10.1007/BF00122574 Search in Google Scholar
[43] Kahneman D., Tversky A., Prospect theory: an analysis of decisions under risk, Econometrica, 1979, 47, 263–291 http://dx.doi.org/10.2307/1914185 10.2307/1914185 Search in Google Scholar
[44] Thaler R.H., Tversky A., Kahneman D., Schwartz A., The effect of myopia and loss aversion on risk taking: an experimental test, Quart. J. Econ., 1997, 112, 647–661 http://dx.doi.org/10.1162/003355397555226 10.1162/003355397555226 Search in Google Scholar
[45] Camerer C.F., Ho T.H., Violations of the betweenness axiom and nonlinearity in probability, J. Risk Uncertainty., 1994, 8, 167–196 http://dx.doi.org/10.1007/BF01065371 10.1007/BF01065371 Search in Google Scholar
[46] Avineri E., Prashker J.N., Violations of expected utility theory in route-choice stated preferences: certainty effect and inflating of small probabilities, Transport. Res. Rec., 2004, 1894, 222–229 http://dx.doi.org/10.3141/1894-23 10.3141/1894-23 Search in Google Scholar
[47] Avineri E., The effect of reference point on stochastic network equilibrium, Transport. Sci., 2006, 40, 409–420 http://dx.doi.org/10.1287/trsc.1060.0158 10.1287/trsc.1060.0158 Search in Google Scholar
[48] Mirchandani P., Soroush H., Generalized traffic equilibrium with probabilistic travel times and perceptions, Transport. Sci., 1987, 21, 133–152 http://dx.doi.org/10.1287/trsc.21.3.133 10.1287/trsc.21.3.133 Search in Google Scholar
[49] Shao H., Lam W.H.K., Tam M.L., A reliability-based stochastic traffic assignment model for network with multiple user classes under uncertainty in demand, Netw. Spat. Econ., 2006, 6, 173–204 http://dx.doi.org/10.1007/s11067-006-9279-6 10.1007/s11067-006-9279-6 Search in Google Scholar
[50] Chen A., Zhou Z., A stochastic α-reliable mean-excess traffic equilibrium model with probabilistic travel times and perception errors, In: Lam W.H.K., Wong S.C., Lo H.K. (Eds.), Proceedings of 18th International Symposium on Transportation and Traffic Theory 2009: Golden Jubilee (July 16–18, 2009), Springer, 2009, 117–145 Search in Google Scholar
[51] Chu Y.L., Work departure time analysis using dogit ordered generalized extreme value model, Transport. Res. Rec., 2009, 2132, 42–49 http://dx.doi.org/10.3141/2132-05 10.3141/2132-05 Search in Google Scholar
[52] Jou R.C., Kitamura R., Weng M.C., Chen C.C., Dynamic commuter departure time choice under uncertainty, Transport. Res. A-Pol., 2008, 42, 774–783 10.1016/j.tra.2008.01.017 Search in Google Scholar
[53] Lemp J.D., Kockelman K.M., Empirical investigation of continuous logit for departure time choice with Bayesian methods, Transport. Res. Rec., 2010, 2165, 59–68 http://dx.doi.org/10.3141/2165-07 10.3141/2165-07 Search in Google Scholar
[54] Lemp J.D., Kockelman K.M., Damien P., The continuous cross-nested logit model: Formulation and application for departure time choice, Transport. Res. B-Meth., 2010, 44, 646–661 http://dx.doi.org/10.1016/j.trb.2010.03.001 10.1016/j.trb.2010.03.001 Search in Google Scholar
[55] Friesz T.L., Luque F.J., Tobin R.L., Wie B.W., Dynamic network traffic assignment considered as a continuous time optimal control problem, Oper. Res., 1989, 37, 893–901 http://dx.doi.org/10.1287/opre.37.6.893 10.1287/opre.37.6.893 Search in Google Scholar
[56] Vickrey W.S., Congestion theory and transport investment, Am. Econ. Rev., 1969, 59, 251–261 Search in Google Scholar
[57] Mahmassani H.S., Herman R., Dynamic user equilibrium departure time and route choice on an idealized traffic arterials, Transport. Sci., 1984, 18, 362–384 http://dx.doi.org/10.1287/trsc.18.4.362 10.1287/trsc.18.4.362 Search in Google Scholar
[58] Merchant D.K., Nemhauser G.L., A model and an algorithm for the dynamic traffic assignment problem, Transport. Sci., 1978, 12, 183–199 http://dx.doi.org/10.1287/trsc.12.3.183 10.1287/trsc.12.3.183 Search in Google Scholar
[59] Small, K.A., The scheduling of consumer activities: work trips, Am. Econ. Rev., 1982, 72, 467–479 Search in Google Scholar
[60] Ran B., Boyce D.E., Modelling dynamic transportation networks: an intelligent transportation system oriented approach, 2nd rev. ed., Springer, Berlin, 1996 10.1007/978-3-642-80230-0_14 Search in Google Scholar
[61] Vythoulkas P.C., Two models for predicting dynamic stochastic equilibria in urban transportation networks, In: Koshi M. (Ed.), Proceedings of 11th International Symposium on Transportation and Traffic Theory (July 18–20, Yokohama, Japan), Elsevier, 1990, 253–272 Search in Google Scholar
[62] Boyce D.E., Ran B., Li I.Y., Considering travellers’ risk-taking behavior in dynamic traffic assignment, in: Bell M.G.H., Transportation networks: recent methodological advances, Elsevier, Oxford, 1999 Search in Google Scholar
[63] Szeto W.Y., Jiang Y, Sumalee A., A cell-based model for multi-class doubly stochastic dynamic traffic assignment, Comput-Aided Civ. Inf., 2011, 26, 595–611 http://dx.doi.org/10.1111/j.1467-8667.2011.00717.x 10.1111/j.1467-8667.2011.00717.x Search in Google Scholar
[64] Simon H.A., Models of bounded rationality (Vol. 3), The MIT Press, Cambridge, 1997 10.7551/mitpress/4711.001.0001 Search in Google Scholar
[65] Simon H.A., A behavioral model of rational choice, Quart. J. Econ., 1955, 69, 99–118 http://dx.doi.org/10.2307/1884852 10.2307/1884852 Search in Google Scholar
[66] Simon H.A., Models of Man, Wiley, New York, 1957 10.2307/2550441 Search in Google Scholar
[67] Mahmassani H.S., Chang G.L., On boundedly-rational user equilibrium in transportation systems, Transport. Sci., 1987, 21, 89–99 http://dx.doi.org/10.1287/trsc.21.2.89 10.1287/trsc.21.2.89 Search in Google Scholar
[68] Szeto W.Y., Lo H.K., Dynamic traffic assignment: properties and extensions, Transportmetrica, 2006, 2, 31–52 http://dx.doi.org/10.1080/18128600608685654 10.1080/18128600608685654 Search in Google Scholar
[69] Lou Y., Yin Y., Lawphongpanich S., Robust congestion pricing under boundedly rational user equilibrium, Transport. Res. B-Meth., 2010, 44, 15–28 http://dx.doi.org/10.1016/j.trb.2009.06.004 10.1016/j.trb.2009.06.004 Search in Google Scholar
[70] zeto W.Y., Lo H.K., A cell-based simultaneous route and departure time choice model with elastic demand, Transport. Res. B-Meth., 2004, 38, 593–612 http://dx.doi.org/10.1016/j.trb.2003.05.001 10.1016/j.trb.2003.05.001 Search in Google Scholar
[71] Pel A.J., Bliemer M.C.J., Hoogendoorn S.P., Hybrid route choice modeling in dynamic traffic assignment, Transport. Res. Rec., 2009, 2091, 100–107 http://dx.doi.org/10.3141/2091-11 10.3141/2091-11 Search in Google Scholar
[72] Kuwahara M., Akamatsu T., Decomposition of the reactive assignments with queues for many-to-many origin-destination pattern, Transport. Res. B-Meth., 1997, 31, 1–10 http://dx.doi.org/10.1016/S0191-2615(96)00020-3 10.1016/S0191-2615(96)00020-3 Search in Google Scholar
[73] Ben-akiva M., De Palma A., Kaysi, I., Dynamic network models and driver information system, Transport. Res. A-Pol., 1991, 25, 251–266 http://dx.doi.org/10.1016/0191-2607(91)90142-D 10.1016/0191-2607(91)90142-D Search in Google Scholar
[74] Sumalee A., Zhong, R.X., Pan, T.L., Szeto, W.Y., Stochastic cell transmission model (SCTM): a stochastic dynamic traffic model for traffic state surveillance and assignment, Transport. Res. B-Meth., 2011, 45, 507–533 http://dx.doi.org/10.1016/j.trb.2010.09.006 10.1016/j.trb.2010.09.006 Search in Google Scholar
[75] Lo H.K., Szeto W.Y., A cell-based dynamic traffic assignment model: formulation and properties, Math. Comput. Model., 2002, 35, 849–865 http://dx.doi.org/10.1016/S0895-7177(02)00055-9 10.1016/S0895-7177(02)00055-9 Search in Google Scholar
[76] Tong C.O., Wong S.C., A predictive dynamic traffic assignment model in congested capacity-constrained road networks, Transport. Res. B-Meth., 2000, 34, 625–644 http://dx.doi.org/10.1016/S0191-2615(99)00045-4 10.1016/S0191-2615(99)00045-4 Search in Google Scholar
[77] Horowitz J.L., The stability of stochastic equilibrium in a two-link transportation network, Transport. Res. B-Meth., 1984, 18, 13–28 http://dx.doi.org/10.1016/0191-2615(84)90003-1 10.1016/0191-2615(84)90003-1 Search in Google Scholar
[78] Cascetta E., A stochastic process approach to the analysis of temporal dynamics in transportation networks, Transport. Res. B-Meth., 1989, 23, 1–17 http://dx.doi.org/10.1016/0191-2615(89)90019-2 10.1016/0191-2615(89)90019-2 Search in Google Scholar
[79] Chang G.L., Mahmassani H., Travel time prediction and departure time adjustment behavior dynamics in a congested traffic system, Transport. Res. B-Meth., 1988, 22, 217–232 http://dx.doi.org/10.1016/0191-2615(88)90017-3 10.1016/0191-2615(88)90017-3 Search in Google Scholar
[80] Lam W.H.K., Huang H.J., Dynamic user optimal traffic assignment model for many to one travel demand, Transport. Res. B-Meth., 1995, 29, 243–259 http://dx.doi.org/10.1016/0191-2615(95)00001-T 10.1016/0191-2615(95)00001-T Search in Google Scholar
[81] Ran B., Boyce D., A link-based variational inequality formulation of ideal dynamic user-optimal route choice problem, Transport. Res. C-Emer., 1996, 4, 1–12 http://dx.doi.org/10.1016/0968-090X(95)00017-D 10.1016/0968-090X(95)00017-D Search in Google Scholar
[82] Carey M., Optimal time-varying flows on congested network, Oper. Res., 1987, 35, 58–69 http://dx.doi.org/10.1287/opre.35.1.58 10.1287/opre.35.1.58 Search in Google Scholar
[83] Carey M., Srinivasan A., Congested network flows: time-varying demands and start-time policies, Eur. J. Oper. Res., 1988, 36, 227–240 http://dx.doi.org/10.1016/0377-2217(88)90429-8 10.1016/0377-2217(88)90429-8 Search in Google Scholar
[84] Lo H.K., Szeto W.Y., A cell-based variational inequality formulation of the dynamic user optimal assignment problem, Transport. Res. B-Meth., 2002, 36, 421–443 http://dx.doi.org/10.1016/S0191-2615(01)00011-X 10.1016/S0191-2615(01)00011-X Search in Google Scholar
[85] Szeto W.Y., The enhanced lagged cell transmission model for dynamic traffic assignment, Transport. Res. Rec., 2008, 2085, 76–85 http://dx.doi.org/10.3141/2085-09 10.3141/2085-09 Search in Google Scholar
[86] Lo H.K., Ran B., Hongola B., Multiclass dynamic traffic assignment model: formulation and computational experiences, Transport. Res. Rec., 1996, 1537, 74–82 http://dx.doi.org/10.3141/1537-11 10.1177/0361198196153700111 Search in Google Scholar
[87] Liu Y.H., Mahmassani H.S., Dynamic aspects of commuter decisions under advanced traveler information systems — modeling framework and experimental results, Transport. Res. Rec., 1998, 1645, 111–119 http://dx.doi.org/10.3141/1645-14 10.3141/1645-14 Search in Google Scholar
[88] Ben-Akiva M., Cuneo D., Hasan M., Jha M., et al., Evaluation of freeway control using a microscopic simulation laboratory, Transport. Res. C-Emer., 2003, 11, 29–50 http://dx.doi.org/10.1016/S0968-090X(02)00020-7 10.1016/S0968-090X(02)00020-7 Search in Google Scholar
[89] Jiang Y.Q., Wong S.C., Ho H.W., Zhang P., et al., A dynamic traffic assignment model for a continuum transportation system, Transport. Res. B-Meth., 2011, 45, 343–363 http://dx.doi.org/10.1016/j.trb.2010.07.003 10.1016/j.trb.2010.07.003 Search in Google Scholar
[90] Hughes R.L., A continuum theory for the flow of pedestrians, Transport. Res. B-Meth., 2002, 36, 507–535 http://dx.doi.org/10.1016/S0191-2615(01)00015-7 10.1016/S0191-2615(01)00015-7 Search in Google Scholar
[91] Hoogendoorn S.P., Bovy P.H.L., Dynamic useroptimal assignment in continuous time and space, Transport. Res. B-Meth., 2004, 38, 571–592 http://dx.doi.org/10.1016/j.trb.2002.12.001 10.1016/j.trb.2002.12.001 Search in Google Scholar
[92] Hoogendoorn S.P., Bovy P.H.L., Pedestrian routechoice and activity scheduling theory and models, Transport. Res. B-Meth., 2004, 38, 169–190 http://dx.doi.org/10.1016/S0191-2615(03)00007-9 10.1016/S0191-2615(03)00007-9 Search in Google Scholar
[93] Xia Y., Wong S.C., Zhang M., Shu C.W., et al., An efficient discontinuous Galerkin method on triangular meshes for a pedestrian flow model, Int. J. Numer. Meth. Eng., 2008, 76, 337–350 http://dx.doi.org/10.1002/nme.2329 10.1002/nme.2329 Search in Google Scholar
[94] Huang L., Wong S.C., Zhang M.P., Shu C.W., et al., Revisiting Hughes’ dynamic continuum model for pedestrian flow and the development of an efficient solution algorithm. Transport. Res. B-Meth., 2009, 43, 127–141 http://dx.doi.org/10.1016/j.trb.2008.06.003 10.1016/j.trb.2008.06.003 Search in Google Scholar
[95] Huang L., Xia Y., Wong S.C., Shu C.W., et al., Dynamic continuum model for bi-directional pedestrian flows, P. I. Civil Eng.: Eng. Comput. Mec., 2009, 162, 67–75 10.1680/eacm.2009.162.2.67 Search in Google Scholar
[96] Xia Y., Wong S.C., Shu C.W., Dynamic continuum pedestrian flow model with memory effect, Phys. Rev. E, 2009, 79, 066113 http://dx.doi.org/10.1103/PhysRevE.79.066113 10.1103/PhysRevE.79.066113 Search in Google Scholar
[97] Jiang Y.Q., Xiong T., Wong S.C., Shu C.W., et al., A reactive dynamic continuum user equilibrium model for bi-directional pedestrian flows, Acta. Math. Sci., 2009, 29, 1541–1555 10.1016/S0252-9602(10)60002-1 Search in Google Scholar
[98] Guo R.Y., Huang H.J., Wong S.C., Collection, spillback, and dissipation in pedestrian evacuation: a network-based method, Transport. Res. B-Meth., 2011, 45, 490–506 http://dx.doi.org/10.1016/j.trb.2010.09.009 10.1016/j.trb.2010.09.009 Search in Google Scholar
[99] Xiong T., Zhang M., Shu C.W., Wong S.C., et al., Highorder computational scheme for a dynamic continuum model for bi-directional pedestrian flows, Comput.—Aided Civ. Inf., 2011, 26, 298–310 http://dx.doi.org/10.1111/j.1467-8667.2010.00688.x 10.1111/j.1467-8667.2010.00688.x Search in Google Scholar
[100] Nagurney A., Network economics: a variational inequality approach, Kluwer Academic Publishers, Norwell, Massachusetts, 1993 http://dx.doi.org/10.1007/978-94-011-2178-1 10.1007/978-94-011-2178-1 Search in Google Scholar
[101] Chow A.H.F., Properties of system optimal traffic assignment with departure time choice and its solution method, Transport. Res. B-Meth., 2009, 43, 325–344 http://dx.doi.org/10.1016/j.trb.2008.07.006 10.1016/j.trb.2008.07.006 Search in Google Scholar
[102] Chow A.H.F., Dynamic system optimal traffic assignment — a state-dependent control theoretic approach, Transportmetrica, 2009, 5, 85–106 http://dx.doi.org/10.1080/18128600902717483 10.1080/18128600902717483 Search in Google Scholar
[103] Long J.C., Szeto W.Y., Gao Z.Y., Discretised link travel time models based on cumulative flows: formulations and properties, Transport. Res. B-Meth., 2011, 45, 232–254 http://dx.doi.org/10.1016/j.trb.2010.05.002 10.1016/j.trb.2010.05.002 Search in Google Scholar
© 2011 Versita Warsaw
This work is licensed under the Creative Commons Attribution-NonCommercial-NoDerivatives 3.0 License.
- X / Twitter
Supplementary Materials
Please login or register with De Gruyter to order this product.
Journal and Issue
Articles in the same issue.
Traffic Assignment: A Survey of Mathematical Models and Techniques
- First Online: 17 May 2018
Cite this chapter
- Pushkin Kachroo 14 &
- Kaan M. A. Özbay 15
Part of the book series: Advances in Industrial Control ((AIC))
1043 Accesses
2 Citations
This chapter presents the fundamentals of the theory and techniques of traffic assignment problem. It first presents the steady-state traffic assignment problem formulation which is also called static assignment, followed by Dynamic Traffic Assignment (DTA), where the traffic demand on the network is time varying. The static assignment problem is shown in a mathematical programming setting for two different objectives to be satisfied. The first one where all users experience same travel times in alternate used routes is called user-equilibrium and another setting called system optimum in which the assignment attempts to minimize the total travel time. The alternate formulation uses variational inequality method which is also presented. Dynamic travel routing problem is also reviewed in the variational inequality setting. DTA problem is shown in discrete and continuous time in terms of lumped parameters as well as in a macroscopic setting, where partial differential equations are used for the link traffic dynamics. A Hamilton–Jacobi- based travel time dynamics model is also presented for the links and routes, which is integrated with the macroscopic traffic dynamics. Simulation-based DTA method is also very briefly reviewed. This chapter is taken from the following Springer publication and is reproduced here, with permission and with minor changes: Pushkin Kachroo, and Neveen Shlayan, “Dynamic traffic assignment: A survey of mathematical models and technique,” Advances in Dynamic Network Modeling in Complex Transportation Systems (Editor: Satish V. Ukkusuri and Kaan Özbay) Springer New York, 2013. 1-25.
This chapter is taken from the following Springer publication and is reproduced here, with permission and with minor changes: Pushkin Kachroo, and Neveen Shlayan, “Dynamic traffic assignment: A survey of mathematical models and techniques,” Advances in Dynamic Network Modeling in Complex Transportation Systems (Editor: Satish V. Ukkusuri and Kaan Özbay) Springer New York, 2013. 1–25.
This is a preview of subscription content, log in via an institution to check access.
Access this chapter
Institutional subscriptions
Similar content being viewed by others
Dynamic Traffic Assignment: A Survey of Mathematical Models and Techniques
Traffic Assignments to Transportation Networks
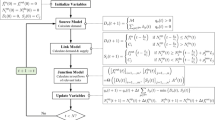
Computing Dynamic User Equilibria on Large-Scale Networks with Software Implementation
Gazis DC (1974) Traffic science. Wiley-Interscience Inc, New York, NY
MATH Google Scholar
Potts RB, Oliver RM (1972) Flows in transportation networks. Elsevier Science
Google Scholar
Stouffer SA (1940) Intervening opportunities: a theory relating mobility and distance. Am Sociol Rev 5:845–867
Article Google Scholar
Hitchcock FL (1941) The distribution of a product from several sources to numerous localities. J Math Phys 20:224–230
Article MathSciNet Google Scholar
Voorhees AM (2013) A general theory of traffic movement 40:1105–1116. https://doi.org/10.1007/s11116-013-9487-0
Wilson AG (1967) A statistical theory of spatial distribution models. Transp Res 1:253–269
Ben-Akiva ME, Lerman SR (1985) Discrete choice analysis: theory and application to travel demand. MIT Press series in transportation studies, MIT Press
Wardrop JG (1952) Some theoretical aspects of road traffic research. Proc Inst Civil Eng PART II 1:325–378
Sheffi Y (1985) Urban transportation networks: equilibrium analysis with mathematical programming methods. Prentice-Hall
Peeta S, Ziliaskopoulos AK (2001) Foundations of dynamic traffic assignment: the past, the present and the future. Netw Spat Econ 1(3/4):233–265
Kachroo P, Sastry S (2016a) Travel time dynamics for intelligent transportation systems: theory and applications. IEEE Trans Intell Transp Syst 17(2):385–394
Kachroo P, Sastry S (2016b) Traffic assignment using a density-based travel-time function for intelligent transportation systems. IEEE Trans Intell Transp Syst 17(5):1438–1447
Dafermos SC, Sparrow FT (1969) The traffic assignment problem for a general network. J Res Natl Bur Stan 73B:91–118
Beckmann MJ, McGuire CB, Winsten CB (1955) Studies in the economics of transportation. Technical report, Rand Corporation
Dafermos S (1980) Traffic equilibrium and variational inequalities. Transp Sci 14:42–54
Dafermos S (1983) An iterative scheme for variational inequalities. Math Prog 26:40–47
Kinderlehrer D, Stampacchia G (2000) An introduction to variational inequalities and their applications, vol 31. Society for Industrial Mathematics
Avriel M (2003) Nonlinear programming: analysis and methods. Dover Publications
Bazaraa MS, Sherali HD, Shetty CM (2006) Nonlinear programming: theory and algorithms. John Wiley & Sons
Mangasarian OL (1994) Nonlinear programming, vol 10. Society for Industrial Mathematics. https://doi.org/10.1137/1.9781611971255
Nugurney A (2000) Sustainable transportation networks. Edward Elgar Publishing, Northampton, MA
Facchinei F, Pang JS (2007) Finite-dimensional variational inequalities and complementarity problems. Springer Science & Business Media
Nagurney A, Zhang D (2012) Projected dynamical systems and variational inequalities with applications, vol 2. Springer Science & Business Media
Zhang D, Nagurney A (1995) On the stability of projected dynamical systems. J Optim Theory Appl 85(1):97–124
Nagurney A, Zhang D (1997) Projected dynamical systems in the formulation, stability analysis, and computation of fixed-demand traffic network equilibria. Transp Sci 31(2):147–158
Zhang D, Nagurney A (1996) On the local and global stability of a travel route choice adjustment process. Transp Res Part B: Methodol 30(4):245–262
Dafermos S (1988) Sensitivity analysis in variational inequalities. Math Oper Res 13:421–434
Dupuis P, Nagurney A (1993) Dynamical systems and variational inequalities. Ann Oper Res 44(1):7–42
Skorokhod AV (1961) Stochastic equations for diffusion processes in a bounded region. Theory Probab Appl 6:264–274
Chiu Y-C, Bottom J, Mahut M, Paz A, Balakrishna R, Waller T, Hicks J (2010) A primer for dynamic traffic assignment. Trans Res Board, 2–3
Ran B, Boyce DE (1996) Modeling dynamic transportation networks: an intelligent transportation system oriented approach. Springer
Chapter Google Scholar
Friesz TL (2001) Special issue on dynamic traffic assignment. Netw Spat Econ Part I 1:231
Merchant DK, Nemhauser GL (1978a) A model and an algorithm for the dynamic traffic assignment problems. Transp Sci 12(3):183–199
Merchant DK, Nemhauser GL (1978b) Optimality conditions for a dynamic traffic assignment model. Transp Sci 12(3):183–199
Boyce D, Lee D, Ran B (2001) Analytical models of the dynamic traffic assignment problem. Netw Spat Econ 1:377–390
Friesz TL, Luque J, Tobin RL, Wie BW (1989) Dynamic network traffic assignment considered as a continuous time optimal control problem. Oper Res 37:893–901
Friesz TL, Bernstein D, Smith TE, Tobin RL, Wie BW (1993) A variational inequality formulation of the dynamic network user equilibrium problem. Oper Res 41:179–191
Chen HK (2012) Dynamic travel choice models: a variational inequality approach. Springer Science & Business Media
Carey M (1992) Nonconvexity of the dynamic traffic assignment problem. Transp Res Part B: Methodol 26(2):127–133
Lighthill MJ, Whitham GB (1955) On kinematic waves II. A theory of traffic on long crowded roods. Proc Roy Soc London A Math Phys Sci 229:317–345. https://doi.org/10.1098/rspa.1955.0089
Richards PI (1956) Shockwaves on the highway. Oper Res 4:42–51
Greenshields B, Channing W, Miller H (1935) A study of traffic capacity. In: Highway Research Board Proceedings. National Research Council (USA), Highway Research Board
LeVeque RJ (1990) Numerical methods for conservation laws. Birkhäuser Verlag
Bressan A (2000) Hyperbolic systems of conservation laws: the one-dimensional Cauchy problem. Oxford University Press
Strub I, Bayen A (2006) Weak formulation of boundary conditions for scalar conservation laws: an application to highway modeling. Int J Robust Nonlinear Control 16:733–748
Garavello M, Piccoli B (2006) Traffic flow on networks. American Institute of Mathematical Sciences, Ser Appl Maths 1:1–243
Holden H, Risebro NH (1995) A mathematical model of traffic flow on a network of unidirectional roads. SIAM J Math Anal 26:999–1017
Lebacque JP (1996) The godunov scheme and what it means for first order traffic flow models. In: Transportation and Traffic Theory, Proceedings of The 13th International Symposium on Transportation and Traffic Theory, Lyon, France, pp 647–677
Coclite GM, Piccoli B (2002) Traffic flow on a road network. Arxiv preprint math/0202146
Garavello M, Piccoli B (2005) Source-destination flow on a road network. Commun Math Sci 3(3):261–283
Gugat M, Herty M, Klar A, Leugering G (2005) Optimal control for traffic flow networks. J Optim theory Appl 126(3):589–616
Lebacque JP, Khoshyaran MM (2002) First order macroscopic traffic flow models for networks in the context of dynamic assignment. In: Patriksson M, Labbé M (eds) Transportation, Planning: State of the Art. Springer US, Boston, MA, pp 119–140. https://doi.org/10.1007/0-306-48220-7_8
Buisson C, Lebacque JP, Lesort JB (1996) Strada, a discretized macroscopic model of vehicular traffic flow in complex networks based on the godunov scheme. In: CESA’96 IMACS Multiconference: computational engineering in systems applications, pp 976–981
Mahmassani HS, Hawas YE, Abdelghany K, Abdelfatah A, Chiu YC, Kang Y, Chang GL, Peeta S, Taylor R, Ziliaskopoulos A (1998) DYNASMART-X; Volume II: analytical and algorithmic aspects. Technical report ST067 85
Ben-Akiva M, Bierlaire M, Koutsopoulos H, Mishalani R (1998) DynaMIT: a simulation-based system for traffic prediction. In: DACCORS short term forecasting workshop, vol TRANSP-OR-CONF-2006-060
Kachroo P, Özbay K (2012) Feedback control theory for dynamic traffic assignment. Springer Science & Business Media
Kachroo P, Özbay K (2011) Feedback ramp metering in intelligent transportation systems. Springer Science & Business Media
Kachroo P, Özbay K (1998) Solution to the user equilibrium dynamic traffic routing problem using feedback linearization. Transp Res Part B: Methodol 32(5):343–360
Kachroo P, Özbay K (2006) Modeling of network level system-optimal real-time dynamic traffic routing problem using nonlinear \(\text{ h }{\infty }\) feedback control theoretic approach. J Intell Transp Syst 10(4):159–171
Kachroo P, Özbay K (2005) Feedback control solutions to network level user-equilibrium real-time dynamic traffic assignment problems. Netw Spat Econ 5(3):243–260
Spiess H (1990) Conical volume-delay functions. Transp Sci 24(2):153–158
Download references
Author information
Authors and affiliations.
Department of Electrical and Computer Engineering, University of Nevada, Las Vegas, NV, USA
Pushkin Kachroo
Department of Civil and Urban Engineering, New York University, Brooklyn, NY, USA
Kaan M. A. Özbay
You can also search for this author in PubMed Google Scholar
Corresponding author
Correspondence to Pushkin Kachroo .
Rights and permissions
Reprints and permissions
Copyright information
© 2018 Springer International Publishing AG, part of Springer Nature
About this chapter
Kachroo, P., Özbay, K.M.A. (2018). Traffic Assignment: A Survey of Mathematical Models and Techniques. In: Feedback Control Theory for Dynamic Traffic Assignment. Advances in Industrial Control. Springer, Cham. https://doi.org/10.1007/978-3-319-69231-9_2
Download citation
DOI : https://doi.org/10.1007/978-3-319-69231-9_2
Published : 17 May 2018
Publisher Name : Springer, Cham
Print ISBN : 978-3-319-69229-6
Online ISBN : 978-3-319-69231-9
eBook Packages : Engineering Engineering (R0)
Share this chapter
Anyone you share the following link with will be able to read this content:
Sorry, a shareable link is not currently available for this article.
Provided by the Springer Nature SharedIt content-sharing initiative
- Publish with us
Policies and ethics
- Find a journal
- Track your research
- DOI: 10.17226/22872
- Corpus ID: 15677103
Dynamic Traffic Assignment: A Primer
- Y. Chiu , J. Bottom , +4 authors Jim Hicks
- Published 1 June 2011
- Engineering
- Transportation research circular
216 Citations
Application of dynamic traffic assignment to advanced managed lane modeling., improved calibration method for dynamic traffic assignment models, investigating regional dynamic traffic assignment modeling for improved bottleneck analysis: final report, a general framework for modeling shared autonomous vehicles with dynamic network-loading and dynamic ride-sharing application, extending travel-time based models for dynamic network loading and assignment, to achieve adherence to first-in-first-out and link capacities, advances in dynamic traffic assignment models, deploying a dynamic traffic assignment model for the sydney region, study on traveler oriented dynamic traffic assignment problems.
- Highly Influenced
A Discrete-Flow Form of the Point-Queue Model
Strategic dynamic traffic assignment incorporating travel demand uncertainty, 32 references, online deployment of dynamic traffic assignment: architecture and run-time management, linear programming formulations for system optimum dynamic traffic assignment with arrival time-based and departure time-based demands, dynamic traffic assignment modeling for incident management, location configuration design for dynamic message signs under stochastic incident and atis scenarios, foundations of dynamic traffic assignment: the past, the present and the future, how reliable is this route, calibration and application of a simulation-based dynamic traffic assignment model, dynamic traffic assignment in design and evaluation of high-occupancy toll lanes, a simultaneous route and departure time choice equilibrium model on dynamic networks, off-line calibration of dynamic traffic assignment models, related papers.
Showing 1 through 3 of 0 Related Papers

Dynamic Traffic Assignment
Early Experiences
Current Practices
Research Needs
Page categories
Activity Based Models
Network Assignment
Topic Circles
More pages in this category:
(opens new window) is a hot topic in travel forecasting.
# Background
Traditional user equilibrium highway assignment models predict the effects of congestion and the routing changes of traffic as a result of that congestion. They neglect, however, many of the details of real-world traffic operations, such as queuing, shock waves, and signalization. Currently, it is common practice to feed the results of user equilibrium traffic assignments into dynamic network models as a mechanism for evaluating these policies. The simulation models themselves, however, do not predict the routing of traffic, and therefore are unable to account for re-routing owing to changes in congestion levels or policy, and can be inconsistent with the routes determined by the assignment. Dynamic network models overcome this dichotomy by combining a time-dependent shortest path algorithm with some type of simulation (often meso or macroscopic) of link travel times and delay. In doing so it allows added reality and consistency in the assignment step, as well as the ability to evaluate policies designed to improve traffic operations. These are some of the main benefits of dynamic network models .
DTA models can generally be classified by how they model link or intersection delay. Analytical DTA models treat it in the same manner as static equilibrium assignment models, with no explicit representation of signals. Link capacity functions, often similar or identical to those used in static assignment, are used to calculate link travel times. Analytical models have been widely used in research and for real-time control system applications. Simulation-based DTA models include explicit representation of traffic control devices. Such models require detailed signal parameters to include phasing, cycle length, and offsets for each signal in the network. Delay is calculated for each approach, with vehicles moving from one link to the next only if available downstream capacity is available. The underlying traffic model is often different, but at the network level such models behave in a similar fashion.
Demand is specified in the form of origin–destination matrices for short time intervals, typically 15 minutes each. Trips are typically randomly loaded onto the network during each time interval. As with traffic microsimulation models, adequate downstream capacity must be present to load the trips onto the network. The shortest paths through time and space are found for each origin–destination pair, and flows loaded to these paths. A generalized flowchart of the process is shown below.

As with static assignment models, the process shown above is iteratively solved until a stable solution is reached. The memory and computing requirements of DTA, however, are orders of magnitude larger than for static assignment, reducing the number of iterations and paths that can be kept in memory. Instead of a single time period, as with static assignment, DTA models must store data for each time interval as well. A three-hour static assignment would involve only one time interval. A DTA model of the same period, however, might require 12 intervals, each 15 minutes in duration. These are all in addition to the memory requirements imposed by the number of user classes and zones.

# Early Experiences
Research into DTA dates back several decades, but was largely limited to academics working on its formulation and theoretical aspects. DTA overcomes the limitations of static assignment models, although at the cost of increased data requirements and computational burden. Moreover, software platforms capable of solving the DTA problem for large urban systems and experience in their use are recent developments.
(opens new window) has been successfully applied to a large subarea of Calgary and to analyses of the Rue Notre-Dame in Montreal. Although user group presentations of both applications have been made, and reported very encouraging results, the work is currently unpublished and inaccessible except through contact with the developers.
(opens new window) . The network from the Atlanta Regional Commission (ARC) regional travel model formed the starting point for the DTA network. Intersections were coded, centroid connectors were re-defined, and network coding errors were corrected. A signal synthesizer derived locally optimal timing parameters for more than 2,200 signalized intersections in the network. Trip matrices from the ARC model were divided into 15-minute intervals for the specification of demand. Approximately 40 runs of the model were required to diagnose coding and software errors. Unfortunately, the execution time for the model was approximately one week per run. The resulting model eventually validated well to observed conditions; however, the length of time required to render it operational and the run time required prevented it from being used in studies as originally intended. Subsequent work by the developer has resulted in substantial reductions in run time, but this remains a significant issue that must be overcome before such models can be more widely used.
# Current Practices
# research needs.
A number of cities are currently testing DTA models, but are not far enough along in their work to share even preliminary results. At least a dozen such cases are known to be in varying stages of planning or execution, suggesting that the use of DTA models in planning applications is about to expand dramatically. However, in addition to the issue of long run times, a number of other issues must be addressed before such models are likely to be widely adopted:
- Criteria for the validation of such models have not been widely accepted. The paucity of traffic counts in most urban areas, and especially at 15, 30, or 60 minute intervals, is a significant barrier to definitive assessment of these models.
← Network assignment Land Use-Transport Modeling →
This site uses cookies to learn which topics interest our readers.
Grab your spot at the free arXiv Accessibility Forum
Help | Advanced Search
Computer Science > Machine Learning
Title: heterogeneous graph sequence neural networks for dynamic traffic assignment.
Abstract: Traffic assignment and traffic flow prediction provide critical insights for urban planning, traffic management, and the development of intelligent transportation systems. An efficient model for calculating traffic flows over the entire transportation network could provide a more detailed and realistic understanding of traffic dynamics. However, existing traffic prediction approaches, such as those utilizing graph neural networks, are typically limited to locations where sensors are deployed and cannot predict traffic flows beyond sensor locations. To alleviate this limitation, inspired by fundamental relationship that exists between link flows and the origin-destination (OD) travel demands, we proposed the Heterogeneous Spatio-Temporal Graph Sequence Network (HSTGSN). HSTGSN exploits dependency between origin and destination nodes, even when it is long-range, and learns implicit vehicle route choices under different origin-destination demands. This model is based on a heterogeneous graph which consists of road links, OD links (virtual links connecting origins and destinations) and a spatio-temporal graph encoder-decoder that captures the spatio-temporal relationship between OD demands and flow distribution. We will show how the graph encoder-decoder is able to recover the incomplete information in the OD demand, by using node embedding from the graph decoder to predict the temporal changes in flow distribution. Using extensive experimental studies on real-world networks with complete/incomplete OD demands, we demonstrate that our method can not only capture the implicit spatio-temporal relationship between link traffic flows and OD demands but also achieve accurate prediction performance and generalization capability.
Comments: | 9 pages, 5 figures |
Subjects: | Machine Learning (cs.LG) |
Cite as: | [cs.LG] |
(or [cs.LG] for this version) | |
Focus to learn more arXiv-issued DOI via DataCite (pending registration) |
Submission history
Access paper:.
- HTML (experimental)
- Other Formats

References & Citations
- Google Scholar
- Semantic Scholar
BibTeX formatted citation

Bibliographic and Citation Tools
Code, data and media associated with this article, recommenders and search tools.
- Institution
arXivLabs: experimental projects with community collaborators
arXivLabs is a framework that allows collaborators to develop and share new arXiv features directly on our website.
Both individuals and organizations that work with arXivLabs have embraced and accepted our values of openness, community, excellence, and user data privacy. arXiv is committed to these values and only works with partners that adhere to them.
Have an idea for a project that will add value for arXiv's community? Learn more about arXivLabs .
Navigation Menu
Search code, repositories, users, issues, pull requests..., provide feedback.
We read every piece of feedback, and take your input very seriously.
Saved searches
Use saved searches to filter your results more quickly.
To see all available qualifiers, see our documentation .
dynamic-traffic-assignment
Here are 9 public repositories matching this topic..., toruseo / uxsim.
Vehicular traffic flow simulator in road network, written in pure Python
- Updated Aug 7, 2024
jdlph / Path4GMNS
An open-source, cross-platform, lightweight, and fast Python path engine for networks encoded in GMNS.
- Updated Apr 20, 2024
jdlph / TAP101
A collection of open-source projects on the Traffic Assignment Problem
- Updated Sep 11, 2023
jdlph / TransOMS
State-of-the-art open-source applications for Transportation Optimization, Modeling, and Simulation
- Updated Jul 17, 2024
LeonSering / multi-commodity-flows-over-time
Given network and commodity information this tool computes a feasible multi-commodity flow over time.
- Updated Feb 5, 2024
jdlph / DTALite
A clean and common C++ code base to build both executable and shared library of DTALite across platforms.
- Updated Jul 27, 2024
LeonSering / NashFlowComputation
A GUI-tool for computing Nash flows over time with or without spillback as well as (spillback) thin flows.
- Updated Feb 25, 2024
sarthak268 / amubulance-routing-with-dynamic-traffic
Ambulance routing problem with dynamic traffic constraints
- Updated Dec 12, 2019
ArbeitsgruppeTobiasHarks / dynamic-flow-visualization
A JS-based visualization tool for dynamic (Vickrey) flows
- Updated Aug 22, 2023
Improve this page
Add a description, image, and links to the dynamic-traffic-assignment topic page so that developers can more easily learn about it.
Curate this topic
Add this topic to your repo
To associate your repository with the dynamic-traffic-assignment topic, visit your repo's landing page and select "manage topics."
Co-evolutionary traffic signal control using reinforcement learning for road networks under stochastic capacity
New citation alert added.
This alert has been successfully added and will be sent to:
You will be notified whenever a record that you have chosen has been cited.
To manage your alert preferences, click on the button below.
New Citation Alert!
Please log in to your account
Information & Contributors
Bibliometrics & citations, view options, recommendations, road traffic optimisation using an evolutionary game.
In a commuting scenario, drivers expect to arrive at their destinations on time. Drivers have an expectation as to how long it will take to reach the destination. To this end, drivers make independent decisions regarding the routes they take. ...
Road following using neural networks and reinforcement learning
A method of reinforcement learning based automatic traffic signal control.
To improve performance of traffic signal control system in urban area, a novel method is proposed in this paper. The roads, vehicles and the traffic control systems are all modeled as intelligent agents. Wireless communication network provides the ...
Information
Published in.
Elsevier Science Publishers B. V.
Netherlands
Publication History
Author tags.
- Intelligent traffic signal control
- Coordinated co-evolutionary decomposition
- Bi-level optimization
- Traffic assignment
- Stochastic capacity
- Research-article
Contributors
Other metrics, bibliometrics, article metrics.
- 0 Total Citations
- 0 Total Downloads
- Downloads (Last 12 months) 0
- Downloads (Last 6 weeks) 0
View options
Login options.
Check if you have access through your login credentials or your institution to get full access on this article.
Full Access
Share this publication link.
Copying failed.
Share on social media
Affiliations, export citations.
- Please download or close your previous search result export first before starting a new bulk export. Preview is not available. By clicking download, a status dialog will open to start the export process. The process may take a few minutes but once it finishes a file will be downloadable from your browser. You may continue to browse the DL while the export process is in progress. Download
- Download citation
- Copy citation
We are preparing your search results for download ...
We will inform you here when the file is ready.
Your file of search results citations is now ready.
Your search export query has expired. Please try again.

IMAGES
COMMENTS
A semi-dynamic traffic assignment model can be considered a series of connected STA models (e.g., Nakayama and Conors, 2014). Unlike STA, a semi-dynamic traffic assignment model has multiple time periods for route choice and allows the residual traffic of one period to transfer to the following time periods.
Now, after decades of research and intensive market readiness developments, dynamic traffic assignment (DTA) models have become a viable modeling option. DTA models supplemental existing travel forecasting models and microscopic traffic simulation models. Travel forecasting models represent the static regional travel analysis capability ...
1 Dynamic Traffic Assignment: Model Classifications and Recent Advances in Travel Choice Principles W.Y. SZETO a, S.C. WONG a,b a Department of Civil Engineering, The University of Hong Kong, Hong Kong, China b E-mail: [email protected] Abstract: Dynamic Traffic Assignment (DTA) has been studied for more than four decades and numerous reviews of this research area have been conducted.
Dynamic Traffic Assignment (DTA) has been studied for more than four decades and numerous reviews of this research area have been conducted. This review focuses on the travel choice principle and the classification of DTA models, and is supplementary to the existing reviews. The implications of the travel choice principle for the existence and uniqueness of DTA solutions are discussed, and the ...
Dynamic traffic flow, which can facilitate the efficient operation of traffic road networks, is an important prerequisite for the application of reasonable assignment of traffic demands in an urban road network. In order to improve the accuracy of dynamic traffic flow assignment, this paper proposes a dynamic traffic flow assignment model based on GPS trajectory data and the influence of POI.
This guidebook on the utilization of Dynamic Traffic Assignment (DTA) complements and enhances other existing guidebooks on modeling by traffic providing guidance on DTA. Since DTA modeling is a new and emerging technique, basic DTA modeling methods and techniques are discussed in this guidebook.
Dynamic Traffic Assignment (DTA) has been studied for more than four decades and numerous reviews of this research area have been conducted. This review focuses on the travel choice principle and the classification of DTA models, and is supplementary to the existing reviews. The implications of the travel choice principle for the existence and uniqueness of DTA solutions are discussed, and the ...
Dynamic traffic assignment: model classifications and recent advances in travel choice principles 2 tim e so t h a t t hey arri ve at B a t 9:00 am sh arp, bu t the y wast e a lot of tim e que u ...
Abstract. This paper presents a survey of the mathematical methods used for modeling and solutions for the traffic assignment problem. It covers the static (steady-state) traffic assignment techniques as well as dynamic traffic assignment in lumped parameter and distributed parameter settings. Moreover, it also surveys simulation-based solutions.
This chapter is taken from the following Springer publication and is reproduced here, with permission and with minor changes: Pushkin Kachroo, and Neveen Shlayan, "Dynamic traffic assignment: A survey of mathematical models and techniques," Advances in Dynamic Network Modeling in Complex Transportation Systems (Editor: Satish V. Ukkusuri and Kaan Özbay) Springer New York, 2013. 1-25.
Srinivas Peeta1 and Athanasios K. Ziliaskopoulos2. Abstract: Dynamic Traffic Assignment (DTA) has evolved substantially since the pioneering work of Merchant and Nemhauser. Numerous formulations and solutions approaches have been introduced ranging from mathematical programming, to variational inequality, optimal control, and simulation-based.
This paper developed a cell-based dynamic traffic assignment (DTA) formulation that follows the ideal dynamic user optimal (DUO) principle. Through defining an appropriate gap function, we transformed a formulation based on the nonlinear complementarity problem to an equivalent mathematical program. To improve the accuracy of dynamic traffic ...
This circular is designed to help explain the basic concepts and definitions of dynamic traffic assignment (DTA) models and addresses the application, selection, planning, and execution of a DTA model. The report also describes the general DTA modeling procedure and modeling issues that may concern a model user.
1. Introduction. Simulation-based dynamic traffic assignment (DTA) is an effective tool for analyzing transportation systems for both operational and planning purposes [1], [2], [3].A DTA model replicates network traffic dynamics and captures the interactions between travelers and the transportation network.
Dynamic network assignment models (also referred to as dynamic traffic assignment models or DTA) capture the changes in network performance by detailed time-of-day, and can be used to generate time varying measures of this performance. They occupy the middle ground between static macroscopic traffic assignment and microscopic traffic simulation ...
This paper handles the findings of three recent dynamic traffic assignment models, namely; 1) A dynamic traffic assignment model for highly congested urban networks; 2) System-optimal dynamic traffic assignment with and without queue spillback: Its path-based formulation and solution via approximate path marginal cost; and 3) A dynamic traffic ...
The properties of dynamic traffic assignment (DTA) have important implications on its ability to portray the actual travel behaviour, and hence on the fidelity and accuracy of the model results. These properties depend strongly on the two components of DTA: the travel choice principle and the traffic-flow component. The travel choice principle
Traffic assignment and traffic flow prediction provide critical insights for urban planning, traffic management, and the development of intelligent transportation systems. An efficient model for calculating traffic flows over the entire transportation network could provide a more detailed and realistic understanding of traffic dynamics. However, existing traffic prediction approaches, such as ...
The three classes of users are integrated into one dynamic traffic assignment (DTA) model and solved using a newly proposed algorithm. In this paper, variables of link flow and exit flow are represented solely by in-flow. The resulting linear program subproblem in the inner iteration is proved and solved as a typical time-dependent shortest ...
comparison of static traffic assignment with the VISTA model, a simulation-based dynamic traffic assignment approach, and with an approximation to DTA using an add-in for TransCAD software. A novel demand profiling algorithm based on piecewise linear curves is developed, and a method to enable reasonable comparisons of static traffic assignment ...
An efficient model for calculating traffic flows over the entire transportation network could provide a more detailed and realistic understanding of traffic dynamics.
A dynamic traffic assignment model 57 problems in using network optimization algorithms as will be clear later. It is indeed possible to think of zero-cost backward links connecting the head node of a dJink to the tail node of the dJink the next time step, thus forming a conventional connected path). The static assignment problem can be solved ...
2 SEMI-DYNAMIC TRAFFIC ASSIGNMENT MODEL WITH MODE CHOICE. In our semi-dynamic approach, a day is divided into several periods. In addition, the duration of each period is not as short as the length of the time interval for ordinary discrete-time DTA models. The semi-dynamic approach presupposes that a majority of OD flows reach their ...
A discrete time model is presented for dynamic traffice assignment with a single destination. Congestion is treated explicitly in the flow equations. The model is a nonlinear and nonconvex mathematical programming problem. A piecewise linear version of the model, with additional assumptions on the objective function, can be solved for a global ...
Add this topic to your repo. To associate your repository with the dynamic-traffic-assignment topic, visit your repo's landing page and select "manage topics." GitHub is where people build software. More than 100 million people use GitHub to discover, fork, and contribute to over 420 million projects.
We study the implementation of traffic assignment engineering in conjunction with the network stochastic model: first, we study the Bayesian algorithm theoretical model of control layer stripping in the network based on the discrete dynamic Bayesian algorithm theory and analyze the resource-sharing mechanism in different queuing rules; second ...
Numerical experiments are performed at a real-data city road network and various sizable traffic grids. As compared to state-of-the-art traffic signal control for various traffic conditions, obtained results showed that CCTPC exhibits sufficient gain of achieving road network performance and suffers from the least computational cost in all cases.