Grab your spot at the free arXiv Accessibility Forum
Help | Advanced Search

Computer Science > Robotics
Title: a comprehensive review of recent research trends on uavs.
Abstract: The growing interest in unmanned aerial vehicles (UAVs) from both scientific and industrial sectors has attracted a wave of new researchers and substantial investments in this expansive field. However, due to the wide range of topics and subdomains within UAV research, newcomers may find themselves overwhelmed by the numerous options available. It is therefore crucial for those involved in UAV research to recognize its interdisciplinary nature and its connections with other disciplines. This paper presents a comprehensive overview of the UAV field, highlighting recent trends and advancements. Drawing on recent literature reviews and surveys, the review begins by classifying UAVs based on their flight characteristics. It then provides an overview of current research trends in UAVs, utilizing data from the Scopus database to quantify the number of scientific documents associated with each research direction and their interconnections. The paper also explores potential areas for further development in UAVs, including communication, artificial intelligence, remote sensing, miniaturization, swarming and cooperative control, and transformability. Additionally, it discusses the development of aircraft control, commonly used control techniques, and appropriate control algorithms in UAV research. Furthermore, the paper addresses the general hardware and software architecture of UAVs, their applications, and the key issues associated with them. It also provides an overview of current open-source software and hardware projects in the UAV field. By presenting a comprehensive view of the UAV field, this paper aims to enhance understanding of this rapidly evolving and highly interdisciplinary area of research.
Comments: | 32 pages, 4 figures, and 5 tables |
Subjects: | Robotics (cs.RO); Systems and Control (eess.SY) |
Cite as: | [cs.RO] |
(or [cs.RO] for this version) | |
Focus to learn more arXiv-issued DOI via DataCite |
Submission history
Access paper:.
- Other Formats

References & Citations
- Google Scholar
- Semantic Scholar
BibTeX formatted citation

Bibliographic and Citation Tools
Code, data and media associated with this article, recommenders and search tools.
- Institution
arXivLabs: experimental projects with community collaborators
arXivLabs is a framework that allows collaborators to develop and share new arXiv features directly on our website.
Both individuals and organizations that work with arXivLabs have embraced and accepted our values of openness, community, excellence, and user data privacy. arXiv is committed to these values and only works with partners that adhere to them.
Have an idea for a project that will add value for arXiv's community? Learn more about arXivLabs .
UAV-Based Delivery Systems: A Systematic Review, Current Trends, and Research Challenges
New citation alert added.
This alert has been successfully added and will be sent to:
You will be notified whenever a record that you have chosen has been cited.
To manage your alert preferences, click on the button below.
New Citation Alert!
Please log in to your account
Information & Contributors
Bibliometrics & citations.
- Franke M Sommer C (2024) Obstacle Shadowing in Vehicle-to-Satellite Communication: Impact of Location, Street Layout, Building Height, and LEO Satellite Constellation 2024 IEEE Vehicular Networking Conference (VNC) 10.1109/VNC61989.2024.10575991 (305-312) Online publication date: 29-May-2024 https://doi.org/10.1109/VNC61989.2024.10575991
- Yao A Pal S Li X Zhang Z Dong C Jiang F Liu X (2024) A privacy-preserving location data collection framework for intelligent systems in edge computing Ad Hoc Networks 10.1016/j.adhoc.2024.103532 161 (103532) Online publication date: Aug-2024 https://doi.org/10.1016/j.adhoc.2024.103532
Index Terms
Computing methodologies
Network algorithms
Recommendations
Same-day delivery with drone resupply.
Unmanned aerial vehicles, commonly referred to as drones , have recently seen an increased level of interest as their potential use in same-day home delivery has been promoted and advocated by large retailers and courier delivery companies. We introduce a ...
Drone Delivery: Why, Where, and When
Drone technology has the potential to revolutionize various sectors, including healthcare, food, and everyday goods, enabling efficient and convenient deliveries. However, in an expected competitive airspace environment with multiple companies, it ...
MiKe: Task Scheduling for UAV-based Parcel Delivery
Unmanned aerial vehicle (UAV) networks represent an ecological alternative to truck-based delivery systems, especially in urban areas prone to traffic congestion. In this paper, we formalize MiKe as the problem of assigning deliveries to a fleet of ...
Information
Published in.

Purdue University, United States
Association for Computing Machinery
New York, NY, United States
Publication History
Check for updates, author tags.
- Unmanned aerial vehicles (UAVs)
- drone delivery
- sustainable delivery
- transportation of blood and organs
- drone energy models
- delivery safety and security
Funding Sources
- GNCS – INdAM
- BREADCRUMBS
- PRIN 2022 PNRR
Contributors
Other metrics, bibliometrics, article metrics.
- 2 Total Citations View Citations
- 612 Total Downloads
- Downloads (Last 12 months) 612
- Downloads (Last 6 weeks) 134
View Options
Login options.
Check if you have access through your login credentials or your institution to get full access on this article.
Full Access
View options.
View or Download as a PDF file.
View online with eReader .
View this article in Full Text.
Share this Publication link
Copying failed.
Share on social media
Affiliations, export citations.
- Please download or close your previous search result export first before starting a new bulk export. Preview is not available. By clicking download, a status dialog will open to start the export process. The process may take a few minutes but once it finishes a file will be downloadable from your browser. You may continue to browse the DL while the export process is in progress. Download
- Download citation
- Copy citation
We are preparing your search results for download ...
We will inform you here when the file is ready.
Your file of search results citations is now ready.
Your search export query has expired. Please try again.
Information
- Author Services
Initiatives
You are accessing a machine-readable page. In order to be human-readable, please install an RSS reader.
All articles published by MDPI are made immediately available worldwide under an open access license. No special permission is required to reuse all or part of the article published by MDPI, including figures and tables. For articles published under an open access Creative Common CC BY license, any part of the article may be reused without permission provided that the original article is clearly cited. For more information, please refer to https://www.mdpi.com/openaccess .
Feature papers represent the most advanced research with significant potential for high impact in the field. A Feature Paper should be a substantial original Article that involves several techniques or approaches, provides an outlook for future research directions and describes possible research applications.
Feature papers are submitted upon individual invitation or recommendation by the scientific editors and must receive positive feedback from the reviewers.
Editor’s Choice articles are based on recommendations by the scientific editors of MDPI journals from around the world. Editors select a small number of articles recently published in the journal that they believe will be particularly interesting to readers, or important in the respective research area. The aim is to provide a snapshot of some of the most exciting work published in the various research areas of the journal.
Original Submission Date Received: .
- Active Journals
- Find a Journal
- Proceedings Series
- For Authors
- For Reviewers
- For Editors
- For Librarians
- For Publishers
- For Societies
- For Conference Organizers
- Open Access Policy
- Institutional Open Access Program
- Special Issues Guidelines
- Editorial Process
- Research and Publication Ethics
- Article Processing Charges
- Testimonials
- Preprints.org
- SciProfiles
- Encyclopedia
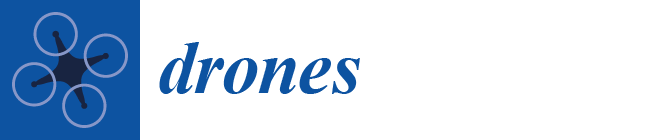
Article Menu
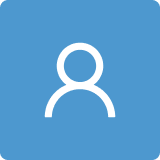
- Subscribe SciFeed
- Recommended Articles
- Google Scholar
- on Google Scholar
- Table of Contents
Find support for a specific problem in the support section of our website.
Please let us know what you think of our products and services.
Visit our dedicated information section to learn more about MDPI.
JSmol Viewer
Unmanned aerial vehicle obstacle avoidance based custom elliptic domain, 1. introduction, 1.1. related prior work, 1.2. organization, 2. review of velocity obstacle method, 2.1. velocity obstacle method theory, 2.1.1. minkowski sum, 2.1.2. velocity obstacle cone construction, 2.2. velocity obstacle method defects, 2.2.1. velocity obstacle excessive conservatism defect, 2.2.2. velocity adjustment defect, 2.2.3. velocity oscillation defects, 2.3. our contributions, 3. custom elliptic domain construction, 3.1. comparison of vo in circular and elliptic domains.
- The mathematical principle of the velocity obstacle space is the Minkowski sum of the boundary curves of two spatial objects. Geometrically, the Minkowski sum of two colliding entities represents the region swept by object A along the boundary of object B as it moves continuously for one revolution, combined with object B.
- When both objects are circles, their Minkowski sum is a circle with a radius equal to the sum of the radii of the two objects. For circles of the same size, their Minkowski sum is a circle with twice the radius.
- Based on the proof that the Minkowski sum of two circles remains a circle, it can be anticipated that the precise calculation of the Minkowski sum and velocity obstacle cone for two elliptical objects will be more difficult. The reason for this is that in Equation ( A1 ), the radius ‘ r ’ becomes the non-uniform semi-axis of the ellipse, making it challenging to simplify the computation of the maximum value. The distances from any point on ellipse A to the farthest point from the center of ellipse B obtained through iterative calculations will vary, indicating that the boundary of the Minkowski sum of two ellipses may not possess simple geometric characteristics. Therefore, further algorithmic solutions are required to address the velocity obstacle for elliptical boundaries.
3.1.1. Description of UAV Collision Stations
3.1.2. comparison of uav collision stations.
- Comparison of station α 0 and station α 1 : A and B both have circular protective domains. In the same collision scenario, the double protective domain of B in station α 1 will cause a significantly larger velocity obstacle space compared to station α 0 . The target avoidance velocity obtained in station α 1 will require a greater angular deviation. Therefore, a critical issue in applying the velocity obstacle principle to ensure collision-free operation for UAVs is determining the appropriate range of these protective domains. It is clear that avoidance decisions and outcomes are sensitive to the initial radius of this area. If the protective domain is too large, it compresses the available free space, leading to increased avoidance costs. Conversely, if the protective domain is too small, the performance requirements for the UAV during avoidance maneuvers increase, along with associated safety risks. Exploring a suitable and safe structure for the protective domain is a primary focus of this research.
- Comparison of station α 0 and station α 2 : While maintaining a constant protective distance in the direction of the speed, the protective distance in the normal direction of the speed is reduced. Consequently, the absolute speed obstacle angle is also decreased. This indicates that constructing a collision-free zone in the shape of an elliptical domain with a short axis can not only ensure safe obstacle avoidance but also minimize the utilization of airspace resources.
- Comparison of station α 0 and station α 3 : In station α 3 , the elliptical domain completely encompasses the circular domain from station α 0 , resulting in a velocity obstacle space that also covers the velocity obstacle space obtained from the circular domain. In station α 3 , the protective distance in the normal direction of the speed remains at r , while the protective distance in the velocity direction increases by 0.5 r . As a result, the velocity obstacle angle also increases accordingly. This demonstrates that both axes of the ellipse have an impact on the calculated results of the velocity obstacle space.
3.2. Elliptic Domain Flight Collision Risk Phase Division
3.3. collision risk phase uncertainty analysis, 3.3.1. collision risk phase uncertainty assumptions.
- Assumption 1: Segmented Multiple Tiny Deflections Assume that the drone’s directional adjustments are linearly varied over each time interval. Segmented, multiple, tiny deflections provide a smoother representation of the actual flight process. This method suggests that the angular velocity during the deflection process remains constant throughout each tiny time interval. The UAV produces consistent angles of deflection over identical, short periods, with the cumulative deflection angle increasing incrementally. Simply put, Assumption 1 is a gradual deflection process, which corresponds to the blue line in Figure 9 .
- Assumption 2: Deflection Along the Average Deflection Angle Deflection along the average deflection angle means that the UAV is oriented towards the target with the average angle θ ¯ of Assumption 1 . The endpoint of the blue line in Figure 9 represents the flight position obtained from Assumption 1, and the heading angle towards this endpoint from the initial position is denoted as θ ¯ . The UAV will fly along this direction instantly when the risk of collision is detected, which corresponds to the red dashed line in the middle of Figure 9 .
- Assumption 3: Deflected Along the Target Deflection Angle This assumption is the default assumption of the VO method. It specifies that upon detecting a collision threat, the UAV will immediately navigate in the direction of a velocity selected outside the AVO , which is named as the target deflection angle. In simple terms, the drone initially adjusts its heading to fly along the final flight angle defined by Assumption 1 , which corresponds to the red dashed line at the bottom of Figure 9 . The flight process for a given time interval τ under the three assumptions is demonstrated in Figure 9 . It is obvious that the flight endpoints are different across the three different assumptions.
3.3.2. Collision Risk Phase Error Expression Derivation
3.3.3. collision risk phase error analysis, 3.4. elliptic domain size construction considering uncertainty errors, 4. evo algorithm-based custom elliptic domains, 4.1. evo algorithm preparations, 4.2. evo algorithm steps.
- Step 1: Computation of Minkowski sum and convex hull boundary points
- Step 2: Finding the approximate EVO space tangent line
- Step 3: Return EVO space tangent points
- Step 4: Computation of EAVO
4.3. EVO Algorithm Obstacle Avoidance Velocity Control
4.4. evo algorithm oscillation elimination in velocity reback.
- Solution 1: Find an optimal position at which UAV-A initiates obstacle avoidance to ensure safety. Concurrently, UAVs A and B pass each other, ensuring that subsequent conditions satisfy o A τ i ∉ E A V O τ i . This approach prevents the occurrence of velocity oscillations. However, determining this optimal position introduces another layer of complexity, which this article will not explore in detail at this time.
- Solution 2: We impose a constraint on UAV-A to continue moving in the direction of the avoidance velocity until UAVs A and B have passed each other, after completing the avoidance maneuver. This approach simplifies the management of UAV trajectories, ensuring that the avoidance maneuver results in a successful and stable transition. Therefore, we have the constraint d v τ i < d v τ i + 1 of mutual passing. After completing the initial obstacle avoidance, UAV-A’s velocity direction is continuously adjusted towards the endpoint, ensuring a smooth flight without the need for secondary obstacle avoidance maneuvers. The adjustment processes still need to satisfy the limit of deflection: when o n e w τ i + 1 = o A τ i = o A τ i d v τ i > d v τ i + 1 o t a r g e t i = arc tan y g o a l − y A τ i x g o a l − x A τ i d v τ i < d v τ i + 1 o A τ i + φ τ , d v τ i < d v τ i + 1 & o t a r g e t i − o A τ i > φ τ (34)
5. Simulation
5.1. vo and evo obstacle avoidance evaluation indicators, 5.2. uav simulation parameters, 5.3. simulations and conclusions, 5.3.1. scenarios 1 simulation experiment, 5.3.2. scenarios 2 simulation experiment, 5.4. defects of custom elliptic domains for proximity uavs, 6. summary and outlook.
- Custom protection domains for arbitrary flight scenarios
- The lower limit of the elliptic protection domain
- Exploring elliptical domain applications in more complex experimental scenarios
Author Contributions
Data availability statement, conflicts of interest, abbreviations.
UAV | Unmanned aerial vehicle |
VO | Velocity obstacles method |
EVO | Elliptical velocity obstacles method |
RVO | Reciprocal velocity obstacles method |
VO | Relative velocity obstacle cone in the circle domain |
AVO | Absolute velocity obstacle cone in the circle domian |
EVO | Relative velocity obstacle cone in th elliptic domain |
EAVO | Absolute velocity obstacle cone in the elliptic domian |
Appendix A. Instruction and Analysis
Appendix a.1. convex polygons minkowski sum methods.
- Method 1: For the set of points formed by the boundary points of A and B P o i n t s A m , P o i n t s B n , m and n represent the number of elements in the point set, respectively, and the new point set generated by the corresponding addition contains at most mn elements. The convex hull of the new point set is the Minkowski sum of A and B. Its complexity is o m n log m n .
- Method 2: For the set of vectors to the boundary of A and B B o u n d a r y A m , B o u n d a r y B n , calculating the Minkowski sum of two convex sets is simply a matter of joining and merging the ‘ m + n ’ edge vectors after sorting them by their polar angles and then connecting and merging them in descending order of polar angle size. It can be guaranteed that the resulting graph is still convex, and the resulting convex hull is the Minkowski sum of A, B. Its complexity is o m + n log m n .
Appendix A.2. VO Space ‘Expanding’ to Twice under Two Identical Circle Domains
Appendix a.3. descriptions of the symbols used in the text.
Velocity obstacle space imposed by A on B in the circle domian | |
Velocity obstacle space imposed by A on B in the elliptic domian | |
Length of the major and minor semi-axes of the ellipse | |
Arbitrary length time slice | |
Time slice sequence | |
Time slice for the UAV to return to the endpoint | |
Maximum deflection angle of the UAV in one second | |
UAV flight endpoint error under Assumptions 1 and 2 | |
UAV flight endpoint error under Assumptions 2 and 3 | |
Velocities of UAVs A and B at moment | |
Elliptic velocity obstacle tangent direction | |
Projected distance between the two ellipses in the direction of the minor axis at |
- Fu, Q.; Liang, X.; Zhang, J.; Hou, Y. Cooperative conflict detection and resolution for multiple UAVs using two-layer optimization. Harbin Gongye Daxue Xuebao/J. Harbin Inst. Technol. 2020 , 52 , 74–83. [ Google Scholar ]
- Sarim, M.; Radmanesh, M.; Dechering, M.; Kumar, M.; Pragada, R.; Cohen, K. Distributed detect-and-avoid for multiple unmanned aerial vehicles in national air space. J. Dyn. Syst. Meas. Control 2019 , 141 , 071014. [ Google Scholar ] [ CrossRef ]
- Sunberg, Z.N.; Kochenderfer, M.J.; Pavone, M. Optimized and trusted collision avoidance for unmanned aerial vehicles using approximate dynamic programming. In Proceedings of the 2016 IEEE International Conference on Robotics and Automation (ICRA), Stockholm, Sweden, 16–21 May 2016; IEEE: New York, NY, USA, 2016; pp. 1455–1461. [ Google Scholar ]
- Phung, M.D.; Ha, Q.P. Safety-enhanced UAV path planning with spherical vector-based particle swarm optimization. Appl. Soft Comput. 2021 , 107 , 107376. [ Google Scholar ] [ CrossRef ]
- Pehlivanoglu, Y.V.; Pehlivanoglu, P. An enhanced genetic algorithm for path planning of autonomous UAV in target coverage problems. Appl. Soft Comput. 2021 , 112 , 107796. [ Google Scholar ] [ CrossRef ]
- Hao, G.; Lv, Q.; Huang, Z.; Zhao, H.; Chen, W. UAV Path Planning Based on Improved Artificial Potential Field Method. Aerospace 2023 , 10 , 562. [ Google Scholar ] [ CrossRef ]
- Liu, Y.; Zhao, Y. A virtual-waypoint based artificial potential field method for UAV path planning. In Proceedings of the 2016 IEEE Chinese Guidance, Navigation and Control Conference (CGNCC), Nanjing, China, 12–14 August 2016; IEEE: New York, NY, USA, 2016; pp. 949–953. [ Google Scholar ]
- Pan, Z.; Zhang, C.; Xia, Y.; Xiong, H.; Shao, X. An Improved Artificial Potential Field Method for Path Planning and Formation Control of the Multi-UAV Systems. IEEE Trans. Circuits Syst. II Express Briefs 2022 , 69 , 1129–1133. [ Google Scholar ] [ CrossRef ]
- Fiorini, P.; Shiller, Z. Motion planning in dynamic environments using velocity obstacles. Int. J. Robot. Res. 1998 , 17 , 760–772. [ Google Scholar ] [ CrossRef ]
- Best, A.; Narang, S.; Manocha, D. Real-time reciprocal collision avoidance with elliptical agents. In Proceedings of the 2016 IEEE International Conference on Robotics and Automation (ICRA), Stockholm, Sweden, 16–21 May 2016; IEEE: New York, NY, USA, 2016; pp. 298–305. [ Google Scholar ]
- Guo, H.; Guo, X. Local path planning algorithm for UAV based on improved velocity obstacle method. Hangkong Xuebao/Acta Aeronaut. et Astronaut. Sin. 2023 , 44 , 327586. [ Google Scholar ]
- Bi, K.; Wu, M.; Zhang, W.; Wen, X.; Du, K. Modeling and analysis of flight conflict network based on velocity obstacle method. Xi Tong Gong Cheng Yu Dian Zi Ji Shu/Syst. Eng. Electron. 2021 , 43 , 2163–2173. [ Google Scholar ]
- Zhang, H.; Gan, X.; Li, A.; Gao, Z.; Xu, X. UAV obstacle avoidance and track recovery strategy based on velocity obstacle method. Xi Tong Gong Cheng Yu Dian Zi Ji Shu/Syst. Eng. Electron. 2020 , 42 , 1759–1767. [ Google Scholar ]
- Yang, W.; Wen, X.; Wu, M.; Bi, K.; Yue, L. Three-Dimensional Conflict Resolution Strategy Based on Network Cooperative Game. Symmetry 2022 , 14 , 1517. [ Google Scholar ] [ CrossRef ]
- Peng, M.; Meng, W. Cooperative obstacle avoidance for multiple UAVs using spline_VO method. Sensors 2022 , 22 , 1947. [ Google Scholar ] [ CrossRef ]
- Adouane, L.; Benzerrouk, A.; Martinet, P. Mobile robot navigation in cluttered environment using reactive elliptic trajectories. IFAC Proc. Vol. 2011 , 44 , 13801–13806. [ Google Scholar ] [ CrossRef ]
- Braquet, M.; Bakolas, E. Vector field-based collision avoidance for moving obstacles with time-varying elliptical shape. IFAC-PapersOnLine 2022 , 55 , 587–592. [ Google Scholar ] [ CrossRef ]
- Gérin-Lajoie, M.; Richards, C.L.; McFadyen, B.J. The negotiation of stationary and moving obstructions during walking: Anticipatory locomotor adaptations and preservation of personal space. Mot. Control 2005 , 9 , 242–269. [ Google Scholar ] [ CrossRef ]
- Chraibi, M.; Seyfried, A.; Schadschneider, A. Generalized centrifugal-force model for pedestrian dynamics. Phys. Rev. E 2010 , 82 , 046111. [ Google Scholar ] [ CrossRef ] [ PubMed ]
- Lee, B.H.; Jeon, J.D.; Oh, J.H. Velocity obstacle based local collision avoidance for a holonomic elliptic robot. Auton. Robot. 2017 , 41 , 1347–1363. [ Google Scholar ] [ CrossRef ]
- Wang, G.; Liu, M.; Wang, F.; Chen, Y. A novel and elliptical lattice design of flocking control for multi-agent ground vehicles. IEEE Control Syst. Lett. 2022 , 7 , 1159–1164. [ Google Scholar ] [ CrossRef ]
- Du, Z.; Li, W.; Shi, G. Multi-USV Collaborative Obstacle Avoidance Based on Improved Velocity Obstacle Method. ASCE-ASME J. Risk Uncertain. Eng. Syst. Part A Civ. Eng. 2024 , 10 , 04023049. [ Google Scholar ] [ CrossRef ]
- Mao, S.; Yang, P.; Gao, D.; Bao, C.; Wang, Z. A Motion Planning Method for Unmanned Surface Vehicle Based on Improved RRT Algorithm. J. Mar. Sci. Eng. 2023 , 11 , 687. [ Google Scholar ] [ CrossRef ]
- Singh, Y.; Sharma, S.; Sutton, R.; Hatton, D.; Khan, A. A constrained A* approach towards optimal path planning for an unmanned surface vehicle in a maritime environment containing dynamic obstacles and ocean currents. Ocean Eng. 2018 , 169 , 187–201. [ Google Scholar ] [ CrossRef ]
- Liu, Y.; Bucknall, R. Path planning algorithm for unmanned surface vehicle formations in a practical maritime environment. Ocean Eng. 2015 , 97 , 126–144. [ Google Scholar ] [ CrossRef ]
- Munasinghe, S.R.; Oh, C.; Lee, J.J.; Khatib, O. Obstacle avoidance using velocity dipole field method. In Proceedings of the International Conference on Control, Automation, and Systems, ICCAS, Budapest, Hungary, 26–29 June 2005; pp. 1657–1661. [ Google Scholar ]
- Abdallaoui, S.; Aglzim, E.H.; Kribeche, A.; Ikaouassen, H.; Chaibet, A.; Abid, S.E. Dynamic and Static Obstacles Avoidance Strategies Using Parallel Elliptic Limit-Cycle Approach for Autonomous Robots. In Proceedings of the 2023 11th International Conference on Control, Mechatronics and Automation (ICCMA), Agder, Norway, 1–3 November 2023; IEEE: New York, NY, USA, 2023; pp. 133–138. [ Google Scholar ]
- Shiller, Z.; Large, F.; Sekhavat, S. Motion planning in dynamic environments: Obstacles moving along arbitrary trajectories. In Proceedings of the 2001 ICRA. IEEE International Conference on Robotics and Automation (Cat. No. 01CH37164), Seoul, Republic of Korea, 21–26 May 2001; IEEE: New York, NY, USA, 2001; Volume 4, pp. 3716–3721. [ Google Scholar ]
- Large, F.; Laugier, C.; Shiller, Z. Navigation among moving obstacles using the NLVO: Principles and applications to intelligent vehicles. Auton. Robot. 2005 , 19 , 159–171. [ Google Scholar ] [ CrossRef ]
- Chen, P.; Huang, Y.; Mou, J.; Van Gelder, P. Ship collision candidate detection method: A velocity obstacle approach. Ocean Eng. 2018 , 170 , 186–198. [ Google Scholar ] [ CrossRef ]
- Chen, P.; Huang, Y.; Papadimitriou, E.; Mou, J.; van Gelder, P. An improved time discretized non-linear velocity obstacle method for multi-ship encounter detection. Ocean Eng. 2020 , 196 , 106718. [ Google Scholar ] [ CrossRef ]
- Van den Berg, J.; Lin, M.; Manocha, D. Reciprocal velocity obstacles for real-time multi-agent navigation. In Proceedings of the 2008 IEEE International Conference on Robotics and Automation, Pasadena, CA, USA, 19–23 May 2008; IEEE: New York, NY, USA, 2008; pp. 1928–1935. [ Google Scholar ]
- Van Den Berg, J.; Guy, S.J.; Lin, M.; Manocha, D. Reciprocal n-body collision avoidance. In Robotics Research: The 14th International Symposium ISRR ; Springer: Berlin/Heidelberg, Germany, 2011; pp. 3–19. [ Google Scholar ]
- Han, R.; Chen, S.; Wang, S.; Zhang, Z.; Gao, R.; Hao, Q.; Pan, J. Reinforcement learned distributed multi-robot navigation with reciprocal velocity obstacle shaped rewards. IEEE Robot. Autom. Lett. 2022 , 7 , 5896–5903. [ Google Scholar ] [ CrossRef ]
- Giese, A.; Latypov, D.; Amato, N.M. Reciprocally-rotating velocity obstacles. In Proceedings of the 2014 IEEE International Conference on Robotics and Automation (ICRA), Hong Kong, China, 31 May–7 June 2014; IEEE: New York, NY, USA, 2014; pp. 3234–3241. [ Google Scholar ]
- JEON, J.D. A Velocity-Based Local Navigation Approach to Collision Avoidance of Elliptic Robots. Ph.D. Thesis, Seoul National University, Seoul, Republic of Korea, 2017. [ Google Scholar ]
- Feurtey, F. Simulating the Collision Avoidance Behavior of Pedestrians ; The University of Tokyo, School of Engineering, Department of Electronic Engineering: Tokyo, Japan, 2000. [ Google Scholar ]
- Snape, J.; Van Den Berg, J.; Guy, S.J.; Manocha, D. The hybrid reciprocal velocity obstacle. IEEE Trans. Robot. 2011 , 27 , 696–706. [ Google Scholar ] [ CrossRef ]
- Snape, J.; Van Den Berg, J.; Guy, S.J.; Manocha, D. Independent navigation of multiple mobile robots with hybrid reciprocal velocity obstacles. In Proceedings of the 2009 IEEE/RSJ International Conference on Intelligent Robots and Systems, St. Louis, MO, USA, 11–15 October 2009; IEEE: New York, NY, USA, 2009; pp. 5917–5922. [ Google Scholar ]
- Kavraki, L.E. Computation of configuration-space obstacles using the fast Fourier transform. IEEE Trans. Robot. Autom. 1995 , 11 , 408–413. [ Google Scholar ] [ CrossRef ]
- Lee, I.K.; Kim, M.S.; Elber, G. Polynomial/rational approximation of Minkowski sum boundary curves. Graph. Model. Image Process. 1998 , 60 , 136–165. [ Google Scholar ] [ CrossRef ]
- Wein, R. Exact and efficient construction of planar Minkowski sums using the convolution method. In European Symposium on Algorithms ; Springer: Berlin/Heidelberg, Germany, 2006; pp. 829–840. [ Google Scholar ]
- Yan, Y.; Chirikjian, G.S. Closed-form characterization of the Minkowski sum and difference of two ellipsoids. Geom. Dedicata 2015 , 177 , 103–128. [ Google Scholar ] [ CrossRef ]
- Cheng, Z.; Chen, P.; Mou, J.; Chen, L. Multi-ship Encounter Situation Analysis with the Integration of Elliptical Ship Domains and Velocity Obstacles. TransNav. Int. J. Mar. Navig. Saf. Od Sea Transp. 2023 , 17 , 895–902. [ Google Scholar ] [ CrossRef ]
- Abichandani, P.; Lobo, D.; Muralidharan, M.; Runk, N.; McIntyre, W.; Bucci, D.; Benson, H. Distributed Motion Planning for Multiple Quadrotors in Presence of Wind Gusts. Drones 2023 , 7 , 58. [ Google Scholar ] [ CrossRef ]
- Phadke, A.; Medrano, F.A.; Chu, T.; Sekharan, C.N.; Starek, M.J. Modeling Wind and Obstacle Disturbances for Effective Performance Observations and Analysis of Resilience in UAV Swarms. Aerospace 2024 , 11 , 237. [ Google Scholar ] [ CrossRef ]
Click here to enlarge figure
Evaluation Indicators | Implication |
---|---|
VO space | VO in elliptic domains or circular domains |
Flight distance | Distance between UAVs during flight |
Occupied area | AVO-occupied area in 2D space |
Angle of velocity direction | Change in velocity direction throughout the flight of the UAVs |
Detour distance | Detour distance in compared to the original flight direction |
Total detour distance | Detour distance + remaining distance |
Single obstacle avoidance time | Average time per calculation of obstacle avoidance direction for UAV-A |
Parameters of UAV | UAV | v | o | |||||
---|---|---|---|---|---|---|---|---|
Scenario 1 | A | 20 | 0 | 0 | 0.2 | |||
B | 20 | 0.2 | ||||||
Scenario 2 | A | 20 | 0 | 0 | 0.2 | |||
B | 20 | 0.2 |
Pre-Set Scenario | Domain Hypothesis | Total Detour Distanc (m) | Single Obstacle Avoidance Time (s) | Velocity Reback Moment (s) |
---|---|---|---|---|
Scenario 1 | Elliptic domain | 54.37 | 0.0016 | |
Circle domain | 69.29 | 0.00056 | ||
Scenario 2 | Elliptic domain | 44.96 | 0.0043 | |
Circle domain | 56.65 | 0.0014 |
The statements, opinions and data contained in all publications are solely those of the individual author(s) and contributor(s) and not of MDPI and/or the editor(s). MDPI and/or the editor(s) disclaim responsibility for any injury to people or property resulting from any ideas, methods, instructions or products referred to in the content. |
Share and Cite
Liao, Y.; Wu, Y.; Zhao, S.; Zhang, D. Unmanned Aerial Vehicle Obstacle Avoidance Based Custom Elliptic Domain. Drones 2024 , 8 , 397. https://doi.org/10.3390/drones8080397
Liao Y, Wu Y, Zhao S, Zhang D. Unmanned Aerial Vehicle Obstacle Avoidance Based Custom Elliptic Domain. Drones . 2024; 8(8):397. https://doi.org/10.3390/drones8080397
Liao, Yong, Yuxin Wu, Shichang Zhao, and Dan Zhang. 2024. "Unmanned Aerial Vehicle Obstacle Avoidance Based Custom Elliptic Domain" Drones 8, no. 8: 397. https://doi.org/10.3390/drones8080397
Article Metrics
Article access statistics, further information, mdpi initiatives, follow mdpi.
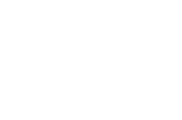
Subscribe to receive issue release notifications and newsletters from MDPI journals
IEEE Account
- Change Username/Password
- Update Address
Purchase Details
- Payment Options
- Order History
- View Purchased Documents
Profile Information
- Communications Preferences
- Profession and Education
- Technical Interests
- US & Canada: +1 800 678 4333
- Worldwide: +1 732 981 0060
- Contact & Support
- About IEEE Xplore
- Accessibility
- Terms of Use
- Nondiscrimination Policy
- Privacy & Opting Out of Cookies
A not-for-profit organization, IEEE is the world's largest technical professional organization dedicated to advancing technology for the benefit of humanity. © Copyright 2024 IEEE - All rights reserved. Use of this web site signifies your agreement to the terms and conditions.

An official website of the United States government
The .gov means it’s official. Federal government websites often end in .gov or .mil. Before sharing sensitive information, make sure you’re on a federal government site.
The site is secure. The https:// ensures that you are connecting to the official website and that any information you provide is encrypted and transmitted securely.
- Publications
- Account settings
Preview improvements coming to the PMC website in October 2024. Learn More or Try it out now .
- Advanced Search
- Journal List
- Sensors (Basel)
- PMC10490491

A Survey on Unmanned Underwater Vehicles: Challenges, Enabling Technologies, and Future Research Directions
Arif wibisono.
1 Department of Intelligent Mechatronics Engineering and Convergence Engineering for Intelligent Drone, Sejong University, Seoul 05006, Republic of Korea; rk.ca.ujs@onosibiwfira
Md. Jalil Piran
2 Department of Computer Science and Engineering, Sejong University, Seoul 05006, Republic of Korea; rk.ca.gnojes@narip
Hyoung-Kyu Song
3 Department of Information and Communication Engineering and Convergence Engineering for Intelligent Drone, Sejong University, Seoul 05006, Republic of Korea; rk.ca.gnojes@khgnos
Byung Moo Lee
Associated data.
Not applicable.
Unmanned underwater vehicles (UUVs) are becoming increasingly important for a variety of applications, including ocean exploration, mine detection, and military surveillance. This paper aims to provide a comprehensive examination of the technologies that enable the operation of UUVs. We begin by introducing various types of unmanned vehicles capable of functioning in diverse environments. Subsequently, we delve into the underlying technologies necessary for unmanned vehicles operating in underwater environments. These technologies encompass communication, propulsion, dive systems, control systems, sensing, localization, energy resources, and supply. We also address general technical approaches and research contributions within this domain. Furthermore, we present a comprehensive overview of related work, survey methodologies employed, research inquiries, statistical trends, relevant keywords, and supporting articles that substantiate both broad and specific assertions. Expanding on this, we provide a detailed and coherent explanation of the operational framework of UUVs and their corresponding supporting technologies, with an emphasis on technical descriptions. We then evaluate the existing gaps in the performance of supporting technologies and explore the recent challenges associated with implementing the Thorp model for the distribution of shared resources, specifically in communication and energy domains. We also address the joint design of operations involving unmanned surface vehicles (USVs), unmanned aerial vehicles (UAVs), and UUVs, which necessitate collaborative research endeavors to accomplish mission objectives. This analysis highlights the need for future research efforts in these areas. Finally, we outline several critical research questions that warrant exploration in future studies.
1. Introduction
Unmanned vehicles are developed to operate in various environments, including those operating on the surface of the water, known as unmanned surface vehicles (USVs) [ 1 ], those operating in the air as unmanned aerial vehicles (UAVs) [ 2 ], and those that operate underwater as unmanned underwater vehicles (UUVs). Currently, UUVs are growing in communication, control systems, and automation, and are even using Machine Learning processes such as setting trajectories, sensing, and managing flocks of unmanned vehicles [ 3 ].
As compared to unmanned vehicles that operate on the ground and those that operate in the air, which are able to access wireless communication systems properly, the conditions for unmanned vehicles that operate on the ground are different. This is due to the reliability of air in transmitting communication signals, especially with relay technology and shared resource management [ 4 ]. In spite of the fact that there are several disturbances, fault handling, recovery, and fault management can still be used to handle them [ 5 ]. Meanwhile, UUVs are affected by fluid properties, which interfere with signal propagation [ 6 ]. Furthermore, the energy required to transmit the signal is relatively high [ 7 ], whereas the signal received is lower, resulting in the loss of large data [ 6 , 8 ].
The underwater communication technologies can be categorized into five models: (1) acoustic communication that uses sound waves as a communication signal [ 9 ]; (2) optical communication uses visible and invisible light waves [ 10 ]; (3) wireless communication via radio waves [ 11 ]; (4) Satellite communication communicates with devices in the water through intermediary relays on the surface [ 12 ]; (5) direct electrical communication used for UUV charging docks [ 13 , 14 ].
There are four indicators that can be used to measure communication effectiveness: bit-error-rate (BER); signal-to-noise ratio (SNR) in decibels (dB); spectral efficiency, which is decrypted as the number of bits per second that can be transmitted through a specific bandwidth unit (bps/Hz); and energy efficiency. Specific propagation loss coefficient and noise floor are employed for different signal frequencies, as well as water characteristics (such as temperature, salinity, and depth) [ 6 ]. The signal-to-noise ratio measures the strength of the signal compared to the background noise. Better communication conditions are indicated by a higher SNR.
The propulsion system and dive system allow the UUV to move and control its depth in the water [ 15 ]. To propel the vehicle through the viscosity of water, one or more thrusters are used [ 16 , 17 ]. The propulsion system also controls the vehicle’s buoyancy, usually using a ballast system, which absorbs or releases water to regulate the vehicle’s buoyancy, as well as control systems to control dives and ascents [ 18 ]. UUVs can also be propelled by wings, fins, and hydrojets [ 19 , 20 , 21 , 22 ].
Five types of UUVs are considered in the control system, which focuses on control algorithms: the first is proportional–integral–derivative (PID) [ 23 ], which utilizes sensor feedback and processes it proportionally, integrally, and derivatively. Integrational means accumulating the errors over time and adjusting the control output based on those errors, while proportional means comparing; for example, comparing the output with the desired output. Derivative means calculating the error rate and adjusting the output based on that rate. The second type, model predictive control (MPC), predicts the future behavior of the system [ 24 ]. It uses a mathematical technique called sliding mode to achieve robust control of a system, regardless of variations in its dynamics or disturbances in the environment. Sliding mode control or SMC is a third method [ 25 ]. Fourth, adaptive control (AC) uses a mathematical model of the system to estimate the current state of the system [ 26 ] and then adjusts the control inputs accordingly. Last but not least, adjusting UUV trajectory [ 27 ] or UUV herd localization uses Artificial Intelligence and Machine Learning (ML) [ 28 ]. Control algorithms are chosen based on the application and the requirements given, but there are a number of testing models that can be used, including (1) simulation; (2) closed-loop testing; (3) comparison with other algorithms; and (4) measuring key performance indicators (KPIs).
UUV uses several sensor devices in environmental sensing and recognition systems, and they are grouped based on how they work into four types: acoustic-sensors, such as sound navigation and ranging (SONAR) [ 29 ] and hydrophones [ 30 ], that detect and locate objects in water using sound waves; optical sensors, such as cameras and light detection and ranging (LiDAR) [ 31 , 32 ], that use light waves to capture images and gather data on the surface of the water; chemical-sensors [ 33 ], which can detect and measure the concentration of dissolved gases, pollutants, and other substances in the water [ 34 ]; and physical-sensors [ 35 ], which can detect and measure the properties of water and its surroundings. Using these four types of sensors together can provide a comprehensive picture of the underwater environment for navigation, object detection, and environmental monitoring. As a result of the sensing function, UUV operations can be conducted to collect various types of data, such as bathymetry [ 36 ], water quality [ 32 ], images of the seafloor [ 32 ], etc., depending on the mission and the sensors and instruments installed on the vehicle.
Several types of navigation systems can be used by unmanned vehicles underwater, including inertial navigation systems (INS) which use accelerometers and gyroscope sensors to measure linear and angular motion, and once the angular position and orientation are known, the location of the UUV can be estimated [ 37 , 38 ]. Another application using similar measurement data is the Doppler velocity log (DVL), which measures the relative velocity of UUV to water by using the theory of the Doppler effect [ 39 , 40 , 41 , 42 ]. Furthermore, the third system is the use of the global navigation satellite system (GNSS) and global positioning system (GPS) which utilize satellite signals to determine UUV position and speed [ 43 ] based on the difference between a signal transmitted by a satellite and a signal received by a GPS receiver. In addition, the GNSS system transmits longitude, latitude, altitude and time signals simultaneously [ 44 , 45 , 46 ]. Currently GPS/GNSS technology can only be used for vehicles operating in the sea surface environment, such as the Vessel UUV, and further research is needed in order to apply it to the underwater environment. Furthermore, the fourth system introduced in this paper is the use of sound waves to determine the position and speed of the UUV using an acoustic navigation system (ANS) [ 47 ]. Acoustic signals can be measured by measuring the time delay or the Doppler shift [ 48 ]. To track the position and orientation of the UUV, the last or fifth system is the use of visual odometry (VO) [ 49 ]. UUV localization optimization can be improved by integrating navigation systems, various sensors, and other data sources, as mentioned above. In summary, the key point is that GNSS can provide absolute position measurements, while INS and DVL can provide accurate speed measurements. This integration is referred to as sensor fusion, i.e., when sensors and data sources are integrated in one unit.
The primary source of energy for most unmanned underwater vehicle is batteries [ 50 ]. Depending on the specific application and mission requirements, these batteries can be rechargeable or disposable. UUVs have also used fuel cells [ 51 ], hydrogen [ 52 ], and solar panels [ 53 ], which require cooperative control systems to harvest solar energy continuously if the energy source battery backup is low. The UUV’s energy requirements and supply will be determined by the specific mission and operating conditions, such as mission duration, water depth, and payload type.
Additionally, several studies predict an increase in demand for underwater vehicles in the future [ 54 , 55 ]. The underwater wireless sensor network (UWSN) [ 56 ] infrastructure is also being utilized to support the continuity of operation of the UUV which has limitations when operating in water. Because it is supported by air–water boundary communication technology (AWBC) [ 57 , 58 ] and underwater cyber physical system (UCPS) for data collection needs [ 59 , 60 ], it is possible to collaborate on communication and control systems [ 57 , 58 ] for multi-environmental unmanned vehicles. Internet of Underwater Things or (IoUT) localization techniques based on reinforcement learning [ 61 ] can be used in collaboration with vessel-based UUVs to collect marine survey information [ 62 ]. Several other studies, such as those based on controversy adjudication (CATM) [ 63 ], are expected to further improve the effectiveness of UUV operations underwater and eliminate its limitations [ 64 ].
While the poor supporting technology in the underwater environment has implications for many things, including difficulties controlling the UUV, reduced situational awareness, difficulty executing missions, and the risk of losing the UUV [ 54 ], reliable supporting technology is urgently needed. Furthermore, the entire discussion outlined above opens this survey paper and invites readers to better understand the concept of unmanned vehicles operating underwater, as well as the importance of technological support.
This paper employs state-of-the-art writing, which flows and makes it easy for readers to read, including an introduction, which provides information about unmanned vehicles, their types, communication technology, propulsion and dive systems, control systems, sensors, localization, energy resources, and supplies that support the operation of UUVs under water. It explains the workings of the supporting technology from a technical and a mathematical perspective. We also explain how the literacy survey was conducted, starting with frequently asked research questions, statistical trends, and the keywords used to find articles that support the general and specific statements.
An integrated mathematical approach is then used to discuss the work system of each UUV-supporting technology, including communication technology, propulsion, control systems, sensing, localization, and energy resources. Next, through simulations, the performance of technology supporting UUV operations is simulated. Then, we examine the performance gaps found in research in supporting technology performance, discussing the latest issues such as the implementation of the Thorp model for the distribution of shared resources for communication and energy, as well as a joint-design of USV–UAV–UUV operations for completing a mission that requires future research contributions. Finally, we outline several critical open research challenges for future studies.
The paper is organized as follows: an introduction that covers types of unmanned vehicles, UUV support technology, research contributions, and the state of the art. This is followed by a discussion of related works, including research questions, existing surveys, and statistical trends. Next, a coherent and mathematical approach is used to discuss the UUV work system and conduct a performance simulation. The paper concludes with a discussion on future research directions and a summary of the performance gaps found.
Recent research advances in the field of underwater vehicles encompass various aspects, including sensing, cross-boundary cooperation patterns of autonomous vehicles, optimization of cross-boundary communication utilizing signal propagation theories within water and signal modulation engineering, the adoption of successful models from autonomous vehicle types operating in terrestrial-aerial environments, and the incorporation of deep learning and deterministic Artificial Intelligence technologies. Collectively, these aspects represent future research avenues that can be developed based on what the researcher has presented in this review.
Figure 1 shows the structure of this survey paper more detail.

Organization of the paper.
2. Related Works
In addition to using a correlational method, this survey considers literacy novelty. In order to perform this task, we follow the following steps.
- Identifying research topics by considering the need for survey contributions, summarizing questions that are frequently asked in similar surveys, etc.;
- Examining similar survey papers to identify subtopics that have not been reviewed;
- Searching for answers using general and specific keywords;
- Identifying future research directions by looking at trends statistically.
3. Research Questions
In this field of research, we are motivated to explore and answer some frequently asked questions (FAQ). These questions are clearly summarized in Table 1 .
FAQ about UUV surveys and their supporting technologies.
S. No | Related Research Questions | Answer |
---|---|---|
RQ1 | How can UUVs be used to collect data? | Depending on the mission and the sensors and instruments installed on the vehicle, UUVs can collect a variety of data such as bathymetry, water quality, imagery of the seafloor, and other types [ , , , , , , ]. |
RQ2 | How do UUVs navigate and control their movements in water? | To move through water, UUVs use navigation and control systems. An inertial navigation system, a GPS system, and a sonar system are a few examples [ , , , , , , , , , ]. |
RQ3 | What are the ways in which UUVs communicate and store data? | UUVs have wireless communication systems for transmitting data and storage devices for storing data, such as hard drives or solid-state drives [ , , , , ]. |
RQ4 | How do UUVs obtain power? | Alternative energy sources such as fuel cells, batteries, and lithium-ion batteries are used to power UUVs [ , , , ]. |
RQ5 | How can UUVs be equipped with payloads? | UUVs can be equipped with various payloads to perform specific tasks such as sampling, imaging, and mapping [ , , , , , , , ]. |
RQ6 | What are the steps involved in planning and controlling a UUV survey? | In order to plan and control their missions, UUVs and UAVs use mission planning and control software. The software can be used for navigation, sensor control, and data analysis [ , , , , , , , ]. |
RQ7 | Why should UUVs be used for surveys? | UUVs provide many advantages over traditional survey methods, such as flexibility, cost-effectiveness, and the ability to access areas that are difficult or dangerous for divers [ , , , , , ]. |
RQ8 | How do UUV surveys present challenges? | UUV surveys can be challenging due to the need for specialized equipment and expertise, as well as the inability to operate in poorly lit or difficult-to-access underwater environments [ ]. |
3.1. Existing Surveys
By comparing FAQs with answers, we provide a summary of statements in other research articles that answer the questions raised, which can be seen clearly in Table 2 .
Using existing surveys as references, mindmaps are compiled, comparisons are made, research approach models are applied, future trends are examined, and contributions are determined.
Research | Year | RQ1 | RQ2 | RQ3 | RQ4 | RQ5 | RQ6 | RQ7 | RQ8 |
---|---|---|---|---|---|---|---|---|---|
Al Guqhaiman et al. [ ] | 2021 | ||||||||
Wang et al. [ ] | 2022 | ||||||||
Zhang et al. [ ] | 2022 | ||||||||
Shi et al. [ ] | 2020 | ||||||||
Wu et al. [ ] | 2020 | ||||||||
Hong et al. [ ] | 2020 | ||||||||
Nakath et al. [ ] | 2022 | ||||||||
Karmozdi et al. [ ] | 2020 | ||||||||
Klein et al. [ ] | 2022 | ||||||||
Braginsky et al. [ ] | 2020 | ||||||||
Perea-Storm et al. [ ] | 2020 | ||||||||
Jiang et al. [ ] | 2022 | ||||||||
Yin et al. [ ] | 2022 | ||||||||
Sezgin et al. [ ] | 2022 | ||||||||
Hou et al. [ ] | 2023 | ||||||||
Neira et al. [ ] | 2021 | ||||||||
Luo et al. [ ] | 2021 | ||||||||
Lindsay et al. [ ] | 2022 | ||||||||
Purser et al. [ ] | 2022 | ||||||||
Luo et al. [ ] | 2022 | ||||||||
Yan et al. [ ] | 2020 | ||||||||
Fang et al. [ ] | 2022 | ||||||||
Jiang et al. [ ] | 2023 |
3.2. Keyword Used
As part of this research, general and specific keywords are used to locate supportive references, compile mindmaps, compare studies, search for appropriate research approach models, examine future trends, and determine what contribution is needed in this area of research. References used have a publication year limit of 2017–2023 with a minimum citation level of 2 and are from reputable journals. Keywords are used to identify research directions and support general theoretical and technical statements. For technical discussions, special formulations, and simulations, special keywords are used. As shown in Figure 2 , the search results based on general and specific keywords in the field of UUV and its supporting technology are illustrated in a branching graph.

Survey taxonomy based on general to specific keywords.
3.3. Statistics Trends
We classify acronyms used as unique identifiers for several studies using search engines and reference management applications. To understand trends in this field of research, we also identify publications by the publisher in the second table. The grouping data is shown in Table 3 and Table 4 :
List of acronyms.
Acronym | Definition | Acronym | Definition |
---|---|---|---|
USV | Unmanned Surface Vehicle | GPS | Global Positioning System |
UAV | Unmanned Aerial Vehicle | ANS | Acoustic Navigation System |
UUV | Unmanned Underwater Vehicle | VO | Visual Odometry |
EC | Energy Consumption | UWSN | Underwater Wireless Sensor Network |
TX | Tranceiver | ROV | Remotely Operated Vehicle |
RX | Receiver | AWBC | Air–Water Boundaries Communication System |
SS | Spherical Spreading | UCPS | Underwater Cyber–Physical System |
BER | Bit Error Rate | IoUT | Internet of Underwater Things |
SNR | Signal-to-Noise Ratio | CATM | Controversy-Adjudication-Based Trust Management |
PID | Proportional Integral Derivative | MPC | Model-Predictive Control |
SMC | Sliding Mode Control | AWBC | Air–Water Boundaries Communication System |
AC | Adaptive Control | UCPS | Underwater Cyber–Physical System |
AI | Artificial Intelligence | IoUT | Internet of Underwater Things |
ML | Machine Learning | CATM | Controversy-Adjudication-Based Trust Management |
KPI | Key Performance Indicators | CDV | Cross-Domain Vehicle |
SoNAR | Sound Navigation and Ranging | DOF | Degrees of Freedom |
LiDAR | Light Detection and Ranging | ITSM | Integral Terminal Sliding Mode |
INS | Inertial Navigation System | FITSM | Fast Integral Terminal Sliding Mode |
DVL | Doppler Velocity Log | AUV | Autonomous Underwater Vehicle |
GNSS | Global Navigation Satellite System | DRL | Deep Reinforcement Learning |
RGB | Red Green Blue | HSV | Hue Saturation Value |
DVL | Doppler Velocity Log | UTM | Universal Transverse Mercator |
WGS | World Geodetic System | RIS | Reconfigurable Intelligence Surface |
List of publishers and the number of publications surveyed.
Database | Number of Papers |
---|---|
IEEE Xplore | 49 |
ScienceDirect-Elsevier | 4 |
MDPI | 7 |
SpringerLink | 8 |
Hindawi | 5 |
Wiley | 4 |
Inder Science Online | 1 |
4. UUV Work System
A mission scenario illustrates how an unmanned vehicle works in an underwater environment.
4.1. Underwater Commmunication
In communication systems that use the hexagonal model for localization (buoy) as a ground station transmitter relay, the hexagonal model represents the sphere-shaped Earth, which is mathematically divisible by the hexagonal model. Relay placement by measuring the effective beacon distance is calculated as follows [ 67 ]:
Network coverage ratio is represented by C , summazation volume monitoring area is denoted by V , and monitoring area of node V n ( i ) is denoted as n i . It is assumed that the nodes are equipped with an omidirectional antenna that monitors in all directions (sphere area) which has a radius and is denoted in r s .
The signal transmission system uses a combination of acoustic communication for long-distance transmission at sea depth [ 9 ], optical for fast short-range communication in the depths of the sea [ 10 ], and radio waves for communication at sea level between the USV, relay station, and ground transmitting station [ 11 ]. Additionally, the USV is equipped with a direct electrical [ 13 , 14 ] system that allows for the recharging of UUV swarms operating underwater and a swarm drone carrier with an air–water boundary communication system [ 29 ]. Repeating the previous statement that a higher SNR ratio indicates better communication conditions, to calculate the SNR value [ 29 ], we proceed with the following:
where I r is the received light intensity and σ n 2 is the variance of noise within the system. I r can be represented as
In the context of the study, the following terms are defined: I represents turbulence-induced channel fading, following a lognormal distribution; L t and L c h denote temperature and channel loss, respectively; I s represents irradiance in the pattern of ideal emission; ψ is the incident angle of the receiving plane; and A represents the active area of the photodiode.
In the given context, the following terms are defined: q is the charge of an electron; P n represents the solar noise power, B denotes the signal bandwidth; and ℜ is the responsivity of the photodiode.
4.2. Dive System
A herd of UUVs in the mission scenario consists of three types, each distinguished by its propulsion and dive system. The first UUV is propelled by propellers on all sides, referred to as omnidirectional [ 15 , 16 , 17 ], and is able to move in any direction to maximize its efficiency. The Buoyancy force generated by the object expressed in newton ( N ) , Δ B o u y a n c y can be represented as:
where π is the mathematical constant pi, approximately equal to 3.14159; ρ w a t e r is the density of water expressed in (kg/m 3 ); D o u t is the outer diameter of the submerged object expressed in meters (m); and L is the length of the submerged object expressed in meters (m).
The origin axis of the vehicle is located at the middle of the x – y axis and at the bottom of the z -axis. To calculate the center of gravity with respect to the origin axis, the following formula can be used:
The following terms are defined in the context of the study: W i , W a i , X i , Y i , Z i , and X a i , Y a i , Z a i represent the weight component, added weight by ballast water, offset of the center of weight component, and added weight component, respectively. For UUVs that utilize an omnidirectional dive system, a visual representation of the system can be observed in Figure 3 a.

( a ) Omnidirectional, ( b ) hydrojet, ( c ) hydrojet maneuver and ( d ) undulating propulsion and dive system [ 16 , 21 , 22 ].
The second type of UUV uses a hydrojet propulsion system and diving system [ 22 ]. Known as a cross-domain vehicle (CDV), this vehicle can operate in both surface and underwater environments because it is equipped with a ballast tank, propulsion system, and rudder. The buoyancy law [ 68 ] is used in this approach, which is based on:
In the given context, the following terms are defined: Δ B represents the net buoyancy in k g ; F G denotes the gravitational force; F B represents the buoyant force exerted by the fluid on the floating object; M is the total mass of the object; ∇ denotes the volume of the fluid displaced by the object; and ρ is the density of the fluid. The rudder is responsible for directing the CDV into three modes of motion, as outlined below.
- Dynamic model of surface state: m U i = P cos θ − F cos ( α − θ ) − F f cos ( β − θ ) − F r cos ( γ − θ ) , (10) m U j = F sin ( α − θ ) + F f sin ( β − θ ) + F r sin ( γ − θ ) + P sin θ − G , (11) J k θ = M + F f l f sin β − F r l r sin γ . (12)
- Dynamic model of the underwater state: m U i = P cos θ − F cos ( α + θ ) − F f cos ( β − θ ) − F r cos ( γ − θ ) , (13) m U j = F v cos θ + F f sin ( β − θ ) + F r sin ( γ − θ ) + P sin θ + G − F sin ( α + θ ) , (14) J k θ = F v l v + F f l f sin β − F r l r sin γ − M . (15)
- Underwater and surface transition state: m U i = P cos θ − F cos ( α − θ ) − F f cos ( β − θ ) − F r cos ( γ − θ ) − F v sin θ , (16) m U j = F v cos θ + F f sin ( β − θ ) + F r sin ( γ − θ ) + P sin θ + F sin ( α + θ ) − G , (17) J k θ = F v l v + F f l f sin β + M − F r l r sin γ . (18)
Table 5 provides detailed information regarding each symbol and unit. An illustration of a UUV that uses a propulsion system and hydrojet diving is shown in Figure 3 b, while Figure 3 c illustrates movement maneuvers resulting from the three equations above.
The definitional variable furnishes comprehensive insights into every symbol and unit employed to elucidate the equations within a cross-domain vehicle (CDV) motion system [ 22 ].
Symbol | Explanation |
---|---|
The angle of pitch | |
The force exerted by the front hydrofoil | |
The distance between the front hydrofoil and the center of gravity | |
The distance between the rear hydrofoil and the center of gravity | |
The force applied by the rear hydrofoil | |
The thrust generated by the water jet propeller | |
The combined buoyancy and drag force of the CDV | |
The force of gravity | |
The force generated by the vertical propeller | |
The moment caused by buoyancy | |
The angle between and the axial direction of the CDV | |
The angle formed between and the axial direction of the CDV | |
The angle included between and the axial direction of the CDV | |
The angle of roll | |
The velocity components in the and directions | |
The distance between the vertical propeller and the center of gravity | |
The moment around the axis |
The pitch angle ( θ ) of the cross-domain vehicle (CDV) induces lift forces ( F f ) on the hull through the action of the front hydrofoil. The magnitudes of these forces are determined by considering the distances ( l f and l r ) from the center of gravity to the front and rear hydrofoils, respectively. The angle β represents the included angle between the lift force ( F f ) and the axis direction of the CDV. This angle is calculated through inverse trigonometric functions based on the lift and drag components of the front hydrofoil. A similar analysis can be performed for the lift force ( F r ) generated by the rear hydrofoil on the hull. This interplay of pitch angles, hydrofoil forces, and geometric considerations contributes to the dynamic equilibrium and motion characteristics of the CDV in its operational environment.
Futhermore, a third type of UUV is the undulating UUV; this means that the UUV is equipped with fins that function simultaneously as a propulsion and diving system. In their study, the authors provided a comprehensive account of the intricate six-degrees-of-freedom (DOF) motion exhibited by the manta ray robot [ 21 ]. This encompassing movement involves a spectrum of displacements including longitudinal, sideways, and vertical shifts, in addition to the nuanced roll, pitch, and yaw rotations. The manta ray robot’s overall motion finds representation through elegant flowing vectors: η = [ x , y , z , φ , θ , ψ ] T , v = [ u , v , w , p , q , r ] T , and τ = [ X , Y , Z , K , M , N ] T . Here, η captures the vector describing the robot’s position and attitude in the earth-fixed frame, while v characterizes the body-fixed linear and angular velocity vector. The intricate interplay of forces and moments acting on the robot within the body-fixed frame is succinctly described by τ , as depicted in Figure 4 . By assuming the robot’s movement takes place in an ideal fluid at a consistent velocity of V 0 and by disregarding the effects of water’s viscosity and inertia, the equations governing the robot’s motion are elegantly simplified, particularly in the vertical plane.

Sensing tracking target involves a sequential process depicted in ( a ). Initially, upon target detection, the measured aspect angle remains unresolved, as evidenced by both port and starboard rays extending from the vehicle. Subsequent maneuvering and data collection during the second leg facilitate the completion of the resolve-ps-ambiguity behavior, leading to the determination of target orientation. Once resolved, the keep-broadside behavior utilizes the obtained vehicle-relative bearing measurement, indicated by a single ray extending from the vehicle, to effectively track the target’s movements. ( b ) In the realm of optical sensing, a progression unfolds from the top left to right, followed by a downward transition. The initial view showcases the original image captured. Subsequently, the HSV image processing unveils a distinct perspective, enabling the extraction of key color information. Finally, the journey culminates in the presentation of color masks, representing specific regions of interest and aiding in targeted analysis. This holistic sensing approach harmonizes various stages of processing, culminating in a comprehensive understanding of the tracked target’s dynamics and optical properties [ 30 , 34 ].
The intricate interplay of forces and factors influencing the manta ray robot’s behavior is encapsulated by a set of key variables. These include G , representing the robot’s gravitational force; G 0 , which captures the robot’s gravity in the absence of the mass block; and Δ G , signifying the gravitational impact of the mass block itself. Furthermore, the buoyant forces at play are delineated by B , indicating general buoyancy; B 0 , reflecting the buoyancy when equated to the robot’s gravity; and Δ B , representing an adjustable buoyancy component. Within this fluid dynamic context, F X and F Z step forward as vital descriptors of fluid resistances acting along the x -axis. The fundamental physical attributes of the robot and its environment are given voice by m denoting the robot’s mass and ρ standing for the water density. The geometric characteristics of the robot are encapsulated by S x and S y , the maximum transverse and longitudinal cross-sectional areas, while the nuanced hydrodynamics are unveiled by C x and C y , the hydrodynamic parameters that contribute to the robot’s interaction with its surroundings. Each of these variables weaves together to define the intricate dance of forces and dynamics that shape the manta ray robot’s journey. Refer to Figure 3 d for a visualization of the propulsion and undulating diving system.
4.3. Control
The first drone used a model-predictive-control algorithm to set the maneuvers and trajectory of the herd UUV, which predicts future behavior and optimizes a control action based on that prediction. According to Saback et al. [ 24 ] and Heshmati-Alamdari et al. [ 68 ], whose logic flow employs a discrete-time form:
x k = [ η k T , v r k T ] T ∈ R 8 represents the state vector at time step k , which includes the position and orientation of the vehicle with respect to the inertial frame τ , and the relative linear and angular velocity of the vehicle with respect to the water. m 11 , i = 1 , … , 4 , X u , Y v , Z w , N r < 0 , X u | u | , Y v | v | , Z w | w | , N r | r | > 0 , and d t denote the mass terms, linear, and quadratic drag terms, and sampling period, respectively. The control input of the system is τ k = [ τ p k , τ s k , τ v k , τ l k ] T ∈ R 4 , representing the thrusters’ forces. Ocean current profile uncertainties are presented by δ k = [ 0 1 × 4 , δ u k , δ v k , δ w k , δ r k ] T ∈ D ⊂ R 8 , with D being a compact set and | | δ k | | ≤ δ . The perturbed system is modeled by taking into consideration the disturbances caused by ocean current profile uncertainties and dynamic parameter uncertainties denoted by Δ f ( x k , τ k ) . The vehicle’s dynamic parameters are assumed to have been identified through a proper identification scheme.
where T is the compact set of uncertainties bounded by γ ≥ 0 .
Let w k = γ k + δ k ∈ W ⊂ R 8 be the vector of uncertainties and external disturbances affecting the system. W is a compact set, defined as W = D ⊕ T , where D and T are also compact sets. Hence, W is bounded by | | w k | | ≤ w , where w = Δ y + δ . The dynamical equation of the system includes the vector of disturbances. However, for the nominal model, we neglect the effect of disturbances.
Employing a sliding-mode control algorithm, the second drone distinguishes itself through the implementation of a nonlinear control strategy. This strategy, characterized by its simplified logical framework, stands resilient against various disturbances and uncertainties that might arise during operation. The foundation of this innovative approach is rooted in the insightful work of Qiao et al., who have introduced a significant advancement in trajectory tracking control. Referred to as the fast integral terminal sliding mode control (FITSM) method, it represents a refined iteration of the ITSM method, as discussed in their authoritative references [ 25 ]. This amalgamation of cutting-edge techniques underscores the second drone’s prowess in achieving precise and robust control, poised to navigate challenges with a balanced blend of sophistication and adaptability.
In the context of this control framework, let us denote e ( t ) ∈ R as the tracking error, where its significance cannot be understated. To further shape the dynamics, a positive constant α is introduced, playing a pivotal role in influencing the system’s behavior. Additionally, we introduce the integers m and n , both of which are odd, with a clear constraint ensuring that n holds a greater value than m , and both remain greater than zero. The interplay of these elements intertwines to orchestrate a controlled system marked by intricate relationships and carefully orchestrated dynamics.
In the scenario where s ( t ) remains consistently at zero, effectively dictating that e ( t ) takes on the form − α e I ( t ) , a fascinating consequence unfolds within the realm of the fractional integrator. Under these conditions, the fractional integrator demonstrates its distinctive behavior and characteristics, showcasing the remarkable interplay between the components involved. This alignment not only offers insights into the system’s response but also unveils a unique facet of the fractional integration process that emerges when specific constraints are meticulously maintained.
The solution to the error dynamic provides crucial insights into the behavior and evolution of the system, unraveling the intricate interplay of variables and shedding light on its underlying dynamics.
Subsequently, the time at which e I ( t ) achieves convergence is determined, yielding a fundamental understanding of the temporal aspect of this critical variable’s behavior.
The convergence of the tracking error e ( t ) to the ITSM surface s ( t ) = 0 is achieved within a finite timeframe under the condition e ( t ) = − α e I ( t ) . This pivotal observation encapsulates the essence of the FITSM approach, characterizing it as a dynamic system where the interplay of variables culminates in this precise convergence scenario.
On the FITSM surface, s ( t ) = 0 (i.e., e ( t ) = − α e I ( t ) ), with β > 1 and other parameters defined as in the ITSM. The integrator is equivalent to
We adapt Chu et al.’s approach presented in [ 69 ] for developing a nervous system-based control system for AUVs using DRL-based control. This control system transforms local environmental information into an array S 1 = ( s 1 , s 2 ) , where s 1 represents the direction of the ocean current.
where, X ∈ [ 0 , 360 ] and s 1 represent the ocean current direction, while s 2 is a matrix with information on obstacles and ocean currents. To ensure AUV safety, we define a forbidden area around each obstacle considering changing ocean current directions and eddy currents.
where “1” represents the obstacle area; “2” indicates the prohibited zone; and “3” is the navigation region. The navigation state vector, S 2 = ( ϑ 1 , s 1 , s 2 , υ 1 ) , represents the angle between vector α → and β → , where α → and β → depict the points from the current and initial locations to the destination, respectively. This crucial value can be acquired through the following method:
The allocation of the destination within the AUV coordinate system is succinctly represented by the direction variables ( s 1 , s 2 ) , encapsulating the spatial arrangement of the target point. This system is meticulously defined as follows:
4.4. Sensing
The underwater unmanned vehicle (UUV) is outfitted with an array of sensors, which encompass passive, active, or fused sensing capabilities, enabling it to perceive the surrounding environment, underwater entities, and fellow UUVs. As detailed in [ 30 ], precision in measurements is attainable solely when the target resides within the sonar’s effective field of view. Upon acquiring a set of N measurements during the initial leg, the UUV’s behavior dictates both its operational mode and the corresponding turn angle δ ∈ [ 0 , π ] , calculated through the utilization of α ¯ . Operating under a non-preferential turning direction, the vehicle consistently executes right turns, expressed in radians as the turn angle unfolds.
The array configuration follows a structured pattern: the initial row corresponds to the first leg, the subsequent row pertains to the second leg, and the final row is designated for the broadside target. This systematic arrangement effectively organizes the data collected. To visualize the operational process of the system, refer to Figure 4 a, which visually captures the step-by-step functioning of the system.
Song et al. [ 34 ] suggested using a color screening filter based on hue–saturation value (HSV) to detect oil leaks. However, RGB color space, which is commonly used in optical displays, is not accurate enough for oil spill segmentation. In HSV space, the video can be converted to screen the oil spill region under the foreground mask. The computation methodologies for the S and V channels are well-defined: S is determined as v − min ( R , G , B ) , while V is calculated as m a x ( R , G , B ) . Equally integral is the calculation of the H channel, which follows the subsequent process:
By applying the threshold screening process to the HSV model, areas suspected of being affected by oil spills can be described effectively using the following equation:
The process involves setting lower threshold T _ i and upper threshold T ¯ i values for each color channel. These thresholds are applied to individual pixel values, denoted as I i , within the HSV color space. The resultant mask pixel value is assigned as 1 if and only if the pixel values across all three channels satisfy the threshold conditions; otherwise, it is assigned a value of 0. For a more comprehensive understanding of the HSV concept, refer to Figure 4 b, which provides a visual elucidation of the HSV model’s intricacies. This visual aid serves to enhance clarity in grasping the nuances of HSV-based thresholding.
Employing the HSV extraction method within the realm of autonomous intelligence for autonomous underwater vehicles (AUVs) holds profound significance. This technique empowers AUVs to not only detect but also comprehend intricate color details present within their aquatic surroundings. Such an ability proves indispensable for the AUVs’ capacity to conduct thorough and insightful analyses of the underwater environment, thereby enhancing their overall capabilities and contributions to underwater exploration and research. AUV can detect objects based on distinctive color patterns, understand its surroundings, aid in navigation and obstacle avoidance, and enhance underwater observation and monitoring. Machine learning techniques can also be used for color-based object recognition, identifying environmental changes, and predicting environmental conditions. The development of autonomous intelligence through the combination of HSV extraction and Machine Learning holds the potential to improve AUV’s adaptability and interaction with the underwater environment, enhancing AUV’s mission performance in various applications, such as resource exploration, marine environmental monitoring, and scientific research beneath the ocean’s surface. More about the potential use of Machine Learning in AUV operations is discussed in the future research directions chapter.
4.5. Localization
We use two models for UUV localization. The first model, adapted from Braginsky et al. [ 42 ], uses the Doppler velocity log (DVL) method. DVL sends out acoustic beams and measures the Doppler frequency shift to compute the velocity and direction of each beam. Relevant definitions and calculations are as follows: R b n is the rotation matrix defined by Euler angles ( ϕ , θ , ψ ) .
Using the DVL method for UUV localization involves two models. The first sends out acoustic beams and measures the Doppler frequency shift for each beam to compute velocity and direction. To transform a vector from body-fixed to DVL coordinates, we use the following coordinate transformation with c α = cos ( α ) and s ( α ) . Correction of DVL measurement requires considering seafloor-to-platform angles during velocity calculations. Assuming the local seafloor is represented by the plane equation z i = a + b x i + c y i for i = [ 1 ⋯ 4 ] representing the DVL’s four altitude measurements, the measurement can be expressed in matrix form.
Using the seafloor equation and linear algebra, we estimate angles ϕ ^ and θ ^ s . Figure 5 a provides an illustration of the DVL and its measurement.

( a ) Two-dimensional seafloor example. The blue line represents the DVL signal that performs seafloor measurements and estimates. ( b ) GPS–GNSS Work [ 42 ].
We are demonstrating GPS- or GNSS-based location techniques for unmanned surface vehicles (USV). However, GPS measurements in water are inaccurate and misleading. According to Jiang et al. [ 43 ], GPS works on the principle of 2D Cartesian coordinates ( x , y ) and their respective covariances, using Universal Transverse Mercator (UTM) from the World Geodetic System (WGS84) ellipsoid. This theory is illustrated in Figure 5 b.
Measurement by GPS z t G P S provides the position and orientation parameters written in the equation:
Assuming that position ( x , y ) and orientation ( θ ) follow a Gaussian probability density function (PDF), the posterior given a GPS reading can be obtained according to the following:
where the PDF for the position is in
and the PDF corresponding to the orientation angle, which follows a wrapped normal distribution:
4.6. Energy Supply
In addition to internal battery power, UUVs can utilize potential renewable energy sources in the aquatic environment. Baik et al. [ 51 ], Sezgin et al. [ 52 ], and Tian et al. [ 53 ] have explored this topic, and we summarize their findings in Table 6 .
Summary of references regarding renewable energy sources that can be considered as alternative sustainable energy supplies for UUV operations [ 53 ].
Sustainable Energy Sources | The Form of Energy Generated | Types of Vehicles That Can Apply It |
---|---|---|
Hydrogen–Oxygen fuel cell | Heat–Electric energy | ROV, AUV |
Photovoltaic energy | Heat–Electric energy | AUV, USV, and UG |
Ocean wave power | Mechanical–Electrical energy | USV, AUV |
Heat energy | Pressure–Electric energy | USV, AUV, and UG with profilling float |
Marine current energy | Mechanical–Electric energy | UG, AUV |
Abbreviations: ROV, remotely operated vehicle; AUV, autonomous underwater vehicle; USV, unmanned surface vehicle; UG, underwater glider.
Lindsay et al. [ 59 ] and Fang et al. [ 63 , 64 ] suggest using track lines and a collaborative approach with unmanned underwater vehicles and systemized underwater communication resources to improve energy efficiency during underwater survey missions.
We propose using UAVs equipped with reconfigurable intelligence surface (RIS) devices [ 70 , 71 ] as communication relays to expand coverage to previously unreachable water areas.
The joint operation scenario for UAV, USV, and UUV is shown in Figure 6 and summarized in Table 7 .

Scenario joint-operation: UAV, USV, UUV.
Summary of reference used to describe mission scenarios.
Research | Contribution | Outcome |
---|---|---|
Shen et al. [ ] | Buoy transmitter relay | Cover communications for surface waters area |
Qu et al. [ ] | Underwater wireless acoustic communication | Cover long-distance transmission at sea depth |
Al-Halafi et al. [ ] | Underwater wireless optical communication | Cover short-range communication at sea depth |
Gupta et al. [ ] | Underwater wireless communication radio-waves | Cover communication at sea level |
Page et al. [ , ] | Direct electrical system | A system that allows recharging for UUV |
Luo et al. [ ] | Air–water boundaries communication | Air–water communication link |
Wang et al. [ , , ] | Omnidirectional propulsion and dive system | A system that allows UUV free to move to all directions in underwater |
Shi et al. [ ] | Hydrojet propulsion and dive system | Allows the UUV to capable of operating in surface and underwater environments |
Zhang et al. [ ] | Undulating propulsion and dive system | UUV can move in an ideal fluid with a constant velocity |
Saback et al. [ , ] | MPC algorithm | Capable to optimize control of UUV based on the prediction |
Qiao et al. [ ] | Sliding mode control algorithm | Considers simpler logical systematics but can withstand disturbances and uncertainties |
Chu et al. [ ] | Deep reinforcement learning control base | Control system based on the nervous system with the ability to make decisions based on training and past learning experience in recognizing the environment |
Wolek et al. [ ] | Use of fusion sensors | Make UUV have the ability to recognize the environment, detect the presence of underwater objects, or detect the presence of fellow UUV herds |
Song et al. [ ] | Use of optical sensors | Accurately used to recognize the environment based on image capture |
Braginsky et al. [ ] | Localization using DVL method | Can compute velocity and direction UUV using acoustic-beam |
Perea-Strom et al. [ ] | Localization using GPS–GNSS | UUV localization using GPS–GNSS, while currently only being able detect USV, will, in the future, be applicable for types of UUV after the discovery of air–water boundaries communication technology |
Baik et al. [ , , ] | Supply of power | Potential renewable energy based on fuel cell, solar, wind, wave, thermal, and tidal current energy |
Reference was used to describe mission scenario.
In a series of sequentially arranged mission illustrations, we can form a comprehensive overview of potential collaboration patterns in the execution of missions involving various types of unmanned vehicles (Unmanned Aerial Vehicle (UAV); Unmanned Surface Vehicle (USV); and Unmanned Underwater Vehicle (UUV)). This concept is supported by networking and communication resources operating both underwater and on the surface. This collaborative approach integrates diverse unmanned vehicle platforms and holds the potential to revolutionize cross-domain mission execution.
Previous research references have discussed the benefits of each type of unmanned vehicle separately within the contexts of maritime and aerial missions. UAVs prove valuable for aerial monitoring and data collection, USVs are suitable for surface water monitoring and patrols, and UUVs can perform exploration and reconnaissance in underwater depths. However, to optimize these potentials, the integration of these elements into a coordinated framework is necessary.
In the described scenario, cooperation between UAVs, USVs, and UUVs is facilitated by a robust network and communication infrastructure in both the air and water environments. This enables these vehicles to share real-time information, coordinate movements, and execute complementary tasks across various environmental layers. For instance, UAVs can gather data from the air and transmit them to USVs on the surface, which can then direct UUVs to conduct further surveys in the ocean depths.
The application of this concept holds extensive potential, ranging from environmental monitoring missions, military reconnaissance, and exploration of marine resources to disaster response in maritime settings. By harnessing the strengths of various types of unmanned vehicles and the support of cross-air and water communication networks, we can create an adaptable, responsive, and efficient system for cross-domain missions. In conclusion, through the amalgamation of ideas from various preceding research references, we have delineated a comprehensive vision of how collaboration among unmanned vehicles, supported by cross-air and water communication networks, can shape new working paradigms in solving cross-domain missions. This concept harbors substantial potential for optimizing resource utilization, expanding operational scope, and delivering innovative solutions to diverse challenges in both maritime and aerial environments.
5. Performance Simulations
The previous section described a swarm of UUVs and their six leading supporting technologies: underwater communication; dive system; control; sensing; localization; and energy supply. These technologies are computationally simulated to estimate malfunctions and performance gaps.

5.1. Underwater Communication
In the introduction to this paper, we stated our aim to achieve a minimum B E R and maximum S N R for the communication system [ 6 ]. Direct communication is ideal for achieving this, while other methods such as acoustic communication have a long transmission time [ 72 ], optical communication has a short range [ 10 , 73 ], and wireless radio and satellite communications experience high attenuation in water.
We assumed that wired communication is the best alternative with low SNR and BER. However, it lacks flexibility. Optical communication is the second-best option with low SNR and BER, but it has limited range. On the other hand, the most equitable and feasible alternative for use is the acoustic communication model, which is directly transmitted underwater. Wireless and satellite communications perform poorly because they still require intermediary media to connect between the aerial and underwater domains.
5.2. Dive System
A diving system’s performance is measured by synchronizing the required energy with the resulting buoyancy. It uses the δ i = ( i t W − i b W ) approach, where δ i is a coefficient attitude-dependent magnitude of the moment produced by any offset between the center of mass ( i t ) and buoyancy ( i b ), and ( W ) is the weight of the vehicle [ 15 , 19 ]. This statement is supported by the simulation results shown in Figure 7 .

Simulation of a diving system using the law of buoyancy: δ i = ( i t W − i b W ) approach, where δ i is a coefficient attitude-dependent magnitude of the moment produced by any offset between the center of mass ( i t ) and buoyancy ( i b ) and ( W ) is the weight of the vehicle.
5.3. Control
The accuracy of the control system can be measured by the unmanned vehicle’s ability to follow a predetermined path using Cartesian coordinates ( X , Y , Z ) . The path-following controller’s goal is to enable autonomous navigation through a series of waypoints represented by a vector p k , defined by the equation ω k = [ x p k , y p k , z p k , V p k ] . The KPIs for the control system are V p k , which are shown in the simulation results in Figure 8 .

Control system: these waypoints, represented by a vector p k , can be written in the equation ω k = [ x p k , y p k , z p k , V p k ] , where x p k , y p k , and z p k are the absolute coordinates of the waypoints in the environment frame. V p k is the desired norm of the AUV velocity vector (mostly surge and dive) at the considered waypoint (can be 0).
5.4. Sensing
The UUV sensing performance, simulated as in Song et al. [ 35 ], converts environmental parameters into hue–saturation value (HSV) images for easy recognition via binary computing systems. The HSV threshold values T _ i and T ¯ i are set for each color channel, and the pixel value on the mask is 1 only when the pixel values on all three channels meet the threshold requirements. This is illustrated in Figure 9 .

Sensing: works by changing the environment image into light–dark parameters, where the light path (1 I i ≤ T ¯ i ) means it can be skipped and the dark band (0 T _ i ≤ I i ) must be avoided.
5.5. Localization
Localization performance is indicated by the ability to identify UUV position and orientation [ 43 ], with the GPS reading represented as p ( x t ∣ z t G P S ) ! ∼ p ( z t G P S ∣ x t ) · p ( x t ) = f ( x , y ) · f W N ( θ ) · p ( x t ) . This is supported by simulation results in Figure 10 .

Localization: works by identifying ( x , y ) position and ( θ ) as orientation.
5.6. Energy Supply
UUV battery performance depends on ambient temperature during charging. Low temperatures can reduce capacity, while high temperatures can increase battery aging and shorten lifespan. The equation P l o a d = ( R e ( Z r 0 ) ) I p 2 = ω 0 M 2 L s I P 2 Q s (Teeneti et al. [ 15 ]) can be modified by adding temperature ( T ) , as P l o a d = ( R e ( Z r 0 ) ) I p 2 = ω 0 M 2 L s I P 2 Q s · T . Figure 11 shows the optimal temperature range for storage capacity and lifespan.

Energy supply: battery state of charge (SoC), which is affected by ambient temperature, where P l o a d = ( R e ( Z r 0 ) ) I p 2 = ω 0 M 2 L s I P 2 Q s · T .
6. Performance Gaps
This research takes a general approach to the field of underwater vehicles and communications with the aim of identifying gaps for further investigation.
- Currently, underwater communication technology lacks a description of actual underwater signal conditions and instead relies on calculated approaches using existing research and surveys.
- We only simulate buoyancy and its energy requirements, without making comparisons.
- We have only measured UUV control system effectiveness based on time. However, we have not compared models using other parameters such as system autonomy or algorithm capabilities during system failure. Additionally, the optimal control algorithm should trace the shortest path based on our assumptions.
- We use the HSV method to convert environmental parameters into computer-readable notations of 0 and 1. However, due to the complex underwater environment and its impact on sensing functions, more research is necessary to identify additional parameters.
- Underwater localization technology only tracks GPS locations, identifying ( x , y ) position and θ orientation. Real-time, accurate positions of underwater vehicles require consideration of factors such as speed and orientation, whether diving or floating. Combining sensor functions to form an IMU and calculate position with GPS is also worth considering. Therefore, further research on this topic is needed.
- Simulations provided an overview of the ambient temperature’s effect on the vehicle’s state of charge capability for energy supply. However, further simulations are necessary, including battery life calculations, as underwater vehicles operate remotely and system failures due to power outages can be challenging to evacuate. Therefore, more research is required on this topic.
7. Future Research Directions
The study analyzes the performance gap between UUV operation support technology and the latest research in the field. Further research on resource management, including communication, dive system, control, sensing, localization, and energy supply, is being considered. The Throp model approach, adapted from Menaka et al. [ 74 ] and the CATM model by Jiang et al. [ 65 ], is used to optimize UUV operational capabilities using energy network infrastructure resources, communication, and underwater environment monitoring. Menaka [ 74 ] emphasizes the importance of resource management in communication and underwater vehicle research, which will become the backbone of future sea-related research. The study by Jiang et al. [ 65 ] supports this claim. Mathematical and computer simulations are conducted using IoUT-assisted underwater communication and sensing resource-sharing management.
In future research, we will propose joint operations of AUV, UUV, and USV, optimize the air–water boundary communication model [ 29 ], and model the use of reconfigurable intelligence surfaces (RIS) in AUVs to support these joint operations [ 71 ].
In the implementation of UUV operations, it is possible that Doppler effect may occur, similar to what happens in mobile cellular communication. This is due to the changes in the location and distance between the transmitter (TX) and receiver (RX) as the UUV moves relative to such changes. The extent of the impact of this can be calculated using basic mathematical approaches:
where f D is the detected frequency; f S is the frequency emitted by the source; v is the speed of sound waves through the underwater environment; v D is the relative detector speed with respect to the underwater environment; and v S is the speed of sound waves. A simple illustration is that there is a stronger frequency change as the source approaches the detector, and the opposite occurs as the source moves away. The values of the other parameters will automatically adjust to this phenomenon.
Some studies have anticipated this phenomenon and offered solutions, such as Li et al. [ 75 ] who also discuss the Doppler shift phenomenon in underwater acoustic (UWA) communication and propose a multicarrier orthogonal frequency division multiplexing (OFDM) communication system to overcome this challenge. Furthermore, Abdelkareem et al. [ 76 ] offer a Doppler shift compensation scheme by modifying the carrier frequency of OFDM subcarriers to match the Doppler shift frequency. In the study by Li et al. [ 75 ], an experiment was conducted using different numbers of subcarriers—512, 1024, and 2048—each with the corresponding number of active subcarriers: 484, 968, and 1936, respectively. The experiment focused on bit rates, employing a fixed guard interval of T g = 25 and an OFDM block duration T representing values of 42.8, 85.38, and 170.73, respectively. For these setups, the bit rates without coding resulted in 10.52 kb/s, 12.90 kb/s, and 14.55 kb/s. Furthermore, after applying a rate 2/3 channel coding, the bit rates were observed to 7.0 kb/s, 8.6 kb/s, and 9.7 kb/s, respectively [ 75 ].
From the observation above, it can be concluded that the working mechanism of OFDM multicarrier is capable of addressing the classic issue of Doppler shift, as indicated by the constant interval. However, there are certain concerns related to the limitations of this approach. Therefore, an examination of the Doppler effect, its implications, and strategies by which to mitigate it should also be included as part of future research directions in the field of unmanned underwater vehicles.
In addition to future issues regarding the technologies that can be integrated into AUV operations, there are also concerns about potential applications that may arise in the future given the rapid and advancing field of research. We predict that with the development of communication technology and the increasing demands in underwater vehicle research for various purposes such as resource exploration, marine environmental monitoring, and scientific research, there may also be a demand for research in the field of maritime transportation assisted by underwater vehicles, autonomous surface vehicles, and the utilization of Artificial Intelligence technology. Due to the limited references on the utilization of underwater vehicles, autonomous surface vehicles, and Artificial Intelligence technology, we have drawn some references from similar research fields applied to different devices and environments, such as the use of autonomous aerial vehicles in some case studies, including unmanned transportation options equipped with cognitive awareness capabilities that enable UAVs to actively recognize and understand their surroundings, making smarter and more responsive decisions in various situations, and studies using UAVs for autonomous traffic monitoring and management assisted by Artificial Intelligence.
Among the researchers examining and presenting their findings are Filippone et al. [ 77 ], who discussed the developments in urban air mobility and the use of rotorcraft as air transportation options in urban areas. Furthermore, Barmpounakis et al. [ 78 ] conducted a review on the application of unmanned aerial vehicle systems in transportation engineering, covering current practices and future challenges. Additionally, Cavaliere et al. [ 79 ] researched the development of proactive Unmanned Aerial Vehicles (UAVs) to enhance cognitive contextual awareness, aiming to integrate Artificial Intelligence and data processing technologies to enable UAVs to actively recognize and understand their surroundings, making smarter and more responsive decisions in various situations, including obstacle avoidance and mission adaptation based on environmental changes. Vlahogianni et al. [ 80 ] conducted research on model-free traffic condition identification using unmanned aerial vehicles (UAVs) and deep learning, developing a data processing model for traffic analysis from aerial perspectives and training deep learning algorithms to recognize traffic density patterns. Moreover, Trivedi et al. [ 81 ] developed a real-time vision-based vehicle detection and speed measurement system using morphology and binary logical operations. The research aims to create a method capable of accurately detecting vehicles in real time using visual data from cameras and accurately measuring vehicle speeds based on inter-frame movement, achieved by combining morphology and binary logical operation techniques.
If successfully implemented in terrestrial and aerial environments, the possibility of applying these techniques to surface and underwater environments in the case of autonomous underwater vehicles may also be feasible. However, researchers must also address factors that could lead to system and operational failures, considering the unique underwater environment distinct from other environments.
Furthermore, the development of applying Machine Learning for optimization across various sectors involving computation is also predicted to have an impact on the research and development of unmanned underwater vehicles. Several studies supporting this statement include Teng et al. [ 82 ], who investigated underwater target recognition methods based on deep learning frameworks (DL); Bhopale et al. [ 83 ], who developed obstacle avoidance systems based on reinforcement learning (RL) for autonomous underwater vehicles (AUVs); and Sands and Timothy [ 84 ], who developed deterministic Artificial Intelligence (AI) for unmanned underwater vehicles, where “deterministic” signifies that decisions made by the AI system possess a high degree of certainty and definiteness. This leverages predictability and consistency in the behavior of the AI system, implying that it produces identical responses or actions when confronted with the same situation.
Research by Sun et al. [ 85 ] involved developing a three-dimensional path tracking control system for autonomous underwater vehicles using a deep reinforcement learning (DRL) approach. Another study by Sun et al. [ 86 ] focused on mapless motion planning systems for AUVs using a policy gradient-based DRL approach. The main emphasis of policy gradient-based methods is on learning policies that link environmental states to actions that need to be taken by the agent. A notable advantage of this approach is its capability to address continuous action spaces and exhibit stochastic (probabilistic) policies.
From the entire series of reviews, several proposals can be summarized which are expected to be able to overcome common problems and issues regarding research in the field of unmanned underwater vehicles, among others shown in Table 8 .
Identified issues and proposed solutions.
Identified Issues | Proposed Solutions |
---|---|
Cross-border communication [ , ] | Optimization can be achieved through collaborative mission management involving UAVs, USVs, and UUVs, utilizing underwater communication network infrastructure resources. Additionally, the use of surface buoys as relays, assisted by satellites, can help extend the coverage area. |
Movement and dive system [ , , ] | Optimization can be achieved by employing biorobotic mechanisms or vehicles inspired by living organisms. This approach is more efficient in generating propulsion and minimizing energy consumption. |
Control system [ , , ] | Optimization can be achieved through the implementation of adaptive control mechanisms, enabling vehicles to autonomously react to obstacles along the mission path and optimize routes based on predictions. |
Sensing [ , ] | Optimization can be achieved by implementing a holistic sensing approach, wherein unmanned underwater vehicles can employ various types of sensors or diverse measurement methods in an integrated manner. This allows for a more comprehensive and profound understanding of the surrounding environment. |
Localization [ , ] | Optimization can be achieved through passive underwater localization techniques that utilize the Doppler Velocity Log (DVL) sensor to ascertain the vehicle’s position in relation to the seafloor surface. |
Supply energy [ , , ] | Optimization can be accomplished by harnessing the potential renewable energy available in the vicinity of the operational area, while considering the ambient temperature of each model. This is essential as the storage capacity of batteries is influenced by ambient temperature. |
Machine learning [ , , , , ] | All the sub-technologies that support the operation of unmanned underwater vehicles can be optimized through the utilization of Machine Learning. This includes the optimization of the sensing system to accurately recognize underwater objects, avoid and prevent collisions, make predictions, and formulate measurable decisions, should similar challenges arise in the future. |
The proposed solutions are expected to address common issues and challenges in the field of unmanned underwater vehicle research.
8. Conclusions
From the overall discussion above, several conclusions can be drawn by the researchers.
First, the prospects for the research and development of autonomous underwater vehicles (AUVs) in the future heavily rely on technological support. Collaborative communication technology capable of overcoming the limitations of signal transmission across air and water or signal transmission at water depths, utilizing support from the underwater communication network infrastructure, is crucial. It is essential for UUVs to have energy-efficient propulsion systems, sustainable power supply, reliable navigation control, and robust sensing capabilities, as the success of missions depends on these technological supports, as demonstrated in several simulations where efficiency and optimization are the primary focus of attention.
Second, resource management can enhance efficiency, and with good resource management supported by available infrastructure, joint operations involving AUVs, USVs, and UUVs can be deployed simultaneously.
Third, future research is likely to focus on implementing new technologies that can potentially be integrated to address research barriers and challenges. The application of autonomous underwater vehicles similar to autonomous vehicles operating on land and in the air can be considered by exploring new theories and utilizing the currently available technological support, including underwater communication technology and Artificial Intelligence.
Funding Statement
This work was supported by the Basic Science Research Program through the National Research Foundation of Korea (NRF) funded by the Ministry of Education (Grant No.: NRF-2020R1A6A1A03038540) and the Korean government (MSIT) (Grant No.: NRF-2023R1A2C1002656).
Author Contributions
This article was prepared through the collective efforts of all the authors. Conceptualization, A.W., M.J.P., H.-K.S. and B.M.L.; writing—original draft, A.W. and M.J.P.; writing—review and editing, A.W., M.J.P., H.-K.S. and B.M.L. All authors have read and agreed to the published version of the manuscript.
Institutional Review Board Statement
Informed consent statement, data availability statement, conflicts of interest.
The authors declare no conflict of interest.
Disclaimer/Publisher’s Note: The statements, opinions and data contained in all publications are solely those of the individual author(s) and contributor(s) and not of MDPI and/or the editor(s). MDPI and/or the editor(s) disclaim responsibility for any injury to people or property resulting from any ideas, methods, instructions or products referred to in the content.
Detection and Localization of Unmanned Aerial Vehicles Based on Radar Technology
- Conference paper
- First Online: 22 July 2021
- Cite this conference paper
- Sally M. Idhis 13 ,
- Takwa Dawdi 13 ,
- Qassim Nasir 13 ,
- Manar Abu Talib 14 &
- Yara Omran 13
Part of the book series: Lecture Notes in Networks and Systems ((LNNS,volume 218))
1203 Accesses
2 Citations
Ever since unmanned aerial vehicles (UAV), also known as drones, became available for civilian use, their risks increased. Along with that, the need to control and monitor them increased rapidly. In the literature, many researchers explored different techniques and developed genuine and hybrid systems to counter UAVs. These systems are not only found in academia, but different commercial options are also available. In this paper, the detection and localization using radar systems method are addressed. This method is one way to thwart drones. Other approaches are briefed in the background of this research study. Different radar technologies exist, and consequently different approaches to apply UAV detection and localization using radars. These approaches that are addressed in academia and the industry are surveyed in this research paper. Furthermore, the different possible radar categories are detailed to help the reader understand radar technology and thus its applications. In addition to commonly used localization algorithms for UAVs, the software and hardware implementation methods are also surveyed in this paper.
This is a preview of subscription content, log in via an institution to check access.
Access this chapter
Subscribe and save.
- Get 10 units per month
- Download Article/Chapter or eBook
- 1 Unit = 1 Article or 1 Chapter
- Cancel anytime
- Available as PDF
- Read on any device
- Instant download
- Own it forever
- Available as EPUB and PDF
- Compact, lightweight edition
- Dispatched in 3 to 5 business days
- Free shipping worldwide - see info
Tax calculation will be finalised at checkout
Purchases are for personal use only
Institutional subscriptions
Similar content being viewed by others
Micro UAV Crime Prevention: Can We Help Princess Leia?
Examination of Different Systems Used for UAV Detection and Tracking
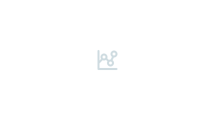
Survey on Computer Vision for UAVs: Current Developments and Trends
Güvenç, I., Ozdemir, O., Yapici, Y., Mehrpouyan, H., Matolak, D.: Detection, localization, and tracking of unauthorized UAS and Jammers. In: AIAA/IEEE Digital Avionics Systems Conference—Proceedings, Nov. 2017, vol. 2017-Sept. https://doi.org/10.1109/dasc.2017.8102043
Mototolea, D.: A study on the methods and technologies used for detection, localization, and tracking of LSS UASs. J. Mil. Technol. 1 (2), 11–16 (2018). https://doi.org/10.32754/jmt.2018.2.02
Article Google Scholar
Coluccia, A., Parisi, G., Fascista, A.: Detection and classification of multirotor drones in radar sensor networks: A review. Sensors (Switzerland), vol. 20, no. 15. MDPI AG, pp. 1–22, 01 Aug. 2020, https://doi.org/10.3390/s20154172
Drone Intrudes on Restricted Airspace Over Red Salmon Fire|News Blog. https://www.northcoastjournal.com/NewsBlog/archives/2020/10/18/drone-intrudes-on-restricted-airspace-over-red-salmon-fire . Accessed Nov. 12 2020
David, J.: Radar Fundamentals. Accessed 12 Nov. 2020. http://www.nps.navy.mil/faculty/jenn
Curry, G.R.: Radar Measurement and Tracking, pp. 165–193 (2005). Accessed 12 Nov. 2020. https://www.mendeley.com/catalogue/8dc6e5ae-ffc6-3e4e-8818-7bb7bc416925/?utm_source=desktop&utm_medium=1.19.4&utm_campaign=open_catalog&userDocumentId=%7Bc0b47b2e-8681-44e9-b8e3-0c2e75c6fd13%7D
Hauzenberger, L., Ohlsson, E.H., Swartling, M.: Drone Detection using Audio Analysis (2015). Accessed 12 Nov. 2020. http://lup.lub.lu.se/student-papers/record/7362609
Busset, J. et al.: Detection and tracking of drones using advanced acoustic cameras. In: Unmanned/Unattended Sensors and Sensor Networks XI; and Advanced Free-Space Optical Communication Techniques and Applications, Oct. 2015, vol. 9647, p. 96470F (2015). https://doi.org/10.1117/12.2194309
Unlu, E., Zenou, E., Riviere, N., Dupouy, P.E.: Deep learning-based strategies for the detection and tracking of drones using several cameras. IPSJ Trans. Comput. Vis. Appl. 11 (1), Dec. 2019. https://doi.org/10.1186/s41074-019-0059-x
Deilamsalehy, H., Havens, T.C.: Sensor fused three-dimensional localization using IMU, camera and LiDAR, Jan 2017. https://doi.org/10.1109/icsens.2016.7808523
Nguyen, P. et al.: Towards RF-based Localization of a Drone and Its Controller (2019). https://doi.org/10.1145/3325421.3329766
Nguyen, P., Ravindranathan, M., Nguyen, A., Han, R., Vu, T.: Investigating cost-effective RF-based detection of drones. In: DroNet 2016 - Proceedings of the 2nd Workshop on Micro Aerial Vehicle Networks, Systems, and Applications for Civilian Use, co-located with MobiSys 2016, June 2016, pp. 17–22 (2016). https://doi.org/10.1145/2935620.2935632
Singh Yadav, A., Kumar, S.: A Review Paper on Radar System, pp. 2349–6010 (2016)
Google Scholar
Hill, M.M.: Developing a Generic Software-Defined Radar Transmitter using GNU Radio (2012)
Patton, L.K.: A GNU Radio Based Software-Defined Radar, 12 Nov. 2020. https://corescholar.libraries.wright.edu/etd_all , https://corescholar.libraries.wright.edu/etd_all/91
Wellig, P. et al.: Radar systems and challenges for C-UAV. In: Proceedings International Radar Symposium, Aug. 2018, vol. 2018-June. https://doi.org/10.23919/irs.2018.8448071
Zhang, Y., Cruz, J.R., Dyer, J.W.: Effectiveness of Electronic Counter Measures (ECM) on Small Unmanned Aerial Systems (Suas): Analysis and Preliminary Tests a Thesis Approved For The School Of Electrical and Computer Engineering (2018). Accessed 12 Nov. 2020. https://shareok.org/handle/11244/299802
Ezuma, M., Erden, F., Anjinappa, C.K., Ozdemir, O., Guvenc, I.: Micro-UAV Detection and Classification from RF Fingerprints Using Machine Learning Techniques. In: IEEE Aerospace Conference Proceedings, Mar. 2019, vol. 2019-Mar. https://doi.org/10.1109/aero.2019.8741970
Radartutorial (2020). https://www.radartutorial.eu/index.en.html . Accessed 12 Nov. 2020
Jahangir, M., Baker, C. : Persistence surveillance of difficult to detect micro-drones with L-band 3-D holographic radar TM , Oct. 2017. https://doi.org/10.1109/radar.2016.8059282
Ivashko, I.: Radar Networks Performance Analysis and Topology Optimization (2016). https://doi.org/10.4233/uuid:1a6dab8e-ebbd-41a1-bd5e-866a9050fc68
Teng, Y.: Fundamental Aspects of Netted Radar Performance (2010)
521-1984-521-1984-IEEE Standard Letter Designations for Radar-Frequency Bands-IEEE Standard. Accessed 12 Nov. 2020. https://ieeexplore.ieee.org/document/29086
US7002511B1—Millimeter wave pulsed radar system—Google Patents
Curry, G.R.: Radar Essentials: A Concise Handbook for Radar Design and Performance Analysis. Institution of Engineering and Technology (2012)
Chapter 14: CW and FM Radar|Engineering360
Continuous wave ranging radar, 12 Oct. 1971
Brooker Graham, M.: Understanding Millimetre Wave FMCW Radars. Accessed 12 Nov. 2020. https://www.researchgate.net/publication/228979037_Understanding_millimetre_wave_FMCW_radars
RF Wireless Vendors and Resources|RF Wireless World (2020). https://www.rfwireless-world.com/ Accessed 12 Nov. 2020
4,176,351 1. Method of Operating A Continuous-Wave Radar, 18 Aug. 1978
Zhang, D., Kurata, M., Inaba, T.: FMCW radar for small displacement detection of vital signal using projection matrix method. Int. J. Antennas Propag. 2013 (2013). https://doi.org/10.1155/2013/571986
Moore, E.G., Rutherford, M.J., Valavanis, K.P.: Radar Detection, Tracking and Identification for UAV Sense and Avoid Applications (2019)
Caris, M., Johannes, W., Stanko, S., Pohl, N.: Millimeter wave radar for perimeter surveillance and detection of MAVs (Micro Aerial Vehicles). In: Proceedings International Radar Symposium, Aug. 2015, vol. 2015-August, pp. 284–287. https://doi.org/10.1109/irs.2015.7226314
Drozdowicz, J. et al.: 35 GHz FMCW drone detection system. In: Proceedings International Radar Symposium, June 2016, vol. 2016-June. https://doi.org/10.1109/irs.2016.7497351
Caris, M., Johannes, W., Sieger, S., Port, V., Stanko, S.: Detection of small UAS with W-band radar, Aug. 2017. https://doi.org/10.23919/irs.2017.8008143
Multerer, T. et al.: Low-cost jamming system against small drones using a 3D MIMO radar based tracking. In: European Microwave Week 2017: “A Prime Year for a Prime Event”, EuMW 2017—Conference Proceedings; 14th European Microwave Conference, EURAD 2017, June 2017, vol. 2018-January, pp. 299–302. https://doi.org/10.23919/eurad.2017.8249206
Laučys, A., et al.: Investigation of detection possibility of uavs using low cost marine radar. Aviation 23 (2), 48–53 (2019). https://doi.org/10.3846/aviation.2019.10320
Moses, A., Rutherford, M.J., Valavanis, K.P.: Radar-based detection and identification for miniature air vehicles. In: Proceedings of the IEEE International Conference on Control Applications, pp. 933–940 (2011). https://doi.org/10.1109/cca.2011.6044363
Itcia, E., Wasselin, J.-P., Mazuel, S., Otten, M., Huizing, A.: FMCW radar for the sense function of sense and avoid systems onboard UAVs. In: Emerging Technologies in Security and Defence; and Quantum Security II; and Unmanned Sensor Systems X, Oct. 2013, vol. 8899, p. 889914 (2013). https://doi.org/10.1117/12.2028518
Mathumo, T.W., Swart, T.G., Focke, R.W.: Implementation of a GNU radio and python FMCW radar toolkit. In: 2017 IEEE AFRICON: Science, Technology and Innovation for Africa, AFRICON 2017, Nov. 2017, pp. 585–590 (2017). https://doi.org/10.1109/afrcon.2017.8095547
Sundaresan, S., Anjana, C., Zacharia, T., Gandhiraj, R.: Real time implementation of FMCW radar for target detection using GNU radio and USRP. In: 2015 International Conference on Communication and Signal Processing, ICCSP 2015, Nov. 2015, pp. 1530–1534 (2015). https://doi.org/10.1109/iccsp.2015.7322772
Jiaxi zhu Norman, B.: Low-cost, Software Defined FMCW Radar for Observations of Drones (2017)
Fernandes, V.N.: Implementation of a RADAR System using MATLAB and the USRP. California State University—Northridge, 2012. http://oatd.org/oatd/record?record=handle%5C%3A10211.2%5C%2F1039 . Accessed 12 Nov. 2020
Sit, Y.L., Nuss, B., Basak, S., Orzol, M., Wiesbeck, W., Zwick, T.: Real-time 2D + velocity localization measurement of a simultaneous-transmit OFDM MIMO Radar using Software Defined Radios. In: 2016 13th European Radar Conference EuRAD 2016, no. May 2017, pp. 21–24 (2016)
Jankiraman, M.: Design of Multi-Frequency CW Radars. Institution of Engineering and Technology (2007)
Sandström, S.-E., Akeab, I.K.: A study of some FMCW radar algorithms for target location at low frequencies. Radio Sci. 51 (10), 1676–1685 (2016). https://doi.org/10.1002/2016RS005974
Kuschel, H.: VHF/UHF radar. Part 2: operational aspects and applications. Electron. Commun. Eng. J. 14 (3), 101–111 (2002). https://doi.org/10.1049/ecej:20020302
Aldowesh, A., Alnuaim, T., Alzogaiby, A: Slow-Moving Micro-UAV Detection with A Small Scale Digital Array Radar, Apr. 2019. https://doi.org/10.1109/radar.2019.8835567
An Overview of Antenna Systems in Millimeter-Wave Radar Applications—Microwave Product Digest (2020). https://www.mpdigest.com/2017/09/22/an-overview-of-antenna-systems-in-millimeter-wave-radar-applications/ . Accessed 12 Nov. 2020
Cooper, K.B., Chattopadhyay, G.: Submillimeter-wave radar. IEEE Microw. Mag. 15 (7), 51–67 (2014). https://doi.org/10.1109/MMM.2014.2356092
Smith, S.T.: Adaptive radar. In: Wiley Encyclopedia of Electrical and Electronics Engineering. Wiley (1999)
Greco, M.S., Gini, F., Stinco, P., Bell, K.: Cognitive radars: a reality?, Feb. 2018. http://arxiv.org/abs/1803.01000 . Accessed 12 Nov. 2020
Oechslin, R., Wellig, P., Hinrichsen, S., Wieland, S., Aulenbacher, U., Rech, K.: Cognitive radar parameter optimization in a congested spectrum environment. In: 2018 IEEE Radar Conference, RadarConf 2018, June 2018, pp. 218–223 (2018). https://doi.org/10.1109/radar.2018.8378560
Solomitckii, D., Gapeyenko, M., Semkin, V., Andreev, S., Koucheryavy, Y.: Technologies for efficient amateur drone detection in 5G Millimeter-Wave cellular infrastructure. IEEE Commun. Mag. 56 (1), 43–50 (2018). https://doi.org/10.1109/MCOM.2017.1700450
Zhao, J., Fu, X., Yang, Z., Xu, F., Rong, B.: Radar-Assisted UAV detection and identification based on 5G in the internet of things. Wirel. Commun. Mob. Comput. 2019 (2019). https://doi.org/10.1155/2019/2850263
Grossi, E., Lops, M., Venturino, L., Zappone, A.: Opportunistic Radar in IEEE 802.11ad Vehicular Networks, Nov. 2017, pp. 1–5 (2017). https://doi.org/10.1109/vtcspring.2017.8108446
Maggiora, R., Ruka, R.: Politecnico di Torino Implementation of a complete radar system on the NI USRP-2944R software dened radio platform Supervisor: Candidate
IHTAR Anti-Drone System|ASELSAN (2020). https://www.aselsan.com.tr/en/capabilities/air-and-missile-defense-systems/air-and-missile-defense-systems/ihtar-antidrone-system . Accessed 12 Nov. 2020
Tactical) Air Defense Radars—Belgian Advanced Technology Systems|Securing your homeland (2020). https://www.bats.be/solutions/radars/tactical-air-defense-radar . Accessed 12 Nov. 2020
AUDS Anti-UAV Defence System|Counter-UAS|C-UAS|Blighter (2020). https://www.blighter.com/products/auds-anti-uav-defence-system/ . Accessed 12 Nov. 2020
DroneShield Rolls Out Revolutionary Compact Radar|DroneShield (2020). https://www.droneshield.com/press-releases-content/2018/4/12/droneshield-rolls-out-revolutionary-compact-radar . Accessed 12 Nov. 2020
Home|IACIT (2020). https://www.iacit.com.br/en/ . Accessed 12 Nov. 2020
Introducing UWAS, the UAV Watch—Aveillant (2020). https://www.aveillant.com/introducing-uwas-the-uav-watch/ . Accessed 12 Nov. 2020
Security & Surveillance Radars|DeTect, Inc. (2020). https://detect-inc.com/security-surveillance-radars/ . Accessed 12 Nov. 2020
Products—Redefining Possible with Advanced Technologies and Systems|SRC, Inc (2020). https://www.srcinc.com/products/ . Accessed 12 Nov. 2020
Advanced Protection Systems Inc. (2020). https://apsystems.tech/ . Accessed 12 Nov. 2020
Pan, X., Xiang, C., Liu, S., Yan, S.: Low-Complexity time-domain ranging algorithm with FMCW sensors. Sensors 19 (14), 3176 (2019). https://doi.org/10.3390/s19143176
López Martínez, C., Vidal Morera, M.: Simulation of FMCW radar systems based on software defined radio (2016). https://upcommons.upc.edu/handle/2117/96865 . Accessed 12 Nov. 2020
Hassan, A., Gelman, I., Loftus, J.: Adversary UAV localization with software defined radio, Apr 2019. https://digitalcommons.wpi.edu/mqp-all/7115 . Accessed 12 Nov. 2020
Opromolla, R., Fasano, G., Rufino, G., Grassi, M., Savvaris, A.: LIDAR-inertial integration for UAV localization and mapping in complex environments. In: 2016 International Conference on Unmanned Aircraft Systems, ICUAS 2016, June 2016, pp. 649–656 (2016). https://doi.org/10.1109/icuas.2016.7502580
Peng, Z.: Development of portable radar systems for short-range localization and life tracking (2018). Accessed 12 Nov. 2020. https://ttu-ir.tdl.org/handle/2346/82111
Husodo, A.Y., Jati, G., Alfiany, N., Jatmiko, W.: Intruder drone localization based on 2D image and area expansion principle for supporting military defence system. In: 2019 IEEE International Conference on Communication, Networks and Satellite, Comnetsat 2019—Proceedings, Aug. 2019, pp. 35–40. https://doi.org/10.1109/comnetsat.2019.8844103
Ding, G., Wu, Q., Zhang, L., Lin, Y., Tsiftsis, T.A., Yao, Y.D.: An amateur drone surveillance system based on the cognitive internet of things. IEEE Commun. Mag. 56 (1), 29–35 (2018). https://doi.org/10.1109/MCOM.2017.1700452
Shinde, C., Lima, R., Das, K.: Multi-view geometry and deep learning based drone detection and localization. In: 2019 5th Indian Control Conference, ICC 2019—Proceedings, May 2019, pp. 289–294 (2019). https://doi.org/10.1109/indiancc.2019.8715593
Ritchie, M., Fioranelli, F., Griffiths, H., Torvik, B.: Micro-drone RCS analysis. In: 2015 IEEE Radar Conference—Proceedings, Oct. 2015, pp. 452–456 (2015). https://doi.org/10.1109/radarconf.2015.7411926
Seybert, A. et al.: Detect sense and avoid radar for UAV avionics telemetry item type text. In: Proceedings Detect Sense and Avoid Radar for Uav Avionics Telemetry (2020). http://hdl.handle.net/10150/595802 . Accessed 12 Nov. 2020
Simulink—Simulation and Model-Based Design—MATLAB & Simulink (2020). https://www.mathworks.com/products/simulink.html . Accessed 12 Nov. 2020
Parrish, K.: An Overview of FMCW Systems in MATLAB, Cerc.Utexas.Edu, no. July, pp. 1–7 (2015). http://www.cerc.utexas.edu/~kparrish/class/radar.pdf
About GNU Radio GNU Radio. https://www.gnuradio.org/about/ . Accessed 12 Nov. 2020
CST Studio Suite 3D EM simulation and analysis software (2020). https://www.3ds.com/products-services/simulia/products/cst-studio-suite/ . Accessed 12 Nov. 2020
FE Modeling and Visualization|Altair HyperWorks (2020). https://www.altair.com/hyperworks/ . Accessed 12 Nov. 2020
Wireless EM Propagation Software—Wireless InSite—Remcom (2020). https://www.remcom.com/wireless-insite-em-propagation-software/ . Accessed 12 Nov. 2020
About USRP Bandwidths and Sampling Rates—Ettus Knowledge Base (2020). https://kb.ettus.com/About_USRP_Bandwidths_and_Sampling_Rates . Accessed 12 Nov. 2020
Nuss, B., Sit, L., Fennel, M., Mayer, J., Mahler, T., Zwick, T.: MIMO OFDM radar system for drone detection, Aug. 2017. https://doi.org/10.23919/irs.2017.8008141
Technologies|Ancortek Inc (2020). https://ancortek.com/our-technologies . Accessed 12 Nov 2020
Jian, M., Lu, Z., Chen, V.C.: Drone detection and tracking based on phase-interferometric Doppler radar. In: 2018 IEEE Radar Conference, RadarConf 2018, June 2018, pp. 1146–1149. https://doi.org/10.1109/radar.2018.8378723
Syeda, R.Z., Adela, B.B., van Beurden, M.C., van Zeijl, P.T.M., Smolders, A.B.: Design of a mm-wave MIMO radar demonstrator with an array of FMCW radar chips with on-chip antennas 2019, pp. 33–36 (2020). https://research.tue.nl/en/publications/design-of-a-mm-wave-mimo-radar-demonstrator-with-an-array-of-fmcw . Accessed 12 Nov. 2020
Zhang, L., Wei, N., Du, X.: Waveform design for improved detection of extended targets in sea clutter. Sensors 19 (18), 3957 (2019). https://doi.org/10.3390/s19183957
Oechslin, R., Aulenbacher, U., Rech, K., Hinrichsen, S., Wieland, S., Wellig, P.: Cognitive radar experiments with codir. In: IET Conference Publications, vol. 2017, no. CP728 (2017). https://doi.org/10.1049/cp.2017.0386
Quilter, T., Baker, C.: The application of staring radar to the detection and identification of small Unmanned Aircraft Systems in Monaco, Aug. 2017. https://doi.org/10.23919/irs.2017.8008145
Ganti, S.R., Kim, Y.: Implementation of detection and tracking mechanism for small UAS. In: 2016 International Conference on Unmanned Aircraft Systems, ICUAS 2016, June 2016, pp. 1254–1260. https://doi.org/10.1109/icuas.2016.7502513
Poullin, D.: Countering illegal UAV flights: passive DVB radar potentiality. In: Proceedings International Radar Symposium, Aug.2018, vol. 2018-June. https://doi.org/10.23919/irs.2018.8447902
Download references
Acknowledgments
We would like to express our sincere gratitude to the General Civil Aviation Authority (GCAA) in the UAE for establishing the Aerospace Center of Excellence and conduct-ing this research study. We also thank our supervisors and colleagues from OpenUAE Research and Development Group at University of Sharjah, who provided insight and expertise that greatly assisted the research.
Author information
Authors and affiliations.
Department of Electrical Engineering, University of Sharjah, Sharjah, UAE
Sally M. Idhis, Takwa Dawdi, Qassim Nasir & Yara Omran
Department of Computer Science, University of Sharjah, Sharjah, UAE
Manar Abu Talib
You can also search for this author in PubMed Google Scholar
Corresponding author
Correspondence to Sally M. Idhis .
Editor information
Editors and affiliations.
Department of Information Engineering and Mathematics, University of Siena, Siena, Italy
Monica Bianchini
Department of Computer Science, University of Milan, Milan, Italy
Vincenzo Piuri
Regional Campus Manipur, Indira Gandhi National Tribal University, Imphal, Manipur, India
School of Electrical and Electronic Engineering, Galgotias University, Greater Noida, Uttar Pradesh, India
Rabindra Nath Shaw
Rights and permissions
Reprints and permissions
Copyright information
© 2022 The Author(s), under exclusive license to Springer Nature Singapore Pte Ltd.
About this paper
Cite this paper.
Idhis, S.M., Dawdi, T., Nasir, Q., Talib, M.A., Omran, Y. (2022). Detection and Localization of Unmanned Aerial Vehicles Based on Radar Technology. In: Bianchini, M., Piuri, V., Das, S., Shaw, R.N. (eds) Advanced Computing and Intelligent Technologies. Lecture Notes in Networks and Systems, vol 218. Springer, Singapore. https://doi.org/10.1007/978-981-16-2164-2_34
Download citation
DOI : https://doi.org/10.1007/978-981-16-2164-2_34
Published : 22 July 2021
Publisher Name : Springer, Singapore
Print ISBN : 978-981-16-2163-5
Online ISBN : 978-981-16-2164-2
eBook Packages : Intelligent Technologies and Robotics Intelligent Technologies and Robotics (R0)
Share this paper
Anyone you share the following link with will be able to read this content:
Sorry, a shareable link is not currently available for this article.
Provided by the Springer Nature SharedIt content-sharing initiative
- Publish with us
Policies and ethics
- Find a journal
- Track your research
Thank you for visiting nature.com. You are using a browser version with limited support for CSS. To obtain the best experience, we recommend you use a more up to date browser (or turn off compatibility mode in Internet Explorer). In the meantime, to ensure continued support, we are displaying the site without styles and JavaScript.
- View all journals
- Explore content
- About the journal
- Publish with us
- Sign up for alerts
- Open access
- Published: 01 August 2024
MPE-YOLO: enhanced small target detection in aerial imaging
- Yichang Qin 1 ,
- Ze Jia 1 &
- Ben Liang 1
Scientific Reports volume 14 , Article number: 17799 ( 2024 ) Cite this article
470 Accesses
Metrics details
- Aerospace engineering
- Electrical and electronic engineering
Aerial image target detection is essential for urban planning, traffic monitoring, and disaster assessment. However, existing detection algorithms struggle with small target recognition and accuracy in complex environments. To address this issue, this paper proposes an improved model based on YOLOv8, named MPE-YOLO. Initially, a multilevel feature integrator (MFI) module is employed to enhance the representation of small target features, which meticulously moderates information loss during the feature fusion process. For the backbone network of the model, a perception enhancement convolution (PEC) module is introduced to replace traditional convolutional layers, thereby expanding the network’s fine-grained feature processing capability. Furthermore, an enhanced scope-C2f (ES-C2f) module is designed, utilizing channel expansion and stacking of multiscale convolutional kernels to enhance the network’s ability to capture small target details. After a series of experiments on the VisDrone, RSOD, and AI-TOD datasets, the model has not only demonstrated superior performance in aerial image detection tasks compared to existing advanced algorithms but also achieved a lightweight model structure. The experimental results demonstrate the potential of MPE-YOLO in enhancing the accuracy and operational efficiency of aerial target detection. Code will be available online (https://github.com/zhanderen/MPE-YOLO).
Similar content being viewed by others

Improved GBS-YOLOv5 algorithm based on YOLOv5 applied to UAV intelligent traffic
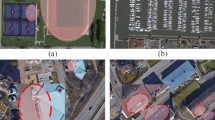
Centralised visual processing center for remote sensing target detection
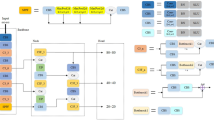
Lightweight aerial image object detection algorithm based on improved YOLOv5s
Introduction.
Aerial images, acquired through aerial photography technology, feature high-resolution and extensive area coverage, providing critical support to fields such as traffic monitoring 1 and disaster relief 2 through the automated extraction and analysis of geographic information. With continuous advancements in remote sensing technology, aerial image detection offers valuable data support for geographic information systems and related applications, playing a significant role in enhancing the identification and monitoring of surface objects and the development of geographic information technology.
Aerial images are characterized by complex terrain, varying light conditions, and difficulties in data acquisition and storage. However, the high-dimensionality and massive volume of aerial image data pose numerous challenges to image detection, particularly because aerial images often contain small targets, making detection even more challenging 3 . In light of these issues, target detection algorithms are increasingly vital as the core technology for aerial image analysis.
Traditional object detection algorithms often rely on manually designed feature extraction methods such as scale-invariant feature transform (SIFT), and speeded up robust feature (SURF). These methods represent targets by extracting local features from images but might fail to capture higher-level semantic information. Machine learning approaches such as support vector machines (SVMs) 4 , random forests 5 , etc., have effectively improved the accuracy and efficiency of aerial detection, but struggle with the detection of complex backgrounds. With the rapid development of deep learning technology, neural network-based image object detection methods have become mainstream. The end-to-end learning capability of deep learning allows algorithms to automatically learn and extract more abstract and higher-level semantic features, replacing traditionally manually designed features.
Deep learning-based object detection algorithms can be divided into single-stage and two-stage algorithms. The two-stage algorithms are represented by the R-CNN 6 , 7 , 8 series, which adopts a two-stage detection process; First candidate regions are created via the region proposal network (RPN), and then the location and classification are fine-tuned through classifiers and regressors. Such algorithms can precisely locate and identify various complex land objects, especially when dealing with small or densely arranged targets, and have received widespread attention and application. However, two-stage detection algorithms still have room for improvement in terms of speed and efficiency. Single-stage detection algorithms, represented by SSD 9 and YOLO 10 , 11 , 12 , 13 , 14 , 15 , 16 , 17 series, approach object detection as a regression problem and predict the categories and locations of targets directly from the global image, enabling real-time detection. These algorithms offer good real-time performance and accuracy, and are particularly suitable for processing large-scale aerial image data. They hold significant application prospects for quickly obtaining geographic information, monitoring urban changes, and natural disasters. However, single-stage object detection algorithms still face challenges in the accurate detection and positioning of small targets.
In the context of UAV aerial imagery, object detection encounters several specific challenges:
Dense small objects and occlusion Images captured from low altitudes often contain a large number of dense small objects, particularly in urban or complex terrains. Due to the considerable distance, these objects appear smaller in the images and are prone to occlusion. For instance, buildings might obscure each other, or trees might cover parked vehicles. Such occlusion leads to partial hiding of target object features, thereby affecting the performance of detection algorithms. Even advanced detection algorithms struggle to accurately identify and locate all objects in highly dense and severely occluded environments.
Real-time requirements vs. accuracy trade-off UAV aerial image object detection must meet real-time requirements, particularly in monitoring and emergency response scenarios. Achieving real-time detection necessitates a reduction in algorithmic computational complexity, which frequently conflicts with detection accuracy. High-accuracy detection algorithms typically require substantial computational resources and time, whereas real-time demands necessitate algorithms that can process vast amounts of data swiftly. The challenge lies in maintaining high detection accuracy while ensuring real-time performance. This requires optimization in network architecture to balance the number of parameters and accuracy effectively.
Complex backgrounds Aerial images often include a significant amount of irrelevant background information like buildings, trees, and roads. The complexity and diversity of background information can interfere with the correct detection of small objects. Moreover, the features of small objects are inherently less pronounced. Traditional single-stage and two-stage algorithms primarily focus on global features and may overlook the fine-grained features crucial for detecting small objects. These algorithms often fail to capture the details of small objects, resulting in lower detection accuracy. Therefore, there is a pressing need for more advanced deep learning models and algorithms that can handle these subtle features, thereby enhancing the accuracy of small object detection.
To address the aforementioned issues, this study proposes an algorithm called MPE-YOLO, which is based on the YOLOv8 model, and enhances the detection accuracy of small objects while maintaining a lightweight model. The main contributions of this study are as follows.
We developed a multilevel feature integrator (MFI) module with a hierarchical structure to merge image features at different levels, enhancing scene comprehension and boosting object detection accuracy.
A perception enhancement convolution (PEC) module is proposed, which uses multislice operations and channel dimension concatenation to expand the receptive field, thereby improving the model’s ability to capture detailed target information.
By incorporating the proposed enhanced scope-C2f (ES-C2f) operation and introducing an efficient feature selection and utilization mechanism, the selective use of features is further enhanced, effectively improving the accuracy and robustness of small object detection.
After comprehensive comparative experiments with various other object detection models, MPE-YOLO has demonstrated a significant improvement in performance , proving its effectiveness.
The rest of this paper includes the following content: Section 2 briefly introduces the recent research results on aerial image detection and the main idea of YOLOv8. Section 3 introduces the innovations of this paper. Section 4 describes the experimental setup, including the experimental environment, parameter configuration, datasets used, and performance evaluation metrics, and presents detailed experimental steps and results, verifying the effectiveness of the improvement strategies. Section 5 summarizes the main contributions of this research and discusses future directions of work.
Background and related works
Related works.
Deep learning-based object detection algorithms are widely applied in fields such as aerial image detection, medical image processing, precision agriculture, and robotics due to their high detection accuracy and inference speed. The following are some algorithms used in aerial image detection: Cheng et al. 18 proposed a method combining cross-scale feature fusion to enhance the network’s ability to distinguish similar objects in aerial images. Guo et al. 19 presented a novel object detection algorithm that improves the accuracy and efficiency of highway intrusion detection by refining feature extraction, feature fusion, and computational complexity methods. Sahin et al. 20 introduced YOLODrone, an improved version of the YOLOv3 algorithm that increases the number of detection layers to enhance the model’s capability to detect objects of various sizes, although this adds to the model’s complexity. Chen et al. 21 enhanced the feature extraction capability of the model by optimizing residual blocks in the multi-level local structure of DW-YOLO and improved accuracy by increasing the number of convolution kernels. Zhu et al. 22 incorporated the CBAM attention mechanism into the YOLOv5 model to address the issue of blurred objects in aerial images. Additionally, Yang 23 enhanced small object detection capability by adding upsampling in the neck part of the YOLOv5 network. And integrated an image segmentation layer into the detection network. Lin et al. 24 proposed GDRS-YOLO, which first constructs multi-scale features through deformable convolution and gathering-dispersing mechanisms, and then introduces normalized Wasserstein distance for mixed loss training, effectively improving the accuracy of object detection in remote sensing images. Jin et al. 25 improved the robustness and generalization of UAV image detection under different shooting conditions by decomposing domain-invariant features, domain-specific features, and using balanced sampling data augmentation techniques. Bai et al.’s CCNet 26 suppresses interference in deep feature maps using high-level RGB feature maps while achieving cross-modality interaction, enhancing salient object detection.
In the field of medical image processing, typical object detection algorithms include: Pacal et al. 27 demonstrated that by improving the YOLO algorithm and using the latest data augmentation and transfer learning techniques, the efficiency and accuracy of polyp detection could be significantly enhanced. Xu et al. 28 showed that the improved Faster R-CNN model exhibited excellent performance in lung nodule detection, particularly in small object detection capability and overall detection accuracy.Xi et al. 29 improved the sensitivity of small object detection by introducing a super-resolution reconstruction branch and an attention fusion module in the MSP-YOLO network. In the agricultural field, Zhu et al. 30 demonstrated how to achieve high-precision drone control systems through a combination of hardware and software. Its application in agricultural spraying provides a reference for the performance of automated control systems in practical applications. In the field of robotics, Wang et al. 31 researched robotic mechanical models and optimized jumping behavior through bionic methods. This combination of biological observation and mechanical modeling can inspire the development of other robots or systems that require motion optimization, using bionic mechanisms to achieve efficient and reliable motion control.
The aforementioned methods face challenges such as the limitations of the receptive field and insufficient feature fusion in highly complex backgrounds or dense small object scenes, resulting in poor performance in low-resolution and densely occluded situations. Driven by these motivations, we propose an algorithm called MPE-YOLO that improves the detection accuracy of small objects while maintaining a lightweight model. Numerous experiments have demonstrated that by integrating multilevel features and strengthening detail information perception modules, we can achieve higher detection accuracy across different datasets.
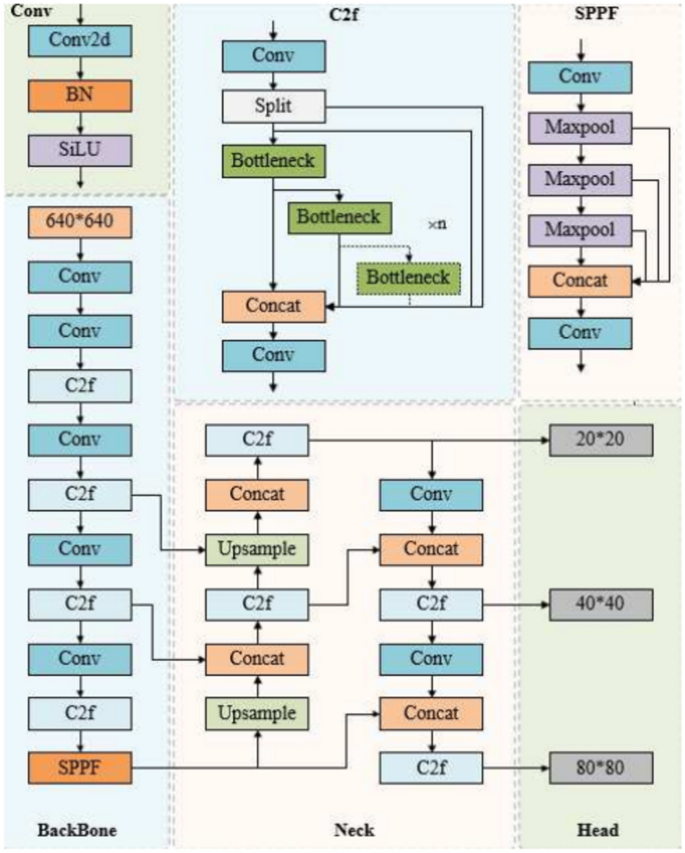
YOLOv8 network structure.
YOLOv8 is the latest generation of object detection algorithms developed by Ultralytics, and officially released on January 10, 2023. YOLOv8 improves upon YOLOv5 by replacing the C3 module with the C2f module. The head utilizes a contemporary decoupled head structure, separating classification and detection heads, and transitions from an anchor-based to an anchor-free approach, resulting in higher detection accuracy and speed. The YOLOv8 model comprises an input layer, a backbone network, a neck network, and a head network, as shown in Fig. 1 . The input image is first resized to 640x640 to meet the size requirements of the input layer, and the backbone network achieves downsampling and feature extraction via multiple convolutional operations, with each convolutional layer equipped with batch normalization and SiLU 32 activation functions. To improve the network’s gradient flow and feature extraction capacity, the C2f block was introduced, drawing on the E-ELAN structure from YOLOv7, and employing multilayer branch connections. Furthermore, the SPPF 33 block is positioned at the end of the backbone network and combines multiscale feature processing to enhance the feature abstraction capability. The neck network adopts the FPN 34 and PAN 35 structures for effective fusion of different scale feature maps, which are then passed on to the head network. The head network is designed in a decoupled manner, including two parallel convolutional branches that handle regression and classification tasks separately to improve focus and performance on each task. The YOLOv8 series offers five different scaled models for users to choose from, including YOLOv8n, YOLOv8s, YOLOv8m, YOLOv8l, and YOLOv8x. Compared to other models, YOLOv8s strikes a balance between accuracy and model complexity. Therefore, this study chooses YOLOv8s as the baseline network.
Methodology
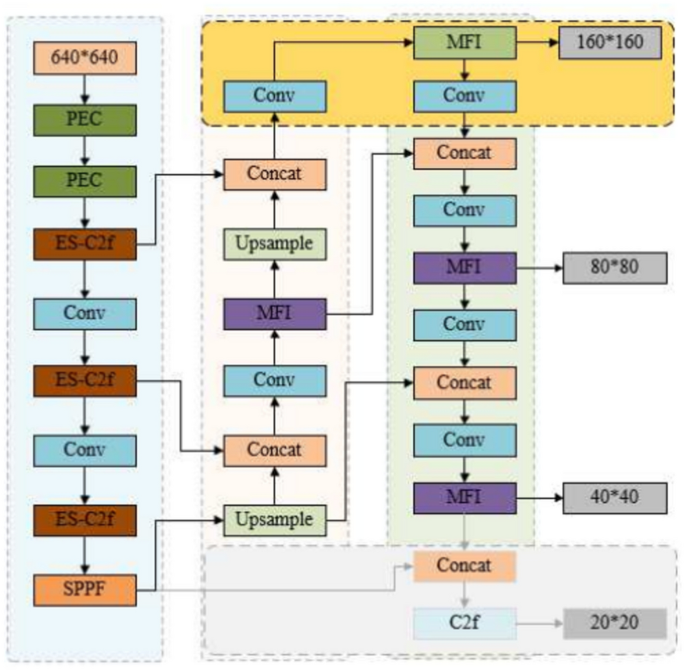
MPE-YOLO network structure.
In response to the need for detecting small objects in aerial and drone imagery, we propose the MPE-YOLO algorithm to adjust the structure of the original YOLOv8 components. As shown in Fig. 2 , by designing the multilevel feature integrator (MFI) module, the representation and information fusion of small target features are optimized, so as to reduce the information loss in the process of feature fusion. The introduction of the perception enhancement convolution (PEC) module replaces the traditional convolutional layer, expands the ability of fine-grained feature processing of the network, and significantly improves the recognition accuracy of small targets in complex backgrounds. We replaced the last two downsampling layers and the detection layer for 20*20 size targets in the backbone network with a detection layer for small 160*160 size targets. This enables the model to focus more on the details of small targets. Finally, through the enhanced scope-C2f (ES-C2f) module, the feature extraction efficiency and operation efficiency of the model are further improved by using channel expansion and the stacking of multi-scale convolution kernels. Combining these improvements, MPE-YOLO performs well in small object detection tasks in complex environments, and significantly improves the accuracy and performance of the model. To differentiate from the baseline model, MPE-YOLO marks the improved modules with darker colors. The gray area at the bottom represents the removal of the 20*20 detection head, while the yellow area at the top represents the addition of the 160*160 detection head.
Multilevel feature integrator
In object detection tasks, the feature representation of small objects is often unclear due to size restrictions, which can lead to them being overlooked or lost in the feature fusion process, resulting in decreased detection performance. To effectively address this issue, we adopted the structure of Res2Net 36 and designed an innovative multilevel feature integrator (MFI). The structure of the MFI module, as shown in Fig. 3 , aims to optimize the feature representation and information fusion of small objects through a series of detailed strategies, reducing the loss of feature information and suppressing redundancy and noise.
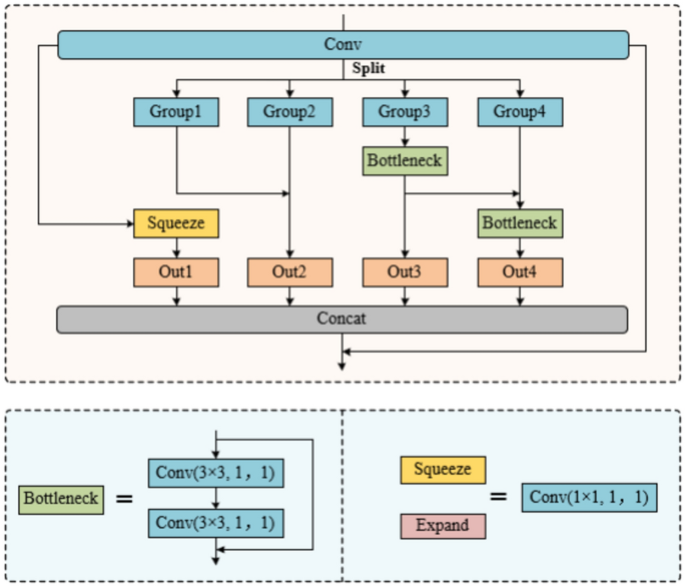
Multilevel feature integrator structure.
First, the MFI module uses convolutional operations to reduce the channel dimensions of the input feature maps, simplifying the subsequent computation process. Immediately following, the reduced feature maps are uniformly divided into four groups (Group 1 to Group 4), with each group containing 25% of the total number of original feature maps. This partition is not random, but a uniform segmentation of the number of channels based on the feature map, aiming to optimize the computational efficiency and the subsequent feature fusion effect. We use a squeeze convolution layer to shape and compress the feature maps from all groups, resulting in output Out1, which aims to focus on key target features, reduce feature redundancy, and preserve details helpful for small object detection. Second, by performing proportional feature fusion of Group 1 and Group 2, we construct complex low-level feature representations, forming the output part Out2, and enhancing the feature details of small objects. Additionally, the bottleneck module 17 is applied to Group 3 to refine high-level semantic information, and produce Out3. This advanced feature output helps capture richer contextual information, improving the detection efficiency of small objects.
Out4 is obtained by fusing the high-level features from Out3 with the Group4 features and then processing them again through the bottleneck module. The purpose of this step is to integrate the low-level features with the high-level features, enabling the model to understand the characteristics of small objects more comprehensively. Then by concatenating and integrating the four different levels of outputs-Out1, Out2, Out3, and Out4-in the channel direction, the features of all the scales are fully utilized, thereby improving the overall performance of the model in small object detection tasks.
Ultimately, MFI module adopts a channel-wise feature integration approach to aggregate features from various levels, enhancing the ability to recognize different target behaviors, particularly improving the accuracy of capturing small object behaviors and interactions in dynamic scenes.
Perception enhancement convolution
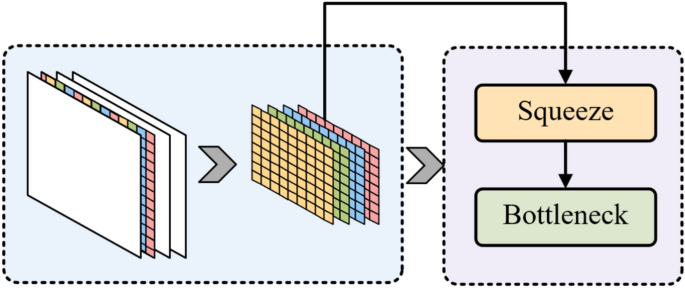
Perception enhancement convolution structure.
When dealing with multiscale object detection tasks, traditional convolutional neural networks typically face challenges such as fixed receptive fields 37 , insufficient use of context information, and limited environmental perception. In particular, in the detection of small objects, these limitations can significantly suppress the performance of the model. To overcome these issues, we introduce Perception-Enhanced Convolution (PEC), as shown in Fig. 4 , which is a module specifically designed for the backbone network and intended to replace traditional convolutional layers. The main advantage of PEC is that it introduces a new dimension during the phase of extracting primary features in the model, which can significantly expand the receptive field and more effectively integrate context information, thus further deepening the model’s understanding of small objects and their environment.
In detail, the PEC module begins by precisely cutting the input feature map into four smaller feature map blocks, each of which is reduced in size by half in the spatial dimension. This cutting process involves the selection of specific pixels, ensuring that representative information from the top-left, top-right, bottom-left, and bottom-right of the original feature map is captured separately in each channel. Through such a meticulous division of the spatial dimension, the resulting small blocks retain important spatial information while ensuring even coverage of information. Subsequently, these small blocks are concatenated in the channel dimension to form a new feature map, with an increased number of channels but reduced spatial resolution, thus significantly reducing the computational burden while maintaining a large receptive field.
To further enhance feature expressiveness and computational efficiency, a squeeze layer is integrated into the PEC, which reduces model parameters by compressing feature dimensions while ensuring that key features are emphasized even as the model is simplified. For deeper feature extraction, we apply the classic bottleneck structure, which not only refines the hierarchical representation of features but also significantly enhances the model’s sensitivity and cognitive ability for small objects, further boosting the computational efficiency of features.
Overall, through the PEC module, the model is endowed with stronger environmental adaptability and understanding of object relations. The innovative design of the PEC enables feature maps to obtain more comprehensive and detailed information on targets and the environment while expanding the receptive field. This is particularly crucial in areas such as traffic monitoring for object classification and behavior prediction, as these aeras greatly depend on accurate interpretations of subtle changes and complex scenes.
Enhanced Scope-C2f
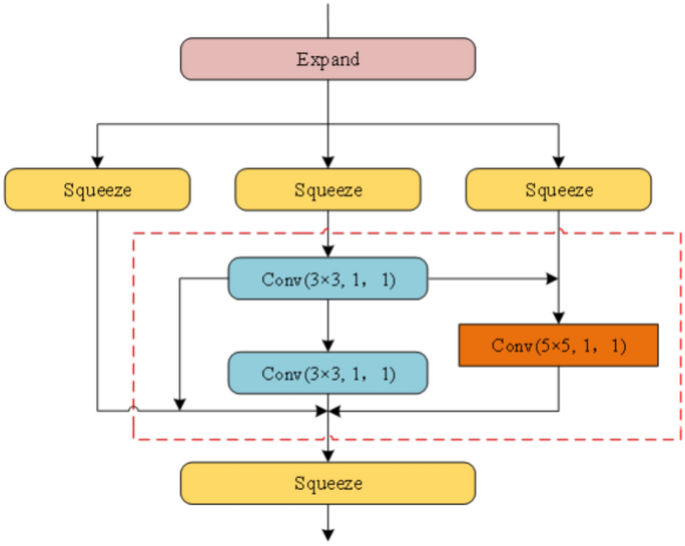
Enhanced Scope-C2f structure.
In the YOLOv8 model, researchers designed the C2f module 17 to maintain a lightweight network while obtaining richer gradient flow information. However, when dealing with small targets or low-contrast targets in aerial images, this module does not sufficiently express fine features, affecting the detection accuracy of targets with complex scales. To address this issue, this study proposes an improved module called Enhanced Scope-C2f (ES-C2f), as shown in Fig. 5 , which focuses on improving the network’s ability to capture details and feature utilization efficiency, especially in expressing small targets and low-contrast targets.
The ES-C2f module enhances the network’s representation capability for targets by expanding the channel capacity of feature maps, enabling the model to capture more subtle feature variations. This strategy is dedicated to enhancing the network’s sensitivity to small target details and improving the adaptability to low-contrast target environments through a wider range of feature representations.
To expand the channel capacity while considering computational efficiency, the ES-C2f module cleverly integrates a series of squeeze layers. These layers perform intelligent selection and compression of feature channels, not only streamlining feature representations but also preserving the capture of key information. The design of this feature operation fully considers the need to enhance identification capabilities while reducing model complexity and computational load. ES-C2f further employs a strategy of stacking multiscale convolutional kernels as well as combining local and global features. This provides an effective means to integrate features at different levels, enabling the model to make decisions on a richer feature dimension. Deep semantic information is cleverly woven with shallow texture details, enhancing the perception of scale diversity.
An optimized squeeze layer is introduced at the end of the module to further refine the essence of the features and adapt to the needs of subsequent processing layers. This engineering not only enhances the feature representation capacity but also improves the information decoding efficiency of subsequent layers, allowing the model to detect and recognize targets with greater precision. With the improvements made to the original C2f module in the YOLOv8 architecture, the proposed ES-C2f module provides a more effective solution for small targets and low-contrast scenes. The ES-C2f module not only maintains the lightweight structure and response speed of the model in extremely challenging scenarios but also significantly improves the overall recognition ability for complex-scale target detection.
Experiments
Experimental setup.
The batch size was set to 4 to avoid memory overflow, the learning rate was set to 0.01, the learning rate was adjusted by the cosine annealing algorithm, the momentum of the stochastic gradient descent (SGD) was set to 0.937, and the mosaic method was used for data augmentation. The resolution of the input graphics is uniformly set to 640 \(\times \) 640. A total of 200 epochs were trained on all models, and no pretrained models were used in training to ensure the fairness of the experiment. We opted for random weight initialization, ensuring that the initial weights of each model originate from the same distribution. Although the specific initial values differ, this guarantees that all models start from a fair and balanced point, enabling comparison under identical training conditions without the influence of historical biases from pretrained models. Pretrained models are typically trained on large datasets that may not align with our target dataset distribution, potentially introducing unforeseen biases. Therefore, we decided against using pretrained models. To mitigate the impact of randomness in weight initialization, we conducted multiple independent experiments and averaged the results. Table 1 lists the training environment configurations.
To ensure the rationality of the experimental data, this article selected three representative public datasets for experiments, namely VisDrone2019 38 , RSOD 39 , and AI-TOD 40 . VisDrone2019, as the main dataset of this experiment, was subjected to very detailed comparative and ablation studies. To validate the generalizability and universality of the model, experiments were conducted on the RSOD and AI-TOD datasets.
Considering the consistency of the dataset and the continuity of the study, we selected the VisDrone2019 dataset, it collected and released by Tianjin University’s Machine Learning and Data Mining Lab, comprises a total of 8629 images. Among them, 6471 images were used for training, 548 images were used for validation, and 1610 images were used for testing. The dataset encompasses 10 categories from daily scenes-pedestrian, person, bicycle, car, van, truck, tricycle, awning tricycle, bus, and motorcycle. In this dataset, the proportion of categories is unbalanced, and most images contain small targets, making detection difficult.
The RSOD dataset is a public dataset released by Wuhan University in 2017, it consists of 976 optical remote sensing images taken from Google Earth and Tianditu, and is composed of four object classes: aircraft, oiltank, overpass, and playground, totalling 6950 targets. To increase the number of samples, the dataset was expanded by means of rotation, translation, and splicing, increasing the total to 2000 images. To avoid data leakage issues, data augmentation is performed only on the training set, and the validation and test sets remain in their original state. Then randomly split it into training, validation, and test sets at a ratio of 8:1:1, with the training set comprising 1,600 images and both the validation and test sets containing 200 images each.
The AI-TOD dataset is a specialized remote sensing image dataset focused on tiny objects, consisting of 28,036 images and 700,621 targets. These targets are divided into eight categories: bridge, ship, vehicle, storage-tank, person, swimming-pool, wind-mill, and airplane. Compared to other aerial remote sensing datasets, the average size of targets in AI-TOD is approximately 12.8 pixels, which is significantly smaller than that in other datasets, increasing the difficulty of detection. The dataset is divided into training, validation, and test sets at a ratio of 6:1:3.
Evaluation criteria
We selected mAP0.5, mAP0.5:0.95, and APs as indicators to measure the model’s accuracy in small target detection. To evaluate the model’s efficiency, we used the number of parameters and model size as indicators of its lightweight nature. Additionally, latency was chosen to assess the model’s real-time detection performance.
Precision is the ratio of the number of samples correctly predicted as positive to the number of all samples predicted as positive. The formula is as follows:
Recall is the ratio of the number of samples correctly predicted as positive to the number of samples of all true cases. The formula is as follows:
TP (true positives) represents the number of correctly identified positive instances, FP (false positives) represents the number of incorrectly identified negative instances as positive, and FN (false negatives) represents the number of incorrectly identified positive instances as negative.
mAP refers to the average AP of all defect categories, AP refers to the area of the curve below the precision recall curve, and the formula for AP and mAP is as follows, the greater the mAP is, the better the comprehensive detection performance of the model in all categories, the specific formula is as follows:
The APs metric is the average accuracy of calculating the detection results of small objects, and this metric can help us understand how well the model performs when detecting small objects. The number of parameters represents the number of the parameters used by the model, measured in millions. The number of parameters provides a direct indicator of the complexity of the model, a greater number of parameters usually means greater representation power, but can likewise lead to longer training times and the risk of overfitting. Model size usually refers to the size of the model file stored on disk and is usually quantified in megabytes (MB). Model size reflects the amount of storage space the model occupies, which is especially important in resource-constrained environments such as mobile devices or where the model needs to be deployed to embedded devices. Latency refers to the time it takes to process a frame in object detection, and is one of the metrics to measure whether a model can meet real-time detection.
Ablation atudy
To validate the effectiveness of the proposed module in aerial image detection, we conducted ablation studies for each module, using the YOLOv8s model as the baseline. The experimental results are shown in Table 2 , where ✓ indicates the addition of the module to the model, A represents adding the MFI module, B represents improving the network structure, C represents adding the PEC module, and D represents adding the ES-C2f module.
By incorporating the multilevel feature integrator (MFI) module, experiments demonstrate a notable enhancement in small object detection performance, notably reflected in a 1.6% increase in mean average precision ([email protected]) and a 0.9% increase in [email protected]:0.95. Simultaneously, the total number of model parameters is reduced by 0.8 million, and the model size decreases by 1.6 megabytes. Additionally, it reduces latency to 8.5 milliseconds, indicating that the MFI module has optimized the model’s computational efficiency and feature extraction capabilities, particularly in integrating multi-level semantic information and reducing redundant calculations.
By optimizing the network structure, removing redundant deep feature mappings, and introducing detection heads optimized for small object detection, the precision of the model is significantly enhanced, as is the model’s ability to capture low-frequency detail information. These changes resulted in an improvement of 1.8% in mAP0.5 and 1.3% in mAP0.5:0.95. By compressing the number of channels and reducing the number of network layers, the model can abstractly extract semantic information from deeper feature maps, further enhancing the recognition of small objects. The simplification of the structure not only reduced the parameter count by 7.2 M but also reduced the model size to 6.3 MB. However, an increase in latency to 12ms suggests that the addition of a specific small object detection head has led to an increase in latency.
Subsequently, by introducing the PEC module, the feature maps are finely sliced and fused along the channel dimension, enhancing the spatial integrity and richness of the features. At the same time, with the introduction of squeeze layers, we compress key information while reducing computational complexity, thus improving the efficiency of feature processing. By using the bottleneck structure for deep feature processing, the small object detection and processing capabilities of the module are enhanced, and the complexity of the model increases only slightly compared to that of the baseline model, maintaining the latency at 12.5 ms, resulting in a 1.2% improvement in the mAP0.5 and a 0.7% improvement in the mAP0.5:0.95. This result shows that even with a slight increase in complexity, the PEC module achieves a significant improvement in the accuracy of small object detection, especially in complex scenarios, where the model’s performance has been effectively improved.
Finally, by integrating the ES-C2f module, the model can combine the advantages of \(3 \times 3\) and \(5 \times 5\) convolutional kernels to capture local detail features of the target more efficiently than the traditional C2f module while integrating a wider range of contextual information. This module not only improves computational efficiency but also enhances the model’s representational capacity through internal feature channel transformation and information compression. This allows the model to more comprehensively analyze the image content and accurately capture the details of small objects. As a result, the model’s mAP0.5 and mAP0.5:0.95 increased by approximately 1.1% and 0.6%, respectively, while the number of parameters and the model size were reduced by 6.7 M and 12.7 MB compared to the baseline, and then seeing an increase to 14 ms, still ensures a reasonable latency time.
These results validate our improvement strategy, which effectively enhances the accuracy of target detection in aerial images while ensuring that the model is lightweight, demonstrating the profound significance of the research.
Compared with the baseline model, MPE-YOLO shows a significant improvement in the detection accuracy of all categories. As shown in Table 3 , the accuracy of both the pedestrian and people categories is improved by more than 8 points, which indicates that the MPE-YOLO model has a strong detail capture ability for small-scale targets. Overall, the average accuracy of the MPE-YOLO model (mAP0.5) reached 37.0%, which is nearly 6% higher than that of YOLOv8, proving the effectiveness of MPE-YOLO.
Comparative experiments
To validate the effectiveness of the model. we selected the most popular object detection algorithms to compare with MPE-YOLO, including YOLOv5, YOLOv6, YOLOv7, YOLOv8, YOLOX 41 , RT-DETR 42 , and Gold-YOLO 43 ,ASF-YOLO 44 , two of the recent research results, as shown in Table 4 .
The test results on the VisDrone2019 dataset show the differences in the performances of different object detection algorithms. First, we observed that the performances of the most classical YOLOv5s model was 26.8% on mAP0.5 and 7.0% on APs for small target detection. This result reflects the challenges of the basic YOLO model for small target detection on aerial image datasets. In comparison, YOLOv6s performed slightly worse, with mAP0.5 at 26.6% and APs at 6.7%, but despite this, the performances of the two methods were not very different. The model size and the number of parameters significantly differ, with the model size of YOLOv6s being nearly three times larger than that of YOLOv5s, and the number of parameters being more than doubled. YOLOX-s increased mAP0.5 to 29.5% and APs to 8.8%, indicating a significant improvement in the detection effect. However, this improvement comes at the cost of an increased model size (50.4 MB) and a larger number of parameters (8.9 M).
We then analyzed more advanced models - YOLOv8s and YOLOv8m. The YOLOv8s model achieves 31.3% on mAP0.5 and 8.2% APs, indicating that structural optimization has led to significant improvements. The YOLOv8m model achieves 35.4% and 9.8% on mAP0.5 and APs, respectively, which further proves that larger models may have better accuracy, especially for the more complex task of small object detection.
The RT-DETR-R18 model has a high score (35.9% vs. 10.2%) on both mAP0.5 and APs compared to the traditional architecture of the YOLO series, and it uses the DETR architecture, indicating the potential of the attention mechanism for more accurate object detection, and its model size and number of parameters are also lower than YOLOv8m.
To further validate the superiority of the MPE-YOLO model, we included two advanced models from existing literature, Gold-YOLO and X-YOLO, for comparative experiments. The experimental results show that Gold-YOLO achieved mAP0.5 and APs of 33.2 % and 9.5% respectively, with a model size of 26.3 MB and 13.4 million parameters. X-YOLO achieved mAP0.5 and APs of 34.0% and 9.6% respectively, with a model size of 22.8 MB and 11.3 million parameters. Both models showed significant improvements in performance and small object detection compared to the early YOLO series.
In the end, the MPE-YOLO model achieved the highest mAP0.5 of 37.0% and APs of 10.8%, while maintaining a model size of only 11.5 MB and 4.4 million parameters. This demonstrates that MPE-YOLO not only outperforms other current models in terms of performance but also achieves low resource consumption through its lightweight design, making it highly practical and attractive for real-world applications.
Visual analytics
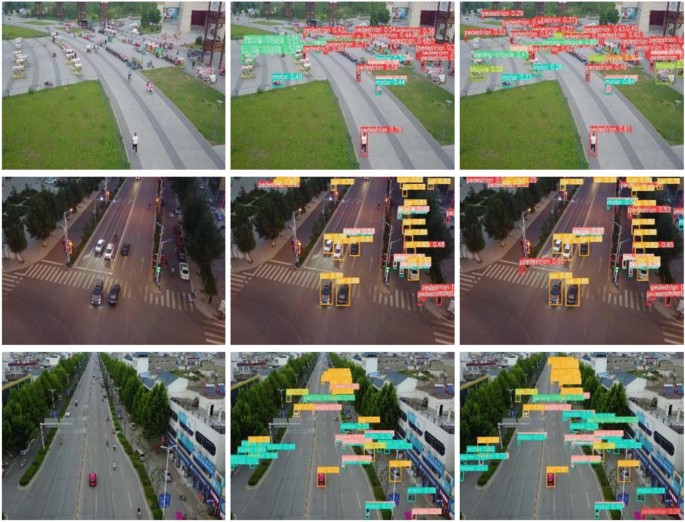
Comparison of YOLOv8(mid)and MPE-YOLO(right)on the RSOD VisDrone dataset.
By carefully selecting image samples, we applied the baseline model and the MPE-YOLO model for object detection. This allowed us to compare and analyze the detection performances of the two models. As shown in Fig. 6 , the detection confidence of the MPE-YOLO model is significantly better than that of the baseline model under multiple scenarios and challenging conditions. This is manifested in the fact that the target bounding boxes it identifies have higher confidence scores, and these scores are more consistent with the actual target. More importantly, MPE-YOLO also shows significant improvements in reducing false positives and false negatives, accurately identifying and identifying most targets, while minimizing misidentification of non-target areas. Moreover, even under suboptimal shading or lighting conditions, MPE-YOLO achieved a low missed detection rate. These comparison results highlight the effectiveness of the enhanced feature extraction network in MPE-YOLO in dealing with overlapping, size changes and complex backgrounds between targets, indicating that it has more robust feature learning and more accurate target prediction capabilities.
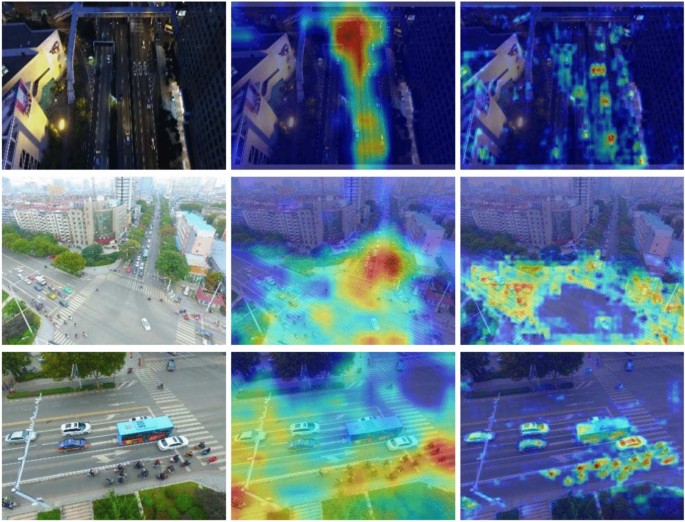
In Fig. 7 , the improved MPE-YOLO model demonstrates its superior feature extraction and targeting capabilities. This is evident by the more concentrated and reinforced high-response regions it reflects. This feature is presented as a brighter area on the heat map, closely following the actual position and contour of the target, demonstrating that the MPE-YOLO model can effectively focus on important signals. In addition, compared with the baseline model, the heat map generated by the improved model shows fewer scattered hot spots around the target, which reduces the possibility of false detection and false alarms, demonstrating the precision and robustness of MPE-YOLO in small target detection tasks. First, the heat map of the night scene in the first row reveals the recognition ability of MPE-YOLO under low-light conditions, in which areas with strong brightness are accurately mapped to the target location, indicating that the model still has efficient feature capture capabilities at low lighting levels. Then, in the second row, when faced with a complex background scene, the heat map generated by MPE-YOLO maintained the ability to accurately identify the target without being affected by the complex environment. The model’s clear positioning of the target verifies its effectiveness in distinguishing the target from the cluttered background in the actual environment. Finally, in the case of dense small targets in the third row, the MPE-YOLO heat map shows excellent discrimination, even when the targets are very close to each other. The highlights of the heat map correspond densely and distinctly to the contours of each small target, showing the model’s ability to accurately locate multiple targets.
These visual evidences are consistent with the increase in mAP0.5 and mAP0.5:0.95 in the experiment, which provides intuitive and strong support for our research.
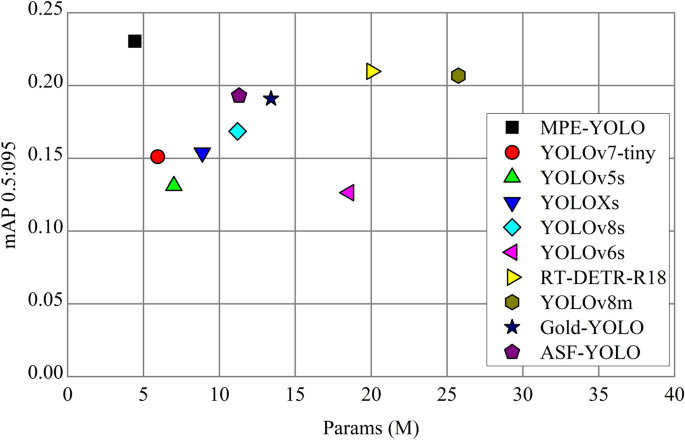
Relationships between the AP50:95 and model parameter count for different models.
Figure 8 shows the relationship between mAP0.5:0.95 and the parameters of each model, where the x-axis represents the parameters of the model and the y-axis represents the detection performance index. As can be seen from the figure, MPE-YOLO achieves an improvement in detection accuracy while maintaining a low weight. Compared to all the comparison models, our model is best suited for drone vehicle inspection tasks.
Generalization study
Through comprehensive comparative tests on two different remote sensing image datasets RSOD and AI-TOD in Table 5 , our MPE-YOLO model demonstrates its superior generalizability. According to these tests, the MPE-YOLO model showed high accuracy in the two key performance indicators of mAP0.5 and mAP0.5:0.95 compared with several existing advanced object detection models, especially on the AI-TOD dataset, for which the average target size was only 12.8 pixels.
The experimental results reveal the strong detection ability of MPE-YOLO, which maintains high accuracy even in small target detection scenarios, confirming its practicability and effectiveness in the field of remote sensing image analysis. These conclusions support the use of the MPE-YOLO model as a remote sensing target detection algorithm with strong adaptability and generalizability, and indicate its broad potential for future practical applications.
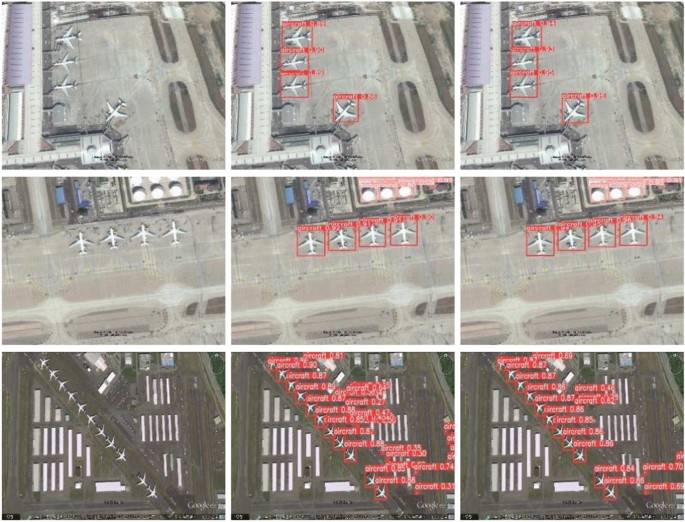
Comparison of YOLOv8(mid)and MPE-YOLO(right)on the RSOD dataset.
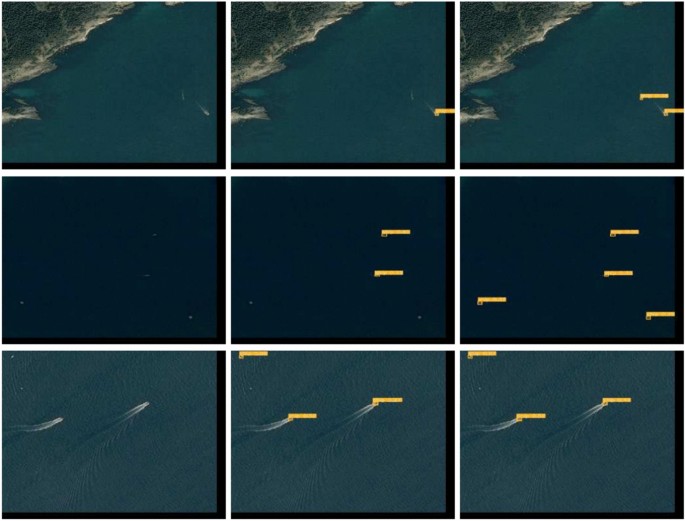
Comparison of YOLOv8(mid)and MPE-YOLO(right)on the AI-TOD dataset.
To more clearly demonstrate the strength of our algorithm in detecting small-sized targets, we selected several representative photographs from both the RSOD and AI-TOD datasets. Figures 9 and 10 show that YOLOv8 has a great number of missed detections on smaller targets than MPE-YOLO, which has significantly fewer missed cases. Additionally, MPE-YOLO shows a general improvement in detection precision. These comparative visuals underscore that MPE-YOLO is a more suitable model for practical detection in aerial imagery applications.
Upon examining these sets of illustrations, it becomes evident that our MPE-YOLO outperforms YOLOv8, especially in scenarios with smaller and easily overlooked targets, reinforcing its efficacy and reliability for deployment in aerial target detection tasks.
Conclusions
In this study, we propose the MPE-YOLO model, which effectively improves the accuracy of small and medium-sized object detection in aerial images, and optimizes the object detection performance in complex environments. First, the MFI module is proposed to effectively improve the efficiency of feature fusion, reduce information loss, and qualitatively improve the detection characteristics of small targets. The PEC module enhances the ability of the network to capture the detailed features of the target, which has a significant effect on the object detection in complex backgrounds. The ES-C2f module further strengthens the feature representation ability of small targets by optimizing the sensing range. The model has been tested on multiple aerial image datasets to confirm its excellent performance, especially in terms of real-time processing power and detection accuracy. Future work will focus on improving the generalization ability of the model and optimizing the operational efficiency, with a view to deploying it in a wider range of practical applications.
Data availability
All the images and experimental test images in this paper were from the open source VisDrone dataset, RSOD dataset and AI-TOD dataset. These datasets analyzed during the current research period can be found at the following website.Visdrone: (https://github.com/VisDrone/VisDrone-Dataset), RSOD: (https://github.com/RSIA-LIESMARS-WHU/RSOD-Dataset-) and AI-TOD :( https://github.com/jwwangchn/AI-TOD).
Liu, H. et al. Improved gbs-yolov5 algorithm based on yolov5 applied to uav intelligent traffic. Sci. Rep. 13 , 9577 (2023).
Article ADS CAS PubMed PubMed Central Google Scholar
Bravo, R. Z. B., Leiras, A. & CyrinoOliveira, F. L. The use of uav s in humanitarian relief. An application of pomdp-based methodology for finding victims. Prod. Oper. Manag. 28 , 421–440 (2019).
Article Google Scholar
Suthaharan, S. & Suthaharan, S. Support vector machine. Machine Learning Models and Algorithms for Big Data Classification: Thinking with Examples for Effective Learning 207–235 (2016).
Biau, G. & Scornet, E. A random forest guided tour. TEST 25 , 197–227 (2016).
Article MathSciNet Google Scholar
Dalal, N. & Triggs, B. Histograms of oriented gradients for human detection. In 2005 IEEE Computer Society Conference on Computer Vision and Pattern Recognition (CVPR’05) Vol. 1, 886–893 (IEEE, 2005).
Girshick, R., Donahue, J., Darrell, T. & Malik, J. Rich feature hierarchies for accurate object detection and semantic segmentation. In Proceedings of the IEEE Conference on Computer Vision and Pattern Recognition 580–587 (2014).
Girshick, R. Fast r-cnn. In Proceedings of the IEEE International Conference on Computer Vision 1440–1448 (2015).
Ren, S., He, K., Girshick, R. & Sun, J. Faster r-cnn: Towards real-time object detection with region proposal networks. Adv. Neural Inform. Process. Syst. 28 (2015).
Liu, W. et al. Ssd: Single shot multibox detector. In Computer Vision-ECCV 2016: 14th European Conference, Amsterdam, The Netherlands, October 11–14, 2016, Proceedings, Part I 14 21–37 (Springer, 2016).
Redmon, J., Divvala, S., Girshick, R. & Farhadi, A. You only look once: Unified, real-time object detection. In Proceedings of the IEEE Conference on Computer Vision and Pattern Recognition 779–788 (2016).
Redmon, J. & Farhadi, A. Yolo9000: Better, faster, stronger. In Proceedings of the IEEE Conference on Computer Vision and Pattern Recognition 6517–6525 (2017).
Redmon, J. & Farhadi, A. Yolov3: An incremental improvement. arXiv preprint arXiv:1804.02767 (2018).
Bochkovskiy, A., Wang, C.-Y. & Liao, H.-Y. M. Yolov4: Optimal speed and accuracy of object detection. arXiv preprint arXiv:2004.10934 (2020).
Glenn, J. Ultralytics yolov5 (2022).
Li, C. et al. Yolov6: A single-stage object detection framework for industrial applications. arXiv preprint arXiv:2209.02976 (2022).
Wang, C.-Y., Bochkovskiy, A. & Liao, H.-Y. M. Yolov7: Trainable bag-of-freebies sets new state-of-the-art for real-time object detectors. In Proceedings of the IEEE/CVF Conference on Computer Vision and Pattern Recognition 7464–7475 (2023).
Glenn, J. Ultralytics yolov8 (2023).
Cheng, G., Si, Y., Hong, H., Yao, X. & Guo, L. Cross-scale feature fusion for object detection in optical remote sensing images. IEEE Geosci. Remote Sens. Lett. 18 , 431–435 (2020).
Article ADS Google Scholar
Guo, J. et al. A new detection algorithm for alien intrusion on highway. Sci. Rep. 13 , 10667 (2023).
Sahin, O. & Ozer, S. Yolodrone: Improved yolo architecture for object detection in drone images. In 2021 44th International Conference on Telecommunications and Signal Processing (TSP) , 361–365 (IEEE, 2021).
Chen, Y., Zheng, W., Zhao, Y., Song, T. H. & Shin, H. Dw-yolo: An efficient object detector for drones and self-driving vehicles. Arab. J. Sci. Eng. 48 , 1427–1436 (2023).
Zhu, X., Lyu, S., Wang, X. & Zhao, Q. Tph-yolov5: Improved yolov5 based on transformer prediction head for object detection on drone-captured scenarios. In Proceedings of the IEEE/CVF International Conference on Computer Vision 2778–2788 (2021).
Yang, Y. Drone-view object detection based on the improved yolov5. In 2022 IEEE International Conference on Electrical Engineering, Big Data and Algorithms (EEBDA) 612–617 (IEEE, 2022).
Lin, Y., Li, J., Shen, S., Wang, H. & Zhou, H. In GDRS-YOLO: More Efficient Multiscale Features Fusion Object Detector for Remote Sensing Images 21 , 1–5 (2024).
Jin, R., Jia, Z., Yin, X., Niu, Y. & Qi., Y. In Domain Feature Decomposition for Efficient Object Detection in Aerial Images Vol. 16, 1626 (2024).
Bai, Z., Liu, Z., Li, G., Ye, L. & Wang, Y. Circular Complement Network for RGB-D Salient Object Detection Vol. 451, 95–106 (Elsevier, 2021).
Google Scholar
Pacal, I. et al. In An efficient real-time colonic polyp detection with YOLO algorithms trained by using negative samples and large datasets 141 , 105031 (2022).
Xu, J., Ren, H., Cai, S. & Zhang, X. An Improved faster R-CNN Algorithm for Assisted Detection of Lung Nodules Vol. 153, 106470 (Elsevier, 2023).
Chen, X., Zheng, H., Tang, H. & Li, F. Multi-Scale Perceptual YOLO for Automatic Detection of Clue Cells and Trichomonas in Fluorescence Microscopic Images 108500 (Elsevier, 2024).
Zhu, H. et al. Development of a PWM Precision Spraying Controller for Unmanned Aerial Vehicles Vol. 7, 276–283 (Elsevier, 2010).
Wang, M., Zang, X.-Z., Fan, J.-Z. & Zhao, J. Biological Jumping Mechanism Analysis and Modeling for Frog Robot Vol. 5, 181–188 (Elsevier, 2008).
Nishiyama, T., Kumagai, A., Kamiya, K. & Takahashi, K. Silu: Strategy involving large-scale unlabeled logs for improving malware detector. In 2020 IEEE Symposium on Computers and Communications (ISCC) 1–7 (IEEE, 2020).
He, K., Zhang, X., Ren, S. & Sun, J. Spatial pyramid pooling in deep convolutional networks for visual recognition. IEEE Trans. Pattern Anal. Mach. Intell. 37 , 1904–1916 (2015).
Article PubMed Google Scholar
Lin, T.-Y. et al. Feature pyramid networks for object detection. In Proceedings of the IEEE Conference on Computer Vision and Pattern Recognition 2117–2125 (2017).
Liu, S., Qi, L., Qin, H., Shi, J. & Jia, J. Path aggregation network for instance segmentation. In Proceedings of the IEEE Conference on Computer Vision and Pattern Recognition 8759–8768 (2018).
Gao, S.-H. et al. Res2net: A new multi-scale backbone architecture. IEEE Trans. Pattern Anal. Mach. Intell. 43 , 652–662 (2019).
Luo, W., Li, Y., Urtasun, R. & Zemel, R. Understanding the effective receptive field in deep convolutional neural networks. Adv. Neural Inform. Process. Syst. 29 (2016).
Du, D. et al. Visdrone-det2019: The vision meets drone object detection in image challenge results. In Proceedings of the IEEE/CVF international conference on computer vision workshops (2019).
Long, Y., Gong, Y., Xiao, Z. & Liu, Q. Accurate object localization in remote sensing images based on convolutional neural networks. IEEE Trans. Geosci. Remote Sens. 55 , 2486–2498 (2017).
Wang, J., Yang, W., Guo, H., Zhang, R. & Xia, G.-S. Tiny object detection in aerial images. In 2020 25th International Conference on Pattern Recognition (ICPR) 3791–3798 (IEEE, 2021).
Ge, Z., Liu, S., Wang, F., Li, Z. & Sun, J. Yolox: Exceeding yolo series in 2021. arXiv preprint arXiv:2107.08430 (2021).
Lv, W. et al. Detrs beat yolos on real-time object detection. arXiv preprint arXiv:2304.08069 (2023).
Wang, C. et al. Gold-yolo: Efficient object detector via gather-and-distribute mechanism. Adv. Neural Inform. Process. Syst. 36 (2024).
Kang, M., Ting, C.-M., Ting, F. & Phan, R. Asf-yolo: A novel yolo model with attentional scale sequence fusion for cell instance segmentation. Image Vis. Comput. 147 , 105057 (2024).
Download references
Acknowledgements
This work was supported by a Grant from the National Natural Science Foundation of China (No.62105093)
Author information
Authors and affiliations.
College of Information Science and Engineering, Hebei University of Science and Technology, Shijiazhuang, 050018, China
Jia Su, Yichang Qin, Ze Jia & Ben Liang
You can also search for this author in PubMed Google Scholar
Contributions
J.S. conceived the experiments, J.S. and Y.Q. conducted the experiments, Z.J. and B.L. analysed the results. Y.Q. wrote the main manuscript text. All authors reviewed the manuscript.
Corresponding author
Correspondence to Yichang Qin .
Additional information
Publisher's note.
Springer Nature remains neutral with regard to jurisdictional claims in published maps and institutional affiliations.
Rights and permissions
Open Access This article is licensed under a Creative Commons Attribution-NonCommercial-NoDerivatives 4.0 International License, which permits any non-commercial use, sharing, distribution and reproduction in any medium or format, as long as you give appropriate credit to the original author(s) and the source, provide a link to the Creative Commons licence, and indicate if you modified the licensed material. You do not have permission under this licence to share adapted material derived from this article or parts of it. The images or other third party material in this article are included in the article’s Creative Commons licence, unless indicated otherwise in a credit line to the material. If material is not included in the article’s Creative Commons licence and your intended use is not permitted by statutory regulation or exceeds the permitted use, you will need to obtain permission directly from the copyright holder. To view a copy of this licence, visit http://creativecommons.org/licenses/by-nc-nd/4.0/ .
Reprints and permissions
About this article
Cite this article.
Su, J., Qin, Y., Jia, Z. et al. MPE-YOLO: enhanced small target detection in aerial imaging. Sci Rep 14 , 17799 (2024). https://doi.org/10.1038/s41598-024-68934-2
Download citation
Received : 29 February 2024
Accepted : 30 July 2024
Published : 01 August 2024
DOI : https://doi.org/10.1038/s41598-024-68934-2
Share this article
Anyone you share the following link with will be able to read this content:
Sorry, a shareable link is not currently available for this article.
Provided by the Springer Nature SharedIt content-sharing initiative
- Object detection
- Aerial image
- Small target
- Model lightweight
By submitting a comment you agree to abide by our Terms and Community Guidelines . If you find something abusive or that does not comply with our terms or guidelines please flag it as inappropriate.
Quick links
- Explore articles by subject
- Guide to authors
- Editorial policies
Sign up for the Nature Briefing newsletter — what matters in science, free to your inbox daily.

- DOI: 10.1117/12.3037673
- Corpus ID: 271833465
Design and implementation of UAV obstacle avoidance based on STM32
- Xusheng Hu , Juan Liu
- Published in International Conference… 9 August 2024
- Engineering, Computer Science
Related Papers
Showing 1 through 3 of 0 Related Papers

COMMENTS
In this paper, we present UAV civil applications and their challenges. We also discuss the current research trends and provide future insights for potential UAV uses. Furthermore, we present the key challenges for UAV civil applications, including charging challenges, collision avoidance and swarming challenges, and networking and security ...
The primary objective of this review paper was to assess recent trends in UAV research over the past three years using the Scopus database as a reliable source. The database was queried using relevant keywords such as "drone", "UAV", "unmanned aerial vehicle", and "unmanned aerial systems".
Unmanned Aerial Vehicle classification, Applications and challenges: A Review. Gaurav Singhal , Babankumar Bansod 1* Lini Ma thew 2, 1 Central Scientific Instruments Organization, Chandigarh ...
The research direction in aforementioned areas includes air-to-ground modeling, optimal deployment of UAVs as BSs, optimization of UAV trajectory, analyzing the performance of UAV-enabled wireless networks, cellular network planning with UAVs, drone UEs in wireless networks, resource management, interference mitigation, collision avoidance, and ...
The growing interest in unmanned aerial vehicles (UAVs) from both scientific and industrial sectors has attracted a wave of new researchers and substantial investments in this expansive field. However, due to the wide range of topics and subdomains within UAV research, newcomers may find themselves overwhelmed by the numerous options available. It is therefore crucial for those involved in UAV ...
4.3 Domains of UAV Research. Based on the survey conducted on various research papers on the topic of UAV across the globe and from top international and national journals, conferences it is observed that the current trend is mainly focusing in the field of UAV's. The top most categories or domains concentrated by researchers are shown in Fig ...
This paper is devoted to providing a brief review of the UAV control issues, including motion equations, various classical and advanced control approaches. The basic ideas, applicable conditions, advantages and disadvantages of these control approaches are illustrated and discussed. Some challenging topics and future research directions are raised.
Recently, unmanned aerial vehicles (UAVs) or drones have emerged as a ubiquitous and integral part of our society. They appear in great diversity in a multiplicity of applications for economic, commercial, leisure, military and academic purposes. The drone industry has seen a sharp uptake in the last decade as a model to manufacture and deliver convergence, offering synergy by incorporating ...
This paper investigates the path planning problem of an unmanned aerial vehicle (UAV) for completing a raid mission through ultra-low altitude flight in complex environments. The UAV needs to avoid radar detection areas, low-altitude static obstacles, and low-altitude dynamic obstacles during the flight process.
1.2. Contributions of this article. Despite the existing UAV communication related articles highlighted in Section 1.1, no contributions have been reported in providing a comprehensive review of the emerging technologies in UAV communication.Therefore, our objective in this paper is to focus more on emerging UAV communication technologies and their applications for the next-generation wireless ...
1. Introduction. Unmanned Aerial Vehicles are becoming popular and are being used for various applications at an accelerating rate [1], [2].UAVs are deployed across various sectors including logistics [3], [4], agriculture [5], remote sensing [6], wireless hotspot services [7], smart city applications [8] and disaster management [9].Notably, UAVs are being used for crowd surveillance, public ...
Unmanned Aerial Vehicles (UAVs) are now used in multiple sectors for a vast array of purposes. These vehicles working in swarms can be used for reconnaissance, search and rescue, photography, and crop monitoring. In addition, the versatility of UAVs is highly utilized by several governments to play an integral role in the defense of a country. This survey paper provides a comprehensive study ...
Abstract. Unmanned Aerial Vehicles (UAVs) have been widely used both in military and civil across the world in recent years. Nevertheless, their design always involves complex design optimization variables and decisions. Therefore, this paper aims to guide through the designing, manufacturing, and testing of an electrically powered radio ...
European Transport Research Review 11, 1 (2019 ... Clarisse Musanabaganwa, Sabin Nsanzimana, and Michael R. Law. 2022. Effect of unmanned aerial vehicle (drone) delivery on blood product delivery time and wastage in Rwanda: a retrospective, cross-sectional study and time series analysis. ... In this paper, we formalize MiKe as the problem of ...
A Review of Unmanned Aerial Vehicle (UAV): Its Impact and Challenges, ICAENS 2022, Konya, Turkey . 86 . spy. Since UAVs can seize accurate data, the y ca n . ... In this research paper, we ...
This paper focuses on the aerodynamics and design of an unmanned aerial vehicle (UAV) based on solar cells as a main power source. ... in this sense, the challenge is to create an aerodynamic design that can increase the endurance of the UAV. In this research, the flight mission starts with the attempt of the vehicle design to get at the ...
The velocity obstacles (VO) method is widely employed in real-time obstacle avoidance research for UAVs due to its succinct mathematical foundation and rapid, dynamic planning abilities. Traditionally, VO assumes a circle protection domain with a fixed radius, leading to issues such as excessive conservatism of obstacle avoidance areas, longer detour paths, and unnecessary avoidance angles. To ...
The aim of this paper is to systematically review the literature to provide pertinent information on UAVs' applications among the association of southeast Asian nations (ASEAN) countries by reviewing 179 documents published from 2012 to the end of 2023. ... The results of the research demonstrate the state of UAV adoption, application areas ...
Organization of the paper. ... made work item (WI) Y.UAV.arch so that unmanned aerial vehicles (UAVs) and unmanned aerial vehicle controllers (UAVs) can have a stable and functional architecture over IMT-2020 networks . IMT-2020 is employed for UAV communication. ... we present several future research directions for further contributions on UAV ...
In the current paper we review the current situation in military communications utilizing UAV platforms, and we also explore its future trends and capabilities taking into consideration the parallel developments in the civil UAV manufacturing sector involving UAV swarms and fifth-generation networks. ... proceedings of the 18th international ...
Domains of UAV Research. Based on the survey conducted on various research papers on the topic of UAV across the globe and from top international and national journals, conferences it is observed that the current trend is mainly focusing in the field of UAV's. The top most categories or domains concentrated by researchers are shown in Fig. 22.
The paper is organized as follows: an introduction that covers types of unmanned vehicles, UUV support technology, research contributions, and the state of the art. This is followed by a discussion of related works, including research questions, existing surveys, and statistical trends.
kala T aluk, Udupi 574110, India. *Corresponding Author Email: [email protected]. ABSTRACT. The modern designs and their developments are essential based on their application; hence, UAV's can be ...
Over the past decade, unmanned aerial vehicle (UAV) technologies have advanced rapidly and gained worldwide interest for their numerous applications across a wide range of fields. ... Some research papers in the literature have discussed UAV detection and localization, but none of them focused on all radar-based techniques for UAV detection and ...
Unmanned Aerial Vehicle Advanced Concept Technology Demonstrator (HAE UAV ACTD) program managed by the Defense Advanced Research Projects Agency (DARPA) and Defense Airborne Reconnaissance Office (DARO) [3]. This ACTD placed the base for the improvement of the Global Hawk. The Global Hawk hovers at heights up to 65,000 feet and flying duration ...
The rest of this paper includes the following content: Section 2 briefly introduces the recent research results on aerial image detection and the main idea of YOLOv8. Section 3 introduces the ...
Praveen Ekka. Abstract: Unmanned Aerial Vehicle (UAV) is commonly known as Drone.It is extensively being used these years.Nowdays drones are used in various Military applications, Commercial Cargo Transport, and 3-D Mapping etc. For supporting the weight of the plane, and shock absorption functions, landing gear design is highly needed.Unmanned ...
Semantic Scholar extracted view of "Design and implementation of UAV obstacle avoidance based on STM32" by Xusheng Hu et al. ... Semantic Scholar's Logo. Search 220,313,689 papers from all fields of science. Search. Sign In Create Free Account. DOI: 10.1117/12. ... AI-powered research tool for scientific literature, based at Ai2. Learn More.